- Interior Design Department, Middle East University, Amman, Jordan
Introduction: This article investigates the revolutionary influence of artificial intelligence (AI) on interior design, with an emphasis on the incorporation of machine learning ML techniques. The advent of AI has resulted in a paradigm change in design methods, prompting a thorough review of research gaps and the potential for ML applications in a variety of areas of interior design.
Methods: A systematic review process was implemented to fill these gaps, consisting of an in-depth evaluation of 28 research publications from Scopus databases categorized into eight themes. The investigation sought to address a pair of primary inquiries: what opportunities exist for using ML in interior design conditions, and what challenges limit its effective implementation.
Result: The study discovered a significant gap in the existing literature, demanding a full assessment to highlight challenges in ML implementation and the potential for applied ML development throughout the whole spectrum of interior design.
Discussion: The findings are intended to provide researchers and enthusiasts with an extensive understanding of ML-based gaps in interior design conditions and to provide various solutions for filling these gaps. This understanding may assist in the development of intelligent ML-driven apps, promoting interior contexts that improve user well-being and psychological comfort.
1 Introduction
Interior design, an encompassing field that combines creative innovation, architectural principles, and utilitarian imperatives, constantly relies on designer evaluations and intuition. However, the intricate nature of interior design has increased in response to rising requirements of resource efficiency, personalization, and environmental sustainability. Machine learning technologies can play. A significant role in enhancing efficiency and sustainability. For resource management optimization, and environmental sustainability (Arabasy et al., 2024). In this transformational context, the emergence of Artificial Intelligence (AI), an aspect of Machine Learning (ML), has provided a compelling paradigm change (Edla et al., 2018). Significantly, in the twenty-first-century environment, ML and interior design integration has emerged as a revolutionary force (Goodfellow et al., 2016) providing unique opportunities to redefine the idea, construction, and utilization of interior spaces. ML appears as a component of AI encompassing algorithms and computational models that permit autonomous learning from data, pattern recognition, and predictive decision-making processes, despite the need for explicit programming (Sepasgozar et al., 2020) Its domain applications span a wide range of industries including healthcare, finance, transportation, and interior design.
Incorporating ML techniques into interior design practices has ushered in an era of creativity, efficiency, and customization. The varied contribution of ML to interior design is highlighted by its proficiency in space optimization, individualized design creation, eco-conscious material selection, exact cost calculation, forecasting developments, immersive visualization, and sustainability enhancement (Sepasgozar et al., 2019). Interior designers possess the ability to construct places that captivate not exclusively aesthetically but also function with efficiency, sensitivity regarding the environment, and customized adaptation to the specific tastes and desires of occupants through the analytical capability inherent in ML algorithms.
At the fundamental level, smart technology enables individuals to switch electronic devices on and off, and researchers in additional areas such as medical services or architecture utilize a range of actuators and sensors for a range of objectives. However, there is a need to learn from diverse techniques and combine them to use the same system, while also analyzing data obtained from devices used for different interior environment management. With regard to the context of the rapid expansion of ML applications in interior design, it is necessary to initiate an extensive review of existing research, assess the present state-of-the-art, and evaluate the technical capacities at work in the sector. In this context (Ringel et al., 2019), demonstrated how an intelligent residence can assist in achieving energy conservation goals while improving occupant wellbeing. Building automation techniques and intelligent residence assistance systems can improve users’ quality of life, while also allowing AI to evaluate their behavior (Nguyen and Aiello, 2013). The system can employ behavioral analysis to forecast user demand and optimize the utilization of technologies and resources (Tao et al., 2018).
According to (Gonçalves et al., 2019) the management of the user’s perspective or demand estimate is crucial and challenging, and consequently, must be handled using smart interior environments and smart grid technologies. A viable solution is the combination of algorithms and source optimization, which will contribute to the development of an efficient interior space supervision system. Smart technology has recently grown rapidly, and new applications are being investigated and supplied by academics and practitioners. According to reports, there will be a substantial increase in the number of wirelessly linked technological devices (Silverio-Fernández et al., 2018). As a result, there is a pressing need to examine prior efforts, analyze the latest research and technological possibilities, and evaluate the effectiveness of their implementation.
Despite the growing interest in ML in interior design, this study is unique due to how it conducted an exhaustive investigation of the obstacles and possibilities associated with using machine learning (ML) approaches through all aspects of interior design. The existing literature frequently lacks an in-depth investigation of particular ML-based uses that involve inner-built environment efficiency, customized design development, eco-conscious selection of materials, accurate estimation of costs, forecasting of trends, deep visualization, and sustainability improvement in interior design contexts. This research aims to fill this gap and provide a more nuanced knowledge of these challenges and possibilities, paving the way for the creation of tailored ML strategies that meet the diverse and developing demands of current interior design practices.
2 Methodology and logic
Wrigh (2020) demonstrated to identify the missing segments in any study or program analysis by literature review gap analysis to achieve our aims, a systematic review was conducted to identify journal papers addressing. Scopus database was chosen because of the ease of discovery of peer-reviewed research, and the proper development. The chronological sequence to review the literature from March 1991 to September 2023, 48 studies have been dedicated. 27 studies were employed for inclusion in the current study based on publications in peer-reviewed journals related to machine learning applications in interior design, with the provision of experimental data or detailed cases. Studies that concentrated on theoretical elements and generic artificial intelligence applications were eliminated. Without regard for internal design, duplicate research, or research presented at conferences, to ensure the selection of high-quality studies that contribute to creating an excellent basis for analysis and objective results, The search workflow is depicted in Figure 1. The following query string is used “TITLE-ABS-KEY ((“interior design” AND ( “machine learning” OR “ml”)))”.
After the collection of related articles, we conducted a scientometric analysis to measure the progress of the scientific production of ML in interior design (Mooghali et al., 2011). Furthermore, in this study, the “VOSviewer” software (Van Eck and Waltman, 2010) was applied to create and show bibliometric networks based on the evaluated literature. We map the relationships between the applications of ML in interior design and the density of each application. We also identified the main theme of each article.
