- Department of Civil Engineering, Zhengzhou University, Zhengzhou, Henan, China
Introduction: This study explores the impact of Building Information Modelling (BIM) staff on construction project performance, with a focus on the roles of the Unified Theory of Acceptance and Use of Technology (UTAUT) and Task-Technology Fit (TTF). The research aims to understand how BIM personnel attributes influence project outcomes and how these effects are mediated by TTF and moderated by UTAUT.
Methods: Partial Least Squares Structural Equation Modelling (PLS-SEM) was employed to analyze the data, allowing for the examination of complex interactions between BIM staff attributes, project characteristics, and outcomes. This method is particularly suited for handling smaller sample sizes and non-normal data distributions.
Results: The analysis revealed that BIM staff attributes—such as team size, expertise, training, and experience—significantly influence construction project performance. Key performance areas affected include design efficiency, error reduction, and adherence to schedules and budgets. TTF emerged as a critical mediator, enhancing performance metrics like stakeholder satisfaction and cost management. UTAUT further moderated the relationship between BIM staff, TTF, and project performance, highlighting the importance of technology acceptance and use within BIM contexts.
Discussion: The findings emphasize the need for organizations to strategically allocate BIM staff and invest in their professional development to optimize project outcomes. Recommendations include fostering supportive organizational structures and promoting a culture of collaboration and innovation to enhance project resilience and performance, particularly in the face of disruptive events. Future research should expand to diverse project types and scales, investigate emerging technologies, and consider cultural factors through cross-cultural studies. Longitudinal studies and cost-benefit analyses of BIM training and technology adoption are also suggested to provide deeper insights and inform strategic decision-making.
1 Introduction
The construction industry is undergoing a transformative phase with the emergence of Construction 4.0, where Building Information Modelling (BIM) stands out as a crucial component (Adepoju, 2022; Azhar, 2011). BIM offers a plethora of benefits including sustainability, cost predictability, time efficiency, and site safety by facilitating digital documentation of building performance throughout its lifecycle (UNECE. United Nations, 2021; Dung et al., 2012). Despite its recognized advantages, global adoption of BIM faces challenges, with varying levels of implementation across different countries (Takyi-Annan and Zhang, 2023; Olanrewaju et al., 2022; Olanrewaju et al., 2020; Khodeir and Nessim, 2018).
BIM staff, including roles such as BIM Managers, Coordinators, Modelers, Technicians, and Engineers, are responsible for planning, designing, and managing building projects using digital models, requiring technical proficiency, attention to detail, communication, problem-solving, project management, collaboration, and adaptability. Their expertise and training ensure high-quality outputs, while the team size and composition are adjusted to meet project demands efficiently (Aladağ et al., 2023). However, existing research has often overlooked the specific attributes and effectiveness of BIM staff, leading to gaps in understanding the dynamics influencing BIM adoption and project performance. This omission leaves a significant gap in the literature, particularly concerning how BIM staff, with focus on BIM staff attributes, such as training, experience, and expertise, impact project outcomes.
Studies primarily focusing on return on investment (ROI) (Giel et al., 2019; Huh et al., 2023) have overlooked crucial aspects related to BIM staff attributes and project characteristics, highlighting the need for a deeper exploration (Olofsson Hallén et al., 2023). Addressing this research gap requires evaluating the influence of BIM personnel on project outcomes, considering attributes like staff size, training, experience, and expertise. Understanding the nuanced relationship between BIM staff attributes and project outcomes can inform industry practices and optimize BIM implementation strategies.
Kenya, characterized by its low adoption of BIM, (Daniel and University of Nairobi, 2016), provides a valuable context for this research despite the lower usage rates. The low adoption does not imply a lack of BIM practitioners; (Ariono et al., 2022; Adekunle et al., 2021; Saka et al., 2020); in fact, there is a sufficient number of professionals whose experiences and challenges offer rich insights into the barriers to BIM adoption. Recent studies highlight that even in regions with limited BIM usage, existing practitioners can provide critical information on the obstacles and opportunities for broader adoption. For example, Alasmari et al. (2022) and Cao et al. (2022) highlight how practitioners can significantly contribute to understanding BIM’s optimization and integration, even in less common contexts (Cao et al., 2022; Alasmari et al., 2022).
By examining these specific barriers—such as limited user awareness, inefficiency, perceptions, and inadequate training—we can develop targeted strategies to enhance BIM adoption and project performance (El Hajj et al., 2023; Kekana et al., 2015; Olugboyega and Windapo, 2023; Mosse et al., 2020; Mtya and Windapo, 2019). By addressing these specific barriers, the research can provide targeted insights and strategies to enhance BIM adoption and project performance. This focus can guide project managers on effective BIM staffing and training needs, and motivate stakeholders to formulate policies that ultimately enhance BIM uptake not only in Kenya but also in other similar contexts globally. Research in Kenya, therefore, serves as a critical case study for understanding and overcoming the obstacles to BIM adoption in developing countries, offering broader applicability and impact (Olugboyega and Windapo, 2023; Mtya and Windapo, 2019).
The construction industry’s diversity in project characteristics such as size, complexity, and delivery method significantly influence the utilization and effectiveness of BIM staff. Examining how these factors interact with BIM staff roles, such as BIM managers, modelers, and engineers, is crucial for tailoring implementation strategies to specific project requirements (Hampson and Tatum, 1997). Empirical evidence is vital in justifying the role of BIM staff and assessing the effectiveness of BIM implementation practices (Raza et al., 2023). By systematically measuring key performance indicators, researchers can quantitatively assess the impact of BIM staff on project outcomes, enabling evidence-based decision-making and targeted interventions.
Despite the importance of BIM staffing, its impact on project productivity and performance has rarely been studied, indicating a significant gap in the literature (Ham and Yuh, 2023; Neelamkavil and Ahamed, 2012). Key issues include limited awareness and expertise among professionals, resistance to change, and inadequate stakeholder support. (Maina and University of Nairobi, 2018; Mwero et al., 2019). Existing literature, primarily from regions like Hong Kong (Chan, 2014), identifies these challenges but often fails to provide effective strategies for overcoming them (Chan et al., 2019). The real issue lies in the lack of examination of BIM staff attributes and their interaction with BIM tools to perform tasks (Semaan et al., 2021). Using the task-technology fit (TTF) framework can assess whether the technology suits the tasks considering user attributes, while the Unified Theory of Acceptance and Use of Technology (UTAUT) can enhance understanding of these attributes. Integrating TTF and UTAUT in empirical studies can provide insights into optimizing BIM staffing and technology use, thereby improving project outcomes and filling a critical gap in the current research. Additionally, optimizing BIM adoption in regions like Kenya necessitates tailored strategies and recommendations addressing specific barriers and contextual challenges (Oyuga et al., 2021). Ultimately, improving the expertise and mindset of BIM staff is essential for overcoming adoption barriers and fostering a robust implementation of BIM practices within the construction industry.
1.1 Theoretical foundations of the research
This study examines the impact of BIM (Building Information Modelling) staff on construction project performance using the Unified Theory of Acceptance and Use of Technology (UTAUT) and Technology Task Fit (TTF) frameworks. In this study, we utilize the Unified Theory of Acceptance and Use of Technology (UTAUT) framework to explore how BIM staff’s perceptions and attitudes—specifically focusing on performance expectancy, effort expectancy, social influence, and facilitating conditions—influence the adoption and utilization of BIM technology, thereby impacting project outcomes (Venkatesh et al., 2003). TTF emphasizes the alignment between technology capabilities and task requirements, suggesting that successful BIM implementation depends on how well the technology meets specific project needs, considering factors like project size, complexity, and delivery method (Goodhue and Thompson, 1995).
Addressing the technology utilization-acceptance gap requires understanding both technological and human factors influencing adoption. Various models like the Technology Acceptance Model (TAM), (Davis et al., 1989; Venkatesh and Davis, 2000), Innovation Diffusion Theory (IDT), (Rogers, 1995), and the Unified Theory of Acceptance and Use of Technology (UTAUT) (Venkatesh et al., 2003; Venkatesh et al., 2012) have been instrumental in explaining technology acceptance. TAM emphasizes perceived usefulness and ease of use, (Ramanayaka and Venkatachalam, 2015), while TTF focuses on the alignment between technology characteristics and user tasks (Goodhue and Thompson, 1995). UTAUT extends these models by considering social and organizational influences.
Combining UTAUT with TTF provides a robust framework for understanding BIM adoption and its impact on project performance. This integrated model includes performance expectancy, effort expectancy, social influence, and facilitating conditions from UTAUT2, while TTF focuses on the alignment between BIM technology and tasks. It considers unique project characteristics in the construction industry and leverages empirical validation from both models. Factors such as error reduction, stakeholder satisfaction, and cost management are included to offer a nuanced examination of how individual and task characteristics interact in technology adoption.
1.1.1 Unified Theory of Acceptance and Use of Technology
The Unified Theory of Acceptance and Use of Technology (UTAUT), Figure 1 developed by Venkatesh et al., integrates several prior models to explain and predict individuals’ acceptance and use of technology (Venkatesh et al., 2003). The framework identifies four primary constructs—performance expectancy, effort expectancy, social influence, and facilitating conditions—that directly influence behavioural intention and technology use.
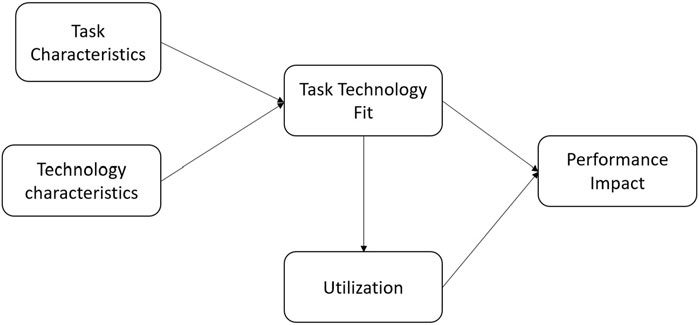
Figure 1. UTAUT (Venkatesh et al., 2003).
UTAUT has been used to study the adoption of electronic health records among healthcare professionals, demonstrating its applicability in predicting user acceptance of health information systems (Bawack and Kala Kamdjoug, 2018; Dash and Sahoo, 2022; Rouidi et al., 2022). In education, UTAUT has helped analyze factors influencing the acceptance of e-learning systems among students and educators (Gunasinghe et al., 2020; Abbad, 2021). The theory has also been applied in the context of information systems to examine user acceptance of enterprise resource planning (ERP) systems (Uddin et al., 2019). In construction, studies have utilized UTAUT to investigate the adoption of BIM and other technologies by construction professionals (Howard et al., 2017).
Specifically, UTAUT helps understand how these constructs affect the adoption of new technologies like BIM in construction. Modifying the UTAUT model for digital transformation in the construction industry demonstrates its adaptability and relevance. Recent studies have shown UTAUT’s flexibility, such as analyzing factors influencing users’ intention to use Open AI’s ChatGPT, illustrating the model’s applicability to emerging technologies (Menon and Shilpa, 2023).
In BIM staff research, UTAUT provides insights into how professionals perceive and adopt new tools, considering factors like perceived usefulness, ease of integration, social influences, and organizational support. Moderating factors like age, gender, experience, and voluntariness help tailor interventions to specific user groups, enhancing technology implementation (Venkatesh et al., 2003; Zhou et al., 2010). UTAUT offers a comprehensive analysis of technology adoption’s impact on project performance, guiding strategies to improve outcomes like error reduction, cost management, and stakeholder satisfaction.
UTAUT research predominantly focuses on user perceptions of technology’s usefulness, compatibility, and relative advantage, but it often overlooks the critical aspect of technology fit in adoption, as well as the conditions and user-perceived factors that explain the utilization of technology (Venkatesh et al., 2012; Bagozzi, 2007). To address these gaps, we integrated the TTF framework with UTAUT to provide a more comprehensive understanding of how well BIM technology aligns with specific project tasks and user needs, thereby enhancing its adoption and effectiveness.
1.1.2 Task-technology fit (TTF) theory
The Task-Technology Fit (TTF) theory in Figure 2, as described by Goodhue and Thompson (1995), emphasizes the alignment between task characteristics, technology features, and user capabilities to enhance individual performance (Goodhue and Thompson, 1995). This theory posits that technology utilization and individual performance improve when there is a good fit between the tasks and the technology used.
