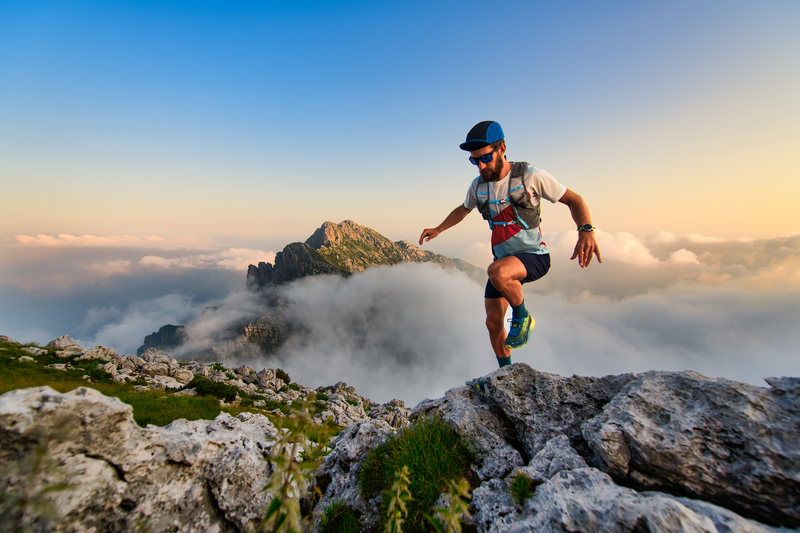
95% of researchers rate our articles as excellent or good
Learn more about the work of our research integrity team to safeguard the quality of each article we publish.
Find out more
ORIGINAL RESEARCH article
Front. Built Environ. , 12 December 2024
Sec. Wind Engineering and Science
Volume 10 - 2024 | https://doi.org/10.3389/fbuil.2024.1485388
This article is part of the Research Topic NHERI 2015-2025: A Decade of Discovery in Natural Hazards Engineering View all 7 articles
The United States experiences more extreme wind events than any other country due to its diverse climate and geographical features. While these events pose significant threats to society, they generate substantial data that can support researchers and disaster managers in resilience planning. This research leverages such data to develop a framework that automates the extraction and summarization of structural and community damage information from reconnaissance reports. The framework utilizes the large Bidirectional and Auto-Regressive Transformers model (BART-large), a deep learning model fine-tuned on the Multi-Genre Natural Language Inference (MNLI) and Cable News Network (CNN) Daily Mail datasets for these tasks. Specifically, the BART-large MNLI model employs zero-shot text classification to identify sentences containing relevant impact information based on user-defined keywords, minimizing the need for fine-tuning the model on wind damage-related datasets. Subsequently, the BART-large CNN model generates comprehensive summaries from these sentences, detailing structural and community damage. The performance of the framework is assessed using reconnaissance reports published by the Structural Extreme Events Reconnaissance (StEER), part of the Natural Hazards Engineering Research Infrastructure (NHERI) network. Particularly, the initial evaluation is conducted with the 2022 Hurricane Ian report. This is followed by a verification of the BART-large MNLI model’s capability to extract impact sentences, utilizing the 2023 Hurricane Otis report. Finally, the versatility of the framework is illustrated through an extended application to the 2023 Türkiye earthquake sequences report, highlighting its adaptability across diverse disaster contexts.
Statistics from the United States spanning from 1980 to July 2024 indicate that tropical cyclones and severe storms are the most frequent and costly natural disasters, with damages totaling $1,912.5B (69.3% of the total cost) (NCEI, 2024). Notable hurricanes such as Katrina in 2005 ($200B), Harvey in 2017 ($158.8B), and Ian in 2022 ($118.5B) have inflicted severe financial tolls. In comparison, severe storms like the two consecutive Midwest/Southeast Tornadoes in April and May 2011 ($14.3B and $12.6B), were less costly but posed significant challenges to disaster management due to their short forecast times.
Despite the substantial economic losses, these extreme wind events, and natural hazards in general, generate vast amounts of data that can aid researchers and disaster managers in mitigating their impacts. These datasets typically exhibit the defining characteristics of big data, known as the 3Vs (Laney, 2001): significant Volume, high Velocity reflected by rapid data generation across all disaster phases, and wide Variety, with data spanning various formats and sources like texts and images from social media and news outlets. Subsequently, three additional aspects—Veracity, Value, and Complexity—have become crucial, underscoring the importance of data quality, the utility of insights extracted from data, and the challenges of data management (Kaisler et al., 2013; Patgiri and Ahmed, 2016). To overcome the challenges presented by these 6Vs, machine learning (ML), a subset of artificial intelligence (AI) focused on developing algorithms that learn from data to make predictions, and deep learning (DL), a branch of ML that employs artificial neural networks to model complex patterns in ways mimicking human decision-making, have been widely utilized. These techniques are pivotal in analyzing big data from disasters, with key resources including real-time Twitter data and structured disaster response documentation such as humanitarian and reconnaissance reports.
Over the last decade, ML and DL algorithms have been increasingly used to extract disaster-related information from Twitter. Particularly, Ikonomakis et al. (2005) leveraged classical ML algorithms to collect disaster-related tweets. Verma et al. (2011) assessed the impact of linguistic features on text classification efficiency. Imran et al. (2013) trained the Naïve Bayesian (NB) model to extract the disaster response nuggets from the 2011 Joplin Tornado-related tweets. Addressing challenges in using hazard-related tweets for model training, Imran et al. (2016) published a multi-lingual, annotated corpus of 52 million tweets. Nguyen et al. (2017) employed Convolutional Neural Networks (CNN) to accelerate the extraction process, and Kersten et al. (2019) examined the robustness and transferability of CNNs across various hazards, utilizing real-time data streams. Finally, ALRashdi and O’Keefe (2019) compared crisis tweet extraction methods by using Bidirectional Long Short-Term Memory (Bi-LSTM) networks.
Twitter, with its diverse yet subjective and inconsistent content, underscores the necessity for more reliable information sources in disaster response. Additionally, the varying needs of different research groups highlight the importance of developing objective, systematic resources like humanitarian and reconnaissance reports. A recent study by Riezebos (2019) involved extracting sentences from humanitarian reports that detail the impacts of natural hazards. A Linear Support Vector Machine model, with an F1 score of around 0.8, was selected as the most effective. This study contributed to the Impact-Based Forecasting tool of the 510 initiative, backed by the Netherlands Red Cross, to assist disaster managers in predicting future disaster impacts.
While humanitarian documentation focuses on the needs and resources required for disaster response—primarily targeting aid organizations and government agencies—this study aims to extract the impacts of these disasters on infrastructure and community from reconnaissance reports. These include building damages, lifeline system failures, population displacement, and economic disturbance. It is crucial to include the communities in the framework as they are directly affected by these hazards. This approach aligns with the resilience definitions since the 2000s that integrate infrastructure and community into resilience frameworks (Koliou et al., 2020).
This research has two main objectives. The first objective is to identify impact sentences from wind disaster reconnaissance reports. This process involves analyzing a particular report to retrieve sentences that provide relevant damage information. The second objective is to generate comprehensive summaries from that reconnaissance report. These summaries serve two important purposes: First, they distill the key points of a report, allowing researchers to quickly grasp essential information from areas of interest. Second, if integrated into data repositories like Design Safe—a cyberinfrastructure for civil engineering—these summaries could enhance the querying system for disaster-related data. This improvement would allow users to perform more nuanced searches using user-defined keywords to automatically extract and summarize information from various reports. This purpose is in line with the decadal vision of the Natural Hazard Engineering Research Infrastructure (NHERI) Schneider and Kosters (2024), which aims to incorporate AI and advanced analytics tools into cyberinfrastructure to improve dataset querying.
Employing supervised and deep learning algorithms to extract impact information from reconnaissance reports presents challenges due to the sequential nature of the content. For instance, a Linear Support Vector Machine (LSVM) analyzes words or sequences independently without considering contextual relationships. Conversely, Long Short-Term Memory (LSTM) networks process data sequentially, allowing them to capture long-range textual dependencies. However, they demand considerable computational resources for large datasets, and their complex architecture requires a deeper understanding of implementation, making them more challenging to use than traditional ML models. Meanwhile, CNNs are proficient at extracting local features but lack the inherent design to comprehend long-range dependencies and sequential context, which are vital for effective natural language processing (NLP).
The Transformer architecture, a type of DL model, addressed these shortcomings through its self-attention mechanism (Vaswani et al., 2017). This mechanism allows each part of the sequence to attend to every other part directly, enabling the model to capture long-range dependencies, parallel data processing, and mitigate the vanishing gradient problem. In line with these advancements, the Transformers library emerged as an open-source platform, offering various pre-trained Transformer-based models (Wolf et al., 2020) for NLP applications.
