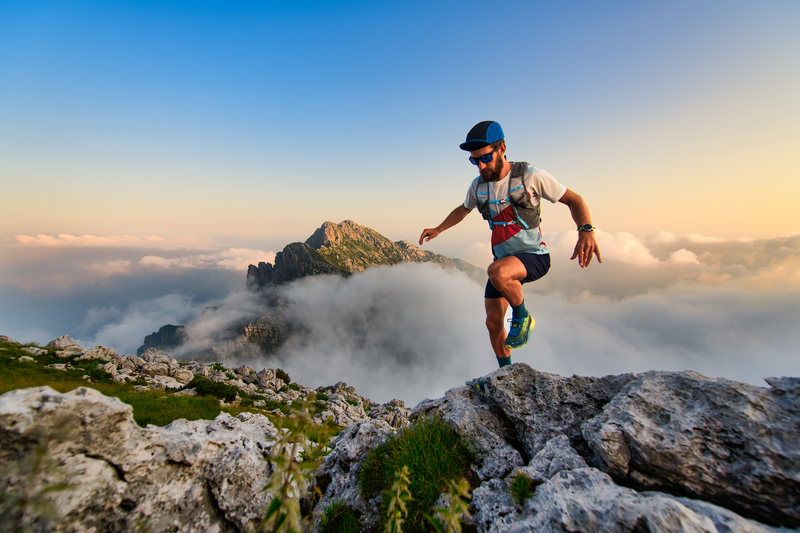
95% of researchers rate our articles as excellent or good
Learn more about the work of our research integrity team to safeguard the quality of each article we publish.
Find out more
REVIEW article
Front. Built Environ. , 05 March 2024
Sec. Transportation and Transit Systems
Volume 10 - 2024 | https://doi.org/10.3389/fbuil.2024.1383144
Traffic accidents are a nationwide public health concern, but autonomous vehicles (AVs) have the potential to significantly reduce accident severity and frequency by eliminating their most common cause, human error. By analyzing the data published by California’s Department of Motor Vehicles, researchers have identified the factors that influence AV crash severity, however, none do so through a literature review. This paper’s aims are multi-faceted: to understand AVs’ operation on public roadways by identifying and classifying the factors contributing to accident severity, to develop a list of strategies that address the public’s safety concerns, and to acknowledge the ethics of unavoidable collisions. To fulfill these objectives, a comprehensive literature review was conducted based on a keyword search. Following a multi-step screening and exclusion process, detailed review was performed of 107 relevant publications, and the factors contributing to increased crash severity were classified into 14 categories. The literature revealed that AVs are not at fault in most accidents, although they have a higher propensity than conventional cars to be involved in rear-end collisions, and they are specifically designed to minimize the number of accidents, but may face unavoidable ones. For the benefit of policymakers and manufacturers, 11 strategies that address the moral dilemma of these accidents and 7 strategies that address concerns about AV safety to improve public perception were identified.
The National Highway Traffic Safety Administration estimates that more than 6 million automobile incidents cause 2 million injuries and 37,000 fatalities per year in the United States (Beck et al., 2023), making it a nationwide public health concern. According to Combs et al. (2019), 94% of the fatalities are due to human error caused by interactions within a complex, volatile, and unpredictable driving environment. Autonomous vehicles (AVs), however, are expected to reduce the human error factor by leveraging efficient detection, hence they have the ability to lower the number of traffic accidents and the accompanying fatalities and injuries.
Autonomous driving systems have the potential to boost self-sufficiency for groups with limited mobility, decrease pollutant emissions, and reduce traffic congestion while improving highway safety (Robinson et al., 2022), and their multi-sensor technology enables them to detect their environment and react dependably and safely. To fully realize these advantages, however, they have to be accepted by the general public (Evans et al., 2020), a factor that is frequently regarded as the biggest obstacle to universal adoption.
The Society of Automotive Engineers (SAE) outlines six levels of automation: Level 0 is completely manual, Levels 1 to 3 are partially autonomous, and Levels 4 to 5 are completely autonomous (Favarò et al., 2018). As the level of automation rises, the vehicle requires less human involvement and support to function safely, which minimizes driver-related road traffic hazards (Ye et al., 2021). Regardless of their level of automation, however, safety remains a concern, and extensive testing is performed to ensure that they do in fact drastically reduce the number of accidents. In the United States, California is one of many states conducting comprehensive testing (Sinha et al., 2021), and the California Department of Motor Vehicles (CA DMV) permits manufacturers to operate AVs on public roads, as they compile and publish reports on disengagements and collisions (Dixit et al., 2016). As these reports are the only datasets that are widely available, researchers globally have analyzed the data to assess the performance of AVs.