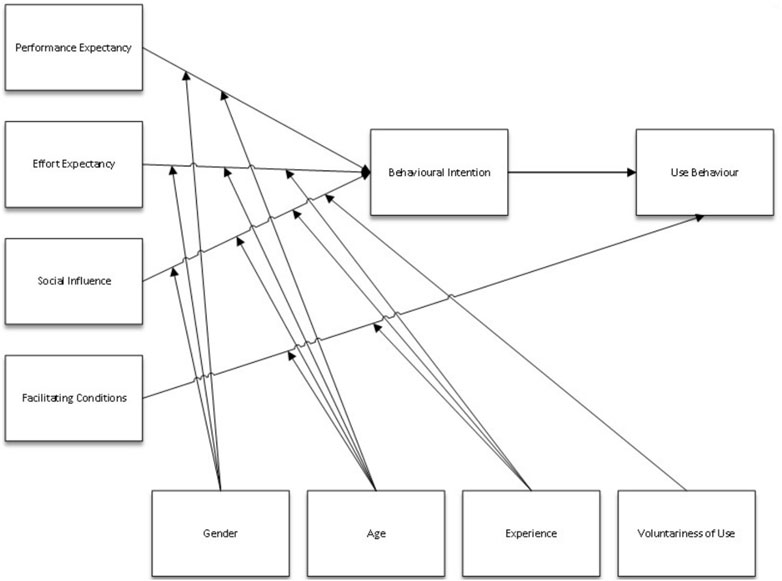
Figure 2. TTF (Goodhue and Thompson, 1995).
In healthcare, it predicts user satisfaction with clinical decision support systems, to understand IoT-based healthcare acceptance, evaluate big data analytics in mobile cloud systems, assess RFID adoption, and explore factors influencing wearable device adoption (Misra et al., 2023; Gu and Black, 2021; Wang and Lin, 2019). In education, it assesses e-learning acceptance (Alyoussef, 2021a; Alyoussef, 2021b). In construction, TTF evaluates the adoption of high off-site construction level technologies. (Cai et al., 2023).
Specifically, TTF helps in construction by evaluating how well BIM tools fit specific tasks, impacting productivity and performance. Recent studies have integrated TTF with UTAUT to understand technology adoption and performance, such as in mobile banking (Abbas et al., 2018).
Recent studies have further demonstrated the adaptability and relevance of TTF by integrating it with UTAUT to understand technology adoption and performance in various settings. An example is the study on mobile banking adoption, showing how task fit and user acceptance jointly influence technology use (Franque et al., 2023).
Researchers can use TTF to assess how BIM tools support tasks like construction planning and facility management. This alignment is crucial for optimizing BIM use, leading to improved efficiency, reduced errors, and enhanced collaboration. Integrating TTF with BIM research provides a comprehensive analysis of how technology adoption affects project performance, offering insights for successful BIM implementation and better project outcomes.
1.1.3 Theory extensions
1.1.3.1 Task technology fit and Unified Theory of Acceptance and Use of Technology
The integration of TTF with UTAUT in the TTF-UTAUT model in Figure 3 represents a significant advancement in understanding technology adoption and utilization (Venkatesh et al., 2012; Marikyan and Papagiannidis, 2023). This combined model leverages key constructs from both TTF and UTAUT, addressing gaps in prior research by emphasizing the importance of task-technology fit alongside performance expectancy, effort expectancy, social influence, and facilitating conditions. By focusing on the alignment between task requirements and technology functionality, the TTF-UTAUT model enhances the predictive power of technology adoption behavior, suggesting that when technology aligns well with tasks, users’ performance expectations and ease of use perceptions improve.
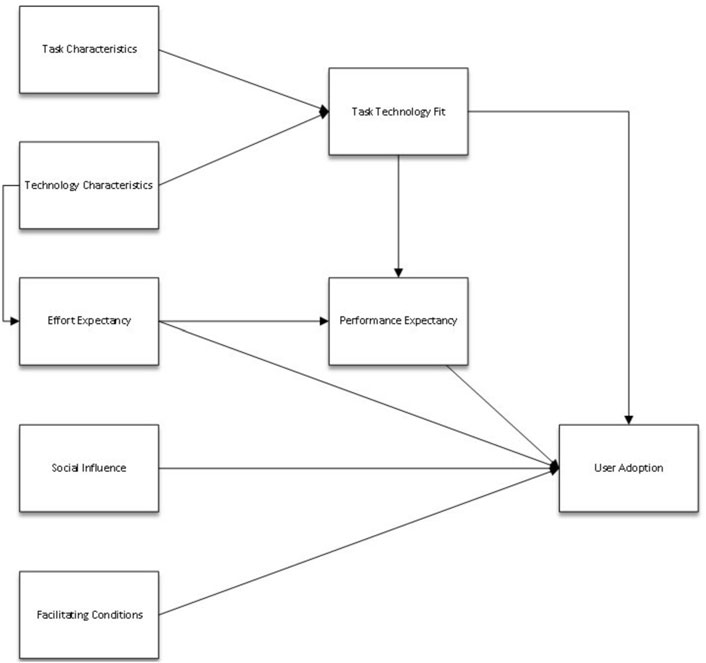
Figure 3. Integrated TTF and UTAUT (Zhou et al., 2010).
The integration of TTF and UTAUT frameworks provides a robust foundation for understanding technology adoption across diverse sectors. In mobile banking, TTF assesses how well technologies align with user tasks, complemented by UTAUT which explores psychological and social factors influencing adoption. This approach has been pivotal in studying mobile banking adoption, as well as illuminating factors shaping consumer intentions in emerging markets. Moreover, TTF-UTAUT models have been applied to assess social media use in education, organizational digital transformations, public acceptance of autonomous buses, and students continued use of MOOCs, demonstrating their versatility and effectiveness across different technological contexts (Zhou et al., 2010; Abbas et al., 2018; Dash et al., 2023; Al-Rahmi et al., 2022; Ojiaku et al., 2024; Kim et al., 2022; Oliveira et al., 2014). In construction, the combined TTF and UTAUT frameworks assess BIM adoption by evaluating how well BIM functionalities align with construction tasks and user acceptance, impacting project outcomes. Efforts to enhance BIM acceptance in facilities management integrate TTF and UTAUT, demonstrating their effectiveness in optimizing BIM utilization across construction project phases (Hilal and Maqsood, 2017; Hilal et al., 2021).
The TTF-UTAUT model suggests that technology adoption hinges on the perceived fit between tasks and technology. According to TTF, this fit is determined by the characteristics of both the technology and the tasks. The theory posits that even if individuals have a positive attitude towards a technology, they are unlikely to adopt it if it does not align with their task requirements (Goodhue and Thompson, 1995).
In line with UTAUT, technology adoption is driven by factors such as effort expectancy, performance expectancy, facilitating conditions, and social influence (Venkatesh et al., 2003). Performance expectancy is particularly influenced by perceived task-technology fit, (Zhou et al., 2010), as confirmed by studies showing that TTF impacts perceived usefulness (Dishaw and Strong, 1999). Both performance expectancy and perceived usefulness gauge the expected performance improvements from technology use. Thus, effective technology functionality that meets task needs enhances performance expectations (Zhou et al., 2010).
Furthermore, effort expectancy is shaped by technology characteristics, with more functional technologies requiring less effort to use (Zhou et al., 2010).
1.2 BIM adoption
Building Information Modelling (BIM) is transforming the architectural, engineering, and construction (AEC) sectors by enabling virtual prototyping of building designs before construction begins, (Dung et al., 2012), integrating scheduling, cost data, sustainability analysis, and facility management into the modelling process. This enhances collaboration, project performance, and lifecycle management (Aouad and L. A. Routledge, 2006; Ganah, 2015).
Globally, BIM adoption has gained traction through initiatives like the United Kingdom government’s BIM Level 2 mandate and government-led efforts in Singapore, South Korea, and Japan. However, adoption in developing countries faces challenges like limited access to technology and resource constraints (Bernstein et al., 2014) (Cabinet Office H. Cabinet Office, 2011) (Technology, 2020). However, adoption in developing countries faces challenges such as limited access to technology and resource constraints, although recognition of BIM’s benefits is steadily increasing (Succar, 2015).
In Kenya, BIM adoption is at 56.6%, with significant barriers including limited understanding of BIM capabilities, insufficient training, resistance to change, and regulatory hurdles (Mosse et al., 2020). This study focuses on Kenya to address these specific barriers, providing insights and strategies to enhance BIM adoption and project performance. By identifying effective BIM staffing and training needs, the research aims to guide project managers and motivate stakeholders to formulate policies that will ultimately enhance BIM uptake in Kenya and similar contexts globally.
Strategies for BIM implementation include bottom-up approaches that foster organizational alignment and a culture of innovation, (Xue et al., 2023), training and education programs to equip staff with necessary skills and knowledge, (Eadie et al., 2013; Girginkaya Akdag and Maqsood, 2020; Khosrowshahi and Arayici, 2012), collaborative workflows to enhance coordination and communication, (Rashidian et al., 2022; Rashidian et al., 2023), adherence to standards and guidelines for consistency and interoperability, (Barbosa et al., 2016; Chegu Badrinath and Hsieh, 2019), and the integration of technology acceptance theories like TAM and UTAUT to understand users’ perceptions and behaviours regarding BIM adoption (Hill et al., 1977) (Venkatesh and Davis, 2000) (Davis et al., 1989) (Hilal et al., 2019). These strategies highlight the critical role of BIM staff in facilitating coordination, communication, and data exchange among project stakeholders, emphasizing the collaborative nature of BIM implementation and its impact on project performance.
1.3 BIM staff and attributes
BIM staff, including roles such as BIM managers, coordinators, modelers, and technicians, are crucial for the successful implementation and utilization of BIM technology in construction projects. These professionals oversee BIM processes, create and maintain models, facilitate stakeholder collaboration, and ensure compliance with project standards (Oraee et al., 2019). Leveraging BIM tools, they optimize workflows, improve coordination, and enhance decision-making throughout the project lifecycle, maximizing BIM benefit (Ismail et al., 2019). However, challenges like a scarcity of technical expertise, resistance to change, and limited training hinder effective utilization, especially in developing countries where adoption is more industry-driven than government-mandated (Adam et al., 2022).
The effectiveness of BIM implementation is significantly influenced by key attributes of BIM staff. Team size is crucial; larger teams with diverse skill sets are better equipped for comprehensive BIM implementation and handling complex projects, leading to improved performance (Li, 2017). Experience level enhances BIM workflows, resulting in efficient coordination and better outcomes (Wang, 2020). Expertise in specific domains, such as structural engineering or architectural design, improves modeling quality and decision-making, contributing to cost savings. Effective training programs are essential for equipping BIM staff with the necessary skills, reducing errors, and enhancing productivity (Zhang et al., 2018).
BIM staff roles are multifaceted, encompassing project management, stakeholder collaboration, model creation, and analysis. They oversee BIM processes from inception to completion, ensuring centralized project information and integrating data across disciplines (Davies et al., 2017; Hosseini et al., 2018). Responsibilities also include creating detailed 3D models, conducting optimization analyses, and providing training to other team members (Joseph, 2011; Habib et al., 2022). These roles are vital for driving project success, optimizing outcomes, and ensuring efficient BIM implementation.
Research on BIM staff’s roles, challenges, and impact on project performance is essential as the construction industry increasingly adopts BIM. Although existing studies explore BIM adoption and user satisfaction, they often overlook the BIM staff influence various project performance on metrics like cost management, schedule adherence, error reduction, and stakeholder satisfaction (Abd Hamid et al., 2020; Azmi et al., 2018). Closing this research gap is crucial for developing strategies to optimize BIM implementation and enhance project outcomes. Future studies should investigate how BIM staff attributes, such as technical skills and organizational proficiency, directly affect project performance, guiding stakeholders to invest in BIM staff training and development.
1.4 Project performance
The successful implementation of BIM in construction projects is closely tied to various project performance indicators such as cost management, schedule adherence, error reduction, and stakeholder satisfaction. Key performance indicators (KPIs) in BIM projects, including cost performance, schedule performance, quality improvement, safety enhancement, and client satisfaction, serve as benchmarks for evaluating BIM’s success (Chan and Chan, 2004; Okudan et al., 2022; Ansari et al., 2022; Cox et al., 2003). BIM-enabled projects typically show improvements in these areas due to enhanced collaboration, clash detection, and data-driven decision-making. However, the specific contributions of BIM staff to these performance improvements require further investigation.