One common method of leveraging pre-trained models for targeted tasks is fine-tuning. For example, Liu et al. (2021) built CrisisBERT upon the Bidirectional Encoder Representations from Transformers (BERT) (Devlin et al., 2019) to detect crisis-related content in tweets. Additionally, Alam et al. (2021) introduced CrisisBench, a benchmarking framework designed to evaluate the effectiveness of various DL and transformer architectures in disaster response applications. Another approach is zero-shot classification, which utilizes pre-trained models for tasks they were not originally trained to perform. In this vein, Yin et al. (2019) conducted benchmarking that included an evaluation of model performance on Zero-Shot Text Classification (0SHOT-TC), using BERT models trained on three mainstream datasets. Facebook AI researchers (Lewis et al., 2020) extended this idea by fine-tuning the large version of the Bidirectional and Auto-Regressive Transformer (BART) model on the Multi-Genre Natural Language Inference (MNLI) dataset (Williams et al., 2018), creating BART-large MNLI model. Originally applied to BERT, this method employs pre-trained Natural Language Inference models as zero-shot sequence classifiers by which the input sequence serves as the premise, while the labels serve as hypotheses. The labels are assigned to the input sequence based on their likelihood of entailment and contradiction.
The selection of ML models for this research is driven by the 0SHOT-TC approach, chosen due to the limited availability of training datasets related to wind damage. Particularly, the BART-large MNLI model is used to extract impact sentences from wind disaster reconnaissance reports. Sentences from these reports are considered premises, and the model uses predefined keywords related to hazard damage as hypotheses to classify impact information. For summaries generation, the large version of the BART model, fine-tuned on the Cable News Network (CNN) Daily Mail dataset (BART-large CNN) (Hermann et al., 2015; See et al., 2017), is employed to generate comprehensive summaries from these impact sentences.
Building upon the advancements within the Transformer family, the fourth iteration of the Generative Pre-trained Transformer (GPT-4) (OpenAI, 2023a) excels in advanced text generation and comprehension, capable of handling a wide range of tasks such as content extraction and summarization without specific training datasets. An upgraded version, GPT-4O (O for Omni) (OpenAI, 2024), extends these capabilities to support human-computer interaction across multiple formats, processing and generating outputs in text, audio, images, and video. However, the application of these models in this research is limited due to their generative nature, which can produce inconsistent results from identical prompts. This inconsistency undermines the reliability required for accurate data synthesis, including but not limited to extracting data on wind damage from reconnaissance reports. To mitigate this issue, the OpenAI Red Teaming Network, comprising experts across various fields, has been formed to rigorously evaluate these models on domain-specific tasks (OpenAI, 2023b). Continued research is essential to assess their effectiveness in analyzing damage from extreme wind events and other natural hazards.
Essentially, this study aims to develop a framework for automatically extracting and summarizing structural and community damage information from wind disaster reconnaissance reports, utilizing the BART-large MNLI and BART-large CNN models. Organized into several sections, the paper starts with an overview of the available datasets, followed by a description of the methodology. Using keywords obtained from documents up to 2022, the framework is first evaluated with the 2022 Hurricane Ian report and then verified with the 2023 Hurricane Otis report, both provided by the Structural Extreme Events Reconnaissance (StEER), part of the NHERI network. To demonstrate the adaptability of the framework to other hazards, its application is extended to the 2023 Türkiye Earthquake report from StEER by simply modifying the keyword list. Subsequently, the paper introduces the implementation framework, assesses computational efficiency, discusses limitations and future works, and concludes with final remarks.
This section first offers an overview of datasets released by various organizations, each producing reports that vary in format, content, and intended audience. Notably, the actual readership of these reports often extends beyond the intended audience, with diverse interests in the specific information presented. Despite the wealth of data available, effectively accessing it remains a significant challenge. The organizations are categorized as follows:
• Government Emergency Management Agencies: This includes the Federal Emergency Management Agency (FEMA), state-level emergency management agencies, and county emergency management offices.
• Federal Scientific and Engineering Agencies: Notable agencies are the U.S. Geological Survey (USGS), the National Oceanic and Atmospheric Administration (NOAA), and the U.S. Army Corps of Engineers (USACE).
• Other Organizations: Examples include the American Society of Civil Engineers (ASCE) and the Structural Extreme Events Reconnaissance (StEER).
Although resources provided by these entities are publicly available, this overview prioritizes damage reports from FEMA and StEER, as they focus more on collecting and analyzing structural and community damage. Between these two, StEER is preferred for its rapid publication cycle and more manageable report lengths. Typically, StEER reports are published within 2 months, significantly faster than FEMA’s Mitigation Assessment Team (MAT) reports, which can take over a year to publish due to additional focus on building code recommendations. This rapid update cycle and targeted focus on damage assessment are well-aligned with the research objectives, which include extracting and summarizing impact information from reconnaissance reports. Furthermore, StEER reports generally range from 40 to 150 pages, making them more suitable for the summary generation processes in this study (Section 3.4), compared to MAT reports, which span between 200 and 400 pages and may require multiple summaries to cover detailed aspects of infrastructure and community damage thoroughly. The following sections will delve into the background, purpose, outputs, and archiving practices of StEER, detailing their contributions to disaster data collection and analysis.
StEER Network was established in 2018 as part of the Natural Hazards Engineering Research Infrastructure (NHERI) CONVERGE node (Peek et al., 2020), funded by the National Science Foundation. It conducts post-disaster reconnaissance on natural hazards that have significant potential for generating new knowledge (Kijewski-Correa et al., 2021). The level of response by StEER can vary from 1 to 3, as illustrated in Figure 1, involving key groups such as Virtual Assessment Structural Teams (VAST), Field Assessment Structural Teams (FAST), Response Coordination, and Data Librarians. Their outputs include Preliminary Virtual Reconnaissance Reports (PVRR), Early Access Reconnaissance Reports (EARR), curated datasets, and journal papers, providing insights into hazard characteristics, local building codes, building and infrastructure performance, geotechnical performance, coastal protective systems performance, and recommended response strategies.
Figure 1. StEER Tiered Response Phasing Model (Source: StEER Network).
Decisions on team engagement and product delivery are driven by the Activation and Escalation Criteria, which consider the severity of the hazard, the level of infrastructure exposure, and the practicability of mounting a response. All published reports are archived in the Data Depot at DesignSafe—a cyberinfrastructure platform dedicated to managing extensive data from natural hazards engineering research (Pinelli et al., 2020). Its web interface enables users to manage data through browsing, uploading, downloading, sharing, curating, and publishing (Rathje et al., 2017).
This study utilizes recent PVRR reports from both hurricane and seismic events. These reports synthesize and provide commentary on hazard characteristics, local building codes, building and infrastructure performance, geotechnical conditions, and recommended response strategies. The hurricane reports are initially used to evaluate and verify the performance of the framework. The seismic report is then utilized to demonstrate its versatility of when applied to other hazards. Table 1 provides an overview of each report, including the number of pages, the number of post-processed sentences, and the total number of words.
Figure 2 presents a framework for automatic extraction and summarization of impact information from damage reports, focusing on structural and community damage from extreme wind events. It includes five main steps: data preprocessing, data labeling, extracting impact sentences, analyzing the results, and generating comprehensive summaries. Each step plays a crucial role in processing reconnaissance documents into informative impact summaries.
The least error-prone process to retrieve text from damage reports stored in Portable Document Format (PDF) is outlined here. Initially, the typical document structure is considered to determine the level of granularity for text extraction. Figure 3 shows that the structure contains sections, paragraphs, sentences, words (unigrams, bigrams, etc.), and individual characters. Qualitatively, words and single characters would be insufficient to represent impact information, thus resulting in under-specification. In contrast, for sections and paragraphs, non-impact data can be intertwined with impact data, leading to over-specification. Sentences strike a balance between these two extremes; therefore, the data is extracted in the form of sentences. Due to the challenges posed by PDFs in preserving exact text positions, such as non-structured text and unclear boundaries between tables and paragraphs, it is beneficial to convert PDFs into a more editable format like.docx. This can be done using advanced PDF editing and conversion software. After conversion, the document becomes more accessible for processing with Python preprocessing code to extract sentences efficiently.
Before labeling impact sentences, it is important to establish the definition of impact sentence. In this context, impact refers to structural and community damages caused by a specific event, such as wind. Structural damage pertains to impacts on the physical infrastructure such as buildings and bridges, while community damage includes societal effects like loss of lives and livelihoods. A sentence is classified as an impact sentence if its content relates to keywords associated with damage caused by the hazard. This keyword-based classification method is informed by the BART-large MNLI model, as described in Section 3.3.1. For example, sentences containing keywords related to structural damage—categorized by building types, components, or damage terms—are classified as structural impact sentences. The creation of the list of keywords for both structural and community damage is provided in Table 2 in Section 3.3.2.
The entire dataset was manually annotated by the authors using the reports outlined in Section 2.2. This dataset is then used to evaluate the ability of the framework to identify impact sentences. Examples of this process, particularly for Hurricane Ian’s PVRR, are presented in Section 4.2.
