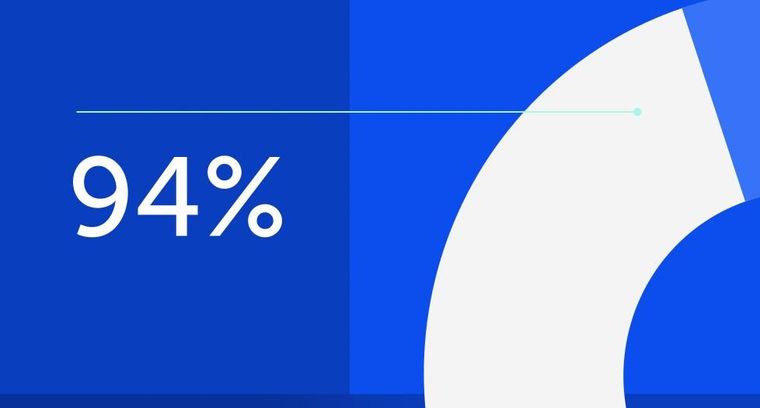
94% of researchers rate our articles as excellent or good
Learn more about the work of our research integrity team to safeguard the quality of each article we publish.
Find out more
ORIGINAL RESEARCH article
Front. Built Environ., 08 April 2025
Sec. Construction Materials
Volume 11 - 2025 | https://doi.org/10.3389/fbuil.2025.1558394
This article is part of the Research TopicInnovative Materials and Techniques for Sustainable ConstructionView all 3 articles
A novel form of high-tech concrete known basalt fiber-reinforced high-performance concrete (BFHPC) has been developed using traditional materials that require extra admixtures to improve its mechanical properties. Machine learning (ML) techniques provide a more flexible and economical way to predict the mechanical property of chopped and minibar basalt fiber-reinforced high-performance concrete based on material properties and processing parameters, enabling durable and environmentally friendly construction. Predicting the mechanical properties of BFHPC precisely is crucial since it reduces design costs and time, and it also minimizes material waste from several mixing experiments. In this study, the compressive strength and flexural strength are predicted via different types of machine learning models. Experiments carried out in the laboratory under standard controlled settings at 7, 14, and 28-day curing periods yielded sample data for analysis and model development. The mechanical characteristics of BFHPC have been predicted using a combination of decision tree, partial least squares, lasso, rigid, random forest regressor, K Neighbours, and linear regressions. According to the results, all types of regression have the best results except KNeighbors Regressor, Random Forest Regressor, and Lasso Regression, with a correlation coefficient of R2 93%. Each model’s performance and application are examined thoroughly, leading to the identification of useful suggestions, existing knowledge gaps, and areas in need of further study.
The production of high-performance concrete (HPC) is a novel form of high-tech concrete that is made with traditional materials and requires additional admixtures to enhance the concrete’s mechanical properties and add high durability, workability, and volumetric stability. Due to its long lifespan and safety features for building structures, HPC is commonly employed in civil engineering projects (Hematibahar et al., 2023; Hematibahar et al., 2024; Chiadighikaobi et al., 2024a). Numerous researches testify to its exceptional performance. Since basalt fiber resists heat, alkali reactions, and corrosion in concrete, its application in building materials is advantageous. Chopped basalt fiber (BF) is a sustainable alternative to carbon fiber because of its improved properties and sustainable production method. Although the production of BF is comparable to that of glass fibers, the absence of chemical additions and low energy consumption make it better for the environment (Wei et al., 2010; Zhao et al., 2022). Due to its single processing method, BF is less expensive than other fibers. Other applications for BF include electrical insulation, automotive parts, sports equipment, fire protection, and so on. In addition, its application in cementitious composites is still in its infancy. The increase in the number of publications over the past 10 years gives the impression of a growing interest in the application of BF to various fields. Summarizes the increasing interest in the use of BF for various purposes. Due to its improved mechanical properties, chemical and thermal stability, sustainability, and other attributes, BF saw an increase in usage (Sim et al., 2005; Jiang et al., 2014; Sondarva and Bhogayata, 2017; Niu et al., 2019).
Enhancing the tensile strength, flexural strength, and durability of High-Performance concrete is another reason to add basalt fibers to it (Ayub et al., 2014b; Kharun and Koroteev, 2018). Their findings demonstrated that the high-strength concrete with basalt fibers was more durable than standard high-strength concrete. Basalt fibers are added To increase the effectiveness of the High-Performance Concrete (HPC) in this case,. The effects of introducing basalt fibers are demonstrated by the greatly improved flexural and tensile strength of HPC (Chiadighikaobi et al., 2021; Lam and Hung, 2021). Basalt fibers offer advantages on the financial front in addition to their mechanical qualities (Hematibahar, 2021). The addition of a portion of 1.2% basalt filaments had a substantial impact on the compressive, tractable, and flexural strength of Superior Execution Concrete despite the High-Performance Concrete containing varied amounts of basalt fiber (0.4%–1.8%) (Ayub et al., 2014a). The addition of basalt fibers to high-performance concrete through a mixture of metacoaline and silica fume was investigated; the results show that basalt fibers increase the durability of the concrete.
Moreover, silica fume can be used to fill the spaces between the cementous matrix and basalt fiber (Mohaghegh et al., 2018). Analysis was done on the addition of various percentages of basalt fibers to high-performance concrete (Canbaz et al., 2022). It was determined that 1.33% of the basalt fibre had the biggest influence on the mechanical properties. In High-Performance Concrete, more than 1.2% of Basal Fibres are generally productive in terms of compressive, tensile, and flexural strength. As an illustration, research has been done on glass fiber’s effects on concrete (Farooq et al., 2021). In concrete, the tensile and compressive strengths were found to be positively impacted by the length of the fiber rather than the proportion of fiber weight. In this study, a 100 mm-cubic sample is subjected to the compression test in accordance with GOST (Russian Standard Code Design) and ASTM (American Society for Testing and Materials) guidelines (GOST 24452-80 Betony, 2005; ASTM C. 109, 2017). Many studies attempt to predict the mechanical properties of Ultra-High-Performance Concrete (UHPC). As an example, (Wakjira et al., 2024), envisioned sustainable economic and environmental (UHPC). They used tree- and reinforcement-based machine learning (ML) models that also predict compressive strength. In another example, (Altayeb et al., 2024), used artificial intelligence (AI) agents that use deep reinforcement learning (DRL) to minimize the cost of UHPC mixes. They found that the AI Agent at 221 MPa reduced more than 1,272 USD per cubic meter. (Guo et al., 2023). forcast the carbon footprint, and compressive strength of UHPC via machine learning. They found the minimum effect of UHPC via mechanical propertis. In another example, (Mahjoubi et al., 2023), predicted the carbon footprint of compressive strength, flexural strength, mini-slump expansion and porosity of UHPC through AI-guided approaches. (Mahjoubi et al., 2022). predict the predicting compressive strength, flexural strength, workability, and porosity of ultra-high-performance concrete (UHPC) through learning framework and Light Gradient Boosting Machine (LightGBM).
