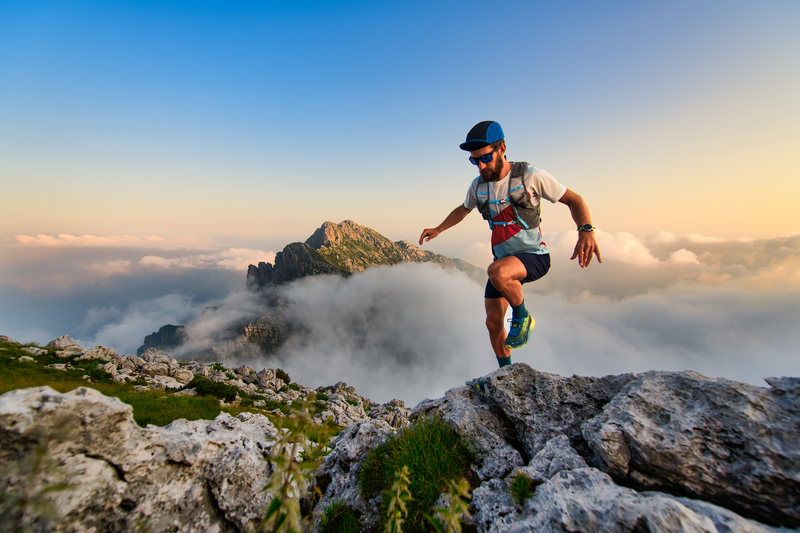
94% of researchers rate our articles as excellent or good
Learn more about the work of our research integrity team to safeguard the quality of each article we publish.
Find out more
ORIGINAL RESEARCH article
Front. Built Environ. , 11 February 2025
Sec. Transportation and Transit Systems
Volume 11 - 2025 | https://doi.org/10.3389/fbuil.2025.1536080
Today, the population growth rate in Thailand is very slow. In particular, the number of older people in Thailand is notably increasing. Many members of this group are reluctant to accept or use new technology. The government is formulating a support plan for a high-speed rail (HSR) system to develop the public transportation system to support better livelihood and reduce traffic problems, which may affect the use intent of older people. The factors that influence HSR use will support social coherence and help older people to adapt to and cope with newly developed technology in the future. This study aims to develop a perspective model of the influence of HSR use among older people by applying the theory of planned behavior (TPB) combined with the unified theory of acceptance and use of technology (UTAUT). Data were collected through a questionnaire survey on 3,200 people older than 60 in four regions in Thailand. Analysis was conducted using the structural equation model. The result indicated that all factors of TPB and UTAUT significantly influenced the behavioral intention of older people to use HSR. The factor with the largest influence was the attitude toward technology use, followed by subjective norms and facilitating conditions. Moreover, performance and effort expectancies play statistically significant roles in the attitude toward technology use.
Thailand aims to enhance travel through a coherent high-speed rail (HSR) system that has thoroughly decentralized the regions of the country. According to the strategic frame of the transportation infrastructure development in Thailand from 2015 to 2022, the HSR project is a collaboration between Thailand and China to promote the strategic development of the HSR system in the ASEAN. This route is intended to link Thailand to other countries in the region and the world. This project aligns with the development strategy of the Belt and Road Initiative of China, which aims to develop connections to Bangkok in Thailand, Vientiane in Laos, and Kunming in the main city of Yunnan in China and will link the HSR network of China seamlessly. It will also develop the HSR network from Bangkok to Malaysia and Singapore in the future. This connection is intended to aid economic development, link the trade markets in the Mekong River Watershed countries, and attract more travelers (Songmuang, 2016). The current operation of the HSR project between Bangkok and Nongkhai is in its first phase. The route from Bangkok to Nakhon Ratchasima is 250.77 km. It features six stations and six service trains that accommodate 590 people per train and depart every 15 min. The fastest speed of travel is 250 km/h. The system is currently under construction, and the overall operation of the project is expected to reach 35.74% in the year 2024 (Transport, 2024).
Research in countries that already have HSR has found that it increases older people’s travel and migration by enhancing accessibility and connectivity. Other findings reveal that policymakers should proceed cautiously when enhancing connectivity and access to HSR (Lyu et al., 2024); urban economic growth can benefit from the development of HSR in China (Chong et al., 2019); HSR better addresses the needs of a changing society while prioritizing urgent housing and sustainability (Kamga, 2015); HSR is targeted to support interurban travel with its various features, such as speed, punctuality, affordability, simple travel process (Yang et al., 2018) and accessibility for older people in long-distance travel. The introduction of the HSR has the potential to significantly contribute to Thailand’s development as a developing country. It can enhance the quality of life, foster social connections, and provide increased opportunities for entertainment and value through more efficient and enjoyable travel experiences.
According to statistics, the aging population in Thailand has notably increased (Figure 1) (Yang et al., 2018). Comparing the statistics for 2013 and 2023, the population older than 60 has reached 9,057,761 and 13,064,929, while the ratios of older people to the total population were 20.08% and 14%, respectively. In other words, the increasing proportion of older people was high at 44% (Department of Older Persons, 2024), which makes Thailand an aged society. When an aging society becomes an aged society, multidimensional challenges such as social barriers, income, and education are critical determinants of health-related Internet use among older adults in Thailand (Robru et al., 2024). Cultural barriers, including a preference for familiarity, also play a role. A study on the mobility of older people in Bangkok found that inadequate transportation infrastructure and services limit their access to public spaces, indicating a reluctance to embrace new transportation modes such as HSR (Srichuae et al., 2015). Physical barriers further compound these challenges. A study on the quality of life of older adults with physical and mobility disabilities in Thailand highlights the difficulties they face in accessing transportation services, which can be exacerbated in the context of HSR (Nanthamongkolchai et al., 2022). Improving access to and provision of facilities for older people, reducing social inequality, and enhancing their quality of life are crucial to ensure that older people do not become a social burden in the future (National Statistical Office, 2021). Therefore, examining the intent of older people to use the HSR system should be prioritized to enable the formulation of relevant policies that address quality of life, travel, and access to facilities.
Figure 1. Number of people in Thailand 2011–2023 older than 60 years (Department of Older Persons, 2024).
Previous studies have examined the behavioral intention (BI) of older people toward technology use or innovations. Most research investigated BI in the use of health-related technology, such as equipment or applications for monitoring, including health services using the UTAUT (Chen et al., 2023; Hoque and Sorwar, 2017). Other studies focused on the use of smart homes by older people using the theory of planned behavior (TPB) and the UTAUT (Siripipatthanakul et al., 2023) as well as smartphones using the UTAUT (Yang et al., 2023). A study on the use intention of HSR found that many research used the TPB (Hsiao and Yang, 2010; Borhan et al., 2019b; Chuang et al., 2011; Mahardika et al., 2022; Fu, 2021), but studies that examined use intention of HSR from the perspective of UTAUT are lacking, especially in the context of older people. Only Hou et al. (2021) explored travel behavior through HSR using older people as a sample, but there is no study of the intention to use HSR specific to older people.
The current study combined the TPB and UTAUT. TPB was developed from the theory of reasoned action (TRA) by Ajzen (1991) to enhance the understanding of human behavior. TPB focuses on prediction and explains behavior during partially controlled scenarios through three major factors that affect BI, namely, attitude toward use (ATU), subjective norms (SN), and perceived behavioral control (PBC). SN is similar to social influence (SI) in the UTAUT. This theory helps to explain or predict behavior by also analyzing BI, which is an important indicator of whether a behavior will happen and whether it affects a decision (Conner, 2020).
