- 1Department of Civil Engineering, National Advanced School of Engineering of Yaoundé, The University of Yaoundé I, Yaoundé, Cameroon
- 2Faculty of Technology, Design and Environment, School of the Built Environment, Oxford Institute for Sustainable Development, Oxford Brookes University, Oxford, United Kingdom
The building environment is predominated almost everywhere in developing countries by fuzzy and unconventional mechanisms, which forms the so-called “informal sector.” The implementation of projects by the owners themselves, small and medium-sized enterprises, in this environment requires severe cost control and time delivery. The construction process in such a complex area is subject to labour fluctuations, productivity variability, difficulties in logistic supply, poor management, use of unsuitable methods, instability of financial means, and therefore delays in the payment of accounts by the contracting authorities. The factors mentioned above are source of major discrepancies between the forecast costs, quality, and deadlines and those obtained at the end of the project. This explains the losses in the execution phase and the prejudices suffered by the main actors in the unconventional construction industry at all levels. By comparing this specific construction sector with the standards, specific uncertainties emerge at several levels of understanding and execution, which, for each task, are the variabilities in quantity, performance, and qualification of workers; the quality and cost fluctuations among supplying networks of materials; the availability and/or inadequacy of equipment used; and the improvised managerial and financial mechanisms. This study, based on matrix modelling of the components of the construction site, leads to implementation of a heuristic approach for assessing those construction costs and delays uncertainties. We proposed analytic formulations of uncertainties that are computed every iteration of possible scenarios. By adapting and applying an iterative (TABU Search), a heuristic algorithm for minimising costs and lead times, it is possible to combine virtually all the possible choices for the different variables in order to anticipate the impact and guide the decision-maker towards the scenario with the least uncertainty and a good reliability of occurrence. Considering the complex parameters of this site of “informal” construction, the model aims to enable stakeholders in the construction sector to incorporate uncertainties into their forecasts before taking decisions not only to reduce or decrease financial losses but also to increase their credibility and ultimately improve this common practice of sustainable construction.
1 Introduction
The informal sector can be defined as the set of non-regulatory mechanisms that are used in developing countries to supply housing and infrastructure. It has specific characteristics in designing the project, material supply, recruitment, remuneration of labour during construction, and modes of financing which are essentially savings and tontines (Pettang, 1995; Pettang et al., 2016; Albertini et al., 2020). It is also often defined negatively as unofficial, unstructured, non-capitalist, and even illegal and clandestine (Jacquemot et al., 1993; Lautier, 2013). The informal sector of construction has emerged through spontaneous responses to various needs of populations in housing and infrastructure that the state cannot meet because of the ease to have resources (without a lot of rules and at low cost) in this sector.
In developing countries, the informal sector has helped support the development action of the state since many decades. In 1995, Pettang C. et al., estimated to 90% the part of the informal sector in housing construction in Cameroon. Nowadays, the informal sector of construction has been able to impose itself in the economies of developing countries and cannot be ignored in the development of effective economic strategies because of its importance despite the complexity.
The informal sector of construction more often develops itself because of the lack of financing and strong legal rules that lead individuals to find ways and means to reduce the cost and to circumvent the law. These ways and means, which are not really determined and regulated, create many uncertainties in construction resources (workforce, materials, and management). These uncertainties added to the fuzzy mechanisms create a very huge complexity in the whole construction sector. For Pettang et al. (2016) and Fandjio Yonzou et al. (2022), the informal sector, on which most companies rely, adds great complexity to the construction process on building sites. These combined uncertainties have a significant impact on the productivity and delivery of building infrastructure (Aibinu and Jagboro, 2002; Badr Alsolami, 2022), whose various and varied hazards make them complex (Meagher, 2011; Fandjio Yonzou et al., 2021).