All of the following bibliometric variables were analyzed: article title, relevant journal name, SJR rank, period of publication, name of the writer, number of citations, and reference keywords. Figure 1 provides a general overview of the work done in this study. Subsequently, the research implemented gap analysis by first, identifying the main themes through the techniques of scanning and skimming to sort the relevant articles (Machi and McEvoy, 2016). Then, all studies were critically analyzed to highlight the aim of the study and method limitations.
2.1 Scientometric analysis
The progress of the scientific production in the applications of ML in interior design is shown in Figure 1. As shown in Figure 2 it can be seen that the first paper on the application of ML in interior design was published in 2006. However, it started to increase steadily increasing from 2021. This is an indication of the significant attention that machine learning applications with interior design received over the last 3 years.
VOSviewer has strong features for discovering links between concepts and displaying complicated networks in a straightforward easy-to-understand format. The program was used to assess the relationships between various articles and important concepts, and the findings are shown in Figures 3, 4. Figure 3 shows the network analysis of (m = 45) papers, it can be seen from Figure 4 that there are specific usages of integrating machine learning interior design, namely, interiors of buildings, interior design and furnishing, color psychology, lighting in interior Environment, Human Behaviour Wellbeing and safety of the structure. Figure 4 presents the density of the mentioned applications of analyzing (n = 45) papers, it is obvious that machine learning technology is highly used in integration with FRP applications to provide a wide range of workable solutions.
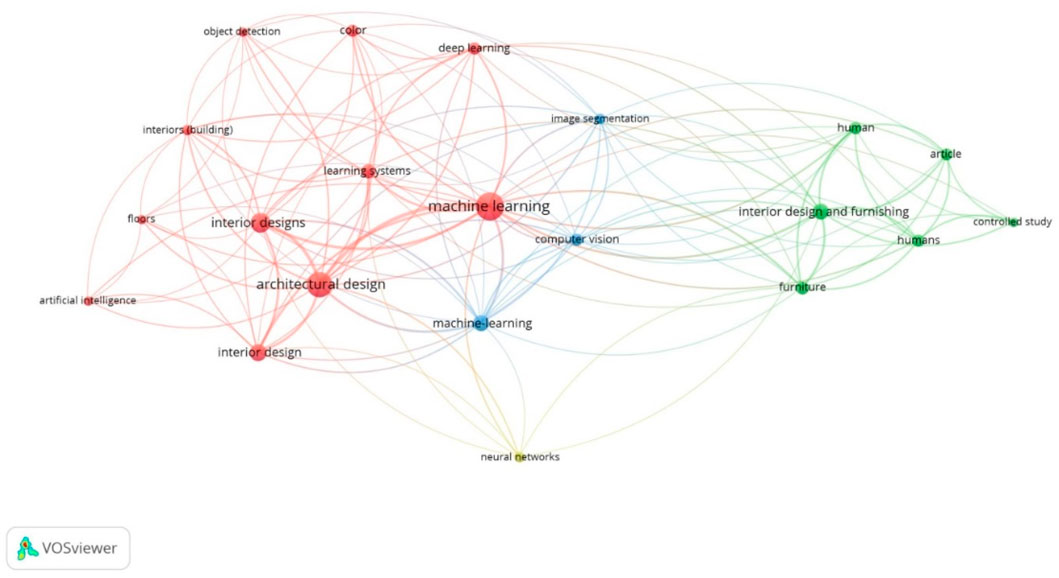
Figure 3. Network analysis of ML applications in interior design. This figure was created using VOSviewer software (Van Eck and Waltman, 2010).
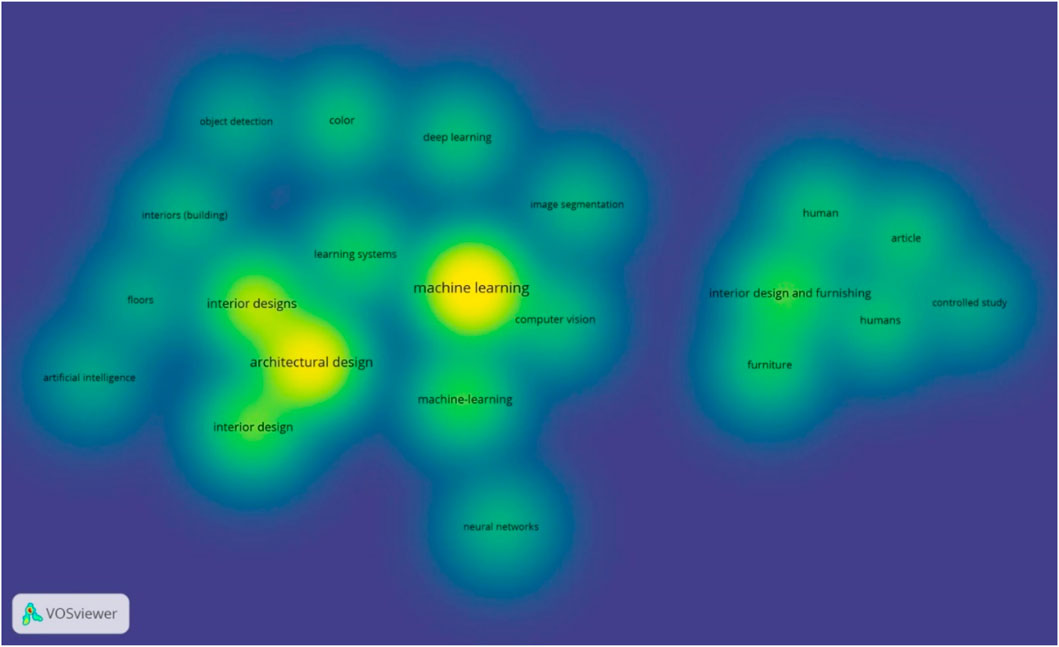
Figure 4. Density analysis of ML applications in interior design. This figure was created using VOSviewer software (Van Eck and Waltman, 2010).