One section of the UTAUT describes the theory to help researchers evaluate the purpose of each person on a specific system use and specify any important factors that affect technology acceptance in the accepted context. Conner (2020) proposed the theory, which was developed from the revision and combination of eight other theories, namely, TRA, the technology acceptance model (TAM), the motivational model, the TPB, a combination of TBP/TAM, the model of PC utilization, innovation diffusion theory (IDT), and social cognitive theory (Williams et al., 2015). A strong model that was established to explain user behavior that affects a new technology or specific system, UTAUT is composed of four major factors: performance expectancy (PE), effort expectancy (EE), social influence (SI), and facilitating conditions (FC). The UTAUT is a direct determinant of the purpose in behavioral performance. These structures are controlled by gender, age, experience, and voluntariness of use and are also identified as variables that affect intention and behavior and can enhance the understanding of the behavior of users. UTAUT and TPB are theories that can explain and verify BI because their structure covers many factors and can be widely adapted in other contexts.
This research links TPB and UTAUT by a role test of ATU as a mediator among EE, PE, and BI because ATU plays an important role in enhancing the understanding of EE. In turn, PE influences BI to use from the perspective of older people (ATU). Furthermore, it helps the analysis of BI to use to have increased accuracy, including efficient prediction. A previous study found only research related to technology and information technology, such as application development, smart home technology, and complaint system importation on the e-government (Zhou et al., 2020; Maswadi et al., 2022; Dwivedi et al., 2019; Khalilzadeh et al., 2017; Rana et al., 2016; Chen and Chang, 2013). According to a study on the relationship between ATU, EE, PE, and BI, if people realize that technology is useful and increases efficient usability, then they will develop a positive attitude toward the use or decision to use new technology. However, studies that examined BI in relation to HSR use among older people remain lacking. The relevant studies are mainly related to technology acceptance and progress among older people (Zhou et al., 2020; Maswadi et al., 2022). In summary, these studies reported that EE and PE exerted an influence on ATU, which affects the BI to use of older people.
The choice of TPB and UTAUT in data analysis is important because both theories address the factors that influence technology acceptance and new technology use, especially those related to decision and intention for behavioral performance. Moreover, these theories encourage the analysis of attitude and social science in behavior prediction, which helps the analysis efficiently address the usability of new technology.
Thus, it is useful to study BI on HSR use from the perspective of older passengers to support long-distance travel using the new public transportation system in Thailand. It could also help in the development of a usability platform that is suitable for older passengers by providing in-depth data on the factors that influence BI on HSR use based on TPB and UTAUT. The combination of the two theories will help researchers find new data related to the factors that influence BI on HSR use among older people for policy development that motivates HSR use by older people in the future.
This study reviews the concept of the intention to use HSR among older passengers and finds that two concepts can be integrated to develop a usability platform that is appropriate for older people (Table 1).
This theory posits a link between belief and behavior. It identifies three major elements, namely, ATU, SN, and PBC, which affect BI, an important indicator of whether or not a person will perform a behavior in which the intention of this behavior performance will lead to the actual behavior (Hou et al., 2021).
H1. ATU exerts a positive influence on the BI of older people on HSR use.
This dimension denotes a person’s perception of whether their own ability and resources will allow them to succeed in that behavior. If people believe that they can perform a behavior, then they tend to do so more frequently. A previous study found that PBC influences the health BI of older adults (Li et al., 2019; Stolte et al., 2017) and physical checkups of retirees (Borhan et al., 2019a). Thus, the current study poses the following hypothesis:
H2. PBC exerts a positive influence on the BI of older people on HSR use.
This dimension describes one’s perception of whether other people influence them with regard to behavior. If people believe that the people around them support their behavior, then they will tend to perform the behavior more frequently. SN is similar to social influencing in UTAUT. A previous study found that SN exerts a positive relationship and impact on the use intention of older people for smart homes (Ku and Hsieh, 2016), online grocery shopping (Pal et al., 2018b), and cloud-based healthcare services (Stolte et al., 2017). Social influencing is very influential on technology use intention and behavior in older adults (Bezirgani and Lachapelle, 2021) and exerts a positive impact on online shopping among older people (Ma et al., 2021). Moreover, it has significance in the use of MHealth services by older people (Soh et al., 2020). Thus, the current study proposes the following.
H3. SN exerts a positive influence on the BI of older people on HSR use.
Venkatesh et al. developed the UTAUT in 2003. It consists of four major dimensions that influence the acceptance and use of technology, namely, PE, EE, SI, and FC, to explain the factors that affect a person’s acceptance and new technology use in an organization and society. This theory was developed by collecting data and improving the designs of previous theories and models, such as the TAM, TRA, and IDT. These models covered clear structures and were used as research concepts to examine the factors that influence the acceptance and use of technology in various contexts (Hoque and Sorwar, 2017).
FC pertains to one’s perception of whether an organization or environment provides the support and resources necessary for technological use. If people know that resources and support are sufficient, then they will tend to accept and use technology. A previous study found that FC is a critical factor in the acceptance of smartphone use in older adults (Venkatesh et al., 2003; Siripipatthanakul et al., 2023) and the use of mobile registration apps in Taiwan (Ma et al., 2016). Thus, the following hypothesis is posed:
H4. FC exerts a positive influence on the BI of older people on HSR use.
This element represents one’s perception of whether a new technology will increase work efficiency. If people believe that technology will enhance job performance, then they will tend to accept and use technology. PE is an important factor that influences the introduction of technology use among older people (Bezirgani and Lachapelle, 2021) and mainly impacts BI in terms of the acceptance and use of smartphones among older people (Siripipatthanakul et al., 2023), equipment or applications for health monitoring and services (National Statistical Office, 2021; Chen et al., 2023), and Internet banking (Hsu and Peng, 2022; Martins et al., 2014). Therefore, Hypothesis 5 is presented:
H5. PE exerts a positive influence on the BI of older people on HSR use.
EE denotes one’s perception of whether a new technology is easy to use. If this is the case, then people will tend to accept and use the technology. A previous study found that a significant positive relationship exists between EE and intention to use technologies among older people, such as at-home telehealth services (Arenas Gaitán et al., 2015), acceptance and use of smartphones (Siripipatthanakul et al., 2023), MHealth services, and virtual nursing homes (Cimperman et al., 2016). Thus, the study presents the following hypothesis.
H6. EE exerts a positive influence on the BI of older people on HSR use.
Almetere et al. (2020) mentioned that a mediator is needed when a study confirms that no direct relationship exists between two constructs, while another study mentioned that ATU (Zhou et al., 2020) plays an important role as a mediator of the relationships between PE and BI (Siripipatthanakul et al., 2023) and among EE, PE, and BI (Maswadi et al., 2022; Chen et al., 2011). Against this background, the study poses the following hypotheses.
H7. PE exerts a positive influence on the ATU of older people on HSR use.
H8. EE exerts a positive influence on the ATU of older people on HSR use.
The abovementioned hypotheses are key to understanding the BI on HSR use among older passengers. The researcher examined the relevant literature on the relationship between factors that aligned with the presented hypotheses (Table 1).
This research combined TPB and UTAUT to build a statistical model for the evaluation of the relationship among the factors of TPB and UTAUT that affect the BI on HSR use among older passengers. This study collected data from self-administered questionnaires. The questionnaire was composed of three sections. The first focuses on the general information of the respondents, including travel behavior. The second presents a survey of factors that affect the choice of HSR use among older people; all questions were based on the factors of the TPB. The final section presents a survey with questions based on the attitude factors of UTAUT. All items per factor were rated using a seven-point Likert-type scale ranging from 1 = strongly disagree to 7 = strongly agree (Joshi et al., 2015).
The questionnaire was developed by reviewing and evaluating its content validity with a panel of five transportation experts. Each question was assessed based on clarity of wording, interpretation, and alignment with the measured objectives. The experts were informed about the study’s objectives and instructed to rate each item as follows: +1 if it met the criteria, 0 if they were uncertain, and −1 if it did not meet the requirements. After collecting the ratings, each index item’s item-objective congruence (IOC) was calculated. Only items with an IOC score of 0.5 or higher were considered acceptable. Items scoring below 0.5 were revised based on the experts’ suggestions, and this process was repeated until all items achieved an IOC score of 0.5 or higher. After developing the questionnaire, a pilot study involving 60 respondents was conducted to assess its validity. The results indicated that the questionnaire demonstrated an acceptable level of reliability, as evidenced by a satisfactory Cronbach’s alpha coefficient.