A comprehensive understanding of BIM’s tangible impacts necessitates a nuanced examination of performance metrics, particularly regarding BIM staff’s role in project outcomes. As organizations increasingly integrate BIM, it is crucial to understand how the expertise, size, training, and characteristics of BIM staff contribute to project performance. Existing literature acknowledges BIM’s transformative potential in enhancing project efficiency and collaboration (Ratajczak et al., 2019; Kim et al., 2021). For example, BIM implementation during construction phases can identify and resolve significant cost issues, with some studies highlighting a 15.92% impact on total project costs due to design error identification and resolution (Kim et al., 2017). This review critically evaluates empirical studies on the relationship between BIM staff attributes—such as expertise, size, training, and characteristics—and project performance, providing a foundation for understanding the interplay between human factors and BIM implementation in the construction industry.
2 Methodology
2.1 Study location
The study was conducted in Kenya specifically the Kenyan Construction Industry in the counties of Nairobi, Kisumu, Nakuru and Kiambu. The population directly involved can be identified as the aggregate of elements possessing the attributes or information of interest to the researcher and for which inferences are to be drawn (Marczyk, 2006). The study focused on construction firms and government agencies in Nairobi, Mombasa, Nakuru, and Kiambu counties in Kenya. These locations were chosen to provide a diverse representation of the construction industry, reflecting various geographic regions, urbanization levels, and development contexts. This selection ensures a comprehensive understanding of BIM technology’s impact across different settings in Kenya.
2.2 Study design
A survey research design was employed, consistent with deductive research methods (Saunders et al., 2012). The research approach highlighted in Figure 4 adopted a deductive approach, which aligns with the positivist model, allowing for theory formulation and empirical testing of anticipated outcomes (Bryman, 2012). Researchers employing the deductive approach begin by establishing an abstract, logical relationship among concepts, before progressing towards concrete empirical validation (Kreuger and Allynand Bacon, 2006).
The study aimed to uncover causal relationships between variables such as BIM staff attributes, task alignment, technology characteristics, individual characteristics, project characteristics, and project performance indicators. A quantitative approach was chosen for its suitability in evaluating theories and generalizing results (Sarstedt et al., 2021; Homburg et al., 2021). The focus was on BIM staff in BIM-enabled construction projects in Kenya.
2.3 Target population
The population involved is identified as the aggregate of elements possessing the attributes or information of interest to the researcher and from which inferences are to be drawn (Marczyk, 2006). For this study, construction firms and government agencies in Kenya constituted the population. This included major firms as well as national and local government agencies located in Nairobi, Mombasa, Nakuru, and Kiambu Counties in Kenya.
These counties were selected to provide a diverse representation of Kenya’s construction industry, encompassing various geographic regions, levels of urbanization, and development contexts.
2.4 Sampling
In the current study, purposive sampling is chosen due to the dispersed nature of Building Information Modelling (BIM) professionals across different sites and cities. Despite the practical challenges of approaching all construction firms and BIM professionals within a restricted timeframe, significant efforts were made to collect data from a sample of BIM professionals in Kenya. This method is deemed appropriate for the study, and BIM staff are selected as respondents based on previous studies, (Stemn et al., 2019; Zhu et al., 2020), ensuring a focus on factors influencing their adoption and implementation of BIM.
2.5 Data collection and tools
Primary data were collected using a questionnaire, a common tool in surveys (Dörnyei and Taguchi, 2009). The study employed the self-administered approach, although respondents were encouraged to contact the researcher for guidance or clarification as needed. Both electronic (e-surveys) and print versions were the designs employed for the questionnaire, to facilitate maximum participation by firms. The questionnaire was organized into three main sections:
Section A: Individual and project profile, covering demographics such as gender, age, years of experience, respondent’s profession, project phase, and expertise level.
Section B: Independent variables, encompassing four constructs—BIM staff attributes, Project Characteristics, Technology Characteristics.
Section C: Moderating variables, consisting of three constructs—UTAUT and TTF.
The questionnaire was initially tested for accuracy with BIM professionals in Henan Province, China. Subsequently, it was distributed to 50 AEC firms approved by the National Construction Authority and Board of Registration of Architects and Quantity Surveyors of Kenya (BORAQS), and emailed to 500 licensed engineers, architects, quantity surveyors, MEP, and construction project manager registered with the Engineers Board of Kenya (EBK) and BORAQS in Kenya. A total of 412 valid responses were received and processed. A five-point Likert scale, ranging from 5 (strongly agree) to 1 (strongly disagree) was used.
2.6 Survey instruments
The survey instruments were designed from the methodological approach in Survey sections
1. Demographics: Basic information about the respondents.
2. BIM Staff Attributes:
• Size: Number of BIM staff members involved in the project.
• Experience: Years of experience working with BIM technologies.
• Expertise: Proficiency and specialization in BIM-related tasks.
• Training: Extent and quality of BIM-specific training received.
3. Task-Technology Fit (TTF) Constructs:
• Fit: Alignment between task requirements and technology capabilities.
• Task Characteristics (Project Characteristics): Nature and requirements of tasks performed in the project.
5. Technology Characteristics:
• Features and capabilities of the BIM technology.
• Usability: Ease of use of BIM technology by staff.
• Compatibility: Integration of BIM technology with existing workflows and systems.
6. Performance Impact (Project Performance Indicators):
• Cost Management: Effectiveness in controlling project costs.
• Schedule Adherence: Ability to meet project timelines.
• Error Reduction: Enhancements in project quality due to BIM.
• Stakeholder Satisfaction: Satisfaction of project stakeholders with BIM-enabled outcomes.
7. UTAUT Constructs (Utilization Construct in TTF):
• Performance Expectancy: Users’ belief in BIM’s contribution to job performance gains.
• Effort Expectancy: Perceived ease of use associated with BIM technology.
• Social Influence: Perception of the importance of BIM use by influential others.
• Facilitating Conditions: Belief in organizational and technical support for BIM use.
Figure 4 to gather data on BIM staff attributes, TTF, and UTAUT. The questionnaire, developed from validated instruments and tailored for BIM in construction, underwent pilot testing to ensure clarity and reliability. Content validity was established through expert reviews, and internal consistency was measured using Cronbach’s alpha.
2.7 Hypothesis deduction process
The conceptual framework in Figure 5 integrates Task-Technology Fit (TTF) and the Unified Theory of Acceptance and Use of Technology (UTAUT) providing an understanding of the factors influencing BIM adoption and its impact on project performance. This study proposes the following hypotheses based on these theoretical foundations.
2.7.1 BIM staff size will significantly influence project performance
2.7.1.1 Theoretical Foundation
According to TTF, the size of the BIM team impacts how effectively BIM functionalities align with project tasks, thereby influencing project outcomes. Larger teams are likely to have more diverse skill sets and resources, enhancing project coordination and decision-making.
2.7.1.2 Contribution of TTF-UTAUT
The integrated TTF-UTAUT framework suggests that the size of the BIM team influences user perceptions (UTAUT constructs) about BIM technology’s effectiveness in supporting project tasks, thus affecting project performance indicators.
2.7.2 BIM staff experience will significantly influence project performance
2.7.2.1 Theoretical Foundation
TTF posits that the experience level of BIM staff affects their ability to leverage BIM functionalities effectively within project contexts. Experienced staff are expected to contribute to smoother project execution and improved outcomes.
2.7.2.2 Contribution of TTF-UTAUT
In the TTF-UTAUT model, BIM staff experience influences their perceptions of effort expectancy and performance expectancy (UTAUT constructs), which in turn impact how BIM tools are utilized and ultimately influence project performance.
2.7.3 BIM staff expertise will significantly influence project performance
2.7.3.1 Theoretical Foundation
TTF emphasizes that expertise in specific domains (e.g., structural engineering, architectural design) enhances the quality of BIM modeling and analysis, leading to better decision-making and cost savings.
2.7.3 2 Contribution of TTF-UTAUT
Within the TTF-UTAUT framework, BIM staff expertise is crucial for perceived usefulness and perceived ease of use (UTAUT constructs), influencing their acceptance and adoption of BIM technologies, thereby affecting project performance outcomes.
2.7.4 BIM staff training will significantly influence project performance
2.7.4.1 Theoretical Foundation
TTF suggests that training programs improve BIM staff’s technical skills and knowledge, leading to more effective utilization of BIM tools and methodologies throughout the project lifecycle.
2.7.4.2 Contribution of TTF-UTAUT
In the TTF-UTAUT model, training programs impact facilitating conditions (UTAUT construct), which support BIM adoption and utilization. Enhanced training is expected to positively influence how well BIM tasks align with technology capabilities, thereby improving project performance.
2.7.5 Technology characteristics (e.g., Usability, Compatibility) positively influence TTF
2.7.5.1 Theoretical Foundation
TTF highlights that favorable technology characteristics enhance the alignment between technology capabilities and user tasks, facilitating better task-technology fit.
2.7.5.2 Contribution of TTF-UTAUT
Within the TTF-UTAUT framework, favorable technology characteristics influence performance expectancy and effort expectancy (UTAUT constructs), which contribute to users’ perceptions of task-technology fit and ultimately impact project performance.
2.7.6 TTF significantly influences project performance indicators
2.7.6.1 Theoretical Foundation
TTF posits that the degree of alignment between technology and task requirements directly affects project performance indicators such as cost management, schedule adherence, and quality improvement.
2.7.6.2 Contribution of TTF-UTAUT
In the integrated model, TTF’s influence on user perceptions (UTAUT constructs) affects their behavioral intentions and actual use of BIM technologies, thereby influencing project performance indicators.
2.7.7 UTAUT will significantly affect TTF
2.7.7.1 Theoretical Foundation
UTAUT explains that user acceptance and behavioral intentions are shaped by performance expectancy, effort expectancy, social influence, and facilitating conditions.
2.7.7.2 Contribution of TTF-UTAUT
UTAUT constructs influence users’ perceptions of how well BIM functionalities fit with their tasks (TTF), indicating that positive UTAUT outcomes lead to greater perceived task-technology fit and vice versa.
2.7.8 UTAUT constructs will significantly mediate the positive relationship between TTF and project performance indicators
2.7.8.1 Theoretical Foundation
UTAUT constructs mediate the relationship between user perceptions and technology adoption outcomes, suggesting that favorable perceptions (e.g., performance expectancy, effort expectancy) lead to better project performance.
2.7.8.2 Contribution of TTF-UTAUT
In the integrated model, UTAUT constructs mediate how TTF influences project performance indicators. For instance, high performance expectancy and effort expectancy (UTAUT) mediated by TTF enhance project outcomes through improved task-technology fit.
These hypotheses are grounded in the foundational theories of TTF and UTAUT, highlighting their relevance and applicability in examining BIM adoption and project performance within the construction industry. This integrated approach aims to provide a nuanced understanding of the complex interplay between human factors, technology fit, and project outcomes.
2.8 Data analysis
Structural Equation Modelling (SEM) was used to explore the impact of BIM staff on project performance. SEM is a flexible statistical technique for modelling hypotheses and examining relationships between variables, both measurable and unmeasurable. (Henseler et al., 2009). Confirmatory Factor Analysis (CFA) in SEM was employed to test the measurement model, ensuring that the latent constructs were measured accurately and reliably. Evaluating convergent validity, construct validity, and discriminant validity was crucial for drawing meaningful conclusions about the relationships between constructs in the theoretical model.
3 Results, discussion and conclusion
3.1 Responses, distribution and profile of respondents
A total of 530 questionnaires were distributed; 412 questionnaires were received, reflecting a response rate of 77.7%. Predominantly, respondents were within the 31–40-year age group, comprising 48.1% of the sample, followed by the 20–30-year age group at 24.5%, and the 40–50-year age group at 16.5% as shown in Table 1.
The concentration within the 31–40-year age group aligns with broader industry trends, suggesting this cohort is a significant part of the construction workforce (Asis et al., 2023).
The data in Figure 6 indicates that a significant portion of staff (219) have received BIM training, with most being “Advanced Beginners” (215) or “Proficient” (27). However, a considerable number (119) are “Novice,” highlighting a need for more training. Most staff have 0–5 years of BIM experience (189), with smaller groups having 6–10 years (99), 11–15 years (39), and over 15 years (36). A notable 49 individuals have never used BIM, suggesting gaps in training or adoption.
3.2 Measurement model
By using CFA in SEM, we rigorously tested the measurement model and ensure that the latent constructs are measured accurately and reliably. This validation process is crucial for drawing meaningful conclusions about the relationships between constructs shown in Table 2 the theoretical model (Wang et al., 2015).