3 Application of machine learning in interior design
Table 1 includes the major research on object and information-detection-based machine learning. Articles were classified into eight main categories, namely, Color Psychology, Interior Environment and Human Behavior Wellbeing, Medical Interior Environment, Residential Design, Lighting in Interior Design, Furniture Factor, Models Measured and Analyze Data, Rendering Image, and Interior Structural Elements. Figures 5, 6 shows that the majority of articles about object detection focus on using machine learning to analyze interior layouts, inspecting the accuracy of spatial arrangements, especially for large furniture elements, and detecting detailed design aspects in medical and residential spaces. The bulk of articles on information detection focus on capturing data on occupant behaviors and equipment usage within interior spaces during everyday interactions.
3.1 Color psychology
Color plays a fundamental role in interior design, not only for providing aesthetic value to spaces but also as a crucial element in maintaining psychological equilibrium for the wellbeing of space users. It serves as a central axis in the field of visual perception, and colors act as potent emotional stimuli. This highlights the psychology of color, a scientific discipline that explores the ability of color to evoke feelings of excitement, tranquility, or mystery, among others. In addition, it encompasses aspects of individual aesthetic preferences in interior spaces, going beyond its connection to the reception of electromagnetic waves by the human brain and significantly influencing human behavior (Jain and Nayak, 2023) Color psychology has been a hotspot in interior design for decades. To improve the performance of the inner spaces, two studies have proposed the use of ML to enhance color psychology and interaction with the users based on pictures and solutions for color detection systems in interior design, (Yu and Egger, 2021). Applied the analysis of sequential images and data given pictures with various highlights utilizing ML approaches which uncovered the connection between color and users and gave rules to advertisers to upgrade the travel industry exercises through moving from aesthetic philosophy to the field of color psychology limitation. Another study by (Dewingong et al., 2022) used ML methods to improve color solutions and interior design operations. This study proposes a method for teaching color detection systems in interior design to recognize colors in the RGB space and predict their names and values using machine learning. OpenCV, an open-source computer vision system, was used for image handling and variety identification. However, the limitations of applications that interact with users through Instagram social media will result in an incomplete depiction of the intricate nature of real-world architectural events and may limit the scope of the study.
3.2 Interior environment and human behaviour wellbeing
Several studies have been undertaken in the field of ML research to investigate various aspects of the built environment. ML has been used in five studies of the built environment and its interaction with human behavior in interior design (Deng and Chen, 2021). The study expanded into the field of indoor behavior adjustment, concentrating on thermo-stats and clothing level changes. For this objective, they built a policy-based reinforcement learning (RL) model and used Q-learning as the training mechanism in MATLAB. Even though they successfully verified their RL model using acquired data, it is crucial to highlight that their research was limited to this specific RL technique and the use of transfer learning within workplace and residential buildings. Moreover (Abdel-Razek et al., 2022), took on the endeavor of room utilization identification, intending to improve accuracy by considering various environmental parameters such as light, CO2, and humidity. Their research included the use of ML ability to interpret approaches such as K-nearest neighbors (KNN), hybrid Adam optimizer-artificial neural network-back-propagation network (AO-ANN (BP)), and decision trees (DT). However, it is important to note that their study was mostly limited to classification models and focused only on indoor environmental quality in relation to energy efficiency. For (Kim, 2021). The study had a distinct focus, concentrating on understanding the relationships between affordances and interior elements within the built environment from the perspective of users. To achieve this, they conducted an ethnographic case study, relying on unobtrusive observations through various multimedia methods, including video recordings, voice recordings, and photographs. However, the study’s limitation lies in its exclusive reliance on qualitative ethnographic data, neglecting quantitative aspects and alternative analytical methods. Consequently (Winter et al., 2022), investigated the implementation of lightweight ML models for human posture valuation and gesticulate categorization through the lens of interactive interior design predictions. The main focus of the study was to improve the involvement of visitors. An empirical evaluation of lightweight ML models is included in the research. Nonetheless, it is crucial to note that the research was limited to the specific use of these models at museums and neglected to investigate possibly additional ML approaches or more general uses. Finally (Bouazizi et al., 2023), study monitored the behaviors of older adults living alone, with a special focus on identifying dangerous incidents, such as falls. The study suggests a unique approach: putting a 2D LIDAR onto a robotic vacuum, using its mobility to constantly gather distance information and identify humans on the ground after a fall, even after a certain amount of time has passed. A convolutional long short-term memory (LSTM) neural network is used to process, interpolate, and evaluate the acquired data. Simulations show high accuracy rates of 81.2% and 99%, outperforming traditional static LIDAR systems (69.4% and 88.6%, respectively). However, the limitations are centered on simulations, and practical problem deployment may provide practical issues that were not anticipated, necessitating additional validation for actual implementation.
3.3 Medical interior environment
The irrefutable truth of global warming has far-reaching consequences for the healthcare sector, which both contributes to and is affected by environmental issues. Most scientific investigations focus on the importance of understanding architecturally justified techniques for lighting internal spaces in healthcare facilities, with extra attention on greenhouse executives, the use of sustainable interior components, and landscaping. According to studies, healthcare facilities, due to their resource-intensive nature, require significant amounts of electricity, water, and building materials to provide the highest level of care. Additionally, the implementation of green measures in healthcare facilities has the potential to drastically reduce their environmental effect while improving the wellbeing of healthcare employees and encouraging patient recovery (Danilov et al., 2020), in the context, of two separate studies investigated the influence of design features in healthcare environments on patient wellbeing in the field of scientific investigation (Jung et al., 2023). investigated biophilic design by examining its impacts in a virtual reality (VR) hospital patient room and its impact on emotional and brain responses. Their research involved a comprehensive examination of interactive emotions using electroencephalogram (EEG) and virtual reality (VR) technologies. They employed ML and statistical analysis to distinguish emotional changes caused by biophilic design, with a specific focus on lowering tension in medical environments. Their work, however, has several limitations, such as the controlled experimental environment, which does not always entirely reflect practical applications in medical situations. In a related vein (Schreuder et al., 2016), focused on the effect of design features (DCs) within patient rooms on self-reported patient wellbeing. Their study included measuring the interior and exterior characteristics of patient rooms across several medical facilities, as well as patient ratings of room design and its relevance to their wellbeing. They attempted to create connections between the physical elements of patient rooms and patient wellbeing using ML techniques and regression analysis. However, their study has limitations. It relies on self-reported ratings, which may be sensitive and affected depending on individual perspectives.