AVs and their various facets and implications have been thoroughly explored in the literature by authors such as Parekh et al. (2022), who reviewed AV technologies and explored environment detection, path planning, and pedestrian detection but neglected to discuss policy implications. Contrastingly, Faisal et al. (2019) focused almost entirely on the policy issues introduced by AVs and how to best prepare for their deployment but did not consider unavoidable collisions or their ethical implications. Robinson et al. (2022) explored the moral dilemma presented by unavoidable collisions and discussed the implications of existing studies; however, they failed to consider the real-world safety of AVs. Many researchers have utilized the CA DMV data to evaluate the actual performance of AVs (Xu et al., 2019; Sinha et al., 2021), and Yuan et al. (2022) and Ren et al. (2022), analyzed the data to determine the factors that influence the severity of the crashes. Unfortunately, however, they failed to combine the data with that obtained from a literature review or discuss the policy impacts of unavoidable collisions and the public perception of AV safety.
Thus, the following objectives were developed to ascertain the influence of AVs on traffic accidents and injuries by analyzing the existing literature on the topic: 1) identify factors contributing to AV crash severity and classify them according to characteristics of the collision, weather conditions, location, and other variables, 2) develop a list of strategies designed to address the public’s AV safety concerns, and 3) define the strategies designed to acknowledge the ethical implications of unavoidable collisions. This study’s findings will be helpful to both AV manufacturers and policymakers and will serve as a catalyst for future research that will enhance AV safety and widespread global adoption of AVs.
A systematic literature review was conducted to compile a database of relevant journal articles, technical reports, conference papers, and theses. Keywords such as autonomous vehicles, vulnerable road users, self-driving vehicles, traffic accidents, road safety, unavoidable collisions, crash analysis, and CA DMV were inputted in different combinations into online search engines Google Scholar, ASCE Library, and Science Direct for the initial selection of 190 articles that underwent the inclusion and exclusion criteria demonstrated in Figure 1. To enhance the database, a variety of articles from various disciplines, including transportation, psychology, and ethics, were considered.
After conducting the keyword search, the literature was screened to exclude any duplicates and inappropriate document types, and the titles and abstracts of the publications were reviewed to verify their relevance. A total of 106 studies met all the criteria and were comprehensively reviewed. The intent of this study was to assess the impact of AVs on roadway safety and through the literature review, three subtopics were identified. Thus, following the multistep screening process, the literature was divided into the following groups: 1) articles analyzing CA DMV collision and disengagement reports, 2) studies discussing the interactions and relationships between AVs and members of the public, and 3) papers concentrating on moral and ethical concerns associated with unavoidable AV collisions. The finalized database was intended to reflect a thorough analysis of the literature’s representation of the state of autonomous vehicles and traffic safety.
Figure 2 demonstrates the frequency of articles based on the year that they were published and shows that the earliest articles were published on autonomous vehicles and road safety in 2012, then beginning with a significant jump in 2021, there has been a steady increase in the number of published articles. The figure also shows that 2022 was the year with the greatest number of articles (36 journal papers), followed by 2021 (26 journal papers). For this study, articles published until July of 2023 were considered in the database.
Figure 3 shows the distribution of 106 articles in the database that were published in 25 countries, which emphasizes the global interest in the impact of AVs on road safety. The United States had the greatest frequency and published about one-third (35%) of all the articles reviewed, followed by China with 14% and the United Kingdom with 8%. Countries such as Hungary, Japan, and Austria that are not depicted in Figure 3 published less than 1% of the articles studied and cumulatively represent 11% of all articles reviewed.
AVs could revolutionize safety in the transportation sector. Elimination of human involvement in the driving environment can reduce traffic accidents, consequently reducing associated injuries and fatalities. In recent years, there has been a steady increase in the research published on AVs and their impact on safety, which may be attributed to the imminent reality of AVs’ widespread deployment. Through an analysis of the published literature, the authors identified the following subthemes: 1) the crash severity of AVs, 2) interactions between vulnerable road users and AVs, and 3) the ethical implications of autonomous driving technologies. Although there are numerous studies on the assessment of AV safety using CA DMV data, the authors found that the existing literature was also rife with research highlighting concerns surrounding the public perception of AV safety and the moral quandary raised by AV’s potential involvement in unavoidable accidents. Thus, this section explores the current state of AVs and traffic safety through each of these subthemes and presents strategies that stakeholders can use to acknowledge the challenges inherent in the technology.
The adoption of AV technology has the potential to increase roadway safety by diminishing the impact of human error, the cause of most accidents involving conventional vehicles (Novat et al., 2023). The lack of crash data and limited number of AVs on roads, however, have necessitated extensive field and simulation studies to ensure that they are safe (Song et al., 2021). California is one of many states that has passed legislation permitting AV testing on public roadways (Schoettle and Sivak, 2015), and the CA DMV has ordered the public release of AV disengagement and crash data to allow individuals to better comprehend their performance in a mixed traffic environment (Das et al., 2020). AVs are being tested globally, but researchers are utilizing CA DMV data for analysis purposes, as it is the only widely available crash and disengagement data (Houseal et al., 2022). Hence, the discussion in the following sections is only indicative of the behavior of AVs within the state of California and might not apply in other geographical settings.