Many studies investigated the impact of machine learning on civil engineering and mechanical properties of special building materials. For example, (Hematibahar et al., 2024) analyzed predict the high-performance concrete and ultra-high-perfomance concrete mechanical properties through Linear, Ridge, Lasso, Random Forest, K-Nearest Neighbors (KNN), Decision tree, and Partial least squares (PLS) regressions. They found that the best result is for PLS regression with more than 93% R2. Another examples is for tensile strength predicted via logistic algorithm. The results show that R2 is more than 93% (Hematibar et al., 2024). In the terms of predict the concrete mixture design, Linear Regression (LR), Ridge Regression (RR), Support Vector Machine Regression (SVR) and Polynomial Regression (PR) has been applied. The best results was 93% for Polynomial Regression (Hematibahar and Kharun, 2024). N. Beskopylny et al. predicted concrete compressive strength via machine learning. They using CatBoost, k-Nearest Neighbors, Support Vector regressions. They found that the best result is for KNN-regression, with 99% R2 (Beskopylny et al., 2022). In another example, concrete properties via vibro-centrifuged variatropic have been predicted through ridge regression, decision tree and extreme gradient boosting (XGBoost), finally R2 is 93% (Beskopylny et al., 2024).
The standard destructive test is the most reliable method to assess the compressive strength of concrete in cast-in-place or pre-existing structures. Ordinarily, conventional cylindrical or cubic samples are considered in the lab for analysis of their compressive strength. Nevertheless, a number of variables, including the kind and size of the cement and aggregate, the fine aggregate modulus, the water-to-cement ratio, the interfacial transition zone, etc., may not have been taken into account, meaning that the test results might not be in detail. Moreover, using the destructive evaluation approach on existing concrete members at the site is inconvenient, and there is a risk that the concrete member will be damaged in the course of the procedure. In this sense, using nondestructive evaluation (NDE) methods to evaluate the concrete strength is an acceptable option when core sampling is not appropriate (Khan et al., 2021). The process of determining concrete strength depends typically on several empirical relationships between the test parameter and the nondestructive variables. Therefore, more steps are required for nondestructive variables, such as the conventional regression analysis method. Computer prediction techniques can reduce resources and time.
Additionally, by employing classical programming, equations and algorithms can be converted from classical programming to commuter programs (Erdal, 2013; Akande et al., 2014; Agrawal, 2022; AlAlaween et al., 2022, p. 3; Khorasani et al., 2022; Peng et al., 2022; Shahmansouri et al., 2022; Yang et al., 2022). For the purpose of forecasting concrete compressive strength at various curing time ages, an algorithm programming technique was created (Chopra et al., 2016). The article concluded that, for all curing period ages, the compressive strength prediction findings of the created algorithm were precise and closely matched the results of laboratory experiments. Additionally, taking into account mixed design properties, ANNs are used as a mechanical property prediction method (Khademi and Behfarnia, 2016). The findings demonstrated that the ANNs’ prediction was reasonable and that the outcomes were nearly identical to the actual experimental findings. Studies were conducted to determine the concrete strength of the initial concrete mixture using the Multi-Linear Regression (MLR) approach (Topçu and Sarıdemir, 2007). The findings demonstrated the efficacy of the MLR estimate method for the first concrete. The form and size of the dry aggregate were taken into consideration when studying the data mining approach for determining the compressive strength of concrete (Kashyzadeh et al., 2022). The study discovered that the key factors influencing compressive strength included the aggregate form and size.
Many studies have used computer modeling and machine learning to predict the properties and mechanical properties of concrete (Dai et al., 2022; Tian et al., 2024; Zhou et al., 2024). Moreover, the ANNs programming technique was used to forecast mechanical characteristics and comprehend the connection between the compressive strength of concrete and the cement-water ratio. Thus, the ability of ANNs and FL techniques to predict a concrete mixture with more fly ash was independently studied (Kaplan et al., 2019). The findings indicate that the coefficient of determination (R2) value, which was equivalent to 0.90, increased when the attention was placed on aggregate diameter as a predictor of the mechanical properties of concrete. Based on the mechanical characteristics of the aggregate, data mining was used to forecast the mechanical properties of the concrete (Kashyzadeh et al., 2022). The outcome demonstrates how aggregate property data mining features influence compressive strength. Due to the design code, which usually needs certain features, it is not easy to forecast the mechanical properties of concrete with accuracy. The machine learning (ML) techniques include ensemble learning (EL), support vector machine regression (SVMR), and Gaussian progress regression (GPR) to predict the mechanical properties of lightweight concrete. The study found that the GPR predicting R2 was 0.98. In a different instance, the compressive strength and compressive stress-strain of concrete were predicted using classical programming, and the outcomes indicate that R2 was greater than 0.97 (Hasanzadeh et al., 2022). Polynomial Regression (PR) may predict mechanical qualities (R2 > 0.99), according to research that used machine learning to predict the mechanical properties of concrete. Hematibahar et al. analyzed different machine learning models to predict High-Performance and Ultra-High-Performance concrete mechanical properties. The study finds that a model to predict mechanical properties is for Partial least squares (PLS) regression with more than 93% for the coefficient of determination (R2). (Chiadighikaobi et al., 2023). analyzed the historical structure design with data analysis and soft programming. The result shows that R2 was more than 0.44. In another example, (Ly et al., 2021), predicted load-carrying capacity of concrete-filled steel tubes (CFST) via artificial intelligence (AI) algorithms. They used adaptive neuro-fuzzy inference system (ANFIS) to predict CFST. They understand that R2 is 0.94 when they used ANFIS model. (Asteris et al., 2021a). analysis the prediction of compressive strength of concrete via machine learning. They used more than 1,030 concrete compressive strength recods. They used onventional machine learning (CML) models, namely, Artificial Neural Network (ANN), Linear and Non-Linear Multivariate Adaptive Regression Splines (MARS-L and MARS-C), Gaussian Process Regression (GPR), and Minimax Probability Machine Regression (MPMR) to predict compressive strength of concrete. In another example (Armaghani et al., 2021), predict the compressive strength of granite through 182 data sets of non-destructive tests reported in the literature. They predict 70% accuracy of compressive strength (Asteris et al., 2021b). predict rectangular concrete-filled steel tubes (CFST) through a very new optimization technique and artificial neural network (ANN). They used a database of 422. They found that the ANN-BCMO model is an optimal model for predicting rectangular CFST. In another example (Kardani et al., 2022), forcast the thermal conductivity of unsaturated soils via propose hybrid adaptive neuro swarm intelligence (HANSI) techniques. They used artificial neural networks (ANNs) and particle swarm optimisation (PSO), finally, they found that R2 is more than 0.94. Moreover, (Cavaleri et al., 2022), predicted the bond strength of reinforced concrete through machine learning. They showed that seven parameters including bar diameter, ratio of concrete cover to diameter of rebar, ratio of diameter of rebar to embedment length, ratio of transverse reinforcement, yield strength, compressive strength of concrete and corrosion level are considered as inputs. They understand that the R2 is more than 0.75.