3.4 Residential design
Mengyao and Yu, (2023) Use ML techniques to conduct an investigation centered on art design. Their primary goal is to simulate an intelligent placement method that will match the actual categorization outcomes from samples tested with network values for output while maintaining accuracy standards. Nonetheless, potential limitations involve the dataset’s availability and representativeness for the development and evaluation of the algorithm. Furthermore (He et al., 2023), investigate online booking systems, with a focus on the context of pictures provided in search results. Their research looks at how visual characteristics of interior aspects like the bedroom, living room, and interior aesthetic features and additionally the clarity, illumination, and contrast of background photos impact booking rates during a 16-night end-of-year holiday period. Their strategy entails the creation of a complete model that encompasses visual data, property features, and host traits. They also address endogeneity problems by calculating propensity scores and constructing instrumental factors in two marketplaces (New York City and San Francisco). The study may, however, have limits in terms of the ability to generalize its outcomes to other historical periods or geographical areas, as the applicable case (New York City and San Francisco). Nevertheless (Zhao et al., 2022), in their publication present Home-Monitor, a device event monitoring system. During training, their major concentration is regarding the unique identification of device activities utilizing network packet size and direction. They want to see how successful the system is in monitoring and analyzing device events. The study’s limitations might include possible difficulties in dealing with varied network settings and the necessity for practical application validation to determine Home-Monitor’s practical value and accuracy.
3.5 Lighting in interior design
The application of ML plays an essential role in interior design lighting due to the optimization of illuminating schemes following consumer preferences, time of day, and daylight factors. ML algorithms can adapt and optimize lighting systems to increase the effectiveness of energy and generate a personalized atmosphere, integrating a combination of aesthetics and functionality in interior environments (Chen et al., 2023). ML has been used in two studies of lighting in interior design (Cadusch et al., 2019), The study concentrated on the experimenting verification of an improved nanostructured silicon-based photodetector machine with adjustable sensitivity via geometric parameter modifications. The study effectively utilized “fishnet pixels” to build a mini spectrometer chip and proved the reconstructed version of four trial spectra employing a two-stage supervised machine-learning-based reconstruction technique. Furthermore, the study developed a novel doping profile featuring vertically stacked, consecutively photodiode areas, thereby increasing the variety of detectors in a given on-chip footprint. Nevertheless, the limitation of the application of this unique design outside the individual experiments shown could potentially be restricted, especially in handling contemporary difficulties (Parsaee et al., 2022). study, on the other hand, demonstrated the compilation of eight massive imaging datasets totaling around 12,000 photos organized into 1,220 sets. The data were painstakingly recorded within a model of architecture to investigate the effect of shade panels on photo-biological illumination parameters. The studies featured varied shade panel configurations in an artificial sky room under a variety of outdoor overcast daylighting circumstances, comprising different color temperatures and levels of light. These datasets, which comprise bracketed low dynamic range (LDR) photos, allow for the creation of high dynamic range (HDR) photographs for photobiological lighting assessments. The study’s limitations, however, stem from the supervised experimentation environment within an architectural model, resulting in probably not completely depicting the intricate nature of real-world architectural events and may limit the study’s scope.
3.6 Furniture factor
In the realm of ML implementations in furniture factor (Tautkute et al., 2019). presented Deep-Style, a novel multimodal search engine designed to address the challenge of retrieving items from a multimedia database based on aesthetic similarity to the query, this approach cleverly used visual and linguistic indications to aid retrieval, notably in the sector of fashion products, which includes apparel and furnishings. This study, while intriguing, has limitations. Deep-Style’s capacity to function successfully in real-world circumstances may be hampered by a lack of broad and thorough datasets for training. Furthermore, the study’s focus on fashion merchandise retrieval raises concerns about its applicability to other fields, and a full investigation of the evaluation measures used is required. Moreover, a study by (Ştefan et al., 2019) conducted in the field of Augmented Reality (AR) and AI combination, to improve AR processes using ML techniques. The study introduces “FURNITURE,” an intelligent AR mobile application that capitalizes on AI and AR cooperation to create a class of applications for AR with two pivotal functionalities: (a) using an ML-trained model to evaluate the originality of images of the furniture, categorizing them into specified styles, and (b) enhancing furniture pieces under actual conditions in a historical context and a representative 3D model corresponding to their originality style. However, the research limitation highlights the idea of the virtual reality application and its capability advantages; however, its actual performance and effectiveness may be altered by practical problems and adoption among users.
In an equivalent vein (Zhao and Hao, 2021). Embarked on a robotics research project in order to solve prevalent challenges plaguing table tennis robot systems. To improve the system’s precision and efficiency, they developed a target trajectory tracking algorithm that used machine vision and the Scaled Conjugate Gradient (SCG) approach. The evidence of their research was using tracking algorithm characteristics as input data for a deep neural network, followed by normalization, to create a robust deep neural network algorithm model. Nonetheless, there are several limitations to the study. The algorithm’s performance is dependent on the amount and variety of the training dataset, which may restrict its generalizability to other techniques and circumstances, the study also lacks extensive real-world testing and validation in a variety of conditions.
Racec et al. (2016) explored the challenges of computer-based optimization in design, highlighting computational intelligence within a wider architectural context and interior design. Despite its value, the fundamental limitation of the suggested commercial solution for autonomous furniture arrangement is the lack of specific details regarding its execution. Moreover (Park et al., 2021), underlined the importance of furniture and color combinations in home design. They provided a data-driven strategy that uses ML along with network analysis to analyze 14,111 interior design in-stances from an Internet-based platform. However, this study is largely concerned with pattern recognition and requires practical applicability. Along with context, a study by (Nishikawa et al., 2019) introduces a revolutionary search engine that proposes customized furniture pairings to customers’ tastes based on picture attributes. The suggested technique analyzes user-selected photos to generate a rule-based combination of furniture pieces adapted to individual tastes, taking form and color into account. The implementation in reality and user satisfaction with the suggested system, on the other hand, limitations are susceptible to conditions in the real world and require further refining to improve its performance.