Participants were selected using the stratified random sampling method. This method involves drawing random samples from a large population divided into distinct strata based on specific characteristics. To ensure the data collected were thorough and representative, a random sample was taken from each stratum. The target group for this study consisted of older individuals aged 60 years and above, as per the definition of older people from transportation in the United Kingdom and the United Nations (Department of Older Persons, 2024). These individuals undergo long trips across many instances of many types of transportation. The aging population is categorized into four regions of Thailand: the North, the Northeast, the Central, and the South, in alignment with the HSR route and government policy. The analysis focuses on the first four provinces in each region with the largest aging populations. The data from each province were categorized based on the respondents’ residential areas: urban areas, defined as regions with large, high-density populations and well-developed infrastructure, and rural areas, characterized by small, low-density settlements with limited infrastructure (Mountrakis et al., 2005; Cromartie and Bucholtz, 2008; Delgado-Viñas and Gómez-Moreno, 2022).
In this research, data were gathered from four regions, each with four provinces, for a total of 16 provinces, 200 samples per province, and 3,200 older volunteers throughout Thailand. The study was conducted according to the guidelines of the Declaration of Helsinki and approved by the Ethics Committee of Suranaree University of Technology (COE No. 2/2567, 15 December 2023). Verbal consent was secured from the volunteers, and a detailed explanation of the questionnaire was provided prior to data collection. Table 2 provides details of the items.
This study used structural equation modeling (SEM) to analyze the relationship between the BI on HSR use and the factors of TPB and UTAUT of older passengers (Figure 2). SEM is a commonly used tool in the social and behavioral sciences. It is in widespread use and helps to improve theoretical models and evaluate model appropriation by referring to empirical data (Tarka, 2018). This analysis has a mandate to test the hypotheses. The researcher identified six latent variables, namely, ATU, PBC, SN, PE, EE, and FC. These variables were not directly measured but were determined through the relationship and explanation of the direct variables or items in the questionnaire.
Data analysis using SEM was conducted using two steps. The first step refers to the measurement model, which is intended to verify the measurement quality of the latent structure used to test the SEM as part of confirmatory factor analysis. This is used to test or estimate statistical relationships with a focus on causal relationships between theoretical latent variables or constructs or on the testing of causal relationships between the latent and observed variables. Measurement models need to consider reliability using the value of Cronbach’s alpha (α) for composite reliability (CR) and average variance extracted (AVE). The second step is a path analysis of the relationship between latent items using maximum likelihood estimation (MLE) to evaluate parameter values. Fit indicators are considered:
This section presents the following data that underwent analysis. The first is demographic characteristics in which the general data of the respondents are mentioned (gender, age, and level of education). The descriptive results were the primary analysis for data diffusion checking (skewness, kurtosis, and mean). The measurement model was derived from the presentation of factor analysis for data reliability checking before leading to the next round of analysis (CR, AVE, and Cronbach’s α). Lastly, the section presents the results of the analysis, which are explained via the structural equation model.
Table 3 displays the survey respondents. More than half (69%) are aged 60–69 years, and the majority (51%) live in urban areas. Approximately 55% are men, of whom half (51%) live in urban areas. In terms of level of education, the majority (81%) completed a bachelor’s degree, with an equal proportion of people living in urban and rural areas. For the majority (70%), income per month is less than 15,000 baht. The income of those living in urban areas is relatively higher than that of people living in rural areas. The number of respondents with chronic diseases is equal to those without. Respondents living in urban areas (6%) are much more than those in rural areas, while the most common travel behavior of older people in terms of frequency of long travel is less than once per month (52%). Most travelers live in rural areas (55%), and the most common mode of transportation is private car (38%).
Table 4 presents the items used for each group of factors. The basic statistical analysis of variables in terms of the factors that influence the attitude of older passengers and BI on HSR use considered the initial data prior to the analysis of the statistical model to confirm normal data diffusion. Table 4 presents the distribution verification of the attitude factor via skewness, kurtosis, and data diffusion. The results indicate that the data followed a normal distribution, with skewness ranging from −0.623 to −1.062 and kurtosis from −0.003 to 1.565. These values fall within the acceptable thresholds suggested by Kline (2023) (skewness: −2 to 2; kurtosis: −7 to 7). Although excessive skewness and kurtosis can signal deviations from normality, potentially leading to biased results in factor analysis, no such issues were observed in this study. Additionally, as noted by Bandalos and Finney (2018), high skewness and kurtosis can adversely affect model fit indices in confirmatory factor analysis (CFA), underscoring the importance of evaluating these statistics before proceeding. Because the skewness and kurtosis values in this study remained within acceptable limits, further investigation or adjustments were deemed unnecessary. The averages of attitude opinion under the TPB of each item inside the factor are ATU: 5.500–5.608, SN: 5.228–5.609, PBC: 4.960–5.232, and BI: 5.454–5.664. The opinion average for UTAUT has the average of each item: PE: 5.634–5.771, EE: 5.398–5.733, and FC: 5.437–5.852.
This study used MPlus 7 for data analysis to evaluate the coefficient and significance of the hypothesis. The correctness of convergent validity, CR, and discriminant validity must be tested to verify the correctness and quality of the construct measurement. Table 5 presents the results. Hair et al. (2009) suggested that the acceptable factor loading of each item should be more than 0.5 and ideally equal to or higher than 0.7. Table 5 indicates that all items displayed factor loadings of more than 0.5. In addition, the AVE value was between 0.541 and 0.653, which aligns with the suggestion of Fornell and Larcker (1981) and Gefen et al. (2000); that is, a value of 0.5 indicates good convergent validity. All factors produced CR values larger than 0.8, which indicates good internal consistency. All Cronbach’s α values are larger than 0.7, which aligns with the suggestion of Peterson (1994); that is, an acceptable value ranges from 0.5 to 0.6 and ideally higher than 0.7.
After the verification of the measurement model, the study then verified the relationship between the major factors to ensure that no overrated relationship exists and that no multicollinearity exists. The results are correct to the suggestion of Mukaka (2012), who mentioned that the relationship between related variables should not be more than 0.8. To evaluate accuracy, a researcher must verify the square root of AVE, which is a good flag for a structural explanation. Hair et al. (2009) suggested that the square root of AVE for each factor should be larger than the correlation coefficient between the compared factors; the result aligns with this suggestion (Table 6).
This study analyzes the influence of ATU, SN, PBC, PE, EE, and FC on the BI on the HSR use of older passengers to verify the hypotheses via SEM. The MLE method was used to estimate the parameter value (Table 7), including the standardized coefficients of the paths to explain the relationship between the latent variables. The fit indices of the research model were calculated as follows:
Figure 3 presents the results of the hypothesis testing. Figure 3 shows the differences between the TPB and UTAUT path coefficients for each factor studied in the model. Therefore, a significant p-value indicates a significant difference between each factor in the studied relationship affecting the BI of older adults to use HSR. Factors with high coefficients indicate that the factor is very important, while factors with low coefficients indicate that the factor is less important. This result shows that the relationship between Hypotheses 1–3 and the TPB support factor constructs is significant. Hypotheses 4–6 positively influence BI using HSR, the UTAUT factor. Hypotheses 7, 8 positively influenced ATU. The results of the analysis follow.
Attitude toward use (ATU) has a significant positive influence on the behavioral intention of older people to use HSR. The analysis reveals that ATU has the highest coefficient among all factors examined (β = 0.483, p < 0.001), underscoring its critical role as a determinant of intention. This finding highlights that a positive attitude—shaped by perceptions of convenience, comfort, and efficiency—strongly motivates older individuals to view HSR as a viable travel option. These results emphasize the central importance of ATU in shaping the decision-making process for adopting this mode of transportation.