Moreover, construction sites are then faced with (i) an unstable and fluctuating workforce with variable productivity, (ii) a complexity linked to the availability of materials and their modes and flows of supply, (iii) equipment whose acquisition and use are complex, (iv) implementation methods which are often unsuitable for the work, and (v) delays in the payment of accounts. It is therefore a field subject to multiple sources of uncertainty about the time and cost of construction work, thus creating a considerable brake on the growth of construction in most developing countries. This situation makes the site environment uncertain and random, stochastic. It is often the laws of probability that enable authors to address this type of problem. For example, as observed in Fan et al. (2018), in their work on developing a multivariate eco-hydrological risk-assessment framework based on the multivariate copula method in order to evaluate the occurrence of extreme eco-hydrological events in China. This can also be seen in the study of Acebes et al. (2022), which shows a purely probabilistic model for monitoring and controlling stochastic projects called the earned duration management (EDM) as an alternative to the commonly used earned value management (EVM). This approach is even more relevant when data are massively available, which is obviously not the case for the informal sector. What would be more appropriate is a continuous improvement approach to decisions taken before and during construction, to minimise decision-making errors. This is where the heuristic approach is widely used to tackle optimisation problems, as Hong et al. (2023)’s recent work on reliability topology optimization illustrates, where the method is based on the reliability-and-optimization decoupled model and teaching–learning-based optimization (TLBO) algorithm.
To improve the building environment in developing countries, complexity of the informal sector should be better mastered using mathematical tools to quantify variables and a heuristic algorithm to reduce their uncertainties. Since informal construction sector practice is deeply anchored in society, mastering these uncertainties could improve the systematic management of construction information for a building construction in developing countries, which would greatly benefit the whole construction industry (Adekunle et al., 2022).
Our model is developed from a methodological approach based on mathematical equations integrating the complex parameters of the informal sector construction site and validated by a case study conducted by our own experimental process. Data collection is based on documentary research and feedback from our construction sites monitored and/or carried out in the informal sector.
Section 2 presents the complexity of an informal construction site based on a literature review and feedback. This complexity generates uncertainties that correspond to the causes of errors in the main indicators of project performance, i.e., cost and time. Thus, a state-of-the-art concept review about cost and delay problems is presented in Section 3. Section 4 presents the methodology for assessing construction site uncertainties using heuristic exploration, on one hand, and matrix derivative calculation, on the other. Section 5 details a test of implementing the model and its potential importance for the different actors and beneficiaries of the construction project.
2 Presenting a construction site in the informal sector
The construction site, the site where construction work is carried out, is a set in a delimited space containing various components necessary for the realisation of the infrastructure, such as materials, equipment, human resources, and methods supported by means pooled in a precise environment, leading to a work under the constraints of predefined cost, time, and quality (Pettang et al., 2016). It is therefore a complex universe in its delimitation, its content, and its methods and mechanisms. Indeed, the construction site is influenced by multiple parameters such as the fluctuation of the workforce, the supply of materials, the variability of the methods used, and the instability of financial resources. Although in developed countries, most of these parameters are mastered a priori at the start of the construction process (WM Chan and M Kumaraswamy, 1997; Shakeel Faridi and Monir El-Sayegh, 2006; de Marcellis-Warin et al., 2014), in developing countries, it is difficult to control these elements because they are uncertain, vague, fluctuating, and even unstable and irrational in many cases (Abanda et al., 2011; Manjia et al., 2014; Pettang C. et al., 2016).
2.1 The problem of the workforce in developing countries and its complexity
In the construction sector in many countries such as Nigeria, Kenya, Cameroon, and Ghana, very few workers have conventional training obtained in technical and professional institutions. Among them, many have been trained by apprenticeship on the construction site without a professional diploma. This situation is more worrying as 90% of Cameroonian workers used on construction sites come from the informal sector (OIT, 2017).
For Maranatha et al. (2022), the informal sector is an increasing phenomenon in developing countries because it allows having a job without special conditions such as education level or job skills and concerns more often the construction sector, particularly the building environment. However, in the formal sector, especially in developed countries, the performance of workers in all trades is measurable and verifiable, and workers in developing countries follow their own rules and their productivity is linked to the environment in which they work. The variability of labour production is, thus, one of the sources of difficulty in managing and paying for labour (Abanda F. H. et al., 2011). Although labour is abundant in developing countries, the approximate qualification of the workforce, resulting in the shortage of skilled workers in the construction industry, is of much greater concern.