Finally (Tanasra et al., 2023), uses ML to automate and improve interior design processes. They generated furniture layouts using conditional generative adversarial networks and a collection of designated floor plans. Furthermore, they presented assessment criteria that integrate architectural design principles with computer vision features. While the study effectively exhibits architectural standards adherence and highlights BicycleGAN as the best-performing model, the limitation lacks insight into the complexities of incorporating their approach into practical application design workflows.
3.7 Models measured and analyzed data and rendered images
Mura et al. (2021) provide Walk2Map, a unique data integration technique that concentrates on modeling data such as RGB-D pictures and 3D point clouds. Their research comprises a full quantitative and qualitative assessment involving generated paths using scanned interior models as practical application paths and a comparison to a base translating image into images approach. These research investigations demonstrate the technique’s potential in reliably retrieving floor layouts from limited walk trajectory data. However, due to different interiors according to the diversity of built environmental circumstances, limits may occur in implementations that exist. Furthermore, (Huiskes and Pauwels, 2005). Presents an extensive examination of the image-regaining process, focusing on global and local features based on picture subdivisions in interior design. They also discuss the usage of MPEG-7 standard metadata and interfaces and learning algorithms for appropriate feedback, intending to improve user interactions with the outcome of queries. The study’s limitations may stem from the generalization of findings across various picture retrieval contexts. Additionally, (Liu, 2023). Proposes an experimental machine learning-based technique for interior design color transfer which employs the K-means algorithm for dealing with unequal brightness areas in prioritize photos. However, the limitations are the algorithm’s flexibility to different design configurations and reliance on original image quality are significant drawbacks. According to (ZHENG et al., 2020), the major of the study is to leverage the potential of Generative Adversarial Networks (GANs) to change the development of architectural plan drawings. The aim was to allow designers to automatically develop precise elements of apartment floor layouts within defined constraints. To accomplish this, an ML framework is used, which learns from picture pairs representing both the borders and full-plan drawings. The learning algorithm creates a model that understands the complex links between the image pairs. Subsequently, the assessment program applies these data to create architectural designs based on boundary photos supplied by the user. However, given the broad and multidimensional character of such plans, study limitations may encounter issues in correctly portraying the intricacy and depth of architectural design.
The major goal of the (Pejic and Pejic, 2022). Study is to pioneer a revolutionary technique for linear kitchen layout design that uses machine-learning algorithms to derive insights from current layouts. This article introduces the Procedural Kitchen Generation (PKG) model, which is a complex pipeline comprising 6 ML classifiers. The aforementioned classifiers underwent training and validation on a dataset meticulously selected by interior designers, with an emphasis on kitchen layouts. The ultimate objective is to allow for the automatic creation of kitchen layout proposals based on the amount of knowledge gained from previous designs. Furthermore, the PKG model is smoothly linked to the Unity Engine, making the automatic improvement and display of 3D kitchen designs possible. However, the limitations of the study are the success of dependent on the quality and variety of the training dataset as well as the inherent limits of any ML-based method in completely capturing the nuances of individual design preferences in kitchen layouts. Along with context, (Terrier and Martin, 2020). Study investigates the possibilities of virtual environments (VE) that use virtual reality (VR) to improve co-design processes by creating 3D prototypes within the VE. The goal of the research addresses populating realistic mock-ups, especially in the early phases of the process of design when common items are employed instead of variants. The study suggests a retrieval approach based on 2D drawing within the VR environment, augmented by ML capabilities, to increase user experience and interactivity. This novel technique recognizes 90 item types essential to VR interior design, achieving an excellent performance rate of up to 86% in the initial evaluation. However, study limitations and user-specific issues may emerge during real-world implementation, demanding more study and improvement. Moreover (Zhang and Ban, 2022). The study analyzes the changing role of interior design in modern culture. Using advances in computer vision and (AI), the focus of the study is to automate aesthetic judgments of interior design, supporting users in picking acceptable and effective interior design solutions from gathered photos or digital devices. The limits of this automated aesthetic evaluation method are potentially related to subjective preferences and cultural subtleties, which may not always be adequately reflected by algorithms.
Finally (Wang et al., 2023), provide critical assessments of representational techniques in five interrelated areas of interior design. They highlight advantages and weaknesses, although limitations may include the possibility of bias in method selection and the depiction of customized interior design (PID) solely from a technology standpoint. The results add to the growing environment of interior design and data analysis by navigating the constraints of their approaches.
3.8 Interior structural elements
Deng, (2021) Proposes research in the domain of structural safety evaluation that focuses on improving the accuracy of evaluating interior load-bearing structures. The research presents an innovative method for obtaining stress distribution features specific to interior load-bearing structures. For this, a hybrid methodology combining genetic algorithms and ML techniques is implemented to locate and evaluate stress patterns within these architectures. While this novel technique has the potential to enhance safety evaluations, it is critical to recognize possible limitations concerning the requirement for an adequate dataset for ML and the generalizability of results to a larger variety of architectural configurations.
4 Discussions
This article investigates the incorporation of ML into several elements of interior design in this study, resulting in the potential to revolutionize the sector. We intend to present a thorough review of the state of the art and current developments in ML applications in interior design. The discussion is divided into categories depending on the various components of interior design inspired by ML approaches.
Color Psychology: Color psychology is important in interior design because it influences not only the visual appeal but also the psychological state of the inhabitants. By evaluating photos and their emotional influence on users, ML has been applied to improve color psychology. Many of these studies, however, have been constrained by their focus on controlled conditions of experimentation or specialized applications, which may not adequately depict the complexities of real-world interior design circumstances. More study is needed to confirm these ML-driven color psychology advances’ practical relevance.
Human Behavior and the Interior-Environment Wellbeing: ML has been used to investigate human behavior and wellbeing in interior environments. Various machine-learning algorithms have been applied to investigate parameters such as the quality of indoor environments, room use, and user behavior. While these studies have yielded useful information, they frequently have drawbacks such as focusing on certain elements or depending on categorization methods. Future research should seek to address these limitations by pursuing more comprehensive and multidisciplinary methods to comprehend the complex interaction between interior environments as well as human wellbeing.
Lighting in Interior Design: ML has been used to improve lighting techniques for interior design by taking customer preferences, the moment during the day, and daylight considerations into account. Many of these examinations, however, are restricted to specific purposes or experimental conditions. More studies should be conducted to extend these findings to real-world circumstances and to investigate the scalability of ML-driven illumination optimization in a larger context.