Perceived behavioral control (PBC) has a positive influence on the BI of older people to use HSR. The results show that PBC has a statistically significant effect (β = 0.054, p < 0.001), although its coefficient is lower than those of ATU (H1), SN (H2), and FC (H4). This finding underscores the importance of PBC in shaping older people’s intention to adopt HSR, ranking as a significant factor with less influence than other determinants.
Subjective norms positively influence the BI of older people to use HSR. The analysis reveals that the coefficient for subjective norms (β = 0.125, p < 0.001), while lower than that for ATU (H1), still makes a significant contribution to shaping BI. This finding underscores the impact of social influence, particularly from close networks such as family and friends, on older people’s decision-making process regarding HSR adoption. Social encouragement and alignment with group norms foster a sense of acceptance, thereby increasing the willingness to consider HSR as a travel option.
Facilitating conditions (FC) positively influence the BI of older individuals to HSR. In UTAUT, facilitating conditions are one of the key factors influencing behavior. The analysis results indicate that the FC coefficient (β = 0.228, p < 0.001), although lower than the coefficients for H1 and H2, still significantly impacts older people’s intention to use HSR. This influence ranked second only to their ATU and SN and serves as a motivator. Practical aspects, such as convenient access to stations (e.g., shuttle buses from local communities) and elder-friendly facilities at stations and on trains, contribute to creating a positive impression, thereby encouraging the adoption of high-speed rail services among older individuals.
Performance expectancy (PE) positively influences the BI of older individuals to adopt and use HSR. The analysis indicates that PE, as hypothesized in H5, has a statistically significant effect (β = 0.030, p < 0.001). However, this coefficient represents the lowest value among all examined factors, suggesting that PE has the least direct impact on the BI of older people in utilizing HSR. Interestingly, while PE demonstrates limited direct influence, it plays a notable indirect role, as evidenced in Hypothesis 7 (H7). Specifically, PE contributes positively to the ATU factor, highlighting a more complex relationship in which PE indirectly enhances the decision-making process for adopting HSR.
Effort expectancy (EE) positively influences the BI of older individuals to adopt and use HSR. The analysis reveals that EE has a statistically significant effect (β = 0.032, p < 0.001). However, this coefficient is relatively low compared to other factors, ranking slightly above performance expectancy in its level of impact. Similar to the findings for Hypothesis 5 (H5), EE demonstrates a limited direct influence on BI, indicating that while it contributes to intention, its effect is not as pronounced. Notably, EE indirectly impacts BI by influencing the ATU factor, as evidenced in the findings for Hypothesis 8 (H8). This underscores a nuanced relationship in which EE supports BI by shaping attitudes, ultimately encouraging older users to consider adopting HSR.
Performance expectancy (PE) positively influences the ATU HSR among older individuals. The analysis reveals that PE has a statistically significant effect (β = 0.336, p < 0.001), emphasizing its substantial role in shaping attitudes toward HSR adoption in this demographic. However, the coefficient for PE is lower than that of EE within the ATU relationship. This finding indicates that while PE contributes significantly, it is relatively less influential than EE in shaping the attitudes of older individuals toward using HSR.
Effort expectancy (EE) has a significant and positive influence on the ATU HSR among older individuals. The analysis indicates that EE (β = 0.423, p < 0.001) is statistically significant, highlighting its critical role in shaping attitudes toward adopting HSR. This finding underscores the importance of ease of use and minimal effort in enhancing the perception and willingness of older users to engage with HSR services. Older individuals place considerable value on systems and technologies that are intuitive, user-friendly, and require minimal effort to access or navigate.
Notably, the coefficient for EE exceeds that of PE in its relationship with ATU, underscoring the relatively greater importance of effort-related factors over performance-related attributes in shaping attitudes among this demographic. This trend reflects the specific needs and preferences of older users, who may prioritize convenience and simplicity over performance-related benefits when considering the adoption of high-speed rail.
These findings provide valuable insights that can inform policy recommendations aimed at increasing the adoption of HSR among older passengers.
Previous studies on the BI of older people are linked with technology use or innovation. Most of these studies mainly focused on the use of health-related technology, such as equipment or applications for the monitoring of health services (Lu and Tsai-Lin, 2024; Chen et al., 2023; Hoque and Sorwar, 2017) or other transportation platforms (Lin and Cui, 2021; Goldbach et al., 2022). Others examined the use of smart homes (Maswadi et al., 2022) and smartphones (Yang et al., 2023) among older people. However, these studies overlooked specific contexts of technology use. Thus, the current study addresses this concern by examining the BI on HSR use among older passengers in terms of long travel and in light of the new public transportation system in Thailand. It also intended to develop a usability platform suitable for older people through in-depth data on the factors that affect the BI on HSR use through TPB and UTAUT via SEM. This section describes the relationship between variables. The first is a direct relationship between ATU and BI, followed by PBC, SN, FC, PE, and EE. Moreover, the analysis follows the effect of attitude between PE and EE.
The result indicated that ATU positively influenced BI, which is statistically significant and supports H1. This result is similar to those of Dwivedi et al. (2019) and Gallardo et al. (2024). Moreover, Maswadi et al. (2022) identified that the attitude toward usability is crucial to the decision of the use intention of older people. A strategy designed to attract older people should educate them about the system’s usability (Ke et al., 2022).
The result confirms H2. This result was supported by Ghahremani et al. (2012), Liu et al. (2022), Xu et al. (2024), and Ku and Hsieh (2016), who mentioned that opinions about health were an important factor in decision making. PBC encouragement of older people may be useful to support their participation in checkups and include resources preparing or supporting older people to participate in checkups more frequently. The most recent research aligned with the study mentioned and can be applied to the context of HSR use supporting older people.
This section pertains to H3, which is supported. The result aligns with those of Wang et al. (2023), Osifeko et al. (2018), and Lu and Tsai-Lin (2024), who cited that SN could affect attitude change of older people because surrounding people are important to the users. Thus, they can convince older people to become users in the future. Moreover, Al-Rashid et al. (2021) and Al-Rashid et al. (2023) emphasized that SN significantly influences the intention of older people to use public transportation systems when older people realize that their friends or family members support its usability. These results imply that the attempt to support HSR use should be aware of SI and the strategy of participation in the community.
This result pertains to H4. The result indicated that FC positively influenced BI in the context of older passengers, which aligned with previous studies (Boontarig et al., 2012; Mun et al., 2006; Bhattacherjee and Hikmet, 2008; Yuen et al. (2022) and directly identified that FC was directly determinant of BI in technology use. It also confirmed the important role of infrastructure support. However, Yuen et al. (2022) and Becker and Axhausen (2017) examined FC and found that the resources and support needed to prepare for service users are related to the user’s belief that they will receive the necessary resources.
The result refers to H5, which displayed a significantly positive relationship between both factors (Yang et al., 2023; Zhou et al., 2020; Hoque and Sorwar, 2017). Aranha et al. (2024) identified that when older people expressed expectations of advantages, it would lead to the intention to use. Various studies showed that the expectation of efficiency influences the BI of older people. For example, Chen et al. (2023) reported that PE exerted a significantly positive effect on the intention to use medical wearable devices among older people. These devices increased the efficiency of older people’s lives, and the intention to use these devices was notable. Similarly, in transportation research, people who believe in more efficient transportation compared with the current transportation system tend to use the more efficient transportation willingly (Goldbach et al., 2022). Previous studies supported the study results that if older people perceived that HSR use would increase the efficiency of long travel, then they may tend to exhibit intention to use HSR more frequently.