Labour control is an important aspect of productivity in the sector. However, it remains poorly controlled by many stakeholders in the informal construction sector. Indeed, one of the major problems in the sector is that of controlling the number of workers throughout the project (Challal, 2014; Kouamou et al., 2016). Currently, in building sites, control of this human resource is not easy: it often happens that a worker suddenly abandons his tasks on one site for another without a second thought, even if the project is at a critical stage. Kouamou et al. (2016) dealt with this difficulty by calculating the optimal number of workers and generating a dynamic schedule according to the number of workers present on the site. The likelihood of possible early departures from construction sites is justified by the insufficient organisation and administrative and legal regulation of the sector. Indeed, most of the workers have neither a contract of employment nor insurance, and they are paid on a daily, hourly, or fortnightly basis and, therefore, feel no permanent responsibility towards the company.
On the other hand, the poor evaluation of the necessary personnel leads to the insufficient or inadequate mobilisation of the trades, reducing the yield and quality of the work carried out. Furthermore, site accidents, generally due to poor working conditions and the use of inadequate materials for the execution of a task, considerably affect the time and cost of the project and make it more difficult to control the workforce.
Workforce, an essential resource for any construction company, is therefore an unstable and complex parameter. This element, being one of the pillars of the smooth running of construction sites, must be controlled if construction work is to be carried out within the constraints of costs and deadlines. Labour movements must be monitored and controlled. Unwanted departures of workers should be considered in the planning of the work.
2.2 The complexity of materials and their supply methods
The complexity of the building material element lies in the difficult access systematically to the right materials, the difficulties of supply, and the poor conditions of storage and implementation in the construction site. Difficulties in sourcing materials, which are more significant in enclosed areas, are generally due to problems of accessibility to the site (Fandjio Yonzou et al., 2021). Indeed, these areas are characterised by the poor state of access roads (roads are degraded and mud roads impassable during rainy periods), the absence or insufficient of quarries to produce quality materials, and the low stock of materials in hardware stores on site. The supply of materials is, therefore, made difficult because the vehicles transporting the materials must travel hundreds of kilometres to deliver them. This process is broken down into three phases: loading the material onto the truck, transporting it to the site, and then unloading it. Difficulties in the success of the process are much more frequent during the transport to the construction site and within the construction site (from their storage places to the different locations of the works to be built). Indeed, many stoppages are likely to occur during transport, leading to delivery delays and additional costs. These include the following:
1) Parking caused by stops on roads or tracks.
2) Traffic jams and drivers’ detours, increasing transportation times.
3) The poor quality of many tracks and roads which cause the vehicle to get stuck in impassable quagmires, as well as abnormal fuel consumption and rapid breakdown.
4) Road accidents due to either poor driving or impassable roads. In some cases, the vehicle may overturn and lead to considerable loss of materials (spillage of materials, theft, etc.) and time (waiting for a new vehicle to be loaded).
The reminder of these different situations shows that they are not so marginal. We should also point out that one of the risk factors in the supply of materials is the high demand, which often exceeds supply, on the part of suppliers, who often encounter difficulties in obtaining supplies on an ongoing basis. It should be noted that failure to meet the resupply deadlines leads to stock-outs and therefore to work stoppages.
On the construction site, badly ordered storage and poor conditioning of materials that can be attacked by atmosphere, such as steel or wood, make them less efficient or even unusable. The multiple risks to which materials are exposed on the building site:
1) Theft due to insecurity on the construction site.
2) Misuse and waste caused by lack of control and monitoring of materials.
3) Errors in calculating the quantities of materials used, which are generally higher than those expected due to inaccurate measurements and dosages during execution.
The movement of materials in the construction process is still uncontrolled as each stage of the process involves variations in quantities, leading to cost overruns and time overruns.
2.3 The complexity of equipment acquisition
Construction equipment generally falls into two categories, namely, small equipment—such as wheelbarrows, shovels, and vibrating needles—and large equipment—such as concrete mixers, lifting machines, and other heavy plant. It goes without saying that the lack or inadequacy of equipment, or the use of equipment by incompetent staff, leads to reduced productivity. The choice of equipment depends on financing and the methods prescribed for the construction of the works. In Cameroon, for example, and sure it should be the same for many other developing countries, the acquisition of large equipment poses some difficulties, which are as follows:
1) Insufficient supply due to the fact that specialised companies are rare, with the national civil engineering equipment park (MATGENIE) covering well under 40% of needs.