Multicollinearity in factor analysis can compromise the validity and reliability of the results. High correlations among variables lead to an unreliable factor structure, making accurate interpretation difficult. This results in inconsistent loadings, inflated standard errors, and reduced discriminant validity, making it hard to distinguish between factors. The presence of multicollinearity can reduce the stability of factors, hindering replication across datasets and limiting generalizability. It can also cause misinterpretation of factor importance and parameter estimation instability, leading to biased conclusions. Reduced power to detect the true factor structure may result in model misfit, preventing the identification of underlying data patterns.
To address multicollinearity, we analysed the correlation matrix to identify highly correlated variables and their impact. The Variance Inflation Factor (VIF) shown in Table 3 measured the inflation of regression coefficients due to correlated predictors, while tolerance (the reciprocal of VIF) indicates the proportion of variance in a predictor not shared with others, providing insight into the severity of multicollinearity (Dohoo et al., 1997).
3.2.1 Convergent validity
Convergent validity Convergent validity measures how well different indicators of the same construct correlate with each other. This is assessed using metrics such as Composite Reliability (CR), Cronbach’s Alpha, and Average Variance Extracted (AVE) (Wang et al., 2015). The data in Table 4 from the PLS-SEM analysis shows that indicators for BIM staff size, experience, expertise, training, project characteristics, and performance have strong loadings (>0.7), reflecting a solid relationship with the constructs. Both Cronbach’s Alpha and CR values exceed 0.7, indicating good internal consistency and reliability.
3.2.2 Discriminant validity
Discriminant validity confirms that each construct is unique and not just an extension of another. It is established when a construct’s value exceeds those of other constructs (Henseler et al., 2015). The Fornell and Larcker criteria, shown in the provided Table 5, present the square root of the Average Variance Extracted (AVE) on the diagonal and correlations off-diagonal (Hair et al., 2012; Hair et al., 2014). The AVE values are higher than the correlations, indicating discriminant validity (Ab Hamid et al., 2017). Additionally, the Heterotrait-Monotrait ratio (HTMT) assessment shows that all HTMT values are below 0.90, confirming non-multicollinearity and further supporting discriminant validity.
3.3 Structural model
In assessing the structural model, we analysed the direct relationships between constructs using a method called bootstrapping, which involved resampling the data 5,000 times to ensure the robustness of the results. Additionally, R2 values were calculated to determine the proportion of variance in the dependent variables that could be explained by the predictor variables in the model. Several common criteria should be considered, including the coefficient of determination (R-squared), the blindfolding-based cross-validated redundancy measure (Q-squared), and the statistical significance and relevance of the path coefficients (Hair et al., 2019).
3.3.1 Coefficient and predictive relevance
PLS predict approach should be utilized to evaluate the model’s out-of-sample predictive power. The R-squared value measures the variance explained by each endogenous construct and serves as an indicator of the model’s explanatory power (Shmueli et al., 2016). It ranges from 0 to 1, with higher values representing greater explanatory power; R-squared values of 0.75, 0.50, and 0.25 indicate strong, moderate, and weak relationships, respectively (Hair et al., 2019). The findings show that the major dependent variable, Project), had a high R-squared value of 0.736, explaining about 71.9% of the variance. A model’s predictive relevance is assessed by its Q-squared value, which should be greater than 0, with values of 0.02, 0.15, and 0.35 indicating weak, moderate, and high predictive relevance, respectively. The study’s Q values suggest that the proposed model has adequate predictive value. The results of the R-squared and Q-squared values can be found in Table 6.
3.3.2 Path model
The path model in Figure 7 shows that technology character, Staff attributes, and the UTAUT explain about 60.8% of the variability in TTF. The moderating effects are not significant in the model. Given that the moderating impacts are not statistically significant, it could be inferred that task technology fit accounts for about 49.5% of the variations in project performance.
3.4 Impact of BIM staff on project performance
The influence of Building Information Modelling (BIM) staff on construction project performance is significant and multifaceted, illustrating a clear connection between BIM team attributes and project success. Research indicates that larger and more skilled BIM teams generally enhance project performance by improving design efficiency, reducing errors, and adhering to deadlines and budgets (Olanrewaju et al., 2021; Begić and Galić, 2021).
The statistical analysis in Table 7 reveals that BIM staff attributes have a significant direct positive effect on project performance, with a beta coefficient (β) of 0.151, a t-value of 3.564, and a p-value of 0.001. This strong statistical significance underscores that the skills, experience, and attributes of BIM staff directly contribute to the success and overall performance of construction projects. Competent BIM teams can streamline workflows, improve coordination, and ensure better adherence to project schedules and budgets (Higgs et al., 2005; Davies et al., 2015). The expertise and training of BIM staff are crucial factors in achieving these positive outcomes, as knowledgeable and well-trained staff are better equipped to handle the complexities and challenges of modern construction projects (Zhang et al., 2018).
Additionally, the attributes of BIM staff also impact project performance indirectly by influencing TTF. The same statistical strength is observed in this indirect relationship, with a beta coefficient (β) of 0.438, a t-value of 7.221, p-value of 0.001, a 95% confidence interval of (0.374, 0.502). This suggests that the competencies of BIM staff significantly enhance how well the technology fits the tasks at hand, which in turn leads to improved project performance. When BIM staff are adept at leveraging the technology available to them, they can more effectively align technological tools with project requirements, thereby maximizing efficiency and minimizing errors (Ham and Yuh, 2023). This alignment is crucial for optimizing project outcomes, as it ensures that the technology supports rather than hinders the construction processes.
While overall trends show positive impacts, variations in staff experience and task alignment can arise from resistance to new methods or organizational barriers. Experienced staff might resist new technologies due to familiarity with traditional practices or perceived complexity (Chan, 2014; Jiang, 2019). Additionally, inadequate training and support can hinder effective technology integration (Hamma-adama et al., 2020; Liao and Ai Lin, 2018). To maximize BIM staff benefits, fostering a learning environment and addressing these challenges is crucial. In summary, skilled and well-supported BIM teams significantly enhance project performance through better design efficiency, fewer errors, and adherence to schedules and budgets. Addressing resistance and organizational barriers is essential for optimizing these benefits.
3.5 Impact of technology characteristics on task technology fit
The research findings reveal a profound influence of Building Information Modelling (BIM) tool characteristics on Task Technology Fit (TTF) and Project Performance in construction projects.
Statistical analysis in Table 7 reveals a significant direct positive impact, supported by a beta coefficient (β) of 0.524, a t-value of 7.630, and a p-value of 0.001. This emphasizes the pivotal function of BIM tool qualities in aligning technology with task requirements, highlighting their importance in project success.
Moreover, the study reveals that Technology Characteristics demonstrate significant direct effects on both Project Performance and TTF, as indicated by respective beta coefficients (β) of 0.181 and 0.524, t-values of 5.765 and 7.630, and p-values of 0.001 for both. This suggests that favourable technology characteristics positively contribute to project outcomes and the alignment of technology with task requirements, further underlining their significance in project success (Sloot et al., 2019; Biancardo et al., 2020; Tsai et al., 2014).
However, the combined influence of Staff Attributes and Technology Characteristics does not show significant direct effects on Project Performance or TTF, with beta coefficients (β) of 0.020 and 0.057, t-values of 1.816 and 1.671, and p-values of 0.069 and 0.095, respectively. The non-significant combined influence of Staff Attributes and Technology Characteristics on Project Performance or TTF can be attributed to the complexity of their interactions, mediating and moderating factors, initial integration challenges, individual variances, and contextual differences. Past research underscores the multifaceted nature of technology adoption and its impacts in organizational settings (Venkatesh et al., 2003; Orlikowski and Iacono, 2001; Delone and McLean, 1992).
Interestingly, the indirect effect pathway in Table 8 from Technology Characteristics to TTF and then to Project Performance is significant, with a beta coefficient (β) of 0.181, a t-value of 5.765, p-value of 0.000, with a 95% confidence interval not including zero (CI: 0.140, 0.222). This suggests that Technology Characteristics influence Project Performance partially through their impact on TTF, further highlighting the intricate relationship between technology attributes, TTF, and project outcomes.
The absence of significant moderating effects for Staff Attributes on the relationship between Technology Characteristics and TTF or Project Performance suggests that individual or team characteristics may not significantly alter the direct impact of technology characteristics. This lack of moderation could be attributed to several factors: complex interactions that require more detailed models, initial integration challenges that overshadow staff attributes’ roles, and contextual differences that might dilute the moderating effects. These aspects highlight the need for further research to better understand the nuanced dynamics between staff characteristics and technology adoption.
Overall, the findings highlight the crucial role of BIM tool characteristics in enhancing construction project performance. Usability, functionality, and reliability are key factors: user-friendly tools facilitate smooth integration and reduce errors, comprehensive functionality addresses various project needs, and reliable tools ensure consistent performance. These attributes improve Task Technology Fit (TTF), boost productivity, and ultimately enhance project success by aligning technology more effectively with task requirements.
3.6 Impact of task technology fit on project performance
The research findings highlight the significant positive impact of Task Technology Fit (TTF) on construction project performance. The statistical analysis in Table 7 robustly supports this conclusion, demonstrating a beta coefficient (β) of 0.346, a t-value of 5.606, and a p-value of 0.001, 95% CI [0.250, 0.450]. These results emphasize the critical importance of ensuring that the technology used in construction projects aligns well with the tasks it is intended to support.
Staff Attributes - > TTF - > Project Performance in Table 8 showed a significant indirect effect (β = 0.151, 95% CI [0.100, 0.200], t = 3.564, p = 0.000), indicating that Staff Attributes influence Project Performance partially through their impact on TTF. A good fit between technology and tasks streamlines processes and enhances efficiency. This alignment provides the necessary tools and features tailored to specific task requirements, allowing staff to complete their work more quickly and with fewer errors. The resulting efficiency leads to significant time savings and cost reductions, which are crucial for successful project performance.
The significant mediation of Task-Technology Fit (TTF) highlights that aligning technology with task requirements is essential for enhancing project performance through staff attributes. This underscores the importance of investing in technology that matches task needs. However, the lack of notable moderating effects of UTAUT constructs on the TTF-project performance relationship suggests that initial integration challenges, insufficient training, and the complexity of construction projects may overshadow the impact of technology acceptance on this fit and project outcome.
A strong Task-Technology Fit (TTF) boosts project performance by ensuring that technology effectively supports tasks, leading to better decision-making, communication, and timely information. This alignment helps meet deadlines, control costs, and maintain quality, reducing delays and overruns. For optimal project outcomes, construction managers should choose and integrate technologies that align well with task requirements, emphasizing the critical role of TTF in achieving efficiency and high-quality deliverables.
3.7 Effects of UTAUT on project performance
This research explores how technology acceptance (UTAUT), Task Technology Fit (TTF), and project performance interact in construction projects. It tests the direct and indirect effects of UTAUT on project performance, mediated by TTF, and examines the moderating effects of UTAUT on the relationship between TTF and project performance.
Notably, the interaction effects between UTAUT and TTF as in Table 7 did not yield a significant direct effect on Project Performance (β = −0.044, 95% CI [0.319, 0.579], t = 1.158, p = 0.247), indicating their combined influence does not notably impact project outcomes.
However, the UTAUT demonstrates significant direct effects on both Project Performance (β = 0.449, t = 7.958, p = 0.001) and Task Technology Fit (β = −0.126, 95% CI [-0.211, −0.041], t = 3.030, p = 0.002), underscoring its influential role in shaping these factors.
The study as in Table 8 further reveals a significant indirect effect pathway, wherein UTAUT influences Project Performance partially through its impact on TTF (β = −0.043, 95% CI [-0.073, −0.013], t = 2.886, p = 0.004), highlighting the mediating role of TTF in technology acceptance’s influence on project outcomes.
Technology acceptance significantly enhances project performance, facilitating improvements in efficiency, effectiveness, and overall success (β = 0.449, t = 7.958, p = 0.001). Employees’ embrace of technology correlates with its more effective use, driving positive project outcomes.
Intriguingly, technology acceptance negatively impacts TTF (β = −0.126, t = 3.030, p = 0.002), suggesting potential challenges in aligning technology with specific task requirements despite high acceptance levels. This may stem from initial integration hurdles or a need for additional training.
TTF partially mediates the relationship between technology acceptance and project performance (β = −0.043, t = 2.886, p = 0.004), as shown Table 8 implying that while technology acceptance directly boosts project performance, its influence is also mediated by its effect on TTF.