H6 verified that EE exerts a positive influence on the BI of older people on HSR use. Empirical evidence revealed that the result significantly supported the hypothesis and aligned with those of other studies (Yang et al., 2023; Aranha et al., 2024; Maswadi et al., 2022; Hoque and Sorwar, 2017). The current study found that EE positively influences the BI of older people and affects decision making. Moreover, Pal et al. (2018a) and Pirzada et al. (2022) mentioned that the convenience and perceived benefit of technology use influence the perception of older people, which makes the expectation an important factor that influences the intention of behavioral performance.
This section pertains to H7 and H8, which are supported: PE and EE positively influenced ATU among older people in terms of HSR use. The result indicated that PE and EE signified ATU, which was supported by the studies of Dwivedi et al. (2019), Ren and Zhou (2023), Chen and Chang (2013), Khalilzadeh et al. (2017), and Rana et al. (2016). The results could be summarized as follows: when people realize that technology is useful and increases efficiency, they tend to develop positive attitudes toward technology use or make the decision to use the new technology. In addition, these attitudes may be specified by ease of use, such as fewer complications. The results of previous studies align with the current results.
Previous studies on the intention of older individuals to adopt technology have typically focused on areas such as healthcare, smart homes, smartphones, and others, but there has been no direct investigation into older people’s intention to use HSR. This study addresses this research gap and applies TPB and UTAUT in the analysis, resulting in new and intriguing findings related to older people’s travel. These insights contribute to the development of policies aimed at enhancing quality of life, travel, and access to HSR for older people. This research aimed to examine the relationship between BI and HSR use through the factors of TPB and UTAUT by conducting a questionnaire survey of older people (aged 60 years old and above). Respondents (3,200) from four regions in Thailand participated. Data were used to build a structural equation model using the MLE method to evaluate parameter values. The result demonstrated that a qualitative indicator of the model, such as the values of the fit indicators, aligns with the specified standard values. The factors of the hypothesis testing related to BI are ATU, PBC, SN, PE, EE, and FC. The study revealed that the factors of the TPB and UTAUT positively and statistically significantly (p = 0.001) influenced the BI on the HSR use of older people, which implied that these factors are important to the BI of travelers aged 60 years and above. The factor with the highest influence value was ATU, followed by SN and FC. In addition, this research presented the relationship between the two theories, which identified PE and EE statistically significantly (p = 0.001) affect ATU. The suggestions focus on communication and education on the use of these services for older people via familiar channels, including improving the service to be appropriate for older people by providing training, using notable guideposts, and supporting the staff.
Publicity should focus on important benefits, such as speed of travel, reliable security, and comfortable access, which help older people perceive and clearly understand the positive points of this service. The publicized strategy should use channels that are familiar and easy to use for older people, such as spreading the news to the community, senior centers, and social media platforms that they frequently use. Data presentation should be clear, using large fonts and simple language, including media for teaching, such as videos or illustrations, to enhance the understanding of the service. Examples of such advertisements are manuals of HSR use that explain usability in detail, as well as posters or brochures that clearly demonstrate and simplify the steps in buying tickets. All media should be designed to satisfy the requirements of older people and to ensure that traveling by HSR is a comfortable, safe, and friendly experience for users from all age groups.
The study suggests conducting training and meetings for information sharing with older travelers, such that they feel more familiar and confident in HSR use. These channels should be designed to cover items that will be beneficial to older people, especially ticket reservations, suggestions for the efficient use of facilities in the stations, and train schedules, which could help older passengers plan more frequent travels. Moreover, additional support should be given on the trains, such as staff who could provide special attention to older people. The staff should be responsible for services onboard, including first aid in the case of an emergency, to enhance the security and comfort of passengers. Knowledge education and support will decrease the worry of older people, which may be due to unfamiliarity with technology or the transportation system. Moreover, confidence in traveling should be encouraged; that is, older passengers should be assured that they can control and manage their travel experience safely and comfortably.
The study suggests the formulation of a friendly service way for older passengers by focusing on efficient communication and understanding their requirements. Communication should be aware of the physical limitations of older people and use simple language and detailed explanations. In addition, building motivation among older people who travel is efficient. Motivation building pertains to support for group travel by offering discounts or travel packages, including activities that encourage group travel. In this manner, older people can feel confident about travel and relieved from the worry of traveling alone. Moreover, group travel supports the feeling of belonging in the community and provides a chance to meet and interact with people, which affects the travel experience. As a result, travel can become smooth and impressive, which can encourage older people to share positive experiences and persuade friends or surrounding people to use the service more frequently.
This aspect should prioritize the design of train stations and railway cars that provide for the physical of older people to ensure comfort and safety. The design of stations and their services should be of suitable quality, including ramps and elevators to support people who require support equipment, such as wheelchairs and crutches, for mobility, and wide pathways. Moreover, designating clear and notable guideposts can help older people to easily locate facilities such as the restroom, relaxing area, or entrances and exits on the train. These accommodations not only increase comfort during travel but also encourage the intention to use the service among older passengers. In addition, providing areas for older people and their companions, including special methods of getting on and off the train, is helpful. The mentioned areas should have staff to help older people, such as pushing wheelchairs or providing assistance while they are stepping in and off trains. This comprehensive support system could help older passengers feel that traveling by HSR is easy and reduce their worry about travel.
These factors influence the feelings of users through ease of use, perceived benefits, and comfort, which can attract older people to use the service. Therefore, developing countries should be aware of the development of a public transportation system that can easily reach older people and expand services to rural areas. Doing so could increase comfort in traveling between one’s home and the station through shuttle services from the community to the train station. Staff could also be assigned to help with the travel issues of older passengers who live far from the station. Travel can be encouraged by offering discounts. These policies should be continually evaluated to ensure that they satisfy the requirements of older people. Providing training and distribution of manuals of use for older people will help enhance their understanding of the uses and benefits of the service. These policies will not only lead to positive attitudes but also increase the number of service users among older people.
Cooperation between community organizations and health service facilitators will better identify the specific requirements of older people. To increase the comfort of service use, the process of ticket purchase should be simplified, such as the use of apps, cash payment, or designation of ticket purchase areas for older people. Examples include setting up a large and readable guidepost, including real-time travel information, such as arrival and delay data, through digital channels, mobile applications, or announcements.
A limitation of this study is that it focused only on BI on HSR use among older passengers in Thailand through TPB and UTAUT. A number of variables are mediators, which cannot conclude the result in another dimension apart from the abovementioned factors. The study overlooked the moderating role of other factors such as income, occupation, chronic disease, and travel frequency, including a span of age specification of older people to compare differences in attitude that affect BI, including service use behavior. In addition, this research is only an initial study prior to the introduction of HSR use in Thailand to evaluate the readiness of older passengers.
Future studies can consider these limitations using other theories related to the motivation of older people, including personal data on income and chronic disease. In this manner, in-depth data can be collected and analyzed to identify similarities and differences in travel behavior. Another important consideration is that Thailand is a developing country, which may impose certain limitations on the generalizability of the findings. Applying these results to other countries requires careful consideration of country-specific characteristics, such as geographic conditions and quality of life, as additional influencing factors. The results could inform policymakers and other stakeholders to better identify trends in behaviors. Further studies should be conducted after the official service opening of HSR to determine satisfaction among passengers.
The raw data supporting the conclusions of this article will be made available by the authors, without undue reservation.
The studies involving humans were approved by the Ethics Committee of Suranaree University of Technology (COE No. 2/2567, 16 January 2024). The studies were conducted in accordance with the local legislation and institutional requirements. The ethics committee/institutional review board waived the requirement of written informed consent for participation from the participants or the participants’ legal guardians/next of kin because this research was deemed low risk by the Human Research Committee, which approved the use of oral consent for participant involvement.
FW: conceptualization, data curation, formal analysis, methodology, and writing–original draft. TP: conceptualization, methodology, and writing–original draft. SS: data curation, formal analysis, and writing–review and editing. TC: validation and writing–review and editing. SJ: software, validation, and writing–review and editing. PW: formal analysis, validation, and writing–review and editing. AD: methodology and writing–review and editing. VR: software, supervision, and writing–review and editing.