2) The costs of hiring and/or purchasing equipment are prohibitive and lead to the use of cheaper equipment, which is often in poor condition and unsuitable for the tasks to be carried out.
As for the supply of materials, the complexity is the same as for the abovementioned materials. It is especially difficult in remote areas.
The use of materials on site is also complex. Indeed, few informal sector sites have a workforce qualified to use equipment such as concrete mixers. The resulting misuse is accompanied by a serious lack of maintenance of the piece of equipment, which leads to breakdowns and interruptions. It should be noted that in the event of a breakdown, the search for an appropriate maintainer can also take several weeks, which prolongs the execution time. In addition, the absence of control of inputs and outputs leads to neglecting the piece of equipment by the workforce, abandonment of the piece of equipment under bad weather conditions which, when they occur repeatedly, damage the equipment, and reduction in the reuse coefficient.
2.4 Complexity related to management and executing methods
In their study on AI-based solutions for strengthening the informal construction sector mechanisms, Fandjio Yonzou et al. (2022) observed that management in this type of construction site is carried out by the owner who acts as the project manager, with the help of a fairly well-trained technician. The construction method is often imposed by these owners who build themselves based on a more or less relative logic (Manjia et al., 2011). The complexity lies in the choice of the construction method in accordance with the directives imposed by the client, which are described in the contractual documents, in particular the technical directives, the plan drawing, the schedule, and some constraints linked to the construction site. Not far from there, the choice of method must allow a good programming of the tasks and the elaboration of a schedule allowing the organisation of different tasks, with respect to the logic of implementation of the construction.
Some of the uncertainties affecting task scheduling include contract revision after the start of the work, which may result in significant changes. Weather conditions, hidden defects in the land, availability and supply of equipment, performance and availability of labour, and efficiency of materials are also factors of uncertainty when not initially mastered in previously well-conducted studies.
It is thus obvious that the choice of appropriate management and execution methods faces multiple difficulties, which are as follows:
1) The weak managerial capacity of small- and medium-sized enterprises.
2) The absence of suitable subcontractors on certain sites. It is often realised that the site manager is his own subcontractor for certain tasks.
3) The poor organisation of the workforce, leading to lowering.
4) The late acceptance of plans or their modification during implementation.
5) Hidden defects in the land, which may be phenomena that are never observed before.
Methods on a construction site are often based on the experience of the workers. Thus, they will be inappropriate in the face of new and unpredictable events. Moreover, as each structure is a unique sample, the choice of the construction method based on the experience of the workers alone is problematic and becomes a source of cost and time overruns. As the management method changes according to the location of the construction site, it imposes variability in the decision-making tools, concerning the choice of an appropriate management method.
2.5 The complexity of the financial mechanisms for conducting construction
The informal construction sector is characterised by low incomes, with the two main sources of finance, namely, tontine (55%), especially used in Cameroon in building financing mechanisms, and personal savings (45%) (Pettang C. et al., 2016). There is also a growing involvement of banks (Fandjio Yonzou et al., 2022) through credits. Whatever the case, it is often very difficult to gather sufficient financial resources at once to complete a construction project. Indeed, with almost no investment banks in the construction environment, contractors have little access to smooth management and continuous construction. It is, therefore, necessary to adopt a planning methodology appropriate to this difficulty.
Payment difficulties very frequent in government projects are also a critical factor in the construction process in developing countries as SMEs are sometimes forced to suspend work due to insufficient financial resources and/or non-payment of bills of exchange. The causes of payment difficulties are multiple, and we can mention the following:
1) The slowness in processing and settling the accounts by the project owner and the government contracting authority. This generally leads to a temporary halt in the work. In many cases, the company first uses its own resources to advance the project.
2) In many cases, the company first uses its own resources to move the project forward, and the delays in paying for the riders increase because the project owner is late in paying the costs of carrying out the additional work unforeseen in the initial contract.
3) High but often unclear taxes and customs duties make it impossible to plan exactly what to pay.