In order to forecast the mechanical properties of cement, the current study used different types of machine learning (ML) to predict the best mechanical properties. The foundation of the continuing review was the chopped and minibar basalt fiber high-performance concrete (BFHPC). The BFHPC mechanical properties are predicted using a Logistic Function. The compressive strengths of HPC comprising have been estimated using linear, lasso, rigid, random forest regressor, K Neighbors, decision tree, and partial least squares regressions. Furthermore, the correlation coefficient (R2), mean absolute errors (MAE), and root mean squared errors (RMSE) have all been used to validate the prediction models.
The conducted tests have presented the compressive strength results of high-performance concrete of basalt fibers BFHPC. The HPC study’s primary objective was to determine the most appropriate and stable percentages of minibar basalt fiber and additional glass fiber. However, the addition of basalt fibers to High-Performance Concrete increased the concrete’s tensile, flexural, and durability. However, the mechanical properties of High-Performance Concrete were significantly durable. The compression test is performed on a 100 mm-cubic sample using the ASTM (American Society for Testing and Materials) and GOST (Russian Standard Code Design) standards. Three different samples are used every day for the seven-, fourteen-, and 28-day compressive strength tests. An SB mini 133-L mixer is used to mix the two kinds of aggregates for about 2 minutes according to the concrete mixture design method. After that, water is added to the cement. After the chemical powders have been added, the concrete is mixed for approximately 2 minutes later. Figure 1a shows that the material was mixed using the Concrete pan mixers at a steady 48 rpm. Figure 1b shows that the concrete mix is placed on 100 mm cubic concrete formworks so that it is ready for moulding. After the moulding procedure, the concrete cube sample is submerged at 20°C for 48 h. Following that, the samples are stored for 7, 14, and 28 days at 15°C in a moist cabinet for the curing phase, as Figure 1c) shows. Lastly, the cubes are cleaned before the compression test. Cleaning the cubes will yield the most accurate and optimal compressive strength findings (Figure 1d). The materials mixture is Micro silica MK-85 was produced by Novolipetsk Steel company (NLMK), quartz flour produced by SIBELCO Russia company, superplasticizer was admixture based on Polycarboxylic Ether (Glenium 115), crushed granite with fractions of 5–20 mm were used in this experiment, quartz sand a fineness modulus of 0.8–2.7 mm, Portland Cement M500 D0 (CEM I 42.5 N) Cement by South Ural mining and processing company in Russia, tap water, and basalt fiber (chopped and minibars as shown in Table 2) for BFHPC.
Figure 1. Test equipment for preparing the mixing and molding process. (a) Pan Mixer, (b) Concrete formwork, (c) Moist Cabinet, (d) Samples cleaning process.
Additionally, silica fume is added to the concrete to close gaps between the basalt fibers and the cementitious matrix. The inclusion of basalt fibers in the mixture improves the flexural and tensile strengths of High-Performance Concrete. Table 1 displays the Basalt Fibre High-Performance Concrete Mixture utilized in this investigation.
The flexural and tensile strengths of high-performance concrete are enhanced despite the basalt fiber’s reduction of the material’s compressive strength. Compared to other concrete combinations, the mechanical qualities of Basalt Fibre High-Performance Concrete (BFHPC-12), which had 1.2% of basalt fiber added, were more resilient. The mechanical characteristics of minibars basalt fiber high-performance concrete and glass fiber are displayed in Figures 2, 3, respectively.
Due to the use of a low water-to-binder ratio and a high volume of fine particles (cement volume), high-performance concrete (HPC) is more durable than conventional concrete. Moreover, silica fumes or micro silica play a durable and void filler role in concrete. Basalt fiber has been added to increase the mechanical properties and durability. Previous experiments have shown that basalt fiber can help improve the mechanical properties of concrete (Chiadighikaobi et al., 2021). The workability of cementitious mixes is important for their appropriate placement and composite performance. The addition of fibers to cementitious mixes diminishes their workability, which decreases with increasing dose and fiber length. The high water uptake of the basalt fiber, resulting in limited water for workability, could be linked to the decrease in slump of the concrete mixes with the inclusion of the basalt fiber (Vatin et al., 2024).
Several results have been reached on the influence of basalt fiber on the compressive strength of cementitious composites. Many studies have demonstrated that adding a specific amount of basalt fiber to cementitious composites can increase their compressive strength. Other investigations have found that the compressive strength of cementitious composites containing basalt fiber is decreased for all doses when compared to the control (Kharun et al., 2022). The current study has shown that 1.2% of glass fiber and 0.9% of minibar basalt fibers were optimal percentages of maximum compressive strength of concrete (Hematibahar et al., 2022; Vatin et al., 2024).
Adding basalt fiber to HPC can reduce the slump, for example, (Niu et al., 2019), understand that when dosage of basalt fiber in HPC increase the slump decerased. They found that control sample of HPC slump is 182 mm, while slump of HPC reinforced with 0.20% was 63 mm. The most effect of basalt fiber to reinforced HPC was improvement of tensile and flexural strengths. Algin and Ozen (Algin and Ozen, 2018) understand when basalt fiber added to concrete (0%–1.5%) tensile strength increase (10 MPa–13 MPa). In another example, (Guo et al., 2019), reinforced concrete via basalt fiber. They found that the flexural strength increased by more than 48% by adding 0.6% basalt fibers to concrete. While reinforced concrete with basalt fibers can significantly improve the flexural and tensile strength, other types of fibers cannot affect the flexural and tensile strength of concrete as much as reinforced basalt fibers (Momeni et al., 2024). Also, sometimes reinforced concrete with a 3D printed structure does not have a great effect like adding special fibers to basalt fibers. The biggest difference between reinforced concrete through basalt fibers and other types such as 3D printed structures is in the change of soft and hard softening (Hematibahar et al., 2023).
The current study was based on the experimental results of high-performance concrete reinforced basalt fiber (Hematibahar, 2021). Equation 4 was found based on previous studies and experimental studies on the HPC (Hasanzadeh et al., 2022). Next, the compressive strength was verified through Correlation Coefficient (R2), Mean Absolute Errors (MAE), and Root Mean Squared Errors (RMSE). According to this study, the linear, rigid, and polynomial regressions have been investigated to predict the compressive strength of BFHPC. The regressions have been applied by the Skylearn library in Python programming language to establish the machine learning method. Moreover, the failure of concrete has been investigated in the current study. First, the equations have been derived into Python through machine learning.
The Linear Regression (LR) Equations are defined (Khademi and Behfarnia, 2016): Ridge Regression is a type of regression that can analyze multiple data with multicollinearity. Moreover, by adding a degree of bias to the regression estimates, Ridge Regression illustrated in Equation 1 decreases errors and obtains more accuracy (Abhishek, 2021; Enwere et al., 2023):
where v is the dependent variable, w is the independent variable, δ0 is the y-intercept and δ1 is the regression coefficient representing the change in v concerning the change in w, also called the slope, and λ is the Ridge Regression penalty ratio, ε is the error term, ∑(
where v is the dependent variable, w is the independent variable, δ0 is the y-intercept, and δ1 is the regression coefficient representing the change in v concerning the change in w, also called the slope. Moreover, the λ is the regularization parameter, ε is the error term,
Figure 4. Random forest scheme (based on Qureshi et al., 2023) Partial least squares (PLS) is multiple linear regression modeling based on simulating predictors and observable and transfer data to new space (based on Rong et al., 2024).