Crash reports include details of the circumstances leading up to a collision, any resulting injuries and/or property damage, the geolocation of the collision, the type of crash, weather, roadway conditions, and time and date of the incident (Sinha et al., 2021; Novat et al., 2023). Disengagement reports provide information on every recorded disengagement, including road and weather conditions and other influencing factors (Dixit et al., 2016), and the open-source accessibility allows researchers to perform studies that use a variety of statistical models and descriptive analyses to examine AV collisions and disengagements and evaluate AV performance and safety concerns. Table 1 provides an overview of the literature reviewed.
As demonstrated in Table 1, multiple authors avow that rear-end and sideswipe collisions are the most frequently occurring types of crashes (Favarò et al., 2017; Leilabadi and Schmidt, 2019; Wang and Li, 2019; Boggs et al., 2020; Das et al., 2020; Petrović et al., 2020). Liu et al. (2021) utilized statistical analysis to identify the distinctions between the pre-crash scenarios of conventional cars and AVs and determined that the two groups differed in the number of collisions when the situation was the same. Based on the data points analyzed, AVs are 1.6 times more likely to be involved in a rear-ended collision than conventional vehicles due to AVs’ insufficient path planning or their inaccurate predictions of the behaviors of human drivers (Liu et al., 2021). The difference between the perception-reaction time of drivers of conventional vehicles and AVs may also play a role (Liu et al., 2021). Petrović et al. (2020) theorized that the frequency of rear-ended collisions may be attributed to the behavior of conventional vehicle drivers, as they are unaccustomed to the dynamic driving style of AVs that encompasses fully complying with traffic regulations and not being susceptible to human driving errors or behaviors. Thus, for most rear-end accidents, drivers of conventional vehicles are found to be at fault for driving too fast and too close to the AV.
Despite the increased likelihood of rear-end accidents, AVs are less likely than conventional vehicles to be involved in sideswipes, head-ons, and other types of collisions (Novat et al., 2023). Broadside and pedestrian collisions are significantly less likely to occur with AVs also, as they represent only about 6% of AV accidents compared to 42% of conventional vehicle accidents (Petrović et al., 2020). This difference can be attributed to AVs’ ability to better evaluate their surrounding environment and locate stationary or moving objects with the use of complex technological systems (Petrović et al., 2020).
Although it was determined that AVs are not responsible for 87% of the accidents they are involved in (Wang and Li, 2019), it is essential to identify the factors that contribute to the severity of the collisions that do occur. When a vehicle is involved in a collision, the severity level can range from no injury to a severe impact on the vehicle and persons involved, and in extreme cases can even result in fatalities (Yuan et al., 2022). It was determined through an analysis of CA DMV collision reports that most AV crashes result in minor damage to both the AV and the other vehicle, and most of the personal injuries involve back pain (Dixit et al., 2016). Back, head and neck injuries are the most common type of injury, with AV occupants representing 70.83% of those injured (Ye et al., 2021). As safety is considered the primary advantage of AVs, the factors associated with increased crash severity were identified and are depicted in Table 2.
While the CA DMV data illuminates how AVs operate in a mixed traffic environment, the findings may not accurately represent their performance due to limitations associated with the data. Specifically, the sample size of AVs considered in the published data is small compared with the number of conventional vehicles on the roads, and the data is limited to a specific geographical location that is not representative of all driving conditions in a global context. For instance, California does not experience adverse weather conditions, thus the findings do not illustrate how AVs perform in severe winter weather. Beyond that, it is unlikely that all driving conditions have been represented, as the driving environment is complex, dynamic, and unpredictable, and the limited amount of available published data on crash and disengagement reports make a comprehensive analysis challenging if not impossible. Furthermore, these findings cannot be generalized globally since the performance of testing vehicles is restricted to California’s roadway infrastructure and traffic regulations. Future research should account for this inadequacy by considering different locations and analyzing how additional factors may impact the operations of the technology.
AV interactions with other road users will likely receive more attention as the technology continues to develop. AV designers and manufacturers are continuously improving the vehicles’ capacity to screen their surroundings, detect nearby road users, and predict other drivers’ movements (Alozi and Hussein, 2022); however, despite their potential to increase road safety by minimizing human involvement, safety concerns are likely to arise if their interactions with other modes of transportation are not considered (Guo et al., 2022; Chen et al., 2023).
Interactions between road users can be unpredictable, even when all other factors remain constant (Chen et al., 2023), and AVs must be able to examine their surroundings and forecast the future behaviors of other road users before making control decisions (Parekh et al., 2022). This assessment process includes how the vehicle perceives its immediate surroundings, taking into account the infrastructure, obstructions, and nearby road users, as well as curbs, lanes, traffic signs, and signals (Hafeez et al., 2022). Radar, visible light cameras (VLC), and light detection and ranging (LiDAR) relay information about objects, traffic conditions, and lane occupancy to the driving system (Iftikhar et al., 2022; Parekh et al., 2022), but the foundation of AV technology is detecting elements of the road to ensure that it reacts appropriately to its surroundings and operates safely. The utilization of a multi-sensor system encompassing radar, VLC, and LiDAR is considered the optimum strategy since the advantages of one sensor offset the limitations of another (Combs et al., 2019). LiDAR uses scanning lasers to create a three-dimensional map that helps avoid collisions, while radar detection systems use radio waves to locate objects, and VLCs capture the surrounding area (Combs et al., 2019; Parekh et al., 2022).