The author(s) declare that financial support was received for the research, authorship, and/or publication of this article. This work was supported by Suranaree University of Technology (SUT), Thailand Science Research and Innovation (TSRI), and the National Science, Research and Innovation Fund (NSRF) (Project code: 195602).
The authors express their gratitude to the Suranaree University of Technology (SUT), Thailand Science Research and Innovation (TSRI), and the National Science, Research and Innovation Fund for their support in undertaking this research.
The authors declare that the research was conducted in the absence of any commercial or financial relationships that could be construed as a potential conflict of interest.
The author(s) declare that no Generative AI was used in the creation of this manuscript.
All claims expressed in this article are solely those of the authors and do not necessarily represent those of their affiliated organizations, or those of the publisher, the editors and the reviewers. Any product that may be evaluated in this article, or claim that may be made by its manufacturer, is not guaranteed or endorsed by the publisher.
Ajzen, I. (1991). The theory of planned behavior. Organ. Behav. Hum. Decis. Process. 50, 179–211. doi:10.1016/0749-5978(91)90020-T
Alam, M. Z., Hoque, M. R., Hu, W., and Barua, Z. (2020). Factors influencing the adoption of mHealth services in a developing country: a patient-centric study. Int. J. Inf. Manag. 50, 128–143. doi:10.1016/j.ijinfomgt.2019.04.016
Almetere, E. S., Kelana, B. W. Y., and Mansor, N. N. A. (2020). Using UTAUT model to determine factors affecting internet of things acceptance in public universities. Int. J. Acad. Res. Bus. Soc. Sci. 10, 142–150. doi:10.6007/ijarbss/v10-i2/6915
Al-Rashid, M. A., Goh, H. C., Harumain, Y. A. S., Ali, Z., Campisi, T., and Mahmood, T. (2021). Psychosocial barriers of public transport use and social exclusion among older adults: empirical evidence from Lahore, Pakistan. Int. J. Environ. Res. public health 18, 185. doi:10.3390/ijerph18010185
Al-Rashid, M. A., Harumain, Y. A. S., Goh, H. C., Ali, Z., Nadeem, M., and Campisi, T. (2023). Perceived norms of public transport use as the determinants of older adults' social exclusion: evidence from Pakistan. Cities 137, 104264. doi:10.1016/j.cities.2023.104264
Aranha, M., Shemie, J., James, K., Deasy, C., and Heavin, C. (2024). Behavioural intention of mobile health adoption: a study of older adults presenting to the emergency department. Smart Health 31, 100435. doi:10.1016/j.smhl.2023.100435
Arenas Gaitán, J., Peral Peral, B., and Ramón Jerónimo, M. (2015). Elderly and internet banking: an application of UTAUT2. J. Internet Bank. Commer. 20 (1), 1–23.
Bandalos, D. L., and Finney, S. J. (2018). Factor analysis: exploratory and confirmatory. The reviewer’s guide to quantitative methods in the social sciences. Routledge.
Becker, F., and Axhausen, K. W. (2017). Literature review on surveys investigating the acceptance of automated vehicles. Transportation 44, 1293–1306. doi:10.1007/s11116-017-9808-9
Bezirgani, A., and Lachapelle, U. (2021). Online grocery shopping for the elderly in Quebec, Canada: the role of mobility impediments and past online shopping experience. Travel Behav. Soc. 25, 133–143. doi:10.1016/j.tbs.2021.07.001
Bhattacherjee, A., and Hikmet, N. (2008). Reconceptualizing organizational support and its effect on information technology usage: evidence from the health care sector. J. Comput. Inf. Syst. 48, 69–76. doi:10.1080/08874417.2008.11646036
Boontarig, W., Chutimaskul, W., Chongsuphajaisiddhi, V., and Papasratorn, B. (2012). “Factors influencing the Thai elderly intention to use smartphone for e-Health services,” in 2012 IEEE symposium on humanities, science and engineering research, 2012 Germany, (IEEE), 479–483.
Borhan, M., Ibrahim, A. N. H., and bin Miskeen, M. (2019a). Extending the theory of planned behaviour to predict the intention to take the new high-speed rail for intercity travel in Libya: assessment of the influence of novelty seeking, trust and external influence. Transp. Res. Part A Policy Pract. 130, 373–384. doi:10.1016/j.tra.2019.09.058
Borhan, M. N., Ibrahim, A. N. H., and Miskeen, M. A. A. (2019b). Extending the theory of planned behaviour to predict the intention to take the new high-speed rail for intercity travel in Libya: assessment of the influence of novelty seeking, trust and external influence. Transp. Res. Part A Policy Pract. 130, 373–384. doi:10.1016/j.tra.2019.09.058
Chen, J., Wang, T., Fang, Z., and Wang, H. (2023). Research on elderly users' intentions to accept wearable devices based on the improved UTAUT model. Front. Public Health 10, 1035398. doi:10.3389/fpubh.2022.1035398
Chen, K.-Y., and Chang, M.-L. (2013). User acceptance of ‘near field communication’mobile phone service: an investigation based on the ‘unified theory of acceptance and use of technology’model. Serv. Industries J. 33, 609–623. doi:10.1080/02642069.2011.622369
Chen, L. S.-L., Kuan, C. J., Lee, Y.-H., and Huang, H.-L. (2011). “Applicability of the UTAUT model in playing online game through mobile phones: moderating effects of user experience,” in First International Technology Management Conference, China, 27-30 June 2011 (IEEE), 625–629.
Chong, Z., Chen, Z., and Qin, C. (2019). Estimating the economic benefits of high-speed rail in China: a new perspective from the connectivity improvement. J. Transp. Land Use 12, 287–302. doi:10.5198/jtlu.2019.1264
Chuang, H., Chu, C., and Lin, Y.-T. (2011). “HSR buying behavior modeling-Taiwan High Speed Railway case,” in 2011 IEEE International Conference on Industrial Engineering and Engineering Management, China, 6-9 Dec. 2011 (IEEE), 679–683.
Cimperman, M., Brenčič, M. M., and Trkman, P. (2016). Analyzing older users’ home telehealth services acceptance behavior—applying an Extended UTAUT model. Int. J. Med. Inf. 90, 22–31. doi:10.1016/j.ijmedinf.2016.03.002
Conner, M. (2020). Theory of planned behavior. Handb. sport Psychol., 1–18. doi:10.1002/9781119568124.ch1
Cromartie, J., and Bucholtz, S. (2008). Defining the “rural” in rural America. Amber waves: the Economics of food, farming, natural resources. America: Rural America, 28–35.
Delgado-Viñas, C., and Gómez-Moreno, M.-L. (2022). The interaction between urban and rural areas: an updated paradigmatic, methodological and bibliographic review. Land 11, 1298. doi:10.3390/land11081298
Department of Older Persons (2024). Elderly statistics. Dep. Older Persons. Available at: https://www.dop.go.th/th/know/side/1/1/2449.
Dwivedi, Y. K., Rana, N. P., Jeyaraj, A., Clement, M., and Williams, M. D. (2019). Re-examining the unified theory of acceptance and use of technology (UTAUT): towards a revised theoretical model. Inf. Syst. Front. 21, 719–734. doi:10.1007/s10796-017-9774-y
Fornell, C., and Larcker, D. F. (1981). Evaluating structural equation models with unobservable variables and measurement error. J. Mark. Res. 18, 39–50. doi:10.1177/002224378101800104
Fu, X. (2021). A novel perspective to enhance the role of TPB in predicting green travel: the moderation of affective-cognitive congruence of attitudes. Transportation 48, 3013–3035. doi:10.1007/s11116-020-10153-5
Gallardo, M. O., Dela Torre, J., and Ebardo, R. (2024). The role of initial trust in the behavioral intention to use telemedicine among Filipino older adults. Gerontology Geriatric Med. 10, 23337214231222110. doi:10.1177/23337214231222110
Gefen, D., Straub, D., and Boudreau, M.-C. (2000). Structural equation modeling and regression: guidelines for research practice. Commun. Assoc. Inf. Syst. 4, 7. doi:10.17705/1cais.00407
Ghahremani, L., Niknami, S., and Nazari, M. (2012). The prediction of physical activity intention and behavior in elderly male residents of a nursing home: a comparison of two behavioral theories. Iran. J. Med. Sci. 37, 23–31.