The classification of impact sentences utilizes a keyword-based approach with the large Bidirectional Auto-Regressive Transformer fine-tuned on the Multi-Genre Natural Language Inference dataset (BART-large MNLI). Specifically, sentences containing relevant keywords to either structural or community damage are labeled as impact, whereas unrelated sentences are marked as non-impact. The large scale of the model and its diverse training allow it to handle topics like wind disaster impacts without requiring additional fine-tuning on specialized datasets for wind damage, which are typically scarce.
BART (Lewis et al., 2020) is a denoising autoencoder designed for sequence-to-sequence models, which excels at various NLP downstream tasks involving sequence classification, token classification, sequence generation, and machine translation. It integrates the bidirectional encoder from BERT (Devlin et al., 2019) and the autoregressive decoder from GPT (Radford et al., 2018) within the Transformer architecture (Vaswani et al., 2017). The model is trained on text corrupted by masking spans of content to reconstruct the original sequences. Such training makes the model adept at understanding and correcting distortions in the input. Figure 4 provides a visual representation of the BART architecture. Specifically, the bidirectional encoder processes each sequence from both directions to grasp the overall context, which is then passed to the autoregressive decoder to regenerate the input sequence. To enhance training efficiency, BART incorporates the Gaussian Error Linear Unit (GeLU) activation function, which allows for the passage of small negative gradients (Hendrycks and Gimpel, 2016). Additionally, it initializes the training weights following a normal distribution of zero mean and standard deviation of 0.02, facilitating more effective training convergence.
The model is available in two sizes: the base model with 6 encoder and decoder layers totaling 140 M parameters, and the large variant with 12 of each, consisting of 400 M parameters. This increased capacity enables BART to capture more complex patterns and nuances in the data, potentially improving performance. However, higher computational resources are required for training and implementation.
BART has been adapted for sequence classification tasks, specifically for Zero-Shot Text Classification (0SHOT-TC), based on a method outlined in Yin et al. (2019). This method involves training models to perform Natural Language Inference on entailment datasets for 0SHOT-TC. Specifically, the model was fine-tuned on the Multi Nomial Language Inference (MNLI) dataset (Williams et al., 2018), which contains roughly 433 k sentence pairs from diverse genres. Each pair includes a premise and a hypothesis, with the pairs labeled as entailment, neutrality, or contradiction depending on the relationship between the two. This extensive and diverse dataset enables BART to classify text by assessing its compatibility with labels across these three dimensions.
This research implements the BART-large MNLI model using sentences extracted from reconnaissance reports as premises and predefined keywords related to wind disasters as hypotheses. These keywords are systematically categorized and derived from sources such as StEER reports (published before 2023), FEMA technical documents (prior to 2023), and ASCE 7-22. The initial list of keywords is selected based on their frequency in these documents, and closely related keywords are grouped and generalized according to the authors’ expertise. The focus is primarily on structural damage, encompassing elements such as building types, components, other forms of infrastructure, and relevant structural terms. To enhance model accuracy, the term damage is appended to keywords related to building types, components, and infrastructure, ensuring that the model accurately identifies sentences discussing damage rather than merely describing structures. Owing to the primary focus of reconnaissance reports on building performance, keywords pertaining to community damage are grouped into a single category. Additionally, keywords that describe the extent of hazards are included in the terrestrial damage category. A separate list of keywords indicative of non-impact sentences is also compiled to help exclude irrelevant sentences. Table 2 presents the keywords used for identifying the impacts of wind disasters, following the categories mentioned.
BART, as a Transformer architecture variant, is integrated into the Hugging Face Transformers library (Wolf et al., 2020), providing a standardized framework for implementation. The end-to-end implementation for BART-large MNLI, illustrated in Figure 5, begins by dividing the dataset into smaller batches to manage the computational demands of the model, which has approximately 407 million parameters. This pipeline consists of three main stages: encoding, model processing, and decoding. During the encoding stage, raw text is converted into a format that the model can process. Each sentence within a batch is paired with relevant keywords to form sentence pairs. Subsequently, each of them undergoes tokenization—the process of breaking down text into smaller units, called tokens, which can be as small as words or subwords. This step is crucial for capturing nuanced meanings, enabling the model to process language more effectively. The specific tokenizer from BART-large MNLI is used, following its tokenization scheme. Post-tokenization, each token is converted into a unique ID. This step is fundamental as the model operates on numerical data, with these IDs enabling it to process the text. It is important to note that sentence pairs vary in length and are thus tokenized independently. To stack them into a 2D tensor, techniques like padding zeros and truncation are applied. This standardization ensures uniform tensor lengths for each sentence pair. The resulting 2D tensor serves as the input to the model processing stage, representing the textual data in a structured, machine-readable format.
The BART-large MNLI model processes a 2D tensor of tokenized IDs, which is optimized for sequence classification tasks. Both the encoder and decoder receive this tensor as their input. The key output of the model is the final hidden state from the last token of the decoder, capturing comprehensive information from the sentence pairs. This hidden state is then fed into a multi-class linear classifier that generates logits, representing the raw class predictions of the model. These logits are structured into a 2D tensor with three columns, corresponding to contradiction, neutral, and entailment, and rows that align the sentence pairs.
In the decoding stage, the logits tensor undergoes softmax normalization across each row, converting it into a probability tensor where each row sums up to one. Given the focus on contradiction and entailment classes, the neutral column is removed before applying the softmax function. This modification ensures that only the pertinent scores are factored into the probability calculations. For a detailed analysis of the entailment class, a 1D tensor representing these probabilities is extracted and reshaped into a 2D format that aligns with the number of sentence pairs and labels. The process concludes with the selection of the maximum value from each row, pinpointing the highest entailment score for each sentence pair.
The pipeline processes each batch sequentially until all batches are completed. A baseline entailment score of 0.9 is set as a threshold which will be verified in Step 5, signifying that sentences with at least 90% compatibility with the labels are considered relevant. These selected sentences are categorized as impact sentences. Based on their alignment with specific keywords, they are further classified into either structural or community damage categories. Additionally, these sentences are restored to their original positions within their respective documents. This repositioning is crucial for generating comprehensive summaries in the subsequent step.
BART-large MNLI brings two key advantages to this application. Firstly, it approaches the classification task through an inference-based method. Unlike traditional classification techniques that often rely on manual feature specification, the bidirectional encoder automatically captures features during training. This enables a more nuanced text analysis, allowing the model to discern underlying patterns and contexts beyond basic categorization. Secondly, the model has been fine-tuned on the extensive MNLI corpus which greatly boosts its inference capabilities, mitigating the need for fine-tuning the model with manually labeled wind-related impact sentences. This is particularly beneficial, as the model can be customized for different hazards, like seismic events, through modifying the keyword list.
Following the identification of impact sentences, the subsequent step involves generating summaries for structural and community damage. This is accomplished by fine-tuning the BART model (BART-large CNN) for sequence generation tasks, specifically on the CNN Daily Mail dataset (Hermann et al., 2015; See et al., 2017). This dataset encompasses over 300 k news articles with accompanying summaries created by CNN and Daily Mail journalists, making it ideal for extractive and abstractive summarization. The substantial model size and the diverse content of the CNN dataset enable it to generate content on topics it has not seen during training, such as wind disaster impact. Consequently, this reduces the necessity for further fine-tuning on tailored datasets for wind damage summaries, which are not readily available.
Figure 6 outlines the BART-large CNN implementation pipeline, encompassing the encoding, model processing, and decoding stages. Unlike BART-large MNLI, which processes sentence pairs in a 2D tensor, BART-large CNN handles continuous text chunks, streamlining the input process. Particularly, during the encoding stage, each text chunk is tokenized according to the BART-large CNN tokenizer scheme, and the resulting tokens are converted into unique IDs. These IDs form the numerical input enabling the bidirectional encoder to process and interpret the text as cohesive sequences.
In addition to the processed data, several parameters critically influence the summary generation process of the autoregressive decoder. The max_length and min_length parameters define the upper and lower limits of the length of the summary in tokens. The do_sample parameter dictates the method by which the autoregressive decoder determines the next word. When set to True for stochastic mode, the model samples the next word from a probability distribution based on the context from the bidirectional encoder and the previously generated words, enhancing diversity but reducing predictability. Conversely, when set to False for deterministic mode, the model uses the probability distribution to determine the most likely next word, ensuring more predictable and stable outputs. The num_beams parameter in beam search determines the number of potential paths the model keeps simultaneously during text generation. Selecting an appropriate num_beams value balances the trade-off between the quality of the generated text and the computational demands. The early_stopping parameter is utilized to terminate processing once all text sequences are sufficiently generated, optimizing both sentence length and computational efficiency.
These parameters collectively guide the autoregressive decoder, which constructs a summary represented by a tensor of new IDs. In the decoding stage, which mirrors the encoding process, the output—a sequence of IDs—is transformed back into text. Specifically, it involves converting each ID into its corresponding token, where each token corresponds to a lexical unit as specified by the tokenization scheme. These tokens are subsequently detokenized to compile a comprehensive summary.