In addition, fluctuating prices for materials and equipment result in high project costs. This forces contractors to inflate the budget to cover both taxes and inflation. If they occur after a contract has been signed, then they will be a source of additional costs. Contractors then make their profits at the expense of quality and productivity.
As we have seen the components of construction, especially in the informal sector, it is appropriate to point out that the management of informal work sites does not always consider their linkages and interdependence, for example, funds acquired in the tontines depending on the level of possible indebtedness and not on the projected level of progress of the work. Another striking point is that the quantity of workers and equipment does not often correspond to a planned consumption of material and financial resources. Therefore, these components can be represented as branches of a tree, as shown in Figure 1.
Figure 1 shows that an informal work site is assimilated to a tree, so it follows the decision support method like the decision tree method and heuristic exploration. Indeed, Manjia et al. (2012) addressed the high complexity of the informal construction site through a combination of multiple linear regression and artificial neural network models that solved both the financial and technical aspects, and the managerial and qualitative aspects. This deterministic approach has been improved by introducing the Markov decision process with Manjia et al. (2014) who evaluated the potential gains and losses of alternative scenarios for a given action. This concept, which consists of reducing uncertainties by seeking the best choice among the various possible scenarios in the decision tree, is suitable for managing tree-based cost and delay problems in an uncertain environment. Heuristic exploration, therefore, appears to us to be an interesting tool for finding, branch by branch, the minimum value of the total uncertainty of the project corresponding to the best successive decisions.
3 Review of the concepts of cost and time uncertainties in a construction project
Conventionally, assessments of the costs and timescales of a project are made at different stages of the project. Challal (2014) referred to the analogical approach based exclusively on feedback which provides quick and low-cost results, such as target costs per square metre built which are used in preliminary studies and sketches. There are also parametric methods which are also based on experience but require extensive statistical calculation to arrive at reliable coefficients. Finally, the analytical methods make it possible to refine the accuracy of the costs at the preliminary design stage but require a lot of detailed information. The decomposition of the project into its different sub-structures and different trades allows the authors (Pettang C. et al., 1997; Louzolo-Kimbembé and Pettang, 2006; Manjia et al., 2010; 2011; 2012) to exploit the matrix approach to improve the efficiency and speed of cost calculations analytically. This matrix approach leads to the decomposition of total construction cost into three fundamental elements, namely, material, labour, and management means, which is represented as follows:
where
The principal sub-structures or sub-works are as follows:
SW1: excavation and setting; SW2: foundation and ground slab; SW3: elevation; SW4: roofing; SW5: ceiling; SW6: carpentry; SW7: sanitary plumbing; SW8: electricity; SW9: plastering; SW10: face work and tiling; SW11: painting.
Theoretically, a construction site can be fully simulated before it is built using BIM (Buhammood et al., 2020; Abanda F. H. et al., 2021). This allows for a view of the workflow, according to an envisaged scenario. The project would then be delivered on time and within budget exactly, as planned. The uncertainties inherent in the components of the project may lead to errors in decision-making that alter the initial assumptions. In the applied sciences, uncertainty is the recognition that there are no events that will occur perfectly as predicted (Quebec, 2015). It is the acceptance of possible errors during the implementation process. This concept, generally perceived as abstract and complex, has perceptible and concrete impacts in the fields of engineering and decision-making. In the context of construction sites, uncertainties correspond to the causes of positive or negative errors on the characteristic indicators of project performance: cost and time.
3.1 Investigating the causes of uncertainty in construction project costs
Iyer and Jha (2005) used a survey to assess the cost effects of project management factors. A principal component analysis was adopted to identify seven critical factors that could affect the achievement of good performance. According to this study, these factors included conflicts between project participants, technical deficiency of the project manager, complexity of the project, lack of adequate project definition, hostile socio-economic environment, a high level of competition in the tender process, and a lack of adequate time for the preparation of bids. A regression analysis finally confirmed that the factor with the greatest effect on project costs is the coordination between project participants.
Doloi (2013) selected 48 attributes associated with project cost performance in the Australian construction projects. Between them, it identified eight key factors which were brought out for an investigation of their quantitative impacts on project cost performance. The significant factors were accurate project planning and monitoring, effective site management, contractors’ efficiency, design efficiency, and communication.