Furniture Factor: ML has been used to improve several areas of furniture planning and selection. These applications include fashion product retrieval systems and the use of augmented reality integration for furnishings visualization. However, this research frequently confronts difficulties with dataset quality and the ability for generalization. Future research should concentrate on extending datasets and enhancing the actual usability of these machine learning-driven solutions to suit a broader range of design requirements and settings.
Medical Interior Environment: The impact of ML on healthcare interior design, particularly biophilic design and its impacts on patient wellbeing, has been investigated. Though these studies have yielded insightful knowledge, they are frequently constrained by restricted experimental conditions. Future studies should try to confirm these findings in real-world healthcare environments and consider a larger variety of environmental factors that impact patient wellbeing.
Models Measured and Analyzed Data and Rendered Pictures: ML approaches have been used to model and analyze a wide range of data types, including pictures and 3D point clouds. The aforementioned applications can increase interior design process accuracy and efficiency. However, the constraints of these methodologies frequently revolve around the quality of the datasets and their application to various design situations. Future research should concentrate on increasing dataset variety and tackling real-world interior design challenges.
Interior Load-Bearing Elements: ML has been used for evaluating the structural safety of interior load-bearing elements. While these techniques show potential, they frequently rely on large datasets and may have generalizability restrictions. Future research should seek to create more robust and flexible ML models for evaluating structural safety in interior design.
Residential Design: ML approaches have been utilized to enhance factors such as art placement and inter-net-based booking systems in home design. These studies’ results, however, frequently have limitations in terms of dataset representativeness and generalizability. Future research should concentrate on increasing datasets and confirming the feasibility of these ML-driven design approaches in a variety of practical situations.
An extensive review of existing literature reveals significant incorporation of ML into the field of interior design, providing notable improvements and discoveries. Nonetheless, a synthesis of recurring facts and trends emerges. Starting with the crucial role of high-quality and diverse datasets in training ML models, a fundamental requirement to ensure dataset representativeness vis-à-vis real-world scenarios for the practical application of ML-driven solutions in interior design is underlined. The need to address this constraint through concentrated research efforts is emphasized. Furthermore, the interdisciplinary nature of the intersection of interior design and ML is obvious, requiring collaborative efforts between designers, architects, data scientists, and domain experts for the effective development of ML-driven solutions tailored to the various intricacies of interior design. Furthermore, a constant concern is the practical application of ML in real-world contexts, leading to a recurring request for additional validation and refining of ML-driven solutions to ensure their efficacy and effectiveness. Finally, when applying ML approaches, each subject within interior design brings distinct obstacles and constraints, needing careful thought and focused efforts by researchers to move the field ahead.
5 Conclusion
The importance of this research lies in its potential to drive significant improvements in interior design practices. By identifying current limitations and proposing directions for future research, this study paves the way for more effective and innovative design solutions, the successful integration of ML in interior design could lead to environments that are more responsive, personalized, and conducive to human wellbeing, marking a substantial leap forward in the field. Underscores the transformative potential of machine learning (ML) in interior design, highlighting both its achievements and areas necessitating further research. Where the study of color psychology in interior design revealed advancements in understanding the emotional influence of colors on individuals. However, limitations such as controlled experimental circumstances require more studies to confirm these findings in real-world design contexts. Although ML optimization of lighting schemes in interior design demonstrated considerable benefits, it was frequently limited to specific applications or experimental circumstances. Future research ought to implement these findings in a variety of real-world scenarios and investigate the scalability of ML-driven lighting optimization. In addition, challenges such as dataset quality and generalizability were observed in furniture factor applications such as fashion product retrieval systems and augmented reality integration. The influence of ML on hospital interior design, particularly biophilic design, revealed interesting insights but also required more validation in real-life circumstances considering a larger variety of environmental elements. The use of machine learning to model and analyze various data types in interior design provided prospects for enhanced accuracy and efficiency, but challenges relating to dataset quality and applicability must be addressed. Structural safety evaluation for interior load-bearing parts using ML approaches revealed promising benefits, however, issues in dataset representative-ness and generalizability must be addressed. High-quality and diverse datasets for training ML models are required for successful ML-driven solutions adapted to the complexities of interior design.
Data availability statement
The original contributions presented in the study are included in the article/supplementary material, further inquiries can be directed to the corresponding author.
Author contributions
SD: Data curation, Funding acquisition, Investigation, Project administration, Supervision, Writing–review and editing. SB: Writing–original draft, Writing–review and editing, Conceptualization, Data curation, Formal Analysis, Funding acquisition, Investigation, Methodology, Resources, Software, Visualization.
Funding
The author(s) declare that no financial support was received for the research, authorship, and/or publication of this article.
Acknowledgments
The authors express their gratitude to the Middle East University in Jordan for providing financial support to cover the publication fees associated with this research article.
While preparing this work, the authors used ChatGPT to improve readability and language. After using these tools, the authors reviewed and edited the content as needed and took full responsibility for the content of the publication.
Conflict of interest
The authors declare that the research was conducted in the absence of any commercial or financial relationships that could be construed as a potential conflict of interest.
Publisher’s note
All claims expressed in this article are solely those of the authors and do not necessarily represent those of their affiliated organizations, or those of the publisher, the editors and the reviewers. Any product that may be evaluated in this article, or claim that may be made by its manufacturer, is not guaranteed or endorsed by the publisher.