The pipeline is designed to simultaneously process all the impact sentences for each category—structural and community—but is constrained by a 1024-token limit for tokenized ID inputs. To circumvent this, another approach is employed where impact sentences are grouped into paragraphs without exceeding the token limit, allowing the pipeline to be applied multiple times. These paragraphs, organized based on their original placement in the report, are summarized by the BART-large CNN model, and the resulting summaries are concatenated. Given the extensive length of this combined summary, a recursive process illustrated in Figure 7 is employed to distill the content further. This process involves grouping impact paragraph summaries into larger chunks, each with a maximum of 1,024 tokens. Overlapping tokens are managed using a sliding window function to maintain coherence. The BART-large CNN model is sequentially utilized for each chunk, and if the combined summary exceeds the token limit, the process is iteratively refined. The final output consists of two distinct summaries, one for structural damage and another for community damage from wind disasters.
This section assesses the performance of BART-large MNLI and BART-large CNN models. For the BART-large MNLI model, the focus is on the baseline entailment score set at 0.9, as discussed in Step 3. It involves comparing predicted impact sentences from Step 3 with those manually labeled in Step 2 to calculate four key metrics: accuracy, precision, recall, and F1 score. If these metrics yield positive results, this baseline entailment score can be applied to other wind damage reports. For the BART-large CNN model, summary quality is evaluated using the Recall-Oriented Understudy for Gisting Evaluation (ROUGE) score, which measures text overlap. An overview of these metrics is provided in the Supplementary Appendix.
The evaluation case is designed to assess the framework described in the previous section. As mentioned in Section 2, while several organizations offer relevant sources, StEER is chosen for its quick publication turnaround and concise report lengths. Accordingly, the StEER Hurricane Ian Preliminary Virtual Reconnaissance Report (PVRR), published on 16 November 2022, is selected for this assessment.
The report is initially converted from PDF to docx format to maintain the document structure. During this conversion, headings that do not contain damage information, such as preface, acknowledgment, and various appendices and administrative sections, are excluded. The document is then processed through a preprocessing script that extracts all remaining sentences, preparing them for subsequent analysis.
Building upon the criteria for defining impact sentences, this section focuses on preparing the dataset used to assess the performance of the BART-large MNLI model. It includes examples from the dataset to demonstrate how sentences indicating structural and community damage are labeled. It also presents examples of sentences that, although not actually reflecting impact, might easily be misinterpreted as such. To enhance clarity, impact-related keywords are highlighted in italics.
• Structural damage sentence example: Mobile/manufactured housing and RV parks were the most susceptible to the damage as they lacked the elevation and lateral force-resisting systems necessary to resist any significant storm surge-induced loads.
• Community impact sentence example: Tragically, preliminary numbers available at the time of this report confirm that Ian has caused over 100 fatalities in Florida, the highest direct loss of life in any hurricane landfalling in Florida since the 1935 Labor Day hurricane.
• Non-impact sentence examples:
1. While the Florida Residential Code provides regulations and guidance for the construction of one and two-family dwellings, the Florida Building Code addresses all other permanent buildings and structures.
2. Lee County, the scene of the heaviest impacts, was under a Tropical Storm Warning by 5 p.m. EDT on Sept. 26th, and a Hurricane Warning by 8 a.m. EDT on Sept. 27th.
3. The first evacuation order for Lee County (Zone A) was issued at 7 a.m. EDT on Sept. 27th, approximately 32 h prior to landfall, and 13 h prior to the most likely time of the arrival of Tropical Storm force winds (
The first sentence details structural components but is limited to code-specific information, lacking impact details. The second sentence, discussing tropical storm and hurricane warnings, i.e., hazard forecasting, similarly lacks details on infrastructure or community impact. The third sentence, mentioning the evacuation order, also does not directly address community impact, deviating from the emphasis of the research on direct effects.
The BART-large MNLI pipeline outlined in Figure 5 is followed to extract impact sentences. Due to the graphics processing unit (GPU) constraints, eight sentences are collectively processed in one batch. Each sentence is associated with 82 labels from Table 2, generating 82 distinct sentence-label pairs. These pairs are then tokenized to produce an encoded two-dimensional tensor, which serves as the input for the Bart-large MNLI model. The resulting logits tensor undergoes preprocessing and normalization via the softmax function, producing a probability tensor. From this tensor, the entailment tensor is extracted and reshaped into a 2D format, which allows for the selection of the highest entailment score for each sentence on a row-by-row basis, producing the final entailment scores.
Given the extensive number of sentences from the report, only the results from the impact examples in Step 2 are presented. Tables 3, 4 display the top five labels ranked by their highest entailment scores. The labels that best match the structural and community impact instances are mobile home damage and fatalities, respectively. It is important to note that sentences with a top entailment score below the assumed baseline of 0.9 are classified as non-impact.
The BART-large CNN model is used to generate summaries for structural and community impacts, following a recursive approach as detailed in Figure 7. The setup involves setting an overlap of 200 tokens and capping the maximum token count at 1,024 to create manageable text chunks. Key parameters for summary generation include setting num_beams to 4, which allows the model to simultaneously explore four potential paths. Additionally, early_stopping is enabled to prevent excessive processing, and do_sample is set to False, ensuring the model deterministically selects the most likely next word from the probability distribution for more consistent and stable outputs. These are the default settings of the model.
Initially, summaries are required to cover at least 80% of the length of the original impact paragraphs. These are then merged into larger text chunks, with subsequent summaries required to contain at least 40% of the total tokens from these chunks, ensuring the final outputs are concise yet substantial. The maximum length of the summaries corresponds to the number of tokens in the chunks prior to generating the summaries. The completed summaries for both structural and community impacts are provided below.
Structural impact summary: Unlike Hurricane Charley (2004), water more so than wind was the impetus behind the disaster that unfolded. Many buildings were completely washed away, and others were left to deal with significant scours and eroded foundations. The west coast of Florida and the Ft. Myers area are the most heavily impacted regions. Seawall collapses were reported along the Atlantic coastline of Florida at Daytona Beach Shores. A levee in Hidden River in Sarasota County, FL, was also breached, causing severe localized flooding (Clowe, 2022). According to the National Levee Database, the Hidden River levee is a 1.98-mile embankment levee along the Myakka River. The surge impacted regions with high population densities housed in both elevated and on-grade residential structures. These include mobile and manufactured home parks, along hundreds of miles of canals and coastal frontage in Cape Coral, Fort. Myers, and nearby barrier islands. It should be noted that 2 injuries and damage at the Florida Atlantic University Campus were reported as a result of the 130-mph estimated peak wind speed. The community has 700,000 solar panels, which continuously provided electricity for 2,000 households throughout Hurricane Ian’s passage. These solar panels showed good structural performance with no damage. Single-family housing performance was variable, with exposure hazard being the driver of structural failures. Mobile/manufactured housing (MH) and RV parks were the most susceptible to damage. Wind damage was primarily limited to the building envelopes in site-built homes. More severe damage was observed in some mobile/manufactured home parks in Fort Myers, FL. Several multi-family units in Delray Beach, FL suffered structural damage from wind, surge, or flooding on inland flooding on MH, or MH parks. The most common pattern of failure was the severe coastal erosion near the causeways and roads that led to the scouring or washout of pavements and causeways. The Sanibel Causeway consists of three bridges (A, B, C) and two islands (Causeway Island Park A, B) as shown in Fig. CS.5. Many structures built at ground level, primarily those exposed to wave action, were completely washed away. Power infrastructure was more critically damaged in southwest Florida near the landfall area, causing power outages for several days. Roof cover loss and flooding were frequently observed in churches, but no reports of structural damage have been found. Damage appears to be somewhat more frequent in Port Charlotte than in Cape Coral and Ft. Myers. Only isolated reports of wind damage to the building envelope have been reported in the inland communities. In contrast to past major hurricanes like Hurricane Katrina, there was almost no damage to bridge superstructures. Extreme coastal erosion near transportation infrastructure led to scours and different damage levels to roads and pavements. The Sanibel Island causeway was washed out. The iconic pier was extensively damaged, and floating docks were twisted at Fort Myers. Damage to port facilities was limited to piers and seawalls damaged by storm surge in Florida. A levee was in danger of failing in the Hidden River area, threatening more extensive flooding.