Pedestrians are often regarded as the most vulnerable road users because of their frequent overrepresentation in traffic-related injuries and fatalities (Alozi and Hussein, 2022). Their actions and behaviors are highly unpredictable and probabilistic, they frequently appear in a variety of settings that are influenced by the surrounding environment, and the weather that can make it challenging to detect their presence (Combs et al., 2019) and distinguish between them and other visages in low visibility conditions and/or with low camera resolutions (Iftikhar et al., 2022; Parekh et al., 2022). Pedestrian-AV interactions are a crucial element in AV adoption and public trust (Kaur and Rampersad, 2018), but AVs have functional issues that make it difficult for them to detect pedestrians (Iftikhar et al., 2022). Fortunately, VLCs and a multi-sensor approach have the potential to change that and reduce pedestrian fatalities by approximately 90% (Combs et al., 2019).
Multiple researchers have conducted surveys that enabled them to evaluate public receptivity or attitudes towards AVs in relation to transportation safety (Pettigrew et al., 2019; Qu et al., 2019; Hasan et al., 2022), and their findings have consistently revealed that public perception is the biggest barrier to AV adoption, with safety being the primary concern. As a result, failure to ensure that vulnerable road users feel safe interacting with AVs could adversely affect other forms of transportation, such as walking and cycling, and hinder AVs’ integration into public roadways. Table 3 identifies strategies for policymakers and manufacturers that are designed to address AV safety concerns and their impacts on vulnerable road users.
Full utilization of AV technology is dependent upon manufacturers gaining the public’s trust and acceptance, and alleviating their concerns about scenarios involving inevitable collisions. The safety image of AVs has a considerable impact on how the public perceives autonomous driving technologies, and many studies have pondered who should be held legally and morally liable if a fully autonomous car causes a collision (Hevelke and Nida-Rümelin, 2015; Aguiar et al., 2022). In a potentially unavoidable accident, an AV has two choices, both of which have serious consequences: (1) stay on course and hit the approaching car; or (2) swerve and hit a pedestrian. This moral conundrum has been extensively discussed in the literature (Martinho et al., 2021), but the many potential outcomes and variables make it challenging to determine which choice is the most morally acceptable (Santoni de Sio, 2017). Autonomous cars take longer than human drivers in conventional cars to make pre-planned decisions that determine the vehicles’ actions (Robinson et al., 2022), and programmers need to consider how AVs react when faced with an unavoidable accident (Santoni de Sio, 2017). Table 4 identifies strategies for policymakers and manufacturers that are designed to reduce the moral dilemmas these unavoidable accidents present.
The ambiguous nature of ethics and individuals’ beliefs, values, opinions, and feelings make it challenging to determine what constitutes an AV’s most morally acceptable action (Martinho et al., 2021; Robinson et al., 2022), and everyone has his or her own set of personal ethical standards that can change, depending on a variety of things such as beliefs, values, opinions, and feelings. Because of this, manufacturers may make judgments based on experimental ethics, in which people are polled over the moral acceptability of various programming possibilities (Bonnefon et al., 2016); however, given the wide range of what is regarded as ethically acceptable or prohibited, this might not be a feasible approach (Santoni de Sio, 2017).
The public’s participation in the decision-making process is necessary to better understand their expectations of AVs’ behavior in unavoidable incidents and what decisions they consider ethical (Robinson et al., 2022). Several possible outcomes can be taken into consideration when an AV has an unavoidable collision, but since public acceptance is thought to be the main obstacle to the adoption of AVs, a widely acceptable solution should be put into place to increase public acceptance and trust. Because everyone has a different understanding of what is the proper course of action, the Massachusetts Institute of Technology conducted a study in which they polled individuals about the moral dilemmas involving AVs using an online experimental platform (Awad et al., 2018). Respondents from 233 nations provided 400 million decisions for the Moral Machine Experiment, which analyzed the preferred course of action that an AV should undertake, such as whether it should swerve, and examined case studies that contained sociodemographic characteristics of those injured and whether they were passengers or pedestrians (Awad et al., 2018). The study sought to quantify society’s expectations for moral behavior that should direct an AV’s decision-making process through the development of common ethical principles, but the findings emphasized the lack of homogeneity in what people view as moral. Thus, the validity of research like the Moral Machine Experiment has been questioned, as the results may be impacted by procedural or natural bias (Robinson et al., 2022).