The lack of significant moderating effects might be due to several factors. High technology acceptance can still face practical hurdles during initial implementation, such as integration issues, resistance to change, or a steep learning curve with new BIM tools, which can temporarily mitigate the benefits of task-technology fit. Additionally, the availability and quality of training and support are crucial for effective BIM utilization. Without adequate training, even positively accepted technology may not reach its full potential, thus limiting its impact on project performance. Furthermore, the inherent complexity and variability of construction projects, including factors like project size, scope, team dynamics, and unforeseen challenges, can overshadow the expected moderating impacts of UTAUT and TTF interactions on project performance (Davies and Harty, 2013; Bekr, 2017; Ghaffarianhoseini et al., 2017).
The research underscores the nuanced nature of technology adoption in construction projects, emphasizing the importance of fostering both a tech-friendly culture and ensuring technology aligns effectively with task requirements. Managers should prioritize efforts to promote technology acceptance while addressing integration challenges, providing comprehensive training, and customizing technology features, ultimately optimizing project performance.
3.8 Critical factors of BIM staff
Several critical factors influence the effectiveness of Building Information Modelling (BIM) staff in enhancing project performance. Research indicates that various aspects such as staff size, training, expertise, experience, project characteristics, and task-technology fit play significant roles in determining how well BIM staff can contribute to successful project outcomes. Larger BIM teams lead to better project outcomes, improving design efficiency, schedule adherence, and budget compliance. They offer diverse skills and knowledge, aiding in handling complex project requirements effectively. Well-staffed teams evenly distribute workload, cross-check thoroughly, and provide diverse perspectives for enhanced project quality (Chan et al., 2019; Van Tam et al., 2023).
The training, expertise, and experience of BIM staff are pivotal in driving project success. Skilled and experienced BIM professionals contribute significantly to project efficiency by minimizing errors and optimizing design processes (Huh et al., 2023; Ham and Yuh, 2023; Ham et al., 2020). Training ensures that staff are up-to-date with the latest BIM technologies and methodologies, enabling them to implement best practices effectively. Expertise and experience allow staff to anticipate potential issues and devise solutions proactively, thereby streamlining workflows and improving project outcomes.
The size and complexity of a project are crucial factors that moderate the impact of BIM staff on project performance. Larger and more complex projects benefit more from well-equipped and experienced BIM teams, as these teams can manage intricate details and extensive coordination requirements better (Bosch-Sijtsema et al., 2019; Wang K. et al., 2022). Understanding the specific characteristics of a project is essential for optimizing the deployment of BIM resources. Tailoring BIM strategies to suit the project’s scale and complexity can lead to more efficient management and execution.
The alignment between tasks and technology features, known as Task Technology Fit (TTF), is significantly influenced by BIM staff attributes and organizational support. The expertise and attributes of BIM staff enhance the alignment between technology and task requirements, facilitating better TTF (Zhao et al., 2023). This alignment is crucial for project performance, as it ensures that the technological tools are used to their full potential, effectively supporting the tasks at hand. When BIM staff can effectively match the capabilities of the technology with the specific needs of the project, they can achieve higher efficiency and better outcomes.
The statistical analysis highlights a significant direct positive effect of BIM staff attributes on Task Technology Fit, with a beta coefficient (β) of 0.438, a t-value of 7.221, and a p-value of 0.001. This indicates that the skills, experience, and attributes of BIM staff greatly enhance the alignment between technology and task requirements. This improved alignment facilitates better TTF, which in turn positively impacts project performance. Competent BIM staff ensure that the technology is appropriately leveraged, leading to more efficient processes and successful project outcomes (Zhao et al., 2023; Ganiyu et al., 2020).
BIM staff effectiveness in improving project performance depends on team size, staff training and experience, and project characteristics like size and complexity. Aligning tasks with technology, aided by skilled BIM staff, is essential for optimizing performance (Ganiyu et al., 2020; Ahadzie et al., 2014; Egbu, 1999). Addressing these factors enables construction projects to fully leverage BIM potential for superior results.
3.9 Theoretical and managerial implications
The findings highlight that technology acceptance positively impacts project performance, leading to improved efficiency, effectiveness, and overall success. However, there is a notable negative effect on TTF, suggesting that while technology is accepted, it may not immediately align perfectly with task requirements, indicating initial integration challenges.
The study also introduces project characteristics, such as size and complexity, as moderating factors in the relationship between BIM staff attributes and project performance. This suggests that understanding these variables is essential for predicting project outcomes accurately.
For practitioners in the Kenyan construction industry, the research offers actionable insights:
Strategic Management of BIM Staff: Optimizing project performance requires strategic management of BIM team size, training, expertise, and experience. Larger and more skilled BIM teams correlate with better project outcomes.
Understanding Project Characteristics: Tailoring BIM strategies to the specific needs of each project, considering its size and complexity, can enhance management and execution, leading to improved outcomes.
Enhancing Organizational Support: Fostering a supportive culture for innovation, providing necessary resources for training, and ensuring access to the latest technology are vital. Enhanced organizational support improves task-technology alignment, reducing errors and increasing efficiency.
Emphasizing Task-Technology Fit: Selecting and implementing technologies that are well-suited to project tasks and ensuring staff proficiency in these tools are crucial for reducing errors, enhancing efficiency, and improving stakeholder satisfaction.
Enhanced Focus on Task-Technology Fit: Since TTF mediates the impact of technology acceptance on project performance, organizations should focus on ensuring a high degree of alignment between BIM tools and specific project tasks. This can be achieved through customization of BIM tools, targeted training programs, and continuous feedback loops.
Broader Strategy Development: The absence of significant moderating effects implies that a broader range of factors should be considered in BIM implementation strategies. This includes organizational culture, project management practices, and external environmental factors.
Theoretically, the study contributes to existing frameworks by emphasizing the importance of technology acceptance and TTF and introducing project characteristics as moderating factors. Managerially, the findings suggest that strategic management of BIM staff, understanding project characteristics, enhancing organizational support, and focusing on task-technology fit are key to optimizing project performance. By incorporating these insights, practitioners in the Kenyan construction industry can achieve more efficient, effective, and successful project outcomes.
3.10 Contribution of the study in BIM adoption and implementation in Kenya
This study advances the existing knowledge of BIM adoption in developing countries, particularly in Kenya, through the following contributions.
3.10.1 Context-specific insights
3.10.1.1 Tailored to Kenya
By focusing on the Kenyan construction industry, the study provides context-specific insights that are directly applicable to the unique challenges and opportunities within the country. This fills a gap in the existing literature, which often generalizes findings from developed countries without considering local nuances.
3.10.1.2 Regional Relevance
The findings highlight the importance of considering regional factors such as local market conditions, regulatory environments, and industry practices, which are crucial for effective BIM implementation in developing countries like Kenya.
3.10.2 Effective use of BIM staff
3.10.2.1 Localized Training Needs
The study underscores the necessity of investing in localized training programs to build a well-trained BIM workforce. This addresses a common barrier in developing countries where access to advanced training resources may be limited (Mwangi et al., 2021).
3.10.2.2 Impact on Project Performance
Demonstrating the significant impact of well-trained BIM teams on project performance provides a compelling case for stakeholders in Kenya to prioritize human resource development, aligning with the findings of Musa et al. on the importance of skilled labor in BIM adoption (Kambur and Akar, 2022; Nguyen et al., 2021; Maharika et al., 2020).
3.10.3 Project characteristics
3.10.3.1 Adaptation to Local Project Types
By emphasizing the need to understand project size and complexity, the study guides the strategic deployment of BIM resources tailored to the types of projects commonly undertaken in Kenya, such as infrastructure and affordable housing projects. This is supported by Olatunji et al., who stress the importance of context in BIM adoption strategies (Olatunji et al., 2021; Ahuja et al., 2020; Wang Z. et al., 2022; Herr and Fischer, 2019).
3.10.3.2 Scalability and Flexibility
The study’s insights on managing large and complex projects with BIM are particularly relevant for Kenya’s growing construction sector, which is increasingly engaging in large-scale urban development projects (Pilehchian et al., 2015).
3.10.4 Task-technology fit (TTF)
3.10.4.1 Appropriate Technology Selection
The study’s emphasis on task-technology fit highlights the need for selecting BIM tools that are suitable for local practices and conditions. This contributes to the broader understanding of technology adoption in developing contexts, where off-the-shelf solutions may not always be the best fit (Yap et al., 2022).
3.10.4.2 Continuous Training and Evaluation
Emphasizing ongoing training and evaluation aligns with the dynamic nature of technology adoption in developing countries, where continuous improvement is crucial for overcoming initial adoption barriers (Arayici et al., 2011; Taib et al., 2023).
3.10.5 Overcoming challenges
3.10.5.1 Organizational Support and Culture
The study identifies organizational support as a key factor for successful BIM adoption, resonating with the findings of He et al. (2012) on the critical role of organizational culture in technology adoption in developing countries (Merschbrock and Erik Munkvold, 2012; Ifenthaler and Egloffstein, 2020; Dasgupta and Gupta, 2019).
3.10.5.2 Promoting Collaboration
Highlighting the need for collaborative environments supports the creation of knowledge-sharing networks, which are essential in contexts where individual firms may lack resources (Brewer and Gajendran, 2012).
3.10.6 Optimizing resources
3.10.6.1 Resource Efficiency
The study’s focus on resource optimization addresses the economic constraints typical in developing countries. By demonstrating how BIM can lead to cost savings and increased profitability, it provides a practical incentive for adoption (Barlish and Sullivan, 2012).
3.10.6.2 Strategic Alignment
Aligning BIM tools with specific project needs and resource availability enhances the practicality of BIM implementation strategies, making them more accessible for firms operating under tight budgets (Jung and Joo, 2011).
By addressing these specific aspects, the study not only provides actionable insights for the Kenyan construction industry but also contributes to the broader discourse on BIM adoption in developing countries. It emphasizes the importance of contextualized strategies, localized training, and organizational support, offering a comprehensive framework for overcoming the unique challenges faced by developing nations in adopting advanced construction technologies.
Data availability statement
The raw data supporting the conclusions of this article will be made available by the authors, without undue reservation.
Author contributions
YC: Funding acquisition, Resources, Supervision, Validation, Visualization, Writing–review and editing, Methodology. HFG: Conceptualization, Data curation, Formal Analysis, Investigation, Methodology, Software, Writing–original draft, Writing–review and editing. GS: Supervision, Validation, Visualization, Writing–review and editing.
Funding
The author(s) declare that no financial support was received for the research, authorship, and/or publication of this article.
Acknowledgments
We are grateful to Drokow Emmanuel Kwateng for proof reading our work.
Conflict of interest
The authors declare that the research was conducted in the absence of any commercial or financial relationships that could be construed as a potential conflict of interest.
Publisher’s note
All claims expressed in this article are solely those of the authors and do not necessarily represent those of their affiliated organizations, or those of the publisher, the editors and the reviewers. Any product that may be evaluated in this article, or claim that may be made by its manufacturer, is not guaranteed or endorsed by the publisher.
References
Abbad, M. M. M. (2021). Using the UTAUT model to understand students’ usage of e-learning systems in developing countries. Educ. Inf. Technol., 26, 7205, 7224. doi:10.1007/s10639-021-10573-56).
Abbas, S. K., Hassan, H. A., Asif, J., Ahmed, B., Hassan, F., and Haider, S. S. (2018). Integration of TTF, UTAUT, and ITM for mobile banking adoption. Int. J. Adv. Eng. Manag. Sci., 4, 375, 379. doi:10.22161/ijaems.4.5.65).
Abd Hamid, A. B., Embi, M. R., Mohd, T. M. Z., and Abdul Razak, A. H. N. (2020). Enhancing the knowledge and proficiency for interior designers in Malaysia through the implementation building information modelling. IOP Conf. Ser. Mater. Sci. Eng., 713(1): 1. doi:10.1088/1757-899X/713/1/012047
Ab Hamid, M. R., Sami, W., and Mohmad Sidek, M. H. (2017). Discriminant validity assessment: use of Fornell and larcker criterion versus HTMT criterion. J. Phys. Conf. Ser. 890 (1), 012163. doi:10.1088/1742-6596/890/1/012163
Adam, V., Manu, P., Mahamadu, A. M., Dziekonski, K., Kissi, E., Emuze, F., et al. (2022). Building information modelling (BIM) readiness of construction professionals: the context of the Seychelles construction industry. J. Eng. Des. Technol. 20 (3), 823–840. doi:10.1108/jedt-09-2020-0379
Adekunle, S. A., Ejohwomu, O., and Aigbavboa, C. O. (2021). Building information modelling diffusion research in developing countries: a user meta-model approach. Buildings, 11, 264, doi:10.3390/buildings110702647).