Community impact summary: The storm-related death toll from Hurricane Ian was 125 as of 10 November 2022. Death toll included 119 storm-related fatalities in Florida, five in North Carolina, and one in Virginia. Risk modelers estimated wind and coastal storm surge losses of $40-$74 billion. The majority of the deaths (57) were reported in Lee County, FL, and an estimated 60% were caused by drowning. The strong hurricane winds associated with Hurricane Ian caused widespread power outages in Florida. North Carolina had a power outage of more than 358,000 (Dean and Cataudella, 2022). The Federal Communications Commission (FCC) reported that cell service outages dropped from 65.0% to around 5.0%. The total number of wireline/cable users affected in Florida dropped from around 320,000 users to around 110,000. 642 patients evacuated from 6 healthcare facilities in Charlotte, Lee, Sarasota, Orange, and Volusia Counties. Hospitals in the impacted area suffered severe staffing shortages due to displaced workers. Telecommunications infrastructure primarily in southwest Florida was not functional following the storm due to loss of power, flooded generators, and in at least one case, the collapse of a cellular tower. The coastal roads to port facilities and the access bridges were severely damaged. Isolated damage to power infrastructure caused several days of outages, notably downed power lines. The damage to agriculture was much larger, particularly for the citrus industry, due to wind damage to crops and the flooded access bridges of Sanibel Island. As such, Hurricane Ian will likely be one of the costliest landfalling hurricanes of all time in the US, claiming over 100 lives. It took almost 9 days to restore power in Lee, Charlotte, and De Soto counties in Florida. As of 3 October 2022, Florida had more than 80% of consumers without power.
The results from Step 3 show the entailment score ranging from 0 to 1 for each sentence, with higher scores indicating a better fit with the corresponding label. A threshold of 0.9 is set for identifying impact sentences, signifying only sentences with entailment scores of 0.9 or above are classified as impact. The effectiveness of this threshold is assessed by analyzing confusion matrices for four different thresholds: 0.6, 0.7, 0.8, and 0.9, as shown in Figure 8.
Figure 8 shows an inverse relationship between the True Positive (TP) and True Negative (TN) versus False Negative (FN) and False Positive (FP) pairs as the thresholds increase. Higher thresholds result in fewer FP (non-impact sentences mistakenly classified as impact sentences) and more TN (correctly classified non-impact sentences). However, they also lead to an increase in FN (impact sentences mistakenly classified as non-impact) and a decrease in TP (correctly classified impact sentences). In this context, FN is prioritized over FP, as misclassifying non-impact sentences is preferable to overlooking actual impact sentences.
While the trends offer some insights, they alone are insufficient to determine the optimal threshold. A more detailed quantitative analysis is necessary, incorporating Precision, Recall, and F1 Score metrics derived from the confusion matrix components. Observing these parameters in Figure 9; Table 5 shows that Precision increases with higher thresholds, while Recall slightly decreases. This narrowing gap between Precision and Recall improves the F1 scores, resulting in its upward trend. Notably, despite the declining trend, all Recall values exceed 0.9, indicating the capability of the BART-large MNLI model to identify impact sentences. Therefore, a threshold of 0.9 is selected for its robust F1 Score and Recall performance.
The quality of generated summaries is typically assessed using the ROUGE score, which measures text overlap between a reference summary and the generated text. However, a significant challenge in this evaluation is the absence of human-generated reference summaries. Specifically, there is currently no available summary for the Hurricane Ian PVRR. While the authors could create their own summaries, this approach risks introducing bias due to their direct involvement in the impact sentence annotation process. Consequently, a summary evaluation using the ROUGE score has not been conducted.
The keyword list for wind disasters, shown in Table 2, is compiled from StEER and FEMA damage reports prior to 2023, including generalized keywords from the Hurricane Ian PVRR used in the evaluation case. To verify the capability of the BART-large MNLI model to extract impact sentences from documents not previously used in designing the keyword list, the Hurricane Otis PVRR report (Dang et al., 2024) is utilized. This approach ensures an unbiased verification of the model’s performance on new data. Although the performance of the BART-large CNN model is not the focus of this verification, summaries generated by this model are provided for reference. All previously established parameters, such as the keyword list, the entailment threshold of 0.9, and the configuration settings for both the BART-large MNLI and CNN models, are maintained.
Similar to the Hurricane Ian evaluation, Figure 10 shows that as the threshold increases: TP and FP decrease, while TN and FN increase. These adjustments result in an inverse relationship between Precision and Recall, which contributes to an increase in F1 scores across thresholds, as detailed in Figure 11; Table 6. The upward F1 score trend, alongside the Recall score remaining above 0.9, verifies the decision to maintain a baseline threshold of 0.9.
Structural impact summary: Acapulco experienced significant damage, especially to residential and high-rise buildings along the coast. High winds dominated the structural responses, inducing failures to multiple glazing, cladding, and roof surfaces. About 120 hospitals and 33 schools in the region were reported as damaged due to the hurricane event. It was estimated that approximately 250,000 families have been left homeless because of Hurricane Otis, according to Mexico’s President Andrés Manuel López Obrador (Rivera, 2023). The impact of blown-off roofs and walls led to widespread flooding inside houses. There was unprecedented damage to the building envelopes (e.g., glazing and cladding) of many structures due to wind loads, though the lateral force-resisting structural systems appeared to perform adequately. Some buildings experienced total exterior glazing loss over the full height of the building. Many buildings now display damaged roofs, loss of glaze, and failure of exterior wall cladding as a result. Rainwater caused significant geotechnical damage in elevated regions of Acapulco. The city’s ports were forced to close during the hurricane, disrupting not only local trade and transportation but also the city’s economic activities, which rely heavily on tourism. Early reports by CFE stated that the airport did not have electricity and it was not clear if the airport was closed or if it had been damaged by the hurricane. The Galerias Mall is one of the most severely damaged structures in the aftermath of Otis. Light-framed commercial properties along the coastline in Puerto Marqués Bay also showed evidence of damage from wind and breaking waves. The “stand-alone” tower appears to be intact, although the poles were severely damaged. Figure 6.2 presents a case where the edge of the road collapsed due to soil failure due to a slope failure. A clear eye and symmetric storm structure are visible, indicating Otis remained a powerful hurricane through landfall. Figure 4.4 shows three screenshots from a video of the damage caused to the Solar Ocean building. No widespread damage was reported, but video evidence showed strong winds blowing inside a hospital in Acapulco. Roof damage was observed in historical buildings. No significant damage has been reported in any of the hard coastal infrastructure (seawalls, port platforms, etc.). The failure of the exterior windows at the airport control tower resulted in water and wind damage to equipment and furniture in the tower. Water and sanitation systems were damaged, and the municipal water system was not operating due to power outages. If buildings are sufficiently damaged that they cannot receive power, the outage may never be restored. This scenario occurred in New Orleans, LA after Hurricane Katrina. Over 500,000 homes and businesses lost power—Water and sanitation systems were damaged. The Telemax power plant was restored. The re-establishment of high, medium, and low voltage poles continues for Avenida Costera Miguel Alaman, the City Center, Costa Azul, and Renacimiento. The following hospitals had their power restored by October 29: General, Military, Cancerology, and IMSS.
Community impact summary: Hurricane Otis left 45 people dead and 47 missing in Mexico. 80% of the hotels in Acapulco were damaged, and about 274,000 homes were destroyed in the region. The electric grid was severely affected, leaving 513,544 customers in the State of Guerrero without power. The government suspended electricity payments from November 2023 to February 2024 and called for the delivery of basic foods to the estimated 250,000 families impacted by the event. The financial implications of the hurricane’s devastation are substantial. According to Enki Research, the cost of Hurricane Otis could potentially reach as high as $15 billion. It is unknown how much effect this had on air traffic control after the storm. As of November 7, locals reported that about 50 percent of the roads were accessible but with military restrictions. Only the military, aid vehicles, Mexican media, and people showing residency in Acapulco are allowed to enter under military escort. On the evening of November 2, CFE posted on X (formerly known as Twitter) that the electricity had been restored to the airport. The port was inoperable, and the airport was closed. Significant disruptions to the transportation system occurred due to several rockslides above the Chilpancingo-Acapulco highway. At the road level, floodwaters caused mudslides that resulted in partial or full collapse of road lanes. The main highway between Acapulco and Mexico City could not be accessed, which hampered electric power restoration. CFE reports 513,524 outages out of 1.4 million users in the state, which is 36.6% of the population. On October 30, CFE reported that 65% of outages had been restored. It also noted that the Telemax power plant for communications was restored (CFE, 2023). By October 31, CFE reported the restoration of 75% of outages for Guerrero. On November 2 the electricity was restored to Acapulco. On Nov. 3, CFE reported that the power service for the Raney water well was restored.
This versatility of the framework is evaluated through its ability to adapt to various hazards, using the 2023 StEER Magnitude 7.8 Kahramanmaraş Türkiye Earthquake Sequence PVRR (Safiey et al., 2023). This adaptability is shown by modifying the keyword list, detailed in Table 7. In earthquake scenarios, keywords related to building components and structural terms are tailored to reflect the impact of seismic loads. Meanwhile, keywords for building types and community damage are kept consistent across various hazards due to their broad applicability. All other configuration settings for the BART-large MNLI and CNN models are also preserved.