K-Nearest Neighbors (KNN) is commonly used for classification, although the regression algorithm of KNN is also used as a prediction method. In the KNN prediction algorithm:Training example as
A decision tree is used for both classification and regression algorithms (Myles et al., 2004), and the direct node includes root nodes, inner nodes, and leaf nodes as a simple algorithm. Commonly, nodes in the direct tree algorithm predict a data category or direct data. Finally, the operation process multiplies judgments to predict values with different characteristics (Wang et al., 2024).
Applied ML models were designed to fit the selected data set and desired results. This section presents the hyperparameters and parameter settings for each ML model. Hyperparameter optimization is the most important factor to minimize the overfitting problem. In this case, the final parameter values are selected through Optuna (Wakjira et al., 2022). Table 3–5 show the hyperparameter tuning of linear, lasso and Ridge Regressions respectively.
According to Table 7, λ is 5 and min-sample-split is 15 for hyperparameter tuning of Ridge Regression model, Table 6 shows that max-depth is 5, n-estimators is 15 and min-sample-split is 18 for forest regressor model.
Table 7–9 illustrate the hyperparameter tuning of decision tree regression model, K Neighbors regression model and partial least squares regressions model respectively.
To find the verified prediction, the correlation coefficient (R2) as expressed in Equation 3, mean absolute errors (MAE), and root mean squared errors (RMSE) have been established.
where y,
RMSE calculates the average deviation of each actual data point and the predicted results. (Equation 5):
Figure 5 shows database condition of test, train and prediction. According to Figure 5, test, train and prediction have been illustrated to each all regression types.
Figure 5. Test and train results of machine learning; (a) Linear (b) Lasso, (c) Ridge, (d) K Neighbors, (e) decision tree (f) partial least squares regressions.
According to machine learning results, Figure 6 shows the current study heatmap. This heatmap illustrates basalt fiber had a negative effect on compressive and flexural strengths. Moreover, the compressive strength of chopped BFHPC had a negative effect on flexural strength, too. Figure 6 demonstrates that the compressive strength of Minibars BFHPC has a positive effect on the flexural strength of Minibars BFHPC. The main resoan of negative correlation between basalt fiber and other elements are related to compressive strength.
Figuring out which dimension to ignore and which is more prone to error is made easier by looking at the Pair Plot graph for feature correlation information across multiple dimensions (Elhishi et al., 2023). According to Figure 7.
• Basalt fiber and compressive strength for chopped BFHPC do not have any correlation with other flexural strengths and compressive strengths.
• Compressive strength of Minibars BFHPC and flexural strength of chopped and minibar
• BFHPC also has the most correlation with other elements.
Figure 8 and Table 10 show regression results. According to Table 10, all regression types had good results with high R2, RMSE and MAE, but some regressions have better results than others. For example, Decision Tree Regressor, Random Forest Regressor, and Ridge Regression had maximum R2 (0.99). While, Lasso Regression, Random Forest Regressor, and KNeighbors Regressor had R2 0.93, 0.98, and 0.98, respectively. Overall, regression results in Figure 8 and Table 10 illustate that the experimental and prediction results are similar. According to the different meta-parameters and different equations of each type of regression, the results of the regressions are different.
Figure 8. The regression of chopped and Minibar BFHPC (MPa) (a), Linear Regression; (b), Lasso Regression; (c), Ridge Regression, (d), Random forest Regression, (e), K-Nearest Neighbors (KNN) Regression; (f), Decision tree Regression (g), Partial least squares (PLS) Regression.
Miao et al. (2024) predict the elasticity modulus, compressive strength and tensile strength via hybrid machine learning algorithms. They found that the best R2 is 0.93for SSA-XGB hybrid prediction algorithm. In another example, (Matthews et al., 2024), predicted the reinforced concrete beam. They examined more than 804 databases to find optimal prediction results. They used radiation boosting regression trees and random forests and consistently produced the best predictions. Their results for R2 GBRT algorithm is more than 0.96. (Shu et al., 2024). analyzed more than 372 database to predict the shear strength via advanced machine learning (ML) models. They found that Explainable Boosting Machine (EBM) with R2 more than 0.92 is the best model with high results. Among these studies and results, the results of the present study show better results than other studies with R2 greater than 0.99 for linear regression, ridge regression, decision tree regression, and PLS regression. There are other types of regression such as Extended Support Vector Regression Method (XSVR), this algorithm can improve to achive the better results than Support Vector Regression Method (SVR) (Yu et al., 2020a; Yu et al., 2020b) developed an algorithm for XSVR method to analyze hydrated cement in acidic environment. They found that the XSVR algorithm is highly efficient with an R2 greater than 0.99. In anther example, (Wang et al., 2022), examined a new algorithim based on the SVR method called Twin Extended Support Vector Regression (T-X-SVR). T-X-SVR is suitable for engineering applications where stability and robustness are used for machine learning techniques. Furthermore, they found that the accuracy of this regression was significantly high. Algorithms. Although T-X-SVR, X-SVR and SVR have high accuracy, this study prefers to focus on chopped and minibars in high-performance concrete through machine learning instead of using different types of regression. But according to the current study, Linear, Ridge and PLS regressions are very accurate and there is no need to use other types of regression.
In this study, the mechanical properties of HPC reinforced with chopped basalt fibers and mini-bars were predicted using machine learning. Unlike traditional methods that are usually based on analytical relationships and empirical models, this study used advanced machine learning models to improve the accuracy of predicting the compressive and flexural strengths of HPC. Traditional methods, such as linear regression, require strict assumptions about the data distribution and relationships between variables, while machine learning allows for the investigation of complex and nonlinear patterns without the need for these assumptions. One of the key differences between traditional methods and machine learning models is the way they process data and the level of prediction accuracy. In conventional methods, such as linear regression and classical models, the relationships between variables are defined as linear and the effect of each parameter is examined independently. However, in machine learning methods, such as random forest and PLS regression, the model is able to identify complex interactions between different parameters and significantly increase the prediction accuracy. This capability leads to more stable and accurate predictions, especially in situations where laboratory data is noisy or uncertain.
In this paper, comparisons are made between traditional methods and machine-based methods for predicting the mechanical properties of HPC. Conventional mathematical methods are usually based on mathematical models and empirical relationships that use analytical relationships to estimate the compressive and flexural strengths of concrete. These methods, such as linear regression, ridge regression, and lasso regression, assume that the independent and dependent variables are linear or quasi-linear. One of the main challenges of these methods is that they do not provide sufficient accuracy in the complexity of the relationships between the two. Also, these methods are subject to assumptions such as the distribution of data software and independent dependent variables that may exist under certain conditions. In contrast, machine-based methods, such as forest networks, PLS regression, and artificial neural models, are able to identify complex and nonlinear relationships. These models can use a large amount of information to make more accurate predictions without having to make strict assumptions about the distribution of the data. One of the main advantages of HPC is that it can handle multiple variables and is better at modeling the compressive and flexural strengths than other models. This feature leads to more stable and reliable predictions, especially when the inputs are noisy or measurements are made with lower precision. In addition, while traditional methods are often developed based on limited data obtained from controlled experiments, machine learning models can identify hidden dependencies and have more generalizable performance by processing a large amount of data. Specifically, in this study, it was observed that machine learning models such as linear regression, ridge regression, and PLS were able to predict HPC compressive strength with an accuracy of more than 99%, while traditional methods such as lasso regression and simple linear models had lower accuracy. These results demonstrate the superiority of machine learning methods in analyzing and predicting the mechanical properties of HPC.