Other authors (Frimpong and Oluwoye, 2003; Vu et al., 2016; Bilal et al., 2019; Liu, 2019; Ndunguru et al., 2020; Sohrabi and Noorzai, 2022) have also investigated independently the causes of the latter to describe and identify, in order of importance, the upstream positions where the most fear should be made. All these studies remain conceptual, where the relative contributions of the causes to each other are obtained, but there is still no mathematical tool to calculate these uncertainties or their interactions. Hanák and Vítková (2022) raised a critical assessment of the interaction of individual factors both at the level of the litigation categories and the project life cycle. Using a robust random forest (RF) regression model, S. Shoar et al. (2022) revealed three factors predictive of cost overruns for engineering services (the level of adoption of computer-aided design technologies, scope definition adequacy, and the level of communication among the project teams) in addition to the factors contract fee and project duration generally discussed in Vu H. A. et al. (2016).
Altogether, the recurring parameters in decision-making issues associated with the main uncertainties encountered on a construction site are summarised in Table 1.
3.2 Cost control and delay uncertainties
Through linear regression, Pettang et al. (2016) highlighted a confidence interval centred on the total cost estimation
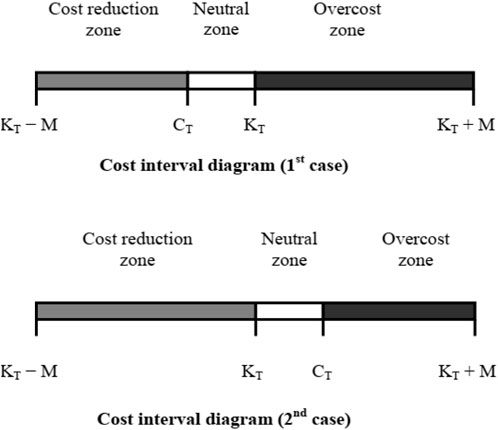
FIGURE 2. Cost interval diagram (Louzolo-Kimbembé P. and Pettang C., 2006).
M is the estimated deviation. At the same time, all the causes identified until now are reconducted for time overruns.
It is known that this approach does not consider some non-linear relations and qualitative considerations. It is the reason why construction complexity is obviously addressed with probabilistic and heuristic approaches. Manjia et al. (2014) used the powerful Markov decision processes to optimise construction site decision-making affected by uncertainties. Based on the method of inaccurate probabilities, this construction site management method guides to the least risky and most profitable choice.
The use of IT tools is essential to lighten the calculation of variations in costs and delays caused by previous enumerated factors (Bilal, 2019; Fandjio Yonzou et al., 2022; Sohrabi and Noorzai, 2022) as digital technologies, which are based on proven mathematical formulae (Hou et al., 2022). Thanks to linear optimization based on the calculation of workers’ yields, Kouamou et al. (2016) set up an enterprise resource planning (ERP) that allows, on one hand, to calculate the optimal size of the workforce and, on the other hand, to generate a dynamic work schedule, according to the available workforce. This approach was made more relevant by the comparative study between push planning and pull planning of Ghanem et al. (2022). Push planning, which uses predetermined dates to control a project, is less efficient in production than pull planning, which utilizes the system’s current state.
It is a well-known fact that system status is highly variable depending on the actual scenario in progress. It is often too late to realise that decisions taken at the start or during the project were the reason for the poor performance of one or more activities on the construction site. Unfortunately, it is not possible to have all the constraints and all the lines of information needed to make the best decision, but it is still imperative to make the right decision every time, to achieve one or more key objectives. This is an arduous decision problem addressed in operational research by several single-objective or multi-objective resolution algorithms. To solve such a problem by an intuitive approach, we have the heuristic method, where the structure of the complex computational problem can be interpreted and exploited intelligently to obtain a reasonable solution (Silver et al., 1980). This concept, which underlies the mathematics of complexity in searching for the solution space, has been the subject of several studies since the 1970s. Its evolution has been marked by the appearance of polynomial and non-polynomial problems (P-NP); then heuristic mechanisms for the resolution of problems addressed and finally metaheuristics for seeking the solution space to complex problems. For example, Joshi et al. (2023) predicted the compressive strength of high-performance concrete and fibre-reinforced high-strength self-compacting concrete by the hybridization of the standard sparrow search algorithm and rock hyraxes swarm optimization, respectively. Dealing with the sources of uncertainties associated with the physical state of the infrastructure, climate change, and the economy, Zhang et al. (2023) made a flexible decision-analysis tool for managers based on both multi-objective particle swarm optimization (MOPSO) and a non-dominated sorting genetic algorithm II (NSGA-II).