The performance of the BART-large MNLI model in the Türkiye earthquake report (779 sentences) is comparable to its performance in the Hurricane Ian (254 sentences) and Hurricane Otis (276 sentences) reports. Figure 12 illustrates that increasing thresholds result in a decrease in TP and FP, but an increase in TN and FN. This creates a trade-off where Precision rises significantly, and Recall slightly declines, leading to an upward F1 score trend shown in Figure 13; Table 8. The combination of this trend and consistently high Recall above 0.9 supports maintaining a baseline threshold of 0.9 and highlights the applicability of the framework to different hazards. Besides, the lack of human-generated reference summaries limits the use of the ROUGE score to evaluate the performance of the BART-large CNN model in generating summaries.
Structural impact summary: The Dead Sea Fault (DSF) is a major transform fault that extends from the Red Sea to the southeast of Turkey. The mechanism and location of the earthquake are consistent with the DSF and the East Anatolian Fault (EAF). The earthquake was followed by many aftershocks, including several larger than magnitude 6 (one with Mw 6.6). As of 19 February 2023, the number of reported completely and partially collapsed buildings was 28,362. 75,717 buildings and 306,563 dwellings were either collapsed or severely damaged. In Syria, more than 22,000 buildings were affected by the earthquakes with 2,850 of them partially/completely collapsed or severely damaged. Almost two-thirds of residential buildings in Gaziantep city are masonry. One-third is Reinforced Concrete (RC). The lessons learned from the 1999 Kocaeli and Duzce Earthquakes led to the implementation of earthquake engineering principles in the whole country. The observations from the recent Mw 6.1 Duzce Earthquake on 23 November 2022, showed the importance of taking action to reduce the number of buildings with seismic deficiencies. The majority of the buildings in the DuzCE region affected by this earthquake were new and constructed following the lessons learned in the 1999 Kocaeli Earthquake. The low level of damage observed during the 2022 Duzce Earthquake demonstrated the effectiveness of code-specified seismic design requirements on the newer buildings. The seismic design/provisions for existing non-reinforced masonry wall systems are made available in Appendix 1 of the Syrian. Code for the Syrian Base Code (TBSC) was published in 1996. These provisions were considered optional to adopt until 2008 and were not considered to be required to adopt. In addition to their stiffness, upper masonry walls, both at the exterior and interior, add considerable mass to the building, resulting in larger inertial forces during ground shaking. Infill walls in the first event could have led to a more common collapse of these structures during the second event. It is possible that the presence of soft stories is accompanied by low ductility. Many buildings in Syria that were damaged during the war are believed to have been rebuilt by individuals with low-quality materials. The heaviest damage and the most collapses occurred in 4 cities in Syria (Aleppo, Latakia, Idlib, and Hama) and 40 towns and villages in Northern Syria. In concrete buildings that experienced severe damage or partial or total collapse, some common deficiencies can be observed in the collapse photographs. Concrete quality/strength appears very low by visual inspection of texture and color. Thin columns can be seen in many damaged buildings. Many buildings had lightly reinforced column sections, with no or little transverse reinforcement, and potentially inadequate lap splices located immediately above the slabs. The absence of drop (projected) beams below the ribbed slab and flat plate systems creates a slab-column connection situation (no beam-column joints) which is known to perform poorly under severe earthquake shaking unless well reinforced. Government facilities play a key role during extreme events like earthquakes. The large displacement due to the directivity effects during these two events likely contributed to the collapse during these directivity events. This practice results in high-mass buildings which induce large seismic forces during ground shaking. There were 11 base-isolated hospitals within 7.8 km from the epicenter of the Mw8 earthquake. Most seismically protected buildings remained functional, but some buildings collapsed. All hospitals have curved surface slider (CSS) pendulum and triple-pendulum systems. The number of seismic isolators used in these hospitals is reported to be high. The Turkish health minister reported that on February 8, 77 field hospitals were set up across 10 regions. Two state hospitals were completely destroyed, one of the three private hospitals collapsed, and the City Hospital was damaged. The affected schools are mainly located in the urban area of TarFGtous (99), Aleppo (71), Lattakia (50), Hama (27), and in the rural area of Idleb (1) World Health Organization (WHO) spokeswoman Harris mentioned impacts on healthcare in Türkiye and Syria as “huge long-term” issues. Road damage occurred throughout the impacted region due to the earthquake sequence. No damage was reported at the Akkuyu nuclear power plant (Figure 5.11). This nuclear plant is under construction. The Sultansuyu Dam in Malatya experienced significant longitudinal cracking at the crest due to a lateral spreading type failure. A lateral spreading-induced landslide was observed on the road between Adana and Gaziantep. The Banias refinery in Syria was partially damaged. The main damage to the refinery was to the concrete bases of equipment. The connectivity was not fully recovered as of 8 February 2023.
Community impact summary: As of 9 March 2023, the total death toll was reported to be 45,968 people in Türkiye and around 6,000 in Syria. More than 100,000 people were reported as injured. The number of fatalities estimated by PAGER for the Mw 7.8 event is shown in Figure 1.2. For the Mw 7.5 earthquake, economic losses are shown in Figures 1.3 and 1.4. Extreme Event Solutions at Verisk predicted that the economic losses and industry-insured losses due to the earthquake sequence will likely exceed $20 billion. In terms of economic loss, the ramifications could include the spoiling of food items requiring refrigeration. There is past evidence of the collapse of war-damaged residential buildings in Aleppo and consequential casualties (Reuters, 2023). There was one casualty in this historical neighborhood, which was known as a symbol of the region due to these old buildings. Hundreds of medical facilities were damaged, disrupting treatment capacities across large regions. It was delivering emergency healthcare assistance in a tent in the hospital garden (Müslüm, 2023). A relevant observation was the significant number of critical patients mobilized to the functional hospitals by helicopter as access was overwhelmed. On February 7, the Ministry of Health reported that injured people from Iskenderun were transferred to Mersin City Hospital in ambulances. Ninety-eight wounded patients were transferred on the day of the earthquake. About 11,780 citizen volunteers, who went to airports to go to the quake zone following an invitation from AFAD, were taken to Adana, Gaziantep, Adiyaman, and Urfa. The Capital Markets Board (SPK) announced that the Turkish Electronic Fund Trading Platform (TEFAS) transactions were suspended. Borsa İstanbul, the sole Turkish stock exchange, announced that all transactions would be halted for at least five business days. The entire network was destroyed during the war except for the two stations in Damascus and Tartus. There was an emergency stairway attached to the exterior of the building on each level of the Tarsus-Adana-Gaziantep Highway. Aftershocks have caused planes to be grounded at airports throughout the region. Roads were also closed due to inclement weather that occurred immediately after the earthquake sequence. Over 30 electrical substations were damaged causing power outages and blackouts reported in Antep, Hatay, and Kilis. Internet connectivity was lost in all cities impacted by the event in Türkiye. Deformation of the railway tracks was observed between Kahramanmaraş and Gaziantep. Phone lines were down in the southern provinces after the quake. Airports remained closed for a few days following the earthquake. Due to this, tanker loading operations were suspended for a day. But the leak was fixed, and the loading operations have resumed.
The performance of the BART-large MNLI model is demonstrated through three use cases, achieving a Recall exceeding 0.9 and an F1 score above 0.8. These metrics confirm the capability of the model to automatically extract impact sentences, tailored to domain-specific keywords. These positive results facilitate the development of an implementation framework, which is built upon the foundational framework shown in Figure 2 and detailed in Figure 14. This framework consists of two main phases: Execution and Evaluation. The Execution phase processes damage reports and disaster-related keywords to generate comprehensive summaries. It encompasses three primary steps: Data Preprocessing, Extracting Impact Sentences, and Generating Comprehensive Summaries. An optional Evaluation phase is proposed to assess the output quality, incorporating Data Labeling and Analyzing The Results steps. For a detailed overview of the code, guidance, and specific examples, refer to the Impact Data Mining repository on GitHub.
The implementation framework in Figure 14 is optimized for the Google Colab environment where each step is executed via a separate Python file. Table 9 outlines these files, indicating the recommended computational resources and their estimated processing times for Hurricane Ian PVRR. For example, the files that execute the machine learning models require an A100 GPU and 40 GB of CPU RAM to handle parallel processing efficiently. The processing times for these tasks are approximately 101 s and 85 s, respectively. Thus, excluding time for input file preparation, executing all computational code for a 40-page damage report generally takes around 4 min.
While the model is equipped with 407 million parameters and fine-tuned on the MNLI dataset to facilitate 0SHOT-TC—yielding positive results across three examples—the model may still misinterpret impact data. This issue stems from a lack of specific tuning for identifying structural and community damage information. To address this issue for the time being, incorporating more user-defined keywords could improve the performance of the model. However, for a viable solution, it is essential to develop a custom entailment corpus tailored to wind disasters, inspired by the format of the MNLI dataset, to enhance Natural Language Inference tasks related to disaster impacts.