Evans et al. (2020) avowed that utilizing a computational framework flexible enough to support a wide range of ethical standards is more effective than developing shared ethical principles since people have different views of the right moral course of action an AV should follow when involved in an unavoidable collision. They viewed AV decision-making as a form of claim mitigation in which people have various moral claims that influence how an AV reacts to both the user’s expectations and its environment. It is generally accepted that an autonomous driving system’s decision-making process is the key to reducing the number of crashes where human injury is likely to result. This has to do with the ability of the AV to simulate human judgment and driving behaviors while reducing human errors that are present in the traffic environment. Driving conditions are complicated, volatile, unpredictable, and include a wide range of diverse means of transportation; thus, potential solutions can be investigated by adopting virtual reality or driving simulation approaches (Robinson et al., 2022) that can enhance immersion and ensure process validity and reliability without compromising safety.
Guidelines, including the German Ethics Code for Automated and Connected Driving (Luetge, 2017) and the European Commission’s report on the Ethics of Connected and Automated Vehicles autonomous driving systems were developed in response to the ethical dilemmas presented by the scenarios of unavoidable collisions, where an AV has no choice but to be involved in a collision where human harm is a likely consequence (Bonnefon et al., 2020). These recommendations can be used to provide a framework for establishing an AV decision-making process that can be employed in the face of an inevitable collision, where an automated machine may have to decide who must live or die. Both guidelines cautioned against programming AVs to deliberately favor one life over another based on personal characteristics, such as prioritizing a child’s life at the expense of an adult’s. The situations elicit moral reflection and call for consideration of issues such as who bears responsibility when an AV runs over a person or whether an AV’s passengers can be held accountable when a crash occurs (Aguiar et al., 2022). Such factors affect how regulations and policies are introduced and have practical implications for stakeholders within the AV industry. Both the European Commission’s report and the German Ethics Code emphasize the importance of designing autonomous driving systems with effective collision avoidance and safeguards against inappropriate or unsafe operation by drivers of conventional vehicles (Luetge, 2017; Bonnefon et al., 2020). The moral dilemma will become less significant as the likelihood of an inevitable collision decreases (Robinson et al., 2022).
Questions regarding who should bear responsibility in the event of an inevitable collision are frequently at the center of discussions about AVs and ethical dilemmas. While putting all of the blame on the manufacturer seems like the obvious course of action, doing so could prevent further development of AVs (Marchant and Lindor, 2012). Due to the potential conflict of interest between the manufacturer’s liability concerns and the consumer’s desire for safety (Robinson et al., 2022), legislation should be created to structure tort liability for AV manufacturers that encourages the development and enhancement of AVs (Hevelke and Nida-Rümelin, 2015). Researchers have also explored the consumer’s role in collisions and whether AV users can be held accountable in the event of an unavoidable accident. While drivers have a duty to pay attention to traffic and the road and have the ability to foresee and prevent accidents by taking action when required (Hevelke and Nida-Rümelin, 2015), this solution has an exclusionary nature because it neglects users with restricted mobility who are dependent on fully autonomous vehicles for transportation.
This paper aims to determine the impacts of AVs on traffic accidents and injuries through the identification of crash severity factors, identify strategies that address the public’s AV safety concerns, and acknowledge the ethical implications of unavoidable collisions. Factors contributing to increased AV crash severity were identified and classified into 14 categories, and strategies for policymakers and manufacturers were developed to improve public perception and address the moral ramifications of unavoidable accidents.
Analysis of the data made public by the CA DMV lends credence to the idea that the use of AVs will increase road safety because of their ability to evaluate their surroundings, as most accidents, including broadside collisions and those involving pedestrians, are caused by drivers of conventional vehicles, even though AVs are more likely to be engaged in rear-ended collisions. Other factors were also identified that are likely to increase crash severity, including rear-end crashes, vehicles operating in autonomous mode or parked roadside, and adverse weather conditions. Although there was limited apparent correlation between AV crash severity and weather or road conditions, this may be a result of the data provided, as California was the only geographical location studied.
Achieving public acceptance is commonly considered the greatest barrier to widespread use of AVs, and the findings of this study showed that those who trust the technology are more likely to be persuaded of the effectiveness and safety of AVs. Multiple authors have also emphasized the importance of road users feeling safe while interacting with AVs in a mixed traffic environment. If they do not, the result will either be reduced pedestrian and cyclist activity or a delay in the integration of AVs into public roadways. In this study, strategies were identified that policymakers and manufacturers can employ to promote public acceptance and improve the societal perception of autonomous driving systems, such as providing opportunities for the public to interact with the technology, and the ethical implications of AVs and unavoidable accidents were discussed. While AVs are designed to reduce accidents, unavoidable collisions are likely to occur, and the complexity of the AV’s decision-making process and the lack of a clear “right” or “wrong” means that vehicles are forced to make moral decisions that may be difficult to justify. Involving the public and prioritizing their input to the AV’s decision-making process is a feasible solution, however, that will ensure that the vehicle is operating in a manner that is acceptable to most. Through this study, transportation specialists will gain a comprehensive understanding of AV crash severity, and AV manufacturers and policymakers will benefit, as the moral and legal ramifications of unavoidable accidents are discussed alongside the public acceptance of autonomous driving systems.