Adepoju, O. (2022) Implications for industry. Academia and government. Springer international publishing, Building Information Modelling Springer Tracts in Civil Engineering. 20220: 43–64.
Ahadzie, D. K., Proverbs, D. G., and Sarkodie-Poku, I. (2014). Competencies required of project managers at the design phase of mass house building projects. Int. J. Proj. Manag., 32, 958, 969. doi:10.1016/j.ijproman.2013.10.0156).
Ahuja, R., Sawhney, A., Jain, M., Arif, M., and Rakshit, S. (2020). Factors influencing BIM adoption in emerging markets – the case of India. Int. J. Constr. Manag., 20, 65, 76. doi:10.1080/15623599.2018.14624451).
Aladağ, H., Demirdöğen, G., Demirbağ, A. T., and Işık, Z. (2023). Understanding the perception differences on BIM adoption factors across the professions of AEC industry. Ain Shams Eng. J., 14(11): 102545, doi:10.1016/j.asej.2023.102545
Alasmari, E., Martinez-Vazquez, P., and Baniotopoulos, C. (2022). A systematic literature review of the adoption of building information modelling (BIM) on life cycle cost (LCC). Buildings, 12, 1829, doi:10.3390/buildings1211182911).
Al-Rahmi, A. M., Shamsuddin, A., Wahab, E., Al-Rahmi, W. M., Alturki, U., Aldraiweesh, A., et al. (2022). Integrating the role of UTAUT and TTF model to evaluate social media use for teaching and learning in higher education. Front. Public Health, 10, 905968, doi:10.3389/fpubh.2022.905968
Alyoussef, I. Y. (2021a). Massive open online course (Moocs) acceptance: the role of task-technology fit (ttf) for higher education sustainability. Sustain. Switz., 13, 7374, doi:10.3390/su1313737413).
Alyoussef, I. Y. (2021b). E-learning acceptance: the role of task–technology fit as sustainability in higher education. Sustain. Switz., , 13, 6450, doi:10.3390/su1311645011).
Ansari, R., Banihashemi, S. A., Taherkhani, R., and Moradi, S. (2022). Decision support system for analyzing key performance ndicators in construction projects management. Int. J. Eng. Trans. A Basics, 35(5). doi:10.5829/ije.2022.35.05b.03
Arayici, Y., Coates, P., Koskela, L., Kagioglou, M., Usher, C., and O’Reilly, K. (2011). Technology adoption in the BIM implementation for lean architectural practice. Automation Constr., 20(2): 189–195.
Ariono, B., Wasesa, M., and Dhewanto, W. (2022). The drivers, barriers, and enablers of building information modeling (BIM) innovation in developing countries: insights from systematic literature review and comparative analysis. Buildings, 12, 1912, doi:10.3390/buildings12111912
Asis, C. A. D. E., Licas, F. S., Bulagao, J. C., and Sciencedomain, I., (2023). Impact of work experience on the level of awareness of construction workers on using personal protective equipment. South Asian J. Soc. Stud. Econ., 2023: 42–48. doi:10.9734/sajsse/2023/v17i1629
Azhar, S. (2011). Building information modeling (BIM): trends, benefits, risks, and challenges for the AEC industry. Leadersh. Manag. Eng., 11(3): 241–252. doi:10.1061/(asce)lm.1943-5630.0000127
Azmi, N. F., Chai, C. S., Chin, L. W., and Singapore, S. (2018). “Building information modeling (BIM) in architecture, engineering and construction (AEC) industry: a case study in Malaysia[G],” in Proceedings of the 21st international symposium on advancement of construction management and real estate, 401–412.
Bagozzi, R. P. (2007). The legacy of the technology acceptance model and a proposal for a paradigm shift. J. Assoc. Inf. Syst., 8, 244, 254. doi:10.17705/1jais.001224).
Barbosa, M. J., Pauwels, P., Ferreira, V., and Mateus, L. (2016). Towards increased BIM usage for existing building interventions. Struct. Surv., 34, 168, 190. doi:10.1108/ss-01-2015-00022).
Barlish, K., and Sullivan, K. (2012). How to measure the benefits of BIM - a case study approach. Automation Constr., 24, 149, 159. doi:10.1016/j.autcon.2012.02.008
Bawack, R. E., and Kala Kamdjoug, J. R. (2018). Adequacy of UTAUT in clinician adoption of health information systems in developing countries: the case of Cameroon. Int. J. Med. Inf., 109. doi:10.1016/j.ijmedinf.2017.10.016
Begić, H., and Galić, M. (2021). A systematic review of construction 4.0 in the context of the BIM 4.0 premise. Buildings, 11, 337, doi:10.3390/buildings110803378).
Bekr, G. A. (2017). Exploring barriers in implementing building information modeling: a preliminary study. Proc. Int. Struct. Eng. Constr. 4 (1). doi:10.14455/isec.res.2017.29
Bernstein, H. M., Jones, S. A., Russo, M. A., Laquidara-Carr, D., Taylor, W., Ramos, J., et al. (2014). The business value of BIM for construction in major GLobal markets: how contractors around the world are driving innovation with building information modeling.
Biancardo, S. A., Capano, A., de Oliveira, S. G., and Tibaut, A. (2020). Integration of BIM and procedural modeling tools for road design. Infrastructures, 5, 37, doi:10.3390/infrastructures50400374).
Bosch-Sijtsema, P. M., Gluch, P., and Sezer, A. A. (2019). Professional development of the BIM actor role. Automation Constr. 97, 44–51. doi:10.1016/j.autcon.2018.10.024
Brewer, G., and Gajendran, T. (2012). Attitudes, behaviours and the transmission of cultural traits: impacts on ICT/BIM use in a project team. Constr. Innov., 12, 198, 215. doi:10.1108/147141712112159492).
Bryman, A. (2012). Social research methods Bryman. 4th Edition. Oxford, United Kingdom: OXFORD University Press.
Cai, J., Li, Z., Dou, Y., Li, T., and Yuan, M. (2023). Understanding adoption of high off-site construction level technologies in construction based on the TAM and TTF. Eng. Constr. Archit. Manag., 30, 4978, 5006. doi:10.1108/ecam-05-2022-043910).
Cao, Y., Kamaruzzaman, S. N., and Aziz, N. M. (2022). Building information modeling (BIM) capabilities in the operation and maintenance phase of green buildings: a systematic review. Buildings, 12, 830, doi:10.3390/buildings120608306).
Chan, A. P. C., and Chan, A. P. L. (2004). Key performance indicators for measuring construction success. Benchmarking, 11, 203, 221. doi:10.1108/146357704105326242).
Chan, C. T. W. (2014). Barriers of implementing BIM in construction industry from the designers’ perspective: a Hong Kong experience. ISSN J. Syst. Manag. Sci. J. Syst. Manag. Sci., 4(2): 1816–6075.
Chan, D. W. M., Olawumi, T. O., and Ho, A. M. L. (2019). Perceived benefits of and barriers to Building Information Modelling (BIM) implementation in construction: the case of Hong Kong. J. Build. Eng., 25, 100764, doi:10.1016/j.jobe.2019.100764
Chegu Badrinath, A., and Hsieh, S.-H. (2019). Empirical approach to identify operational critical success factors for BIM projects. J. Constr. Eng. Manag., 145, doi:10.1061/(asce)co.1943-7862.00016073).
Cox, R. F., Issa, R. R. A., and Ahrens, D. (2003). Management’s perception of key performance indicators for construction. J. Constr. Eng. Manag., 129, 142, 151. doi:10.1061/(asce)0733-9364(2003)129:2(142)2).
Daniel, M.University of Nairobi (2016). Influence of building information modelling adoption on completion of construction projects: a case of Nairobi county, Kenya by daniel kamanza manza a research project report submitted in partial fulfillment of the requirements fr the award of the degree. [M]. , 2016Nairobi.
Dasgupta, S., and Gupta, B. (2019). Espoused organizational culture values as antecedents of internet technology adoption in an emerging economy. Inf. Manag., 56, 103142, doi:10.1016/j.im.2019.01.0046).
Dash, A., and Sahoo, A. K. (2022). Exploring patient’s intention towards e-health consultation using an extended UTAUT model. J. Enabling Technol., 16, 266, 279. doi:10.1108/jet-08-2021-00424).
Dash, B., Sharma, P., and Swayamsiddha, S. (2023). Organizational digital transformations and the importance of assessing theoretical frameworks such as TAM, TTF, and UTAUT: a review. Int. J. Adv. Comput. Sci. Appl., 14, doi:10.14569/ijacsa.2023.01402012).
Davies, K., McMeel, D., and Wilkinson, S. (2015). “Soft skill requirements in a BIM project team[M],” in Proceedings of the 32nd international conference of CIB W78, 2015The Netherlands.
Davies, K., Wilkinson, S., and McMeel, D. (2017). A review of specialist role definitions in bim guides and standards. J. Inf. Technol. Constr., 22.
Davies, R., and Harty, C. (2013). Implementing 《site BIM》: a case study of ICT innovation on a large hospital project. Automation Constr., 30, 15, 24. doi:10.1016/j.autcon.2012.11.024
Davis, F. D., Bagozzi, R. P., and Warshaw, P. R. (1989). User acceptance of computer technology: a comparison of two theoretical models. Manag. Sci., 35(8): 982–1003. doi:10.1287/mnsc.35.8.982
Delone, W., and McLean, E. (1992). Information systems success: the quest for the dependent variable. Inf. Syst. Res., 3: 60–95. doi:10.1287/isre.3.1.60
Dishaw, M. T., and Strong, D. M. (1999). Extending the technology acceptance model with task-technology fit constructs. Inf. Manag., 36, 9, 21. doi:10.1016/s0378-7206(98)00101-31).
Dohoo, I. R., Ducrot, C., Fourichon, C., Donald, A., and Hurnik, D. (1997). An overview of techniques for dealing with large numbers of independent variables in epidemiologic studies. Prev. Veterinary Med., 29, 221, 239. doi:10.1016/s0167-5877(96)01074-43).
Dörnyei, Z., and Taguchi, T. (2009). “Questionnaires in second language research: construction, administration, and processing,” in Questionnaires in second language research: construction, administration, and processing. Second edition.
Dung, D., and Tarar, M.Chalmers University of Technology (2012). Impact of 4D modeling on construction planning. Chalmers, 2012Goteborg. Sweden.
Eadie, R., Browne, M., Odeyinka, H., McKeown, C., and McNiff, S. (2013). BIM implementation throughout the UK construction project lifecycle: an analysis. Automation Constr., 36: 145–151. doi:10.1016/j.autcon.2013.09.001
Egbu, C. O. (1999). Skills, knowledge and competencies for managing construction refurbishment works. Constr. Manag. Econ., 17, 29, 43. doi:10.1080/0144619993718081).
El Hajj, C., Martínez Montes, G., and Jawad, D. (2023). An overview of BIM adoption barriers in the Middle East and North Africa developing countries. Eng. Constr. Archit. Manag., 30, 889, 913. doi:10.1108/ecam-05-2021-04322).
Franque, F. B., Oliveira, T., and Tam, C. (2023). Continuance intention of mobile payment: TTF model with trust in an african context. Inf. Syst. Front., 25(2). doi:10.1007/s10796-022-10263-8
Ganah, A. A. (2015) Exploring the potential for integrating Building Information Modelling (BIM) and geographic information systems (GIS) in construction: a cost-benefit analysis. Automation Constr., 49: 1–8.
Ganiyu, S. A., Oyedele, L. O., Akinade, O., Owolabi, H., Akanbi, L., and Gbadamosi, A. (2020). BIM competencies for delivering waste-efficient building projects in a circular economy. Dev. Built Environ., 4, 100036, doi:10.1016/j.dibe.2020.100036
Ghaffarianhoseini, A., Tookey, J., Ghaffarianhoseini, A., Naismith, N., Azhar, S., Efimova, O., et al. (2017). Building Information Modelling (BIM) uptake: clear benefits, understanding its implementation, risks and challenges. Renew. Sustain. Energy Rev., 75(4): 1046–1053. doi:10.1016/j.rser.2016.11.083
Giel, B., Issa, R. R. A., and Olbina, S. (2019). Return on investment analysis of building information modeling in construction. EG-ICE 2010 - 17th Int. Workshop Intelligent Comput. Eng., 27(5): 511–521. doi:10.1061/(asce)cp.1943-5487.0000164
Girginkaya Akdag, S., and Maqsood, U. (2020). A roadmap for BIM adoption and implementation in developing countries: the Pakistan case. Archnet-IJAR, 14, 112, 132. doi:10.1108/arch-04-2019-00811).