In the summary generation task, the BART-large CNN model operates with default settings including a num_beams value of 4, a do_sample value set to False, and early_stopping option enabled as True. It also utilizes customized parameters such as a 200-token overlap threshold, a minimum length of 80% for initial summary output, 40% for subsequent summaries, and a maximum length equivalent to the number of tokens in the chunk being considered. Establishing well-defined evaluation criteria is essential to optimize these settings. One approach is to incorporate human-generated summaries into the evaluation, enabling the use of metrics such as ROUGE. Future studies should also explore the capabilities of the BART-large CNN model in processing lengthy documents, like FEMA documentation that ranges from 200 to 400 pages, possibly by training the model to self-adjust the number of summaries to be generated based on the volume of the content.
Once the framework demonstrates proficiency in handling text, the next step is to develop it into a multi-modal system that can generate summaries from both text and images. Upon completion, the model would be integrated into a data repository designed to accept keywords and images as inputs, facilitating the automatic extraction and summarization of related content from various reports. Specifically, the model will function as a platform where users can interact by submitting queries in a chat-based format, with the model providing answers and suggesting additional relevant information. Its performance will also be improved by incorporating user feedback, helping to identify both the framework’s strengths and areas for improvement. This approach aligns with the decadal vision of the NHERI network to incorporate AI and advanced analytics into cyberinfrastructure, improving data querying and disaster response strategies (Schneider and Kosters, 2024).
The fully developed model, once implemented as a platform, will serve a diverse range of users, including emergency managers, policymakers, insurance firms, and the natural hazard research community. However, its primary target audience is small to medium-sized communities, which often face systemic disadvantages in building resilience and managing preparedness, mitigation, and recovery efforts. These challenges arise primarily from two factors.
First, there is a lack of centralized, structured, and accessible disaster data. In the U.S., organizations like FEMA, NOAA, USGS, and state/local emergency management agencies release vast amounts of information following natural disasters. This information, which includes situation reports, damage assessments, recovery plans, guidance documents, humanitarian assistance plans, reconnaissance reports, and resilience planning documents, is presented in disparate contexts, formats, and content. Each year, the volume of these documents grows significantly, yet there are insufficient mechanisms to harness the insights contained within this extensive body of free-text data. Currently, manual reading and interpretation of these documents are required to extract valuable insights, which is both time-consuming and inefficient.
Second, many small to medium-sized communities lack the capacity to develop comprehensive disaster management plans, such as Hazard Mitigation Plans. These plans often span long timeframes and may be complicated by compounding hazards during ongoing recovery, leaving communities vulnerable to further damage. Even large, well-resourced states such as Louisiana have faced significant challenges in managing multiple, consecutive disasters. Between 2020 and 2021, Louisiana experienced several disasters, including Hurricanes Laura, Delta, Zeta, and Ida, as well as a severe storm in May. While the state was assessing damage from the earlier hurricanes to secure $600 million in Community Development Block Grant Disaster Recovery funds (OCD, 2023), it had to account for damages from subsequent disasters simultaneously. As a result, Louisiana had to revise its Action Plan, extending the recovery timeline from 1 January 2023, to 31 July 2028. If a state with substantial resources struggles with these complexities, the challenge is even more overwhelming for smaller communities with limited capacities.
The fully developed model addresses these challenges by providing users with access to structured information from various sources and enabling interaction through a chat-based format, where the model answers questions and suggests additional relevant information. For well-prepared communities, the model acts as a support tool for data querying, extraction, and summarization. For less-prepared communities, it provides essential background information to help them get started. The model will then assist all users in assessing their current vulnerabilities and guide them in developing key documents, such as Hazard Mitigation Plans, to mitigate the disaster impact. Ultimately, by gradually enhancing the capacity of its users, the model empowers small to medium-sized communities to better prepare for and recover from natural hazards.
This research develops a framework that leverages BART-large MNLI and BART-large CNN models to extract structural and community damage information from wind disaster reconnaissance reports using user-defined keywords. Fine-tuning this DL model on the MNLI and CNN Daily Mail datasets enables it to perform 0SHOT-TC and summary generation, thereby mitigating the need for specialized training datasets related to wind hazard impacts. Initially, the BART-large MNLI model identifies impact information using user-defined keywords. Subsequently, the BART-large CNN model generates summaries from these identified sentences, helping users grasp the overall content quickly. The performance of this framework was first evaluated with the Hurricane Ian PVRR report, achieving an F1 score of 0.855 and a recall of 0.945. The capability of the BART-large MNLI model to extract impact sentences was further verified on the Hurricane Otis PVRR report, which was not used to derive the keyword list, resulting in an F1 score of 0.831 and a recall of 0.936. The versatility of the framework is illustrated in an extended application to the Türkiye earthquakes PVRR report. Adjustments to the keyword list are the only changes needed, with an F1 score of 0.842 and a recall of 0.909. Overall, the framework performs well across use cases, consistently achieving F1 scores above 0.8 and recall rates above 0.9. These positive outcomes have led to the development of an implementation framework, with instructions and Python code available in the Impact Data Mining repository on GitHub.
Future efforts will focus on improving the accuracy of the BART-large MNLI model by developing a dedicated corpus for wind disaster impacts. Further studies will also establish evaluation criteria to assess the quality of the generated summaries and enhance the capability of the BART-large CNN model to process lengthy documents. The next step will involve creating a multi-modal system in a chat-based format that can interpret both text and images to improve querying capabilities. These advancements will support small to medium-sized communities in managing resilience across both pre- and post-hazard phases.
The original contributions presented in the study are included in the article/Supplementary Material, further inquiries can be directed to the corresponding authors.
HP: Formal Analysis, Methodology, Visualization, Writing–original draft, Writing–review and editing. MA: Funding acquisition, Methodology, Project administration, Supervision, Writing–review and editing.
The author(s) declare that no financial support was received for the research, authorship, and/or publication of this article.
The authors acknowledge the reconnaissance reports provided by StEER and their teams, which have significantly contributed to this research. Furthermore, the authors recognize the assistance of ChatGPT-4 in enhancing the clarity and readability of the paper.
The authors declare that the research was conducted in the absence of any commercial or financial relationships that could be construed as a potential conflict of interest.
All claims expressed in this article are solely those of the authors and do not necessarily represent those of their affiliated organizations, or those of the publisher, the editors and the reviewers. Any product that may be evaluated in this article, or claim that may be made by its manufacturer, is not guaranteed or endorsed by the publisher.
The Supplementary Material for this article can be found online at: https://www.frontiersin.org/articles/10.3389/fbuil.2024.1485388/full#supplementary-material
Alam, F., Sajjad, H., Imran, M., and Ofli, F. (2021). “Crisisbench: benchmarking crisis-related social media datasets for humanitarian information processing,” in Proceedings of the International AAAI Conference on Web and Social Media, 923–932. doi:10.1609/icwsm.v15i1.18115
Alrashdi, R., and O’Keefe, S. (2019). Deep learning and word embeddings for tweet classification for crisis response. arXiv Prepr. arXiv:1903.11024. doi:10.48550/arXiv.1903.11024
CFE (2023). CFE personnel persevere and restore electricity to 65% of those affected by Hurricane Otis in Guerrerog. https://www.cfe.mx/Pages/default.aspx.
Clowe, S. (2022). Sarasota county community affected by levee break cleaning up afterHurricane Ian. https://www.wtsp.com/article/news/local/sarasotacounty/sarasota-county-community-levee-break-cleaning-up-hurricane-ian/67-8c2a4ac4-94ee-4811-81cb-463509963953.
Dang, H., Alawode, K., Lahna, T., Roueche, D., Kalliontzis, D., Mostafa, K., et al. (2024). Steer: hurricane otis preliminary virtual reconnaissance report (pvrr). doi:10.17603/DS2-MB7Z-XW74
Dean, K., and Cataudella, K. (2022). 358,000+ without power in NC as Post-Tropical Cyclone Ian moves inland. https://www.newsobserver.com/news/weather/hurricane/article266608621.html.
Devlin, J., Chang, M.-W., Lee, K., and Toutanova, K. (2019). “BERT: pre-training of deep bidirectional transformers for language understanding,” in Proceedings of the 2019 Conference of the North American Chapter of the Association for Computational Linguistics, (Volume 1: Long and Short Papers) (Minneapolis, Minnesota: Association for Computational Linguistics), 4171–4186. doi:10.18653/v1/N19-1423
Hendrycks, D., and Gimpel, K. (2016). Gaussian error linear units (gelus). arXiv Prepr. arXiv:1606.08415. doi:10.48550/arXiv.1606.08415
Hermann, K. M., Kocisky, T., Grefenstette, E., Espeholt, L., Kay, W., Suleyman, M., et al. (2015). “Teaching machines to read and comprehend,” in Advances in neural information processing systems, 1693–1701.
Ikonomakis, M., Kotsiantis, S., and Tampakas, V. (2005). Text classification using machine learning techniques. WSEAS Trans. Comput. 4, 966–974.