DA: Conceptualization, Formal Analysis, Methodology, Writing–original draft, Writing–review and editing. SK: Conceptualization, Methodology, Supervision, Writing–review and editing. AP: Conceptualization, Methodology, Writing–review and editing.
The author(s) declare that no financial support was received for the research, authorship, and/or publication of this article.
The authors declare that the research was conducted in the absence of any commercial or financial relationships that could be construed as a potential conflict of interest.
All claims expressed in this article are solely those of the authors and do not necessarily represent those of their affiliated organizations, or those of the publisher, the editors and the reviewers. Any product that may be evaluated in this article, or claim that may be made by its manufacturer, is not guaranteed or endorsed by the publisher.
Aguiar, F., Hannikainen, I. R., and Aguilar, P. (2022). Guilt without fault: accidental agency in the era of autonomous vehicles. Sci. Eng. ethics 28 (2), 11. doi:10.1007/s11948-022-00363-8
Alozi, A. R., and Hussein, M. (2022). Evaluating the safety of autonomous vehicle–pedestrian interactions: an extreme value theory approach. Anal. methods Accid. Res. 35, 100230. doi:10.1016/j.amar.2022.100230
Awad, E., Dsouza, S., Kim, R., Schulz, J., Henrich, J., Shariff, A., et al. (2018). The moral machine experiment. Nature 563 (7729), 59–64. doi:10.1038/s41586-018-0637-6
Beck, J., Arvin, R., Lee, S., Khattak, A., and Chakraborty, S. (2023). Automated vehicle data pipeline for accident reconstruction: new insights from LiDAR, camera, and radar data. Accid. Analysis Prev. 180, 106923. doi:10.1016/j.aap.2022.106923
Boggs, A. M., Wali, B., and Khattak, A. J. (2020). Exploratory analysis of automated vehicle crashes in California: a text analytics & hierarchical Bayesian heterogeneity-based approach. Accid. Analysis Prev. 135, 105354. doi:10.1016/j.aap.2019.105354
Bonnefon, J. F., Černy, D., Danaher, J., Devillier, N., Johansson, V., Kovacikova, T., et al. (2020). Ethics of connected and automated vehicles: recommendations on road safety, privacy, fairness, explainability and responsibility. doi:10.2777/035239
Bonnefon, J. F., Shariff, A., and Rahwan, I. (2016). The social dilemma of autonomous vehicles. Science 352 (6293), 1573–1576. doi:10.1126/science.aaf2654
Chen, Y., Li, S., Tang, X., Yang, K., Cao, D., and Lin, X. (2023). Interaction-aware decision making for autonomous vehicles. IEEE Trans. Transp. Electrification 9, 4704–4715. doi:10.1109/TTE.2023.3240454
Combs, T. S., Sandt, L. S., Clamann, M. P., and McDonald, N. C. (2019). Automated vehicles and pedestrian safety: exploring the promise and limits of pedestrian detection. Am. J. Prev. Med. 56 (1), 1–7. doi:10.1016/j.amepre.2018.06.024
Das, S., Dutta, A., and Tsapakis, I. (2020). Automated vehicle collisions in California: applying Bayesian latent class model. IATSS Res. 44 (4), 300–308. doi:10.1016/j.iatssr.2020.03.001
Ding, S., Abdel-Aty, M., Wang, D., Wang, Z., Zheng, O., Barbour, N., et al. (2023). Exploratory analysis of the crash severity between vehicular automation (SAE L2-5) with multi-source data. doi:10.48550/arXiv.2303.17788
Dixit, V. V., Chand, S., and Nair, D. J. (2016). Autonomous vehicles: disengagements, accidents and reaction times. PLoS one 11 (12), e0168054. doi:10.1371/journal.pone.0168054
Evans, K., de Moura, N., Chauvier, S., Chatila, R., and Dogan, E. (2020). Ethical decision making in autonomous vehicles: the AV ethics project. Sci. Eng. ethics 26, 3285–3312. doi:10.1007/s11948-020-00272-8
Faisal, A., Kamruzzaman, M., Yigitcanlar, T., and Currie, G. (2019). Understanding autonomous vehicles: a systematic literature review on capability, impact, planning and policy. J. Transp. land use 12 (1), 45–72. doi:10.5198/jtlu.2019.1405
Favarò, F., Eurich, S., and Nader, N. (2018). Autonomous vehicles’ disengagements: trends, triggers, and regulatory limitations. Accid. Analysis Prev. 110, 136–148. doi:10.1016/j.aap.2017.11.001
Favarò, F. M., Nader, N., Eurich, S. O., Tripp, M., and Varadaraju, N. (2017). Examining accident reports involving autonomous vehicles in California. PLoS one 12 (9), e0184952. doi:10.1371/journal.pone.0184952
Guo, J., Yuan, Q., Yu, J., Chen, X., Yu, W., Cheng, Q., et al. (2022). External human–machine interfaces for autonomous vehicles from pedestrians’ perspective: a survey study. Sensors 22 (9), 3339. doi:10.3390/s22093339
Guo, X., and Zhang, Y. (2022). Maturity in automated driving on public roads: a review of the six-year autonomous vehicle tester program. Transp. Res. Rec. 2676 (11), 352–362. doi:10.1177/03611981221092720