The results of this study show that machine learning can be used as a powerful tool in predicting the mechanical properties of high-performance concrete (HPC). However, it should be noted that machine learning models cannot completely replace physical tests, but rather act as a complementary method to reduce the number of tests, save time and costs, and optimize concrete mix design. In this study, the results of machine learning models are evaluated and validated using experimental data obtained from standard tests at ages of 7, 14, and 28 days. The high values of correlation coefficient (R2 > 0.99) and the comparison of mean square error (MAE and RMSE) between predicted and actual values indicate the accuracy and reliability of the proposed method. However, in practical and applied projects, it is still necessary to conduct experimental tests and physical investigations to ensure the mechanical performance of concrete under different environmental conditions. Machine learning models can be a complementary tool to accelerate the development and design of new concretes, but the ultimate accuracy of predictions needs to be validated through standardized testing. Future research could focus on developing larger databases and integrating laboratory data with more advanced machine learning models to increase the accuracy and generalizability of these models to real-world construction conditions.
To further enhance the prediction accuracy of mechanical properties in basalt fiber-reinforced high-performance concrete (BFHPC), hybrid modeling techniques could be explored. Combining multiple machine learning algorithms, such as integrating neural networks with ensemble methods (e.g., gradient boosting or random forests), may capture complex nonlinear relationships and interactions between material variables more effectively. For instance, a hybrid approach like ANN-SVM (Artificial Neural Network-Support Vector Machine) or XGBoost combined with feature selection techniques could improve robustness and reduce overfitting. Additionally, incorporating physics-based models with data-driven ML could bridge gaps where experimental data is limited, ensuring predictions align with material science principles. Such hybrid strategies could achieve higher R2 values, lower RMSE, and better generalization across diverse concrete mixtures, advancing the reliability of predictive tools in civil engineering.
Hybrid modeling approaches could be investigated to further improve the mechanical property prediction precision in basalt fiber-reinforced high-performance concrete (BFHPC). Combining multiple machine learning algorithms, such as integrating neural networks with ensemble methods (e.g., gradient boosting or random forests), may capture complex nonlinear relationships and interactions between material variables more effectively. For instance, a hybrid approach like ANN-SVM (Artificial Neural Network-Support Vector Machine) or XGBoost combined with feature selection techniques could improve robustness and reduce overfitting. Additionally, incorporating physics-based models with data-driven ML could bridge gaps where experimental data is limited, ensuring predictions align with material science principles. Such hybrid strategies could achieve higher R2 values, lower RMSE, and better generalization across diverse concrete mixtures, advancing the reliability of predictive tools in civil engineering.
This study evaluates the efficacy of machine learning algorithms for the prediction of mechanical properties of concrete using nondestructive testing on high-performance concrete (HPC), which encourages long-lasting and ecologically friendly building. The mechanical properties of minibar and chopped basalt fiber-reinforced high-performance concrete (BFHPC) were investigated. Sample data for analysis and model creation was obtained from laboratory experiments conducted under standard controlled settings at 7, 14, and 28-day curing intervals. In conclusion, this paper examines models for predicting compressive strength using three data mining techniques and experiments. They developed a program to simulate a reinforced building against concrete. They found 96% accuracy, while the current accuracy was 99% with PR for minibars and 93% for glass fibers.
The failure results demonstrate that the ANNs can predict the failure and cracks on the surface of the compression cube. Overall, the current study can help civil engineers predict the compressive strength of High-Performance Concrete with soft programming, and Machine Learning for the failure of compressive strength. The research follows the conclusions.
1. The results indicated that the RMSE was greater than 16.99, the MAE was greater than 2.1, and the R2 was greater than 0.97. The Taylor diagram of the compressive strength for the experimental and prediction results.
2. According to the prediction made by Machine Learning, polynomial regression yields the best results, with correlation coefficient values of greater than 0.93 and 0.99 for glass fiber and minibar basalt fibers, respectively.
3. As the limitation of the current study is the minimum database and the future work can increase the database.
4. The failure test results show that the predictions and the experimental results are very similar. Because of its great precision, the machine learning prediction of mechanical properties will benefit the laboratory’s experimental design. Future research can also benefit from the identification of the most sensitive intensity influencers by machine learning algorithms.
The raw data supporting the conclusions of this article will be made available by the authors, without undue reservation.
NV: Conceptualization, Funding acquisition, Investigation, Project administration, Supervision, Validation, Visualization, Writing – review and editing, Methodology. MH: Conceptualization, Data curation, Formal Analysis, Methodology, Software, Validation, Writing – original draft, Writing – review and editing. TG: Data curation, Investigation, Software, Supervision, Validation, Visualization, Writing – review and editing, Formal Analysis, Methodology.
The author(s) declare that financial support was received for the research and/or publication of this article. This research was funded by the Ministry of Science and Higher Education of the Russian Federation within the framework of the state assignment No 075-03-2025-256 dated 16 January 2025, Additional agreement No 075-03-2025-256/1 dated Marсh 25, 2025, FSEG-2025-0008.
The authors declare that the research was conducted in the absence of any commercial or financial relationships that could be construed as a potential conflict of interest.
The author(s) declare that no Generative AI was used in the creation of this manuscript.
All claims expressed in this article are solely those of the authors and do not necessarily represent those of their affiliated organizations, or those of the publisher, the editors and the reviewers. Any product that may be evaluated in this article, or claim that may be made by its manufacturer, is not guaranteed or endorsed by the publisher.
Abhishek, T. (2021). Comparative assessment of regression models based on model evaluation metrics. Int. Res. J. Eng. Technol. 9, 853–860.