Imran, M., Elbassuoni, S., Castillo, C., Diaz, F., and Meier, P. (2013). “Extracting information nuggets from disaster-related messages in social media,” in Proceedings of the 10th International Conference on Information Systems for Crisis Response and Management (ISCRAM 2013), 791–800.
Imran, M., Mitra, P., and Castillo, C. (2016). “Twitter as a lifeline: human-annotated twitter corpora for nlp of crisis-related messages,” in Proceedings of the Tenth International Conference on Language Resources and Evaluation (LREC’16), 1638–1643.
Kaisler, S., Armour, F., Espinosa, J. A., and Money, W. (2013). “Big data: issues and challenges moving forward,” in 2013 46th Hawaii International Conference on System Sciences (IEEE), 995–1004.
Kersten, J., Kruspe, A., Wiegmann, M., and Klan, F. (2019). “Robust filtering of crisis-related tweets,” in Proceedings of the 16th International Conference on Information Systems for Crisis Response and Management (ISCRAM 2019) (Valencia, Spanien: ISCRAM Association), 19–22.
Kijewski-Correa, T., Roueche, D. B., Mosalam, K. M., Prevatt, D. O., and Robertson, I. (2021). Steer: a community-centered approach to assessing the performance of the built environment after natural hazard events. Front. Built Environ. 7, 636197. doi:10.3389/fbuil.2021.636197
Koliou, M., van de Lindt, J. W., McAllister, T. P., Ellingwood, B. R., Dillard, M., and Cutler, H. (2020). State of the research in community resilience: progress and challenges. Sustain. Resilient Infrastructure 5, 131–151. doi:10.1080/23789689.2017.1418547
Laney, D. (2001). 3D data management: controlling data volume, velocity, and variety. Tech. Rep. META Group.
Lewis, M., Liu, Y., Goyal, N., Ghazvininejad, M., Mohamed, A., Levy, O., et al. (2020). “Bart: denoising sequence-to-sequence pre-training for natural language generation, translation, and comprehension,” in Proceedings of the 58th annual meeting of the association for computational linguistics (ACL 2020), 1234–1245. doi:10.18653/v1/2020.acl-main.703
Liu, J., Singhal, T., Blessing, L. T., Wood, K. L., and Lim, K. H. (2021). “Crisisbert: a robust transformer for crisis classification and contextual crisis embedding,” in Proceedings of the 32nd ACM Conference on Hypertext and Social Media, 133–141. doi:10.1145/3465336.3475117
Müslüm, E. (2023). AKP deputy visiting the earthquake zone: state hospital is in an unusable condition. https://www.sozcu.com.tr/deprem-bolgesine-giden-akpli-vekil-devlet-hastanesi-girilemez-halde-wp7583416 (Accessed November 27, 2024).
Nguyen, D., Al Mannai, K. A., Joty, S., Sajjad, H., Imran, M., and Mitra, P. (2017). “Robust classification of crisis-related data on social networks using convolutional neural networks,” in Proceedings of the International AAAI Conference on Web and Social Media, 632–635.
OCD (2023). Action plan amendment: response to the 2020-21 storms (apa 3). Available at: https://cdn2.assets-servd.host/utopian-bustard/production/2020-21-Storms-APA-3-8.29.23.pdf?dm=1694459353.
OpenAI (2023b). Openai red teaming network. Available at: https://openai.com/index/red-teaming-network/.
OpenAI (2024). Hello gpt-4o. Available at: https://openai.com/index/hello-gpt-4o/.
Patgiri, R., and Ahmed, A. (2016). “Big data: the v’s of the game changer paradigm,” in 2016 IEEE 18th International Conference on High-Performance Computing and Communications; IEEE 14th International Conference on Smart City; IEEE 2nd International Conference on Data Science and Systems (HPCC/SmartCity/DSS), 17–24. doi:10.1109/hpcc-smartcity-dss.2016.0014
Peek, L., Tobin, J., Adams, R. M., Wu, H., and Mathews, M. C. (2020). A framework for convergence research in the hazards and disaster field: the natural hazards engineering research infrastructure converge facility. Front. Built Environ. 6, 110. doi:10.3389/fbuil.2020.00110
Pinelli, J.-P., Esteva, M., Rathje, E. M., Roueche, D., Brandenberg, S. J., Mosqueda, G., et al. (2020). Disaster risk management through the designsafe cyberinfrastructure. Int. J. Disaster Risk Sci. 11, 719–734. doi:10.1007/s13753-020-00320-8
Radford, A., Narasimhan, K., Salimans, T., and Sutskever, I. (2018). Improving language understanding by generative pre-training.
Rathje, E. M., Dawson, C., Padgett, J. E., Pinelli, J.-P., Stanzione, D., Adair, A., et al. (2017). Designsafe: new cyberinfrastructure for natural hazards engineering. Nat. Hazards Rev. 18, 06017001. doi:10.1061/(asce)nh.1527-6996.0000246
Reuters (2023). Thirteen killed after building collapses in Syria’s Aleppo, state media report. https://www.reuters.com/world/middle-east/thirteen-killed-after-building-collapses-syrias-aleppo-state-media-2023-01-22/.
Riezebos, A. (2019). Finding impact data in humanitarian documents. M.S. thesis, Leiden University. M.S. thesis.
Rivera, S. (2023). Family displaced by Hurricane Otis is first to move into new housing. https://www.yahoo.com/news/family-displaced-hurricane-otis-first-202314211.html.
Safiey, A., Roueche, D., Huallpa, L. A. M., Hassan, W., Reis, C., Sorosh, S., et al. (2023). Steer: 2023 mw 7.8 kahramanmaras, Turkiye earthquake sequence preliminary virtual reconnaissance report (pvrr) media repository. doi:10.17603/DS2-MM04-XQ43
Schneider, N., and Kosters, J. (2024). U.S. National science foundation natural hazards engineering research infrastructure (NHERI) decadal visioning study 2026-2035. Tech. Rep., NHERI. doi:10.17603/DS2-AFZA-M544
See, A., Liu, P. J., and Manning, C. D. (2017). “Get to the point: summarization with pointer-generator networks,” in Proceedings of the 55th annual meeting of the association for computational linguistics (volume 1: long papers) (Vancouver, Canada: Association for Computational Linguistics), 1073–1083. doi:10.18653/v1/P17-1099
Vaswani, A., Shazeer, N., Parmar, N., Uszkoreit, J., Jones, L., Gomez, A. N., et al. (2017). “Attention is all you need,” in Advances in neural information processing systems, 5998–6008.
Verma, S., Vieweg, S., Corvey, W., Palen, L., Martin, J., Palmer, M., et al. (2011). “Natural language processing to the rescue? extracting “situational awareness” tweets during mass emergency,” in Proceedings of the Fifth International Conference on Weblogs and Social Media (ICWSM 2011), 385–392. doi:10.1609/icwsm.v5i1.14119
Williams, A., Nangia, N., and Bowman, S. (2018). “A broad-coverage challenge corpus for sentence understanding through inference,” in Proceedings of the 2018 Conference of the North American Chapter of the Association for Computational Linguistics: Human Language Technologies, (Volume 1: Long Papers) (New Orleans, Louisiana: Association for Computational Linguistics), 1112–1122. doi:10.18653/v1/N18-1101
Wolf, T., Debut, L., Sanh, V., Chaumond, J., Delangue, C., Moi, A., et al. (2020). “Transformers: state-of-the-art natural language processing,” in Proceedings of the 2020 Conference on Empirical Methods in Natural Language Processing: System Demonstrations (Association for Computational Linguistics), 38–45. doi:10.18653/v1/2020.emnlp-demos.6
Keywords: wind disaster and resilience, structural damage, community damage, zero-shot text classification, text mining
Citation: Pham H and Arul M (2024) Automated extraction and summarization of wind disaster data using deep learning models, with extended applications to seismic events. Front. Built Environ. 10:1485388. doi: 10.3389/fbuil.2024.1485388
Received: 23 August 2024; Accepted: 06 November 2024;
Published: 12 December 2024.
Edited by:
Cheryl Ann Blain, Naval Research Laboratory, United StatesReviewed by:
Kurtis Robert Gurley, University of Florida, United StatesCopyright © 2024 Pham and Arul. This is an open-access article distributed under the terms of the Creative Commons Attribution License (CC BY). The use, distribution or reproduction in other forums is permitted, provided the original author(s) and the copyright owner(s) are credited and that the original publication in this journal is cited, in accordance with accepted academic practice. No use, distribution or reproduction is permitted which does not comply with these terms.
*Correspondence: Huy Pham, cGhhbWFuaHV5QHZ0LmVkdQ==
Disclaimer: All claims expressed in this article are solely those of the authors and do not necessarily represent those of their affiliated organizations, or those of the publisher, the editors and the reviewers. Any product that may be evaluated in this article or claim that may be made by its manufacturer is not guaranteed or endorsed by the publisher.
Research integrity at Frontiers
Learn more about the work of our research integrity team to safeguard the quality of each article we publish.