Hafeez, F., Ullah Sheikh, U., Mas’ ud, A. A., Al-Shammari, S., Hamid, M., and Azhar, A. (2022). Application of the theory of planned behavior in autonomous vehicle-pedestrian interaction. Appl. Sci. 12 (5), 2574. doi:10.3390/app12052574
Hasan, O., McColl, J., Pfefferkorn, T., Hamadneh, S., Alshurideh, M., and Kurdi, B. (2022). Consumer attitudes towards the use of autonomous vehicles: evidence from United Kingdom taxi services. Int. J. Data Netw. Sci. 6 (2), 537–550. doi:10.5267/j.ijdns.2021.11.010
Hevelke, A., and Nida-Rümelin, J. (2015). Responsibility for crashes of autonomous vehicles: an ethical analysis. Sci. Eng. ethics 21, 619–630. doi:10.1007/s11948-014-9565-5
Houseal, L. A., Gaweesh, S. M., Dadvar, S., and Ahmed, M. M. (2022). Causes and effects of autonomous vehicle field test crashes and disengagements using exploratory factor analysis, binary logistic regression, and decision trees. Transp. Res. Rec. 2676 (8), 571–586. doi:10.1177/03611981221084677
Iftikhar, S., Zhang, Z., Asim, M., Muthanna, A., Koucheryavy, A., and Abd El-Latif, A. A. (2022). Deep learning-based pedestrian detection in autonomous vehicles: substantial issues and challenges. Electronics 11 (21), 3551. doi:10.3390/electronics11213551
Jacobo, A. M., Nobuyuki, U., Kunio, Y., Koichiro, O., Eiichi, K., and Satoshi, T. (2019). “Development of a safety assurance process for autonomous vehicles in Japan,” in Proceedings of ESV Conference.
Kaur, K., and Rampersad, G. (2018). Trust in driverless cars: investigating key factors influencing the adoption of driverless cars. J. Eng. Technol. Manag. 48, 87–96. doi:10.1016/j.jengtecman.2018.04.006
Khattak, Z. H., Fontaine, M. D., and Smith, B. L. (2020). Exploratory investigation of disengagements and crashes in autonomous vehicles under mixed traffic: an endogenous switching regime framework. IEEE Trans. intelligent Transp. Syst. 22 (12), 7485–7495. doi:10.1109/TITS.2020.3003527
Leilabadi, S. H., and Schmidt, S. (2019). “In-depth analysis of autonomous vehicle collisions in California,” in 2019 IEEE Intelligent Transportation Systems Conference (ITSC) (IEEE), 889–893. doi:10.1109/ITSC.2019.8916775
Liu, Q., Wang, X., Wu, X., Glaser, Y., and He, L. (2021). Crash comparison of autonomous and conventional vehicles using pre-crash scenario typology. Accid. Analysis Prev. 159, 106281. doi:10.1016/j.aap.2021.106281
Luetge, C. (2017). The German ethics code for automated and connected driving. Philosophy Technol. 30, 547–558. doi:10.1007/s13347-017-0284-0
Marchant, G. E., and Lindor, R. A. (2012). The coming collision between autonomous vehicles and the liability system. St. Clara L. Rev. 52, 1321.
Martinho, A., Herber, N., Kroesen, M., and Chorus, C. (2021). Ethical issues in focus by the autonomous vehicles industry. Transp. Rev. 41 (5), 556–577. doi:10.1080/01441647.2020.1862355
Novat, N., Kidando, E., Kutela, B., and Kitali, A. E. (2023). A comparative study of collision types between automated and conventional vehicles using Bayesian probabilistic inferences. J. Saf. Res. 84, 251–260. doi:10.1016/j.jsr.2022.11.001
Parekh, D., Poddar, N., Rajpurkar, A., Chahal, M., Kumar, N., Joshi, G. P., et al. (2022). A review on autonomous vehicles: progress, methods and challenges. Electronics 11 (14), 2162. doi:10.3390/electronics11142162
Petrović, Đ., Mijailović, R., and Pešić, D. (2020). Traffic accidents with autonomous vehicles: type of collisions, manoeuvres and errors of conventional vehicles’ drivers. Transp. Res. procedia 45, 161–168. doi:10.1016/j.trpro.2020.03.003
Pettigrew, S., Worrall, C., Talati, Z., Fritschi, L., and Norman, R. (2019). Dimensions of attitudes to autonomous vehicles. Urban, Plan. Transp. Res. 7, 19–33. doi:10.1080/21650020.2019.1604155
Qu, W., Xu, J., Ge, Y., Sun, X., and Zhang, K. (2019). Development and validation of a questionnaire to assess public receptivity toward autonomous vehicles and its relation with the traffic safety climate in China. Accid. Analysis Prev. 128, 78–86. doi:10.1016/j.aap.2019.04.006
Ren, W., Yu, B., Chen, Y., and Gao, K. (2022). Divergent effects of factors on crash severity under autonomous and conventional driving modes using a hierarchical Bayesian approach. Int. J. Environ. Res. public health 19 (18), 11358. doi:10.3390/ijerph191811358
Robinson, J., Smyth, J., Woodman, R., and Donzella, V. (2022). Ethical considerations and moral implications of autonomous vehicles and unavoidable collisions. Theor. issues ergonomics Sci. 23 (4), 435–452. doi:10.1080/1463922X.2021.1978013
Santoni de Sio, F. (2017). Killing by autonomous vehicles and the legal doctrine of necessity. Ethical Theory Moral Pract. 20, 411–429. doi:10.1007/s10677-017-9780-7
Schoettle, B., and Sivak, M. (2015). A preliminary analysis of real-world crashes involving self-driving vehicles. Ann Arbor, MI: University of Michigan Transportation Research Institute.