Abrori, M. A. M., Syukur, A., Affandy, A., and Soeleman, M. A. (2022). Improving C4. 5 algorithm accuracy with adaptive boosting method for predicting students in obtaining education funding. J. Dev. Res. 6, 137–140. doi:10.28926/jdr.v6i2.205
Agrawal, R. (2022). Sustainable design guidelines for additive manufacturing applications. Rapid Prototyp. J. 28, 1221–1240. doi:10.1108/rpj-09-2021-0251
Akande, K. O., Owolabi, T. O., Twaha, S., and Olatunji, S. O. (2014). Performance comparison of SVM and ANN in predicting compressive strength of concrete. IOSR J. Comput. Eng. 16, 88–94. doi:10.9790/0661-16518894
AlAlaween, W., Abueed, O., Gharaibeh, B., Alalawin, A., Mahfouf, M., Alsoussi, A., et al. (2022). The development of a radial based integrated network for the modelling of 3D fused deposition. Rapid Prototyp. J. 29, 408–421. doi:10.1108/RPJ-04-2022-0121
Algin, Z., and Ozen, M. (2018). The properties of chopped basalt fibre reinforced self-compacting concrete. Constr. Build. Mater. 186, 678–685. doi:10.1016/j.conbuildmat.2018.07.089
Altayeb, M., Wang, X., Mahmoud, M., Ali, Y., M. S., Al-Shami, A. M., and Jiang, K. (2024). AI Agents for UHPC experimental design: high strength and low cost with fewer experimental trials. Constr. Build. Mater. 416, 135206. doi:10.1016/j.conbuildmat.2024.135206
Armaghani, D., Mamou, A., Maraveas, C., Roussis, C., Siorikis, G., Skentou, A., et al. (2021). Predicting the unconfined compressive strength of granite using only two non-destructive test indexes. Geomechanics Eng., 317–330. doi:10.12989/gae.2021.25.4.317
Asteris, G., D. Skentou, A., Bardhan, A., Samui, P., and Pilakoutas, K. (2021a). Predicting concrete compressive strength using hybrid ensembling of surrogate machine learning models. Cem. Concr. Res. 145, 106449. doi:10.1016/j.cemconres.2021.106449
Asteris, G., Lemonis, M., E., Nguyen, T.-A., Van, H., and Pham, B. T. (2021b). Soft computing-based estimation of ultimate axial load of rectangular concrete-filled steel tubes. Steel Compos. Struct., 471–491. doi:10.12989/scs.2021.39.4.471
ASTM C. 109 (2017). “Standard test method for compressive strength of hydraulic cement mortars using 2-in or [50-mm] cube specimens,”. West Conshohocken, PA: ASTM International. doi:10.1520/C0109_C0109M-02
Ayub, T., Shafiq, N., and Nuruddin, M. F. (2014a). Effect of chopped basalt fibers on the mechanical properties and microstructure of high performance fiber reinforced concrete. Adv. Mater. Sci. Eng. 14, 1–14. doi:10.1155/2014/587686
Ayub, T., Shafiq, N., and Nuruddin, M. F. (2014b). Mechanical properties of high-performance concrete reinforced with basalt fibers. Procedia Eng. 77, 131–139. doi:10.1016/j.proeng.2014.07.029
Beskopylny, A. N., Stel’makh, S. A., Shcherban’, E. M., Mailyan, L. R., Meskhi, B., Razveeva, I., et al. (2022). Concrete strength prediction using machine learning methods CatBoost, k-nearest Neighbors, support vector regression. Appl. Sci. 10864. doi:10.3390/app122110864
Beskopylny, A. N., Stel’makh, S. A., Shcherban, E. M., Razveeva, I., Kozhakin, A., Pembek, A., et al. (2024). Prediction of the properties of vibro-centrifuged variatropic concrete in aggressive environments using machine learning methods. Buildings 1198. doi:10.3390/buildings14051198
GOST 24452-80 Betony (2005). Metody opredeleniya Prochnosti Po Kontrolnym Obraztsam [concretes. Methods for determination of strength by control samples]. (Moscow, Russia: Standartinform). Available online at: http://gostexpert.ru/gost/gost-24452-80.
Canbaz, M., Bensaoud, M., Erol, H., and Selim Şengel, H. (2022). Effect of steam‒curing on the glass fiber reinforced concrete. Chall. J. Concr. Res. Lett. 13, 107–115. doi:10.20528/cjcrl.2022.04.001
Cavaleri, L., Barkhordari, M. S., C. Repapis, C., Armaghani, D. J., Vladimirovich Ulrikh, D., and Asteris, G. (2022). Convolution-based ensemble learning algorithms to estimate the bond strength of the corroded reinforced concrete. Constr. Build. Mater. 359, 129504. doi:10.1016/j.conbuildmat.2022.129504
Chiadighikaobi, P. C., Hasanzadeh, A., Hematibahar, M., Kharun, M., Mousavi, M. S., A. Stashevskaya, N., et al. (2024a). Evaluation of the mechanical behavior of high-performance concrete (HPC) reinforced with 3D-Printed trusses. Results Eng. 22, 102058. doi:10.1016/j.rineng.2024.102058
Chiadighikaobi, P. C., Jean Paul, V., and Brown, C. K. S. (2021). The effectiveness of basalt fiber in lightweight expanded clay to improve the strength of concrete helicoidal staircase. Mater. Sci. Forum 1034, 187–192. doi:10.4028/www.scientific.net/MSF.1034.187
Chiadighikaobi, P. C., Kharun, M., and Hematibahar, M. (2023). Historical structure design method through data analysis and soft programming. Cogent Eng. 10, 2220499. doi:10.1080/23311916.2023.2220499
Chopra, P., KumarSharma, R., and Kumar, M. (2016). Prediction of compressive strength of concrete using artificial neural network and genetic programming. Hindawi Publ. Corp. Adv. Mater. Sci. Eng. 10, 1–10. doi:10.1155/2016/7648467
Dai, L., Wu, X., Zhou, M., Ahmad, W., Ali, M., Sabri, M. M. S., et al. (2022). Using machine learning algorithms to estimate the compressive property of high strength fiber reinforced concrete. Materials 15, 4450. doi:10.3390/ma15134450
Elhishi, S., Elashry, A. M., and El-Metwally, S. (2023). Unboxing machine learning models for concrete strength prediction using XAI. Sci. Rep. 13, 19892. doi:10.1038/s41598-023-47169-7
Enwere, K., Nduka, E., and Ogoke, U. (2023). Comparative analysis of Ridge, bridge and lasso regression models in the presence of multicollinearity. IPS Intelligentsia Multidiscip. Journa 3, 1–8. doi:10.54117/iimj.v3i1.5
Erdal, H. I. (2013). Two-level and hybrid ensembles of decision trees for high performance concrete compressive strength prediction. Eng. Appl. Artif. Intell. 26, 1689–1697. doi:10.1016/j.engappai.2013.03.014
Farooq, F., Czarnecki, S., Niewiadomski, P., Alabduljabbar, H., Ostrowski, K. A., Sliwa-Wieczorek, K., et al. (2021). A comparative study for the prediction of the compressive strength of self-compacting concrete modified with fly ash. Materials 14, 4934. doi:10.3390/ma14174934
Gandomi, A. H., and Roke, D. A. (2015). Assessment of artificial neural network and genetic programming as predictive tools. Adv. Eng. Softw. 88, 63–72. doi:10.1016/j.advengsoft.2015.05.007
Guo, P., Mahjoubi, S., Liu, K., Meng, W., and Bao, Y. (2023). Self-updatable AI-assisted design of low-carbon cost-effective ultra-high-performance concrete (UHPC). Case Stud. Constr. Mater. 19, e02625. doi:10.1016/j.cscm.2023.e02625
Guo, Y., Hu, X., and Lv, J. (2019). Experimental study on the resistance of basalt fibre-reinforced concrete to chloride penetration. Constr. Build. Mater. 223, 142–155. doi:10.1016/j.conbuildmat.2019.06.211
Hasanzadeh, A., Vatin, N. I., Hematibahar, M., Kharun, M., and Shooshpasha, I. (2022). Prediction of the mechanical properties of basalt fiber reinforced high-performance concrete using machine learning techniques. Materials 15, 7165. doi:10.3390/ma15207165
Hematibahar, M. (2021). Crack resistance in basalt fibred High-Performance Concrete. Moscow, Russia: Depttment Civil Engineering, People Friendship University of Russia RUDN University.