References
Abdel-Razek, S. A., Marie, H. S., Alshehri, A., and Elzeki, O. M. (2022). Energy efficiency through the implementation of an AI model to predict room occupancy based on thermal comfort parameters. Sustainability 14 (13), 7734. doi:10.3390/su14137734
Arabasy, M., Hussein, M. F., Abu Osba, R., and Al Dweik, S. (2024). Smart housing: integrating machine learning in sustainable urban planning, interior design, and development. Asian J. Civ. Eng. doi:10.1007/s42107-024-01144-3
Bouazizi, M., Lorite Mora, A., and Ohtsuki, T. (2023). A 2D-lidar-equipped unmanned robot-based approach for indoor human activity detection. Sensors 23 (5), 2534. doi:10.3390/s23052534
Cadusch, J. J., Meng, J., Craig, B., and Crozier, K. B. (2019). Silicon microspectrometer chip based on nanostructured fishnet photodetectors with tailored responsivities and machine learning. Optica 6 (9), 1171–1177. doi:10.1364/OPTICA.6.001171
Chen, J., Shao, Z., and Hu, B. (2023). Generating interior design from text: a new diffusion model-based method for effi-cient creative design. Buildings 13 (7), 1861. doi:10.3390/buildings13071861
Danilov, A., Benuzh, A., Yeye, O., Compaore, S., and Rud, N. (2020). Design of healthcare structures by green standards. In E3S Web of Conferences 164, 05002. Les Ulis, France: EDP Sciences. doi:10.1051/e3sconf/202016405002
Deng, H. (2021). Retracted ARTICLE: feature extraction method of stress distribution in indoor load-bearing buildings. Arabian J. Geosciences 14 (14), 1358. doi:10.1016/j.enbuild.2021.110860
Deng, Z., and Chen, Q. (2021). Reinforcement learning of occupant behavior model for cross-building transfer learning to various HVAC control systems. Energy Build. 238, 110860. doi:10.1016/j.enbuild.2021.110860
Dewingong, T. F., Afor, M. E., Mishra, P. K., Mishra, S., Mishra, G. S., and Aliyu, B. I. (2022). “Colour detection for interior designs using machine learning,” in International conference on advancements in interdisciplinary research (Cham: Springer Nature Switzerland.), 243–254. doi:10.1007/978-3-031-23724-9_23
D. R. Edla, P. Lingras, and K. Venkatanareshbabu (2018). Advances in machine learning and data science: recent achievements and research directives (Berlin, Germany: Springer.), 705. doi:10.1007/978-981-10-8569-7
Gonçalves, I., Gomes, Á., and Antunes, C. H. (2019). Optimizing the management of smart home energy resources under different power cost scenarios. Appl. Energy 242, 351–363. doi:10.1016/j.apenergy.2019.03.108
Goodfellow, I., Yoshua, B., and Aaron, C. (2016) Deep learning, 12. USA. Available at: http://www.deeplearningbook.org (accessed on May 28, 2023).
He, J., Li, B., and Wang, X. S. (2023). Image features and demand in the sharing economy: a study of Airbnb. Int. J. Res. Marketing. 40, 760–780. doi:10.1016/j.ijresmar.2023.04.001
Huiskes, M. J., and Pauwels, E. J. (2005). Indexing, learning and content-based retrieval for special purpose image databases. Adv. Comput., 65, 203–258. doi:10.1016/S0065-2458(05)65005-X
Jain, S., and Nayak, A. (2023). Impact of color on human behavior case -interior space. Int. J. Emerg. Res. Eng. Sci. Manag. 2. doi:10.58482/ijeresm.v2i1.6
Jung, D., Kim, D. I., and Kim, N. (2023). Bringing nature into hospital architecture: machine learning-based EEG analysis of the biophilia effect in virtual reality. J. Environ. Psychol. 89, 102033. doi:10.1016/j.jenvp.2023.102033
Kim, M. K. (2021). Affordance-based interior design with occupants' behavioural data. Indoor Built Environ. 30 (9), 1373–1389. doi:10.1177/1420326X20948015
Liu, T. (2023). A colour transfer method of interior design based on machine learning. Int. J. Inf. Commun. Technol. 22 (4), 438–455. doi:10.1504/IJICT.2023.131208
Machi, L. A., and McEvoy, B. T. (Editors) (2016). The literature review: six steps to success. 3rd Edn. California, United States: Corwin.
Mengyao, C., and Yu, T. (2023). Intelligent product art design based on smart equipment and machine learning algorithm: practice effect and trend analysis. Soft Comput. 27, 8449–8458. doi:10.1007/s00500-023-08146-4
Mooghali, A., Alijani, R., Karami, N., and Khasseh, A. A. (2011). “Scientometric analysis of the scientometric literature,” in Ternational journal of information science and management (IJISM), 9, 19–31.1
Mura, C., Pajarola, R., Schindler, K., and Mitra, N. (2021). Walk2map: extracting floor plans from indoor walk tra-jectories. In Comput. Graph. Forum. Chichester, United Kingdom: John Wiley & Sons Ltd., 40 (2), 375–388. doi:10.1111/cgf.142640
Nguyen, T. A., and Aiello, M. (2013). Energy intelligent buildings based on user activity: a survey. Energy Build. 56, 244–257. doi:10.1016/j.enbuild.2012.09.005
Nishikawa, A., Ono, K., and Miki, M. (2019). “User-friendly interior design recommendation,” in SIGGRAPH asia 2019 posters, 1–2. doi:10.1145/3355056.3364562
Park, B. H., Son, K., and Hyun, K. H. (2021). Interior design network of furnishing and color pairing with object detection and color analysis based on deep learning. In International conference on computer-aided architectural design futures. 237–249. doi:10.1007/978-981-19-1280-1_15
Parsaee, M., Demers, C. M., Hébert, M., and Lalonde, J. F. (2022). Imagery datasets for photobiological lighting analysis of architectural models with shading panels. Data Brief 42, 108278. doi:10.1016/j.dib.2022.108278
Pejic, J., and Pejic, P. (2022). Linear kitchen layout design via machine learning. Artif. Intell. Eng. Des. Analysis Manuf. 36, e9. doi:10.1017/S089006042100038X
Racec, E., Budulan, S., and Vellido, A. (2016). “Computational intelligence in interior design: state-of-the-art and outlook,” in Ccia, 108–113. doi:10.3233/978-1-61499-696-5-108
Ringel, M., Laidi, R., and Djenouri, D. (2019). Multiple benefits through smart home energy management solutions—a sim-ulation-based case study of a single-family-house in Algeria and Germany. Energies 12 (8), 1537. doi:10.3390/en12081537