Sinha, A., Vu, V., Chand, S., Wijayaratna, K., and Dixit, V. (2021). A crash injury model involving autonomous vehicle: investigating of crash and disengagement reports. Sustainability 13 (14), 7938. doi:10.3390/su13147938
Song, Y., Chitturi, M. V., and Noyce, D. A. (2021). Automated vehicle crash sequences: patterns and potential uses in safety testing. Accid. Analysis Prev. 153, 106017. doi:10.1016/j.aap.2021.106017
Umbrello, S., and Yampolskiy, R. V. (2022). Designing AI for explainability and verifiability: a value sensitive design approach to avoid artificial stupidity in autonomous vehicles. Int. J. Soc. Robotics 14 (2), 313–322. doi:10.1007/s12369-021-00790-w
Wang, S., and Li, Z. (2019). Exploring the mechanism of crashes with automated vehicles using statistical modeling approaches. PloS one 14 (3), e0214550. doi:10.1371/journal.pone.0214550
Xing, Y., Zhou, H., Han, X., Zhang, M., and Lu, J. (2022). What influences vulnerable road users’ perceptions of autonomous vehicles? A comparative analysis of the 2017 and 2019 Pittsburgh surveys. Technol. Forecast. Soc. Change 176, 121454. doi:10.1016/j.techfore.2021.121454
Xu, C., Ding, Z., Wang, C., and Li, Z. (2019). Statistical analysis of the patterns and characteristics of connected and autonomous vehicle involved crashes. J. Saf. Res. 71, 41–47. doi:10.1016/j.jsr.2019.09.001
Ye, W., Wang, C., Chen, F., Yan, S., and Li, L. (2021). Approaching autonomous driving with cautious optimism: analysis of road traffic injuries involving autonomous vehicles based on field test data. Inj. Prev. 27 (1), 42–47. doi:10.1136/injuryprev-2019-043402
Yuan, Q., Xu, X., Wang, T., and Chen, Y. (2022). Investigating safety and liability of autonomous vehicles: bayesian random parameter ordered probit model analysis. J. intelligent connected Veh. 5 (3), 199–205. doi:10.1108/JICV-04-2022-0012
Zhang, J., and Xu, C. (2021). “Investigating the typical scenarios and contributory factors to crash severity of autonomous vehicle involved collisions using association rule analysis,” in Proceedings of the 100th Annual Meeting of the Transportation Research Board, Washington, DC, USA, 5–29.
Keywords: autonomous vehicles, transportation safety, accidents, policy, ethics
Citation: Almaskati D, Kermanshachi S and Pamidimukkala A (2024) Investigating the impacts of autonomous vehicles on crash severity and traffic safety. Front. Built Environ. 10:1383144. doi: 10.3389/fbuil.2024.1383144
Received: 06 February 2024; Accepted: 26 February 2024;
Published: 05 March 2024.
Edited by:
Sakdirat Kaewunruen, University of Birmingham, United KingdomReviewed by:
Mehmet Hamarat, Boğaziçi University, TürkiyeCopyright © 2024 Almaskati, Kermanshachi and Pamidimukkala. This is an open-access article distributed under the terms of the Creative Commons Attribution License (CC BY). The use, distribution or reproduction in other forums is permitted, provided the original author(s) and the copyright owner(s) are credited and that the original publication in this journal is cited, in accordance with accepted academic practice. No use, distribution or reproduction is permitted which does not comply with these terms.
*Correspondence: Sharareh Kermanshachi, c3ZrNTQ2NEBwc3UuZWR1
Disclaimer: All claims expressed in this article are solely those of the authors and do not necessarily represent those of their affiliated organizations, or those of the publisher, the editors and the reviewers. Any product that may be evaluated in this article or claim that may be made by its manufacturer is not guaranteed or endorsed by the publisher.
Research integrity at Frontiers
Learn more about the work of our research integrity team to safeguard the quality of each article we publish.