Hematibahar, M., and Kharun, M. (2024). Prediction of concrete mixture design and compressive strength through data analysis and machine learning. J. Mech. Continua Math. Sci. 19, 1–21. doi:10.26782/jmcms.2024.03.00001
Hematibahar, M., Kharun, M., Beskopylny, A., Stel’makh, S., Shcherban, E., and Razveeva, I. (2024). Analysis of models to predict mechanical properties of high-performance and ultra-high-performance concrete using machine learning. J. Compos. Sci. 8, 287. doi:10.3390/jcs8080287
Hematibahar, M., Vatin, N. I., Alaraza, H. A. A., Khalilavi, A., and Kharun, M. (2022). The prediction of compressive strength and compressive stress–strain of basalt fiber reinforced high-performance concrete using classical programming and logistic map algorithm. Materials 19, 6975. doi:10.3390/ma15196975
Hematibahar, M., Vatin, N. I., Hamid, T. J., and Gebre, T. H. (2023). Effect of using 3D-printed shell structure for reinforcement of ultra-high-performance concrete, Struct. Mech. Eng. Const. Build. 19, 534–547. doi:10.22363/1815-5235-2023-19-5-534-547
Hematibar, M., Kharun, M., and Vatin, I. V. (2024). “Tensile strength prediction method through compressive concrete cube test,” in Construction of unique buildings and structures. doi:10.4123/CUBS.111.2
Jiang, C., Fan, K. E., Wu, F., and Chen, D. A. (2014). Experimental study on the mechanical properties and microstructure of chopped basalt fibre reinforced concrete. Mater. and Des. 58, 187–193. doi:10.1016/j.matdes.2014.01.056
Kaplan, G., Yaprak, H., Memis¸, S., and Alnkaa, A. (2019). Artificial neural network estimation of the effect of varying curing conditions and cement type on hardened concrete properties. Buildings 9, 10. doi:10.3390/buildings9010010
Kardani, N., Bardhan, A., Samui, P., Nazem, M. G., Asteris, P., and Zhou, A. (2022). Predicting the thermal conductivity of soils using integrated approach of ANN and PSO with adaptive and time-varying acceleration coefficients. Int. J. Therm. Sci. 173, 107427. doi:10.1016/j.ijthermalsci.2021.107427
Kashyzadeh, K. R., Amiri, N., Ghorban, S., and Souri, K. (2022). Prediction of concrete compressive strength using a back-propagation neural network optimized by a genetic algorithm and response surface analysis considering the appearance of aggregates and curing conditions. Buildings 12, 438. doi:10.3390/buildings12040438
Khademi, F., and Behfarnia, K. (2016). Evaluation of concrete compressive strength using artificial neural network and multiple linearregression models. Int. J. Optim. Civ. Eng. 6, 423–432.
Khan, M. A., Memon, S. A., Farooq, F., Javed, M. F., Aslam, F., and Alyousef, R. (2021). Compressive strength of fly-ash-based geopolymer concrete by gene expression programming and random forest. Adv. Civ. Eng. 2021. doi:10.1155/2021/6618407
Kharun, M., and Koroteev, D. (2018). Effect of basalt fibres on the parameters of fracture mechanics of MB modifier based high-strength concrete. MATEC Web Conf. 251, 02003. doi:10.1051/matecconf/201825102003
Kharun, M. A., Alaraza, H., Hematibahar, M., Al Daini, R., and Manoshin, A. (2022). Experimental study on the effect of chopped basalt fiber on the mechanical properties of high-performance concrete. AIP Conf. Proc. 2559, 050017. doi:10.1063/5.0099042
Khorasani, M., Loy, J., Ghasemi, A., Sharabian, E., Leary, M., Mirafzal, H., et al. (2022). A review of Industry 4.0 and additive manufacturing synergy. Rapid Prototyp. J. 28, 1462–1475. doi:10.1108/RPJ-08-2021-0194
Lam, N. N., and Hung, L. V. (2021). Mechanical and shrinkage behavior of basalt fiber reinforced ultra-high-performance concrete. 20, 28–35. doi:10.21660/2021.78.86151
Ly, H.-B., Pham, B. T., Le, L. M., Le, T.-T., Le, V. M., and G. Asteris, P. (2021). Estimation of axial load-carrying capacity of concrete-filled steel tubes using surrogate models. Neural Comput. Appl. 33, 3437–3458. doi:10.1007/s00521-020-05214-w
Mahjoubi, S., Barhemat, R., Meng, W., and Bao, Y. (2023). AI-guided auto-discovery of low-carbon cost-effective ultra-high performance concrete (UHPC). Resour. Conservation Recycl. 189, 106741. doi:10.1016/j.resconrec.2022.106741
Mahjoubi, S., Meng, W., and Bao, Y. (2022). Auto-tune learning framework for prediction of flowability, mechanical properties, and porosity of ultra-high-performance concrete (UHPC). Appl. Soft Comput. 115, 108182. doi:10.1016/j.asoc.2021.108182
Matthews, B., Palermo, A., Logan, T., and Scott, A. (2024). Database and optimized machine learning prediction of the deteriorated response of corroded reinforced concrete beams. Dev. Built Environ. 19, 100527. doi:10.1016/j.dibe.2024.100527
Melkumovaa, L., and Shatskikhb, S. (2017). Comparing Ridge and LASSO estimators for data analysis. Elsevier Inc. doi:10.1016/j.proeng.2017.09.615
Miao, X., Zhu, J.-X., Zhu, W.-B., Wang, Y., Peng, L., Dong, H.-L., et al. (2024). Intelligent prediction of comprehensive mechanical properties of recycled aggregate concrete with supplementary cementitious materials using hybrid machine learning algorithms. Case Stud. Constr. Mater. 21, e03708. doi:10.1016/j.cscm.2024.e03708
Mohaghegh, A. L., Silfwerbrand, J., and Årskog, V. (2018). Shear behavior of high-performance basalt fiber concrete—Part II: laboratory punching shear tests on small slabs with macrofibers without bars. Struct. Concr. 19, 331–339. doi:10.1002/suco.201700207
Momeni, K., Ivanovich Vatin, N., Hematibahar, M., and Gebre, T. (2024). Repair overlays of modified polymer mortar containing glass powder and composite fibers-reinforced slag: mechanical properties, energy absorption, and adhesion to substrate concrete. Front. Built Environ. 10, 1479849. doi:10.3389/fbuil.2024.1479849
Myles, A. J., Feudale, R. N., Liu, Y., Woody, N. A., Brown, S. D., and Chemom, J. (2004). An introduction to decision tree modeling. J. Chemom. A J. Chemom. Soc. 18, 275–285. doi:10.1002/cem.873
Niu, D., Su, L., Luo, Y., Huang, D., and Luo, D. (2019). Experimental study on mechanical properties and durability of basalt fiber reinforced coral aggregate concrete. Constr. Build. Mater. 237, 117628. doi:10.1016/j.conbuildmat.2019.117628