4 Methodology for estimating uncertainties in construction sites
Uncertainties are identified on the basis of real-life experiments, i.e., full-scale experiments on a building site, based on previous research studies described in the section below. Our observations are then supported by a review of the literature on the causes of cost and time overruns in the construction sector and on existing approaches to cost and time control in the informal environment in Cameroon, Nigeria, Tanzania, Zimbabwe, Vietnam, Malaysia, South Africa, Thailand, Canada, China, and India (Aibinu and Jagboro, 2002; Iyer and Jha 2005; de Marcellis-Warin et al., 2014; Pettang et al., 2016; Fan et al., 2018; Albertini et al., 2020; Badr Alsolami, 2022). The heuristic approach aims to optimize a method, for a fuzzy or random situation such as the informal construction sector, in which one of the most sensitive points is the evaluation of uncertainties. Indeed, mastering optimization of a construction site is thus the capacity of mastering uncertainties. The proposed model is based on the paradigm of improvement heuristics, which start from a complete solution and improve it by modifying one or more components at each iteration until the predetermined stopping condition is reached. The operations carried out in the algorithm are implemented in the matrix and differential calculations on the indicators, evidenced by the errors observed in some construction sites in the informal sector. This combined approach is justified by the fact that the smaller the uncertainty, the better the choice made. It leads to a decision support tool in a real site situation, where measuring the variables used to calculate these uncertainties is crucial at the task planning stage.
4.1 Equations for calculating uncertainties on the cost of a construction site
Drawing on the work of Pettang C. et al. (2016), we consider ancillary costs and taxes which are also likely to vary, according to the management, method processes, and financial mechanisms (Challal, 2014). We obtain the following equation:
with C representing the cost;
Cmat representing the cost of materials;
Clabour representing the cost of workforce;
CEq representing the cost of equipment;
Cmg representing the cost of management and construction methods; and
Cfm representing the cost of the financial mechanism.
This equation shows that the uncertainties in cost are mainly related to materials, labour, equipment, financial mechanisms, and management and construction methods. Then, we derive
The value of each parameter on which the uncertainty depends represents a decision-making choice in the project. Each of these choices is associated with an uncertainty value, which is part of the total uncertainty. The value of the total uncertainty will therefore depend on the scenario, which is made up of a series of successive decision-making choices. In heuristic exploration, this scenario constitutes an outline or path. There are realities at each site, which generate both constraints and a set of possible solutions. The right solution will, therefore, correspond to the scenario with the lowest total uncertainty and an acceptable risk or probability of failure 1-p. Based on existing historical facts, experience, and learning, it is subjective probabilities that will be used to approximate risks (1-p).
- Extremely likely (0.80
- Very likely (0.6
- Probable (0.3
- Unlikely (0
A construction project transcribed in the matrix form is considered with the following sets: materials (Mat), labour (Lab), equipment (Eq), management (Mg), and financial mechanism (Fm).
At any construction stage, the problem can be formulated as find subsets 5-uplets (x1,x2,x3,x4,x5) = s.
S
The proposed algorithm is modelled on the TABOU SEARCH ALGORITHM, which works as follows:
1) Initialisation:
The s0 initial solution matrix is defined by
Matrices calculation corresponding to the chosen solution s.
Calculate the uncertainty of each component ∆CMat, ∆C Lab, ∆C Eq, ∆C Mg, and ∆C Fm.
Deduce the total uncertainty ∆C0 = ∑∆C and reliability p (s0) = ∏p.
2) Generate a subset of solutions s' in the s vicinity
s’
If f(s') < ∆Cmin and p(s)
End if.
Update T.
3) If stop condition not met, return to step 2.