Schreuder, E., Lebesque, L., and Bottenheft, C. (2016). Healing environments: what design factors really matter according to patients? An exploratory analysis. HERD Health Environ. Res. and Des. J., 10(1), 87–105. doi:10.1177/1937586716643951
Sepasgozar, S., Karimi, R., Farahzadi, L., Moezzi, F., Shirowzhan, S. M., Ebrahimzadeh, S., et al. (2020). A systematic content review of artificial intelligence and the internet of things applications in smart home. Appl. Sci. 10 (9), 3074. doi:10.3390/app10093074
Sepasgozar, S. M., Hawken, S., Sargolzaei, S., and Foroozanfa, M. (2019). Implementing citizen centric technology in de-veloping smart cities: a model for predicting the acceptance of urban technologies. Technol. Forecast. Soc. Change 142, 105–116. doi:10.1016/j.techfore.2018.09.012
Silverio-Fernández, M., Renukappa, S., and Suresh, S. (2018). What is a smart device? a conceptualisation within the para-digm of the internet of things. Vis. Eng. 6 (1), 3–10. doi:10.1186/s40327-018-0063-8
Ştefan, L., Gheorghiu, D., and Hodea, M. (2023). The history of furniture objects: an intelligent augmented reality application. In Augmented reality and artificial intelligence: the fusion of advanced technologies, 171–190. Cham: Springer Nature Switzerland. doi:10.1007/978-3-031-27166-3_10
Tanasra, H., Rott Shaham, T., Michaeli, T., Austern, G., and Barath, S. (2023). Automation in interior space planning: uti-lizing conditional generative adversarial network models to create furniture layouts. Buildings, 13(7), 1793. doi:10.3390/buildings13071793
Tao, F., Cheng, J., Qi, Q., Zhang, M., Zhang, H., and Sui, F. (2018). Digital twin-driven product design, manufacturing and service with big data. Int. J. Adv. Manuf. Technol. 94, 3563–3576. doi:10.1007/s00170-017-0233-1
Tautkute, I., Trzciński, T., Skorupa, A. P., Brocki, Ł., and Marasek, K. (2019). Deepstyle: multimodal search engine for fashion and interior design. IEEE Access 7, 84613–84628. doi:10.1109/ACCESS.2019.2923552
Terrier, R., and Martin, N. (2020). A machine learning tool to match 2D drawings and 3D objects’ category for populating mockups in VR. Virtual Real. Augmented Real. 17th EuroVR Int. Conf. EuroVR 2020, Valencia, Spain, November 25–27, 2020, Proc. 17, 240–246. doi:10.1007/978-3-030-62655-6_17
Van Eck, N., and Waltman, L. (2010). Software survey: VOSviewer, a computer program for bibliometric mapping. scientometrics 84 (2), 523–538. doi:10.1007/s11192-009-0146-3
Wang, Y. T., Liang, C., Huai, N., Chen, J., and Zhang, C. J. (2023). “A survey of personalized interior design,” in Computer graphics forum 42 (6), e14844. Chichester, United Kingdom: John Wiley & Sons Ltd. doi:10.4018/IJMCMC.293752
Winter, M., Sweeney, L., Mason, K., and Blume, P. (2022). Low-power machine learning for visitor engagement in museums. In Proceedings of the 6th international conference on computer-human interaction research and applications (CHIRA 2022). . Setúbal, Portugal: INSTICC, ScitePress, 236–243. doi:10.5220/0011585600003323
Wrigh, S. W. A. B. (2020). Gap analysis for literature reviews and advancing useful knowledge. Available at: www.researchtoaction.org/2020/06/gap-analysis-for-literature-reviews-and-advancing-usefulknowledge/website.
Yu, J., and Egger, R. (2021). Color and engagement in touristic Instagram pictures: a machine learning approach. Ann. Tour. Res., 89, 103204. doi:10.1016/j.annals.2021.103204
Zhang, Z., and Ban, J. (2022). Aesthetic evaluation of interior design based on visual features. Int. J. Mob. Comput. Multimedia Commun. (IJMCMC) 13 (2), 1–12. doi:10.4018/IJMCMC.293752
Zhao, H., and Hao, F. (2021). Target tracking algorithm for table tennis using machine vision. J. Healthc. Engi-neering, 2021, 1–7. doi:10.1155/2021/9961978
Zhao, M., Chen, J., Yang, Z., Liu, Y., and Zhang, S. (2022). HomeMonitor: an enhanced device event detection method for smart home environment. Sensors 22 (23), 9389. doi:10.3390/s22239389
Zheng, H., Keyao, A. N., Jingxuan, W. E. I., and Yue, R. E. N. (2020). “Apartment floor plans generation via gen-erative adversarial networks,” in Proceedings of the 25th international conference of the association for computer-aided architectural design research in asia (CAADRIA 2020): re: anthropocene, design in the age of humans. Editors D. Holzer, W. Nakapan, A. Globa, and I. Koh (Bangkok, Thailand: The Association for Computer-Aided Architectural Design Research in Asia (CAADRIA)), 599–608. doi:10.52842/conf.caadria.2020.2.599
Keywords: machine learning, interior design, artificial intelligence, interior-environment, smart technology, sustainability
Citation: Dwiek SA and Bast SA (2024) The application of machine learning in inner built environment: scientometric analysis, limitations, and future directions. Front. Built Environ. 10:1413153. doi: 10.3389/fbuil.2024.1413153
Received: 06 April 2024; Accepted: 31 October 2024;
Published: 14 November 2024.
Edited by:
Hasim Altan, Prince Mohammad bin Fahd University, Saudi ArabiaReviewed by:
Giovanni Ciampi, Università degli Studi della Campania Luigi Vanvitelli, ItalyRehab Ghoneim, Al-Ahliyya Amman University, Jordan
Copyright © 2024 Dwiek and Bast. This is an open-access article distributed under the terms of the Creative Commons Attribution License (CC BY). The use, distribution or reproduction in other forums is permitted, provided the original author(s) and the copyright owner(s) are credited and that the original publication in this journal is cited, in accordance with accepted academic practice. No use, distribution or reproduction is permitted which does not comply with these terms.
*Correspondence: Safaa Al Bast, c2FmYWFfMTJfOUB5YWhvby5jb20=, c2FsYmFzdEBtZXUuZWR1Lmpv