Peng, X., Zhuang, Z., and Yang, Q. (2022). Predictive modeling of compressive strength for concrete at super early age. Materials 15, 4914. doi:10.3390/ma15144914
Qureshi, H. J., Alyami, M., Nawaz, R., Hakeem, I. Y., Aslam, F., Iftikhar, B., et al. (2023). Prediction of compressive strength of two-stage (preplaced aggregate) concrete using gene expression programming and random forest. Case Stud. Constr. Mater. 19, e02581. doi:10.1016/j.cscm.2023.e02581
Rong, L., Wang, Y., Wang, Y., Jiang, D., Bai, J., Wu, Z., et al. (2024). A fresh-cut papaya freshness prediction model based on partial least squares regression and support vector machine regression. Heliyon 10, e30255. doi:10.1016/j.heliyon.2024.e30255
Shahmansouri, A. A., Yazdani, M., Hosseini, M., Akbarzadeh Bengar, H., and Farrokh Ghatte, H. (2022). The prediction analysis of compressive strength and electrical resistivity of environmentally friendly concrete incorporating natural zeolite using artificial neural network. Constr. Build. Mater. 317, 125876. doi:10.1016/j.conbuildmat.2021.125876
Shu, J., Yu, H., Liu, G., Yang, H., Guo, W., Phoon, C., et al. (2024). Proposing an inherently interpretable machine learning model for shear strength prediction of reinforced concrete beams with stirrups. Case Stud. Constr. Mater. 20, e03350. doi:10.1016/j.cscm.2024.e03350
Sim, J., Park, C., and Moon, D. Y. (2005). Characteristics of basalt fiber as a strengthening material for concrete structures. Compos. Part B Eng. 36, 504–512. doi:10.1016/j.compositesb.2005.02.002
Sondarva, D., and Bhogayata, A. C. (2017). Usage of chopped basalt fibers in concrete composites: a review. Int. J. Eng. Res. and Technol. (IJERT) 6. doi:10.17577/IJERTV6IS090164
Tian, Q., Lu, Y., Zhou, J., Song, S., Yang, L., Cheng, T., et al. (2024). Supplementary cementitious materials-based concrete porosity estimation using modeling approaches: a comparative study of GEP and MEP. Rev. Adv. Mater. Sci. 63, 20230189. doi:10.1515/rams-2023-0189
Topçu, I. B., and Sarıdemir, M. (2007). Prediction of properties of waste AAC aggregate concrete using artificial neural network. Comput. Mater. Sci. 41, 117–125. doi:10.1016/j.commatsci.2007.03.010
Vatin, N. I., Hematibahar, M., and Gebre, T. (2024). Impact of basalt fiber reinforced concrete in protected buildings: a review. Front. Built Environ. 10. doi:10.3389/fbuil.2024.1407327
Wakjira, G., Kutty, A., A., and Shahria Alam, M. (2024). A novel framework for developing environmentally sustainable and cost-effective ultra-high-performance concrete (UHPC) using advanced machine learning and multi-objective optimization techniques. Constr. Build. Mater. 416, 135114. doi:10.1016/j.conbuildmat.2024.135114
Wakjira, T. G., Ibrahim, M., Ebead, U., and Alam, M. S. (2022). Explainable machine learning model and reliability analysis for flexural capacity prediction of RC beams strengthened in flexure with FRCM. Eng. Struct. 255, 113903. doi:10.1016/j.engstruct.2022.113903
Wang, Q., Cheng, T., Lu, Y., Liu, H., Zhang, R., and Huang, J. (2024). Underground mine safety and health: a hybrid MEREC–CoCoSo system for the selection of best sensor. Sensors 24, 1285. doi:10.3390/s24041285
Wang, Q., Feng, W., Yang, C., Yu, Y., Li, G., Beer, M., et al. (2022). Polyphase uncertainty analysis through virtual modelling technique. Mech. Syst. Signal Process. 162, 108013. doi:10.1016/j.ymssp.2021.108013
Wei, B., Cao, H., and Song, S. (2010). Tensile behavior contrast of basalt and glass fibers after chemical treatment. Mater. Des. 31, 4244–4250. doi:10.1016/j.matdes.2010.04.009
Yang, D., Yan, C., Liu, S., Jia, Z., and Wang, C. (2022). Prediction of concrete compressive strength in saline soil environments. Materials 15, 4663. doi:10.3390/ma15134663
Yu, Y., Gao, W., Castel, A., Liu, A., Chen, X., and Liu, M. (2020a). Assessing external sulfate attack on thin-shell artificial reef structures under uncertainty. Ocean. Eng. 207, 107397. doi:10.1016/j.oceaneng.2020.107397
Yu, Y., Wu, D., and Gao, W. (2020b). Stochastic chemo-physical-mechanical degradation analysis on hydrated cement under acidic environments. Appl. Math. Model. 78, 75–97. doi:10.1016/j.apm.2019.10.012
Zhao, Y., Yang, G., Zhu, L., Ding, L., Guan, X., Wu, X., et al. (2022). Effects of rheological properties and printing speed on molding accuracy of 3D printing basalt fiber cementitious materials. J. Mater. Res. Technol. 21, 3462–3475. doi:10.1016/j.jmrt.2022.10.124
Keywords: data mining, dynamic analysis, reinforced concrete beam, prediction, basalt fiber
Citation: Vatin NI, Hematibahar M and Gebre TH (2025) Chopped and minibars reinforced high-performance concrete: machine learning prediction of mechanical properties. Front. Built Environ. 11:1558394. doi: 10.3389/fbuil.2025.1558394
Received: 10 January 2025; Accepted: 27 March 2025;
Published: 08 April 2025.
Edited by:
Hindavi Gavali (Tikate), NICMAR University Pune, IndiaReviewed by:
Valentina Tomei, University of Cassino, ItalyCopyright © 2025 Vatin, Hematibahar and Gebre. This is an open-access article distributed under the terms of the Creative Commons Attribution License (CC BY). The use, distribution or reproduction in other forums is permitted, provided the original author(s) and the copyright owner(s) are credited and that the original publication in this journal is cited, in accordance with accepted academic practice. No use, distribution or reproduction is permitted which does not comply with these terms.
*Correspondence: Nikolai Ivanovich Vatin, dmF0aW5AbWFpbC5ydQ==
Disclaimer: All claims expressed in this article are solely those of the authors and do not necessarily represent those of their affiliated organizations, or those of the publisher, the editors and the reviewers. Any product that may be evaluated in this article or claim that may be made by its manufacturer is not guaranteed or endorsed by the publisher.
Research integrity at Frontiers
Learn more about the work of our research integrity team to safeguard the quality of each article we publish.