In practice, the stopping condition corresponds to the number of iterations without any improvement in s* or to a time limit after which the search must stop.
The tabu list T has a fixed length k, containing the last k solutions visited, which means that a solution that has already been found cannot be accepted and stored in T. The new solution for the next iteration is then chosen from a set of neighbouring solutions outside the elements of this tabu list. When the number k is reached, each new solution selected replaces the oldest in the list (FIFO principle).
The key success factor of the process is the suitable definition of the minimisation function f = ∆C as it follows.
4.1.1 Calculation of material-related cost uncertainties
The cost of a material in a construction site is defined by
with Q representing the quantity of material.
PU represents its unit price, and
The uncertainty about the cost of materials is, therefore,
In the matrix form, it is represented as follows:
N is the number of tasks involved, and m is the number of materials.
The cost of supplying any material is the cost of transporting the material from the supplier to the site. This cost is generally made up of a fixed term and a variable term. The materials in the project must be procured from the geolocated supplier
The cost is formulated as follows:
For each task,
4.1.2 Calculations of labour-related cost uncertainties
Labour uncertainty is measured by the observed difference between the amount of labour planned and the amount of labour performed, but also by variations in the daily outputs of the latter. As a result, labour-related cost uncertainties are expressed as
According to derivation rules, the first term is calculated assuming a constant yield, and conversely, the second term is calculated assuming a constant number of workers. Thus, the first term of uncertainty on the cost corresponds to the following difference:
with
In the matrix form, the uncertainty is written as
where n is the total number of engaged tasks;
T is the number of trades required to complete the tasks;
As the number of workers is supposedly known and fixed for the second term of uncertainty on the cost, the overrun is due to the increase in the number of working hours to be paid caused by the decreases in daily outputs. We obtain the following equation:
4.1.3 Calculation of the equipment-related cost uncertainty
The additional costs due to the piece of equipment correspond mainly to their acquisition or maintenance costs in the event of breakdown. In the case of rentals, the uncertainty on the cost is due to the variation in the expected rental period. This variation is induced by an overrun in the execution of the work.
4.1.4 Consideration of other parameters
Methods are a major part of the cost overruns of works. Indeed, they contribute to 18% of the cost uncertainties (Pettang C. et al., 2016).
Construction site management methods and financial resources cause uncertainty in the cost of construction sites but are although not included in the selling price. In developing countries, delays in the payment of accounts are frequent. The executing companies, obliged to meet the deadline, use their own funds or, more frequently, resort to loans with interest from banks committed to monitoring the project and financing it in the event of late payment.
In the case of this study, we will assume the following:
Assumption 1. construction project experiences a constant delay in payment, d months.
Assumption 2. organization lends 70% of the estimated cash payment at interest.
Assumption 3. creditor’s monthly interest rate is approximately α%.
These assumptions allow us to have the expression of the loan:
4.2 Equations for calculating uncertainties in delays
The lead time of a construction site is the sum of the completion times of the different tasks. Uncertainties in the time frame are related to the activities of the workforce on the site, material procurement processes, payment delays, and poor labour management. The modified Tabu Search algorithm has also been implemented herein, substituting delay for cost.
4.2.1 Calculation of delay uncertainties related to the supply of materials
In estimating material supply times, one must consider uncertain stoppages that can have important consequences. These include stops due to rain during the journey on a dirt road or the breaking of a bridge (Fandjio Yonzou et al., 2021). In case of rain on
The waiting time must consider the various situations where clay roads are blocked by rainwater. According to experience, it varies on average between 12 and 36 h.
In the event of
Based on these intervals, the uncertainty of the delay due to possible stoppages during the supply of materials is defined by the framework:
4.2.2 Calculation of labour-related uncertainties in the delay
Manpower influences the delay through its presence on site and its performance. Labour-related lead time uncertainties are given by
Consider a trade intended to perform a daily task. Given the output r, the quantities of work q, and the number of workers x, the number of days needed to complete the work is given by
Since the quantities of work and outputs are assumed to be fixed, the variation in the number of hours required to complete the work is given by
The equation for determining the coefficients of the hourly variation matrix is represented as follows:
where
-
-
The daily output of a trade is defined by
This leads to the expression of