Goldbach, C., Sickmann, J., Pitz, T., and Zimasa, T. (2022). Towards autonomous public transportation: attitudes and intentions of the local population. Transp. Res. Interdiscip. Perspect. 13, 100504. doi:10.1016/j.trip.2021.100504
Hair Jr., J. F., Black, W. C., Babin, B. J., and Anderson, R. E. (2009). Multivariate Data Analysis. 7th Edn. Upper Saddle River: Prentice Hall, 761.
Hooper, D., Coughlan, J., Mullen, M., and Hooper, D. (2008). Structural equation modelling: guidelines for determining model fit. Electron J. Bus. Res. Methods. 6 (1), 53–60.
Hoque, R., and Sorwar, G. (2017). Understanding factors influencing the adoption of mHealth by the elderly: an extension of the UTAUT model. Int. J. Med. Inf. 101, 75–84. doi:10.1016/j.ijmedinf.2017.02.002
Hou, Z., Liang, L. J., Meng, B., and Choi, H. C. (2021). The role of perceived quality on high-speed railway tourists’ behavioral intention: an application of the extended theory of planned behavior. Sustainability 13, 12386. doi:10.3390/su132212386
Hsiao, C.-H., and Yang, C. (2010). Predicting the travel intention to take High Speed Rail among college students. Transp. Res. Part F Traffic Psychol. Behav. 13, 277–287. doi:10.1016/j.trf.2010.04.011
Hsu, C.-W., and Peng, C.-C. (2022). What drives older adults’ use of mobile registration apps in Taiwan? An investigation using the extended UTAUT model. Inf. Health Soc. Care 47, 258–273. doi:10.1080/17538157.2021.1990299
Hu, L., and Bentler, P. M. (1999). Structural Equation Modeling: a Multidisciplinary Journal Cutoff criteria for fit indexes in covariance structure analysis: conventional criteria versus new alternatives. Struct. Equ. Model. 6, 37–41. doi:10.1080/10705519909540118
Joshi, A., Kale, S., Chandel, S., and Pal, D. K. (2015). Likert scale: explored and explained. Br. J. Appl. Sci. and Technol. 7, 396–403. doi:10.9734/bjast/2015/14975
Kamga, C. (2015). Emerging travel trends, high-speed rail, and the public reinvention of US transportation. Transp. Policy 37, 111–120. doi:10.1016/j.tranpol.2014.10.012
Ke, L.-S., Cheng, H.-C., Ku, Y.-C., Lee, M.-J., Chang, S.-Y., Huang, H.-Y., et al. (2022). Older adults' Behavioral intentions toward advance care planning based on theory of reasoned action. J. Hospice and Palliat. Nurs. 24, E294–E300. doi:10.1097/njh.0000000000000907
Khalilzadeh, J., Ozturk, A. B., and Bilgihan, A. (2017). Security-related factors in extended UTAUT model for NFC based mobile payment in the restaurant industry. Comput. Hum. Behav. 70, 460–474. doi:10.1016/j.chb.2017.01.001
Kline, R. B. (2023). Principles and practice of structural equation modeling. Guilford publications.
Ku, W.-T., and Hsieh, P.-J. (2016). “Acceptance of cloud-based healthcare services by elderly Taiwanese people. Human Aspects of IT for the Aged Population. Design for Aging,” in Second International Conference, ITAP 2016, Held as Part of HCI International 2016, Toronto, ON, Canada, July 17–22, 2016 (Springer), 186–195.
Li, J., Hsu, C.-C., and Lin, C.-T. (2019). Leisure participation behavior and psychological well-being of elderly adults: an empirical study of Tai Chi Chuan in China. Int. J. Environ. Res. Public Health 16, 3387. doi:10.3390/ijerph16183387
Lin, D., and Cui, J. (2021). Transport and mobility needs for an ageing society from a policy perspective: review and implications. Int. J. Environ. Res. Public Health 18, 11802. doi:10.3390/ijerph182211802
Liu, J., Liu, L., and Pei, M. (2022). Analysis of older people's walking behavioral intention with the extended theory of planned behavior. J. Transp. and Health 26, 101462. doi:10.1016/j.jth.2022.101462
Lu, C.-C., and Tsai-Lin, T.-F. (2024). Are older adults special in adopting public eHealth service initiatives? The modified model of UTAUT. SAGE Open 14, 21582440241228639. doi:10.1177/21582440241228639
Lyu, G., Ma, W., Wang, J., and Jiao, J. (2024). How does high-speed rail affect intercity elderly migration? Evidence from China. Res. Transp. Bus. and Manag. 57, 101200. doi:10.1016/j.rtbm.2024.101200
Ma, Q., Chan, A., and Teh, P.-L. (2021). Insights into older adults’ technology acceptance through meta-analysis. Int. J. Human-Computer Interact. 37, 1049–1062. doi:10.1080/10447318.2020.1865005
Ma, Q., Chan, A. H., and Chen, K. (2016). Personal and other factors affecting acceptance of smartphone technology by older Chinese adults. Appl. Ergon. 54, 62–71. doi:10.1016/j.apergo.2015.11.015
Magsamen-Conrad, K., Wang, F., Tetteh, D., and Lee, Y.-I. (2020). Using technology adoption theory and a lifespan approach to develop a theoretical framework for eHealth literacy: extending UTAUT. Health Commun. 35, 1435–1446. doi:10.1080/10410236.2019.1641395
Mahardika, M. D., Irawan, M. Z., and Bastarianto, F. F. (2022). Exploring the potential demand for Jakarta–Bandung high-speed rail. Transp. Res. Interdiscip. Perspect. 15, 100658. doi:10.1016/j.trip.2022.100658
Martins, C., Oliveira, T., and Popovič, A. (2014). Understanding the Internet banking adoption: a unified theory of acceptance and use of technology and perceived risk application. Int. J. Inf. Manag. 34, 1–13. doi:10.1016/j.ijinfomgt.2013.06.002
Maswadi, K., Ghani, N. A., and Hamid, S. (2022). Factors influencing the elderly’s behavioural intention to use smart home technologies in Saudi Arabia. PLoS One 17, e0272525. doi:10.1371/journal.pone.0272525
Mountrakis, G., AvRuskin, G., and Beard, K. (2005). “Modeling rurality using spatial indicators,” in Proceedings of the 8th International Conference on GeoComputation, Berlin, June 23 - 25, 2008 (Citeseer).
Mukaka, M. M. (2012). Statistics corner: a guide to appropriate use of correlation coefficient in medical research. Malawi Med. J. 24, 69–71.
Mun, Y. Y., Jackson, J. D., Park, J. S., and Probst, J. C. (2006). Understanding information technology acceptance by individual professionals: toward an integrative view. Inf. and Manag. 43, 350–363. doi:10.1016/j.im.2005.08.006
Nanthamongkolchai, S., Tojeen, A., Munsawaengsub, C., Yodmai, K., and Suksatan, W. (2022). Quality of life of older adults with physical and mobility disabilities during the COVID-19 pandemic: a cross-sectional study in Thailand. Sustainability 14, 8525. doi:10.3390/su14148525
National Statistical Office (2021). Situation of Thai elderly in the spatial dimension 2021. https://www.nso.go.th/nsoweb/storage/ebook/2023/20230506145038_72776.pdf.