Goodhue, D. L., and Thompson, R. L. (1995). Task-technology fit and individual performance. MIS Q. Manag. Inf. Syst., 19(2): 213–233. doi:10.2307/249689
Gu, V. C., and Black, K. (2021). Integration of TTF and network externalities for RFID adoption in healthcare industry. Int. J. Prod. Perform. Manag., 70, 109, 129. doi:10.1108/ijppm-11-2018-04181).
Gunasinghe, A., Hamid, J. A., Khatibi, A., and Azam, S. M. F. (2020). The adequacy of UTAUT-3 in interpreting academician’s adoption to e-Learning in higher education environments. Interact. Technol. Smart Educ., 17, 86, 106. doi:10.1108/itse-05-2019-00201).
Habib, U. E. H., Nasir, A. R., Ullah, F., Qayyum, S., and Thaheem, M. J. (2022). BIM roles and Responsibilities in developing countries: a dedicated matrix for design-bid-build projects. Buildings, 12, 1752, doi:10.3390/buildings1210175210).
Hair, J. F., Risher, J. J., Sarstedt, M., and Ringle, C. M. (2019). When to use and how to report the results of PLS-SEM. Eur. Bus. Rev., 31, 2, 24. doi:10.1108/ebr-11-2018-02031).
Hair, J. F., Sarstedt, M., Hopkins, L., and Kuppelwieser, V. G. (2014). Partial least squares structural equation modeling (PLS-SEM): an emerging tool in business research. Eur. Bus. Rev., 26, 106, 121. doi:10.1108/ebr-10-2013-01282).
Hair, J. F., Sarstedt, M., Ringle, C. M., and Mena, J. A. (2012). An assessment of the use of partial least squares structural equation modeling in marketing research. J. Acad. Mark. Sci., 40, 414, 433. doi:10.1007/s11747-011-0261-63).
Ham, N., Moon, S., Kim, J. H., and Kim, J. J. (2020). Optimal BIM staffing in construction projects using a queueing model. Automation Constr., 113. doi:10.1016/j.autcon.2020.103123
Ham, N. H., and Yuh, O. K. (2023). Performance analysis and assessment of BIM-based construction support with priority queuing policy. Buildings, 13(1): 153, doi:10.3390/buildings13010153
Hamma-adama, M. H., Kouider, T., and Salman, H. (2020). Analysis of barriers and drivers for BIM adoption. Int. J. BIM Eng. Sci., 3(1): 18–41. doi:10.54216/ijbes.030102
Hampson, K., and Tatum, C. B. (1997). Technology strategy and competitive performance in bridge construction. J. Constr. Eng. Manag., 123(2): 153–161. doi:10.1061/(asce)0733-9364(1997)123:2(153)
Henseler, J., Ringle, C. M., and Sarstedt, M. (2015). A new criterion for assessing discriminant validity in variance-based structural equation modeling. J. Acad. Mark. Sci., 43, 115, 135. doi:10.1007/s11747-014-0403-81).
Henseler, J., Ringle, C. M., and Sinkovics, R. R. (2009). The use of partial least squares path modeling in international marketing. Adv. Int. Mark., 20, 277, 319. doi:10.1108/s1474-7979(2009)0000020014
Herr, C. M., and Fischer, T. (2019). BIM adoption across the Chinese AEC industries: an extended BIM adoption model. J. Comput. Des. Eng., 6, 173, 178. doi:10.1016/j.jcde.2018.06.0012).
Higgs, M., Plewnia, U., and Ploch, J. (2005). Influence of team composition and task complexity on team performance. Team Perform. Manag. An Int. J., 11(7/8): 227–250. doi:10.1108/13527590510635134
Hilal, M., and Maqsood, T. (2017). “Toward improving BIM acceptance in facilities management: a hybrid conceptual model integrating TTF and UTAUT[C],” in Proceedings of the ninth international conference on construction in the 21st century (CITC-9).
Hilal, M., Maqsood, T., and Abdekhodaee, A. (2019). A hybrid conceptual model for BIM in FM. Constr. Innov., 19, 531, 549. doi:10.1108/ci-05-2018-00434).
Hilal, M. A., Maqsood, T., and Abdekhodaee, A. (2021). A hybrid conceptual model for BIM adoption in facilities management: a descriptive analysis for the collected data[g]//advances in science. Technology and Innovation. doi:10.1007/978-3-030-48465-1_55
Hill, R. J., Fishbein, M., and Ajzen, I. (1977). Belief, attitude, intention and behavior: an introduction to theory and research. Contemp. Sociol., 6, 244, doi:10.2307/20658532).
Homburg, C., Klarmann, M., and Vomberg, A.Springer (2021). Handbook of market research[M]. Handbook of market research, 2021.
Hosseini, M. R., Martek, I., Papadonikolaki, E., Sheikhkhoshkar, M., Banihashemi, S., and Arashpour, M. (2018). Viability of the BIM manager enduring as a distinct role: association rule mining of job advertisements. J. Constr. Eng. Manag., 144, doi:10.1061/(asce)co.1943-7862.00015429).
Howard, R., Restrepo, L., and Chang, C. Y. (2017). Addressing individual perceptions: an application of the unified theory of acceptance and use of technology to building information modelling. Int. J. Proj. Manag., 35, 107, 120. doi:10.1016/j.ijproman.2016.10.0122).
Huh, S. H., Ham, N., Kim, J. H., and Kim, J. J. (2023). Quantitative impact analysis of priority policy applied to BIM-based design validation. Automation Constr., 154: 105031–105805. doi:10.1016/j.autcon.2023.105031
Ifenthaler, D., and Egloffstein, M. (2020). Development and implementation of a maturity model of digital transformation. TechTrends, 64, 302, 309. doi:10.1007/s11528-019-00457-42).
Ismail, A. S., Ali, K. N., Mustaffa, N. E., Iahad N, A., and Yusuf, B. Y. (2019). Enhancing the graduates’ employability and career development through building information modelling intensive training. Int. J. Built Environ. Sustain., 6(1–2): 91–99. doi:10.11113/ijbes.v6.n1-2.387
Jiang, Y. H. (2019). Influence of BIM staff experience on project performance: evidence from infrastructure projects. J. Constr. Eng. Manag., 145: 7.
Joseph, J. (2011). BIM titles and job descriptions: how do they fit in your organizational structure ?
Jung, Y., and Joo, M. (2011). Building information modelling (BIM) framework for practical implementation. Automation Constr., 20, 126, 133. doi:10.1016/j.autcon.2010.09.0102).
Kambur, E., and Akar, C. (2022). Human resource developments with the touch of artificial intelligence: a scale development study. Int. J. Manpow., 43, 168, 205. doi:10.1108/ijm-04-2021-02161).
Kekana, G., Aigbavboa, C., and Thwala, W. (2015). Understanding building information modelling in the South Africa construction industry. International organisation Technology and Management in Construction Conference, 12.
Khodeir, L. M., and Nessim, A. A. (2018). BIM2BEM integrated approach: examining status of the adoption of building information modelling and building energy models in Egyptian architectural firms. Ain Shams Eng. J., 9(4): 1781–1790. doi:10.1016/j.asej.2017.01.004
Khosrowshahi, F., and Arayici, Y. (2012). Roadmap for implementation of BIM in the UK construction industry. Eng. Constr. Archit. Manag., 19, 610, 635. doi:10.1108/096999812112775316).
Kim, H. S., Kim, S. K., and Kang, L. S. (2021). BIM performance assessment system using a K-means clustering algorithm. J. Asian Archit. Build. Eng., 20, 78, 87. doi:10.1080/13467581.2020.18004711).
Kim, S., Chin, S., Han, J., and Choi, C.-H. (2017). Measurement of construction BIM value based on a case study of a large-scale building project. J. Manag. Eng., 33(6): 6, doi:10.1061/(asce)me.1943-5479.0000551
Kim, S., Connerton, T. P., and Park, C. (2022). Transforming the automotive retail: drivers for customers’ omnichannel BOPS (buy online and pick up in store) behavior. J. Bus. Res., 139. doi:10.1016/j.jbusres.2021.09.070
Kreuger, L. W., and Allynand Bacon, N. W. L. (2006). Social work research methods: qualitative and quantitative applications[M]. Boston and New York: Pearson and Allyn Bacon.
Li, J. G. (2017). Investigating the impact of BIM team size on project performance: a case study of BIM-enabled construction projects. Automation Constr., 83: 1–9.
Liao, L., and Ai Lin, T. E. (2018). Organizational change perspective on people management in BIM implementation in building projects. J. Manag. Eng., 34, doi:10.1061/(asce)me.1943-5479.00006043).
Maharika, I. F., Irsan, A., Al, A. S. I., Susanto, A., Abma, V., and Yuriandala, Y. (2020). Building information modelling (BIM) adoption model for architectural education. J. Des. Built Environ., 20, 22, 42. doi:10.22452/jdbe.vol20no3.23).
Maina, P. W.University of Nairobi (2018). An investigation into the role of building information modelling in reducing cost and time overruns experienced in 2-dimensional based construction-a survey of construction practitioners in Nairobi[M]. Nairobi.
Marczyk, G. D. (2006). Essentials of research design and methodology[M]. Choice Rev. Online 43 (09).
Marikyan, D., and Papagiannidis, S. (2023). “The unified theory of acceptance and use of technology: a review[g]//PAPAGIANNIDIS S,” in TheoryHub book: unified theory of acceptance and use of technology, 16pg.
Menon, D., and Shilpa, K. (2023). “Chatting with ChatGPT”: analyzing the factors influencing users’ intention to Use the Open AI’s ChatGPT using the UTAUT model. Heliyon, 9, e20962, doi:10.1016/j.heliyon.2023.e2096211).
Merschbrock, C., and Erik Munkvold, B. (2012). A research review on building information modeling in construction-an area ripe for is research. Commun. Assoc. Inf. Syst., 31, doi:10.17705/1cais.031101).
Misra, S., Adtani, R., Singh, Y., Singh, S., and Thakkar, D. (2023). Exploring the factors affecting behavioral intention to adopt wearable devices. Clin. Epidemiol. Glob. Health, 24, 101428, doi:10.1016/j.cegh.2023.101428
Mosse, H. N., Njuguna, M., and Kabubo, C. (2020). Influence of building information modelling (BIM) on engineering contract management in Nairobi, Kenya. World J. Eng. Technol., 08, 329, 346. doi:10.4236/wjet.2020.8302603).
Mtya, A., and Windapo, A. (2019). Drivers and barriers to the adoption of building information modelling (BIM) by construction firms in South Africa[G]//Innovative Production and Construction: transforming Construction through Emerging Technologies.
Mwangi, K. W., Mainye, N., Ouso, D. O., Esoh, K., Muraya, A. W., Mwangi, C. K., et al. (2021). Open science in Kenya: where are we?. Front. Res. Metrics Anal., 6, 669675, doi:10.3389/frma.2021.669675
Mwero, J. N., and Bukachi, P. R. A.University of Nairobi (2019). Building information modelling adoption in structural design in Kenya - a case study of Nairobi. Int. J. Sci. Res. Publ. (IJSRP) 9 (6), p9046. doi:10.29322/ijsrp.9.06.2019.p9046
Neelamkavil, J., and Ahamed, S. (2012). “Ottawa National Research Council Canada,” in The return on investment from BIM-driven projects in construction[M]. National Research Council of Canada.
Nguyen, T. Q., Thieu-Thi, T. T., and Vu, N. N. (2021). BIM-based competitive advantages and competitive strategies for construction consultancy SMEs: a case study in vietnam. Int. J. Sustain. Constr. Eng. Technol., 12, doi:10.30880/ijscet.2021.12.03.0013).
Ojiaku, O. C., Ezenwafor, E. C., and Osarenkhoe, A. (2024). Integrating TTF and UTAUT models to illuminate factors that influence consumers’ intentions to adopt financial technologies in an emerging country context. Int. J. Technol. Mark., 18, 113, 135. doi:10.1504/ijtmkt.2024.1356741).
Okudan, O., Budayan, C., and Arayici, Y. (2022). Identification and prioritization of key performance indicators for the construction small and medium enterprises. Teknik Dergi/Technical J. Turkish Chamb. Civ. Eng., 33, 12635, 12662. doi:10.18400/tekderg.9778495).