Osifeko, M., Oyedeji, A., Abolade, O., Alao, P., Ade-Ikuesan, O., and Folorunsho, O. (2018). An empirical study of unified theory of acceptance and use of technology model for e-health technology in Lagos, Nigeria. Futo J. Ser. (FUTOJNLS) 4, 117–126.
Pal, D., Funilkul, S., Charoenkitkarn, N., and Kanthamanon, P. (2018a). Internet-of-things and smart homes for elderly healthcare: an end user perspective. IEEE Access 6, 10483–10496. doi:10.1109/access.2018.2808472
Pal, D., Triyason, T., Funilkul, S., and Chutimaskul, W. (2018b). Smart homes and quality of life for the elderly: perspective of competing models. IEEE Access 6, 8109–8122. doi:10.1109/ACCESS.2018.2798614
Peterson, R. A. (1994). A meta-analysis of Cronbach's coefficient alpha. J. consumer Res. 21, 381–391. doi:10.1086/209405
Pirzada, P., Wilde, A., Doherty, G. H., and Harris-Birtill, D. (2022). Ethics and acceptance of smart homes for older adults. Inf. Health Soc. Care 47, 10–37. doi:10.1080/17538157.2021.1923500
Rana, N. P., Dwivedi, Y. K., Williams, M. D., and Weerakkody, V. (2016). Adoption of online public grievance redressal system in India: toward developing a unified view. Comput. Hum. Behav. 59, 265–282. doi:10.1016/j.chb.2016.02.019
Ren, Z., and Zhou, G. (2023). Analysis of driving factors in the intention to use the virtual nursing home for the elderly: a modified UTAUT model in the Chinese context. Healthcare 2023. doi:10.3390/healthcare11162329
Robru, K., Setthasuravich, P., Pukdeewut, A., and Wetchakama, S. (2024). Internet use for health-related purposes among older people in Thailand: an analysis of nationwide cross-sectional data. Informatics 11, 55. doi:10.3390/informatics11030055
Schumacker, R. E., and Lomax, R. G. (2004). A beginner's guide to structural equation modeling. United Kingdom: psychology press.
Siripipatthanakul, S., Limna, P., Sriboonruang, P., and Kaewpuang, P. (2023). Applying the TPB and the UTAUT models predicting intentions to use telemedicine among Thai people during the COVID-19 pandemic. Int. J. Comput. Sci. Res. 7, 1362–1384. doi:10.25147/ijcsr.2017.001.1.107
Soh, P., Heng, H., Selvachandran, G., Le, A., Chau, H., Son, L., et al. (2020). RETRACTED ARTICLE: perception, acceptance and willingness of older adults in Malaysia towards online shopping: a study using the UTAUT and IRT models. J. Ambient Intell. Humaniz. Comput. 15, 101. doi:10.1007/s12652-020-01718-4
Songmuang, T. (2016). An analysis of Thailand's opportunities and benefits from rail development plans under the master plans on ASEAN connectivity. RSU Int. J. Coll. Gov. (RSUIJCG) 3.
Srichuae, S., Nitivattananon, V., and Perera, R. (2015). Aging society in Bangkok and the factors affecting mobility of elderly in urban public spaces and transportation facilities. IATSS Res. 40, 26–34. doi:10.1016/j.iatssr.2015.12.004
Stolte, E., Hopman-Rock, M., Aartsen, M. J., Van Tilburg, T. G., and Chorus, A. (2017). The theory of planned behavior and physical activity change: outcomes of the aging well and healthily intervention program for older adults. J. aging Phys. activity 25, 438–445. doi:10.1123/japa.2016-0182
Tabachnick, B. G., and Fidell, L. S. (2013). Using multivariate statistics. International edition. Pearson2012.
Tarka, P. (2018). An overview of structural equation modeling: its beginnings, historical development, usefulness and controversies in the social sciences. Qual. and quantity 52, 313–354. doi:10.1007/s11135-017-0469-8
Transport (2024). A cooperation project between the Government of the Kingdom of Thailand and the Government of the People's Republic of China for the development of a high-speed rail system to enhance regional connectivity. Available at: https://www.highspeedrail-thai-china.com/project/.
Venkatesh, V., Morris, M. G., Davis, G. B., and Davis, F. D. (2003). User acceptance of information technology: toward a unified view. MIS Q. 27, 425–478. doi:10.2307/30036540
Wang, X., He, Y., and Zhang, H. (2023). How to influence behavioral intention toward age-friendly home modifications in urban older people aged 70. Gerontology geriatric Med. 9, 23337214231152697. doi:10.1177/23337214231152697
Wheaton, B., Muthen, B., Alwin, D. F., and Summers, G. F. (1977). Assessing reliability and stability in panel models. Sociol. Methodol. 8, 84–136. doi:10.2307/270754
Williams, M. D., Rana, N. P., and Dwivedi, Y. K. (2015). The unified theory of acceptance and use of technology (UTAUT): a literature review. J. Enterp. Inf. Manag. 28, 443–488. doi:10.1108/jeim-09-2014-0088
Xu, J., Pan, Y., and Li, Q. (2024). Influencing factors of health screening among retirees: an extended TPB approach. Front. Public Health 12, 1320920. doi:10.3389/fpubh.2024.1320920
Yamin, M. A. Y., and Alyoubi, B. A. (2020). Adoption of telemedicine applications among Saudi citizens during COVID-19 pandemic: an alternative health delivery system. J. Infect. public health 13, 1845–1855. doi:10.1016/j.jiph.2020.10.017
Yang, C.-C., Liu, C., and Wang, Y.-S. (2023). The acceptance and use of smartphones among older adults: differences in UTAUT determinants before and after training. Libr. Hi Tech. 41, 1357–1375. doi:10.1108/LHT-12-2021-0432
Yang, H., Burghouwt, G., Wang, J., Boonekamp, T., and Dijst, M. (2018). The implications of high-speed railways on air passenger flows in China. Appl. Geogr. 97, 1–9. doi:10.1016/j.apgeog.2018.05.006
Yuen, K. F., Choo, L. Q., Li, X., Wong, Y. D., Ma, F., and Wang, X. (2022). A theoretical investigation of user acceptance of autonomous public transport. Transportation 50, 545–569. doi:10.1007/s11116-021-10253-w
Keywords: structural equation modeling, mediator, intention, policymakers, psychological theories
Citation: Watcharamaisakul F, Phojaem T, Sum S, Champahom T, Jomnonkwao S, Wisutwattanasak P, Dangbut A and Ratanavaraha V (2025) Perspectives of older people toward Thai high-speed rail promotion using the theory of planned behavior and the unified theory of acceptance and use of technology. Front. Built Environ. 11:1536080. doi: 10.3389/fbuil.2025.1536080
Received: 28 November 2024; Accepted: 08 January 2025;
Published: 11 February 2025.
Edited by:
Sakdirat Kaewunruen, University of Birmingham, United KingdomReviewed by:
Serdar Dindar, Yıldırım Beyazıt University, TürkiyeCopyright © 2025 Watcharamaisakul, Phojaem, Sum, Champahom, Jomnonkwao, Wisutwattanasak, Dangbut and Ratanavaraha. This is an open-access article distributed under the terms of the Creative Commons Attribution License (CC BY). The use, distribution or reproduction in other forums is permitted, provided the original author(s) and the copyright owner(s) are credited and that the original publication in this journal is cited, in accordance with accepted academic practice. No use, distribution or reproduction is permitted which does not comply with these terms.
*Correspondence: Sajjakaj Jomnonkwao, c2FqamFrYWpAc3V0LmFjLnRo
Disclaimer: All claims expressed in this article are solely those of the authors and do not necessarily represent those of their affiliated organizations, or those of the publisher, the editors and the reviewers. Any product that may be evaluated in this article or claim that may be made by its manufacturer is not guaranteed or endorsed by the publisher.
Research integrity at Frontiers
Learn more about the work of our research integrity team to safeguard the quality of each article we publish.