Olanrewaju, O. I., Babarinde, S. A., Chileshe, N., and Sandanayake, M. (2021). Drivers for implementation of building information modeling (BIM) within the Nigerian construction industry. J. Financial Manag. Prop. Constr., 26, 366, 386. doi:10.1108/jfmpc-12-2019-00903).
Olanrewaju, O. I., Chileshe, N., Babarinde, S. A., and Sandanayake, M. (2020). Investigating the barriers to building information modeling (BIM) implementation within the Nigerian construction industry. Eng. Constr. Archit. Manag., 27(10): 2931–2958. doi:10.1108/ecam-01-2020-0042
Olanrewaju, O. I., Kineber, A. F., Chileshe, N., and Edwards, D. J. (2022). Modelling the relationship between Building Information Modelling (BIM) implementation barriers, usage and awareness on building project lifecycle. Build. Environ., 207. doi:10.1016/j.buildenv.2021.108556
Olatunji, O. A., Lee, J. J. S., Chong, H. Y., and Akanmu, A. A. (2021). Building information modelling (BIM) penetration in quantity surveying (QS) practice. Built Environ. Proj. Asset Manag., 11, 888, 902. doi:10.1108/bepam-08-2020-01405).
Oliveira, T., Faria, M., Thomas, M. A., and Popovič, A. (2014). Extending the understanding of mobile banking adoption: when UTAUT meets TTF and ITM. Int. J. Inf. Manag., 34, 689, 703. doi:10.1016/j.ijinfomgt.2014.06.0045).
Olofsson Hallén, K., Forsman, M., and Eriksson, A. (2023). Interactions between human, technology and organization in building information modelling (BIM) - a scoping review of critical factors for the individual user. Int. J. Industrial Ergonomics, 97: 103480–108141. doi:10.1016/j.ergon.2023.103480
Olugboyega, O., and Windapo, A. (2023). Modelling the indicators of a reduction in BIM adoption barriers in a developing country. Int. J. Constr. Manag., 23, 1581, 1591. doi:10.1080/15623599.2021.19881969).
Oraee, M., Hosseini, M. R., Edwards, D. J., Li, H., Papadonikolaki, E., and Cao, D. (2019). Collaboration barriers in BIM-based construction networks: a conceptual model. Int. J. Proj. Manag., 37, 839, 854. doi:10.1016/j.ijproman.2019.05.0046).
Orlikowski, W. J., and Iacono, S. (2001). Research commentary: desperately seeking the 《it》 in it research - a call to theorizing the it artifact. Inf. Syst. Res., 12: 121–134. doi:10.1287/isre.12.2.121.9700
Oyuga, J. O., Gwaya, A., and Njuguna, M. (2021). Kenyan government involvement on BIM and its effect on BIM adoption amongst building contractors East Afr. J. Eng., 3(1): 99–113. doi:10.37284/eaje.3.1.414
Pilehchian, B., Staub-French, S., and Nepal, M. P. (2015). A conceptual approach to track design changes within a multi-disciplinary building information modeling environment. Can. J. Civ. Eng., 42, 139, 152. doi:10.1139/cjce-2014-00782).
Ramanayaka, C. D. D., and Venkatachalam, S. (2015). Reflection on BIM development practices at the pre-maturity. Procedia Eng., 123: 462–470. doi:10.1016/j.proeng.2015.10.092
Rashidian, S., Drogemuller, R., and Omrani, S. (2022). The Compatibility of Existing Bim maturity models with lean Construction and Integrated project delivery. J. Inf. Technol. Constr., 27. doi:10.36680/j.itcon.2022.024
Rashidian, S., Drogemuller, R., and Omrani, S. (2023). Building information modelling, integrated project delivery, and lean construction maturity attributes: a delphi study. Buildings, 13, 281, doi:10.3390/buildings130202812).
Ratajczak, J., Riedl, M., and Matt, D. T. (2019). BIM-based and AR application combined with location-based management system for the improvement of the construction performance. Buildings, 9, 118, doi:10.3390/buildings90501185).
Raza, M. S., Tayeh, B. A., Abu Aisheh, Y. I., and Maglad, A. M. (2023). Potential features of building information modeling (BIM) for application of project management knowledge areas in the construction industry. Heliyon, 9(9): e19697, doi:10.1016/j.heliyon.2023.e19697
Rouidi, M., Elouadi, A. E., Hamdoune, A., Choujtani, K., and Chati, A. (2022). TAM-UTAUT and the acceptance of remote healthcare technologies by healthcare professionals: a systematic review. Inf. Med. Unlocked, 32, 101008, doi:10.1016/j.imu.2022.101008
Saka, A. B., Chan, D. W. M., and Siu, F. M. F. (2020). Drivers of sustainable adoption of building information modelling (BIM) in the nigerian construction small and medium-sized enterprises (SMEs). Sustain. Switz., 12, 3710, doi:10.3390/su120937109).
Sarstedt, M., Ringle, C. M., and Hair, J. F. (2021). Partial least squares structural equation modeling. Handb. Mark. Res., 7(3): 587–632. doi:10.1007/978-3-319-57413-4_15
Saunders, M. A., Lewis, P., and Thornhill, A.Pearson education (2012). Research methods for business students sixth edition research methods for business students[M]. Research Methods for Business Students. United Kingdom: Pearson education.
Semaan, J., Underwood, J., and Hyde, J. (2021). An investigation of work-based education and training needs for effective BIM adoption and implementation: an organisational upskilling model. Appl. Sci., 11(18): 8646, doi:10.3390/app11188646
Shmueli, G., Ray, S., Velasquez Estrada, J. M., and Chatla, S. B. (2016). The elephant in the room: predictive performance of PLS models. J. Bus. Res., 69, 4552, 4564. doi:10.1016/j.jbusres.2016.03.04910).
Sloot, R. N. F., Heutink, A., and Voordijk, J. T. (2019). Assessing usefulness of 4D BIM tools in risk mitigation strategies. Automation Constr., 106. doi:10.1016/j.autcon.2019.102881
Stemn, E., Bofinger, C., Cliff, D., and Hassall, M. E. (2019). Examining the relationship between safety culture maturity and safety performance of the mining industry. Saf. Sci., 113: 345–355. doi:10.1016/j.ssci.2018.12.008
Succar, B. S. (2015) Measures for assessing BIM performance in the construction phase: a literature review. Automation Constr., 59: 109–127.
Taib, M., Quanhua, H., and Taib, N. (2023). Building information modelling (BIM) adoption for cost engineering consultant; case study of southern China. J. Adv. Res. Appl. Sci. Eng. Technol., 29, 21, 36. doi:10.37934/araset.29.3.21363).
Takyi-Annan, G. E., and Zhang, H. (2023). Assessing the impact of overcoming BIM implementation barriers on BIM usage frequency and circular economy in the project lifecycle using Partial least Squares structural Equation modelling (PLS-SEM) analysis. Energy Build., 295: 113329–117788. doi:10.1016/j.enbuild.2023.113329
Tsai, M. H., Kang, S. C., and Hsieh, S. H. (2014). Lessons learnt from customization of a BIM tool for a design-build company. J. Chin. Inst. Eng. Trans. Chin. Inst. Engineers,Series A, 37, 189, 199. doi:10.1080/02533839.2013.7817912).
Uddin, M. A., Alam, M. S., Mamun, A. A. L., Khan, T. U. Z., and Akter, A. (2019). A study of the adoption and implementation of enterprise resource planning (ERP): identification of moderators and mediator. J. Open Innovation Technol. Mark. Complex., 6, 2, doi:10.3390/joitmc60100021).
UNECE. United Nations (2021). Building Information Modelling (BIM) for road infrastructure: TEM requirements and recommendations. Geneva.
Van Tam, N., Quoc Toan, N., Phong, V. V. A. N., and Durdyev, S. (2023). Impact of BIM-related factors affecting construction project performance. Int. J. Build. Pathology Adapt., 41, 454, 475. doi:10.1108/ijbpa-05-2021-00682).
Venkatesh, V., and Davis, F. D. (2000). A theoretical extension of the technology acceptance model: four longitudinal field studies. Manag. Sci., 46(2): 186–204. doi:10.1287/mnsc.46.2.186.11926
Venkatesh, V., Morris, M. G., Davis, G. B., and Davis, F. D. (2003). User acceptance of information technology: toward a unified view. MIS Q. Manag. Inf. Syst., 27(3): 425–478. doi:10.2307/30036540
Venkatesh, V., Thong, J. Y. L., and Xu, X. (2012). Consumer acceptance and use of information technology: extending the unified theory of acceptance and use of technology. MIS Q. Manag. Inf. Syst., 36(1): 157–178. doi:10.2307/41410412
Wang, K., Zhang, C., Guo, F., and Guo, S. (2022a). Toward an efficient construction process: what drives BIM professionals to collaborate in BIM-enabled projects. J. Manag. Eng., 38, doi:10.1061/(asce)me.1943-5479.00010564).
Wang, S. L., and Lin, H. I. (2019). Integrating TTF and IDT to evaluate user intention of big data analytics in mobile cloud healthcare system. Behav. Inf. Technol., 38, 974, 985. doi:10.1080/0144929x.2019.16264869).
Wang, X., French, B. F., and Clay, P. F. (2015). Convergent and discriminant validity with formative measurement: a mediator perspective. J. Mod. Appl. Stat. Methods, 14, 83, 106. doi:10.22237/jmasm/14304534001).
Wang, Y. X. (2020). Investigating the influence of BIM staff experience on project performance: a case study of construction projects. Automation Constr., 113.
Wang, Z., Liu, Z., and Liu, J. (2022b). Innovation strategy or policy pressure? The motivations of BIM adoption in China’s AEC enterprises. J. Asian Archit. Build. Eng., 21, 1578, 1589. doi:10.1080/13467581.2021.19292444).
Xue, X., Sun, X., Xue, W., Wang, Y., and Liao, L. (2023). Investigating building information modeling acceptance in the Chinese AECO industry. Eng. Constr. Archit. Manag., 30, 4253, 4270. doi:10.1108/ecam-08-2021-06859).
Yap, J. B. H., Lam, C. G. Y., Skitmore, M., and Talebian, N. (2022). Barriers to the adoption of new safety technologies in construction: a developing country context. J. Civ. Eng. Manag., 28, 120, 133. doi:10.3846/jcem.2022.160142).
Zhang, J., Wu, W., and Li, H. (2018). Enhancing building information modeling competency among civil engineering and management students with team-based learning. J. Prof. Issues Eng. Educ. Pract., 144, doi:10.1061/(asce)ei.1943-5541.00003562).
Zhao, Y., Sun, Y., Zhou, Q., Cui, C., and Liu, Y. (2023). How A/E/C professionals accept BIM technologies in China: a technology acceptance model perspective. Eng. Constr. Archit. Manag., 30, 4569, 4589. doi:10.1108/ecam-04-2022-030810).
Zhou, T., Lu, Y., and Wang, B. (2010). Integrating TTF and UTAUT to explain mobile banking user adoption. Comput. Hum. Behav., 26(4): 760–767. doi:10.1016/j.chb.2010.01.013
Keywords: building information modelling (BIM) staff, construction project performance, unified theory of acceptance and use of technology (UTAUT), task-technology fit (TTF), BIM adoption, project management, SMART PLS-SEM
Citation: Chen Y, Gacheri HF and Sun G (2024) Empirical investigation of building information modelling (BIM) staff’s impact on construction project performance: evidence in Kenya. Front. Built Environ. 10:1441604. doi: 10.3389/fbuil.2024.1441604
Received: 31 May 2024; Accepted: 19 August 2024;
Published: 29 August 2024.
Edited by:
Hongjiang Yao, Shandong University, ChinaReviewed by:
Muneera Esa, University of Science Malaysia (USM), MalaysiaBingxin Zhang, Henan Finance University, China
Copyright © 2024 Chen, Gacheri and Sun. This is an open-access article distributed under the terms of the Creative Commons Attribution License (CC BY). The use, distribution or reproduction in other forums is permitted, provided the original author(s) and the copyright owner(s) are credited and that the original publication in this journal is cited, in accordance with accepted academic practice. No use, distribution or reproduction is permitted which does not comply with these terms.
*Correspondence: Hazel Faith Gacheri, Z2FjaGVyaWhhemVsQGdtYWlsLmNvbQ==; Yuan Chen, Y2hlbl95dWFuQHp6dS5lZHUuY24=
†ORCID: Yuan Chen, orcid.org/0000-0003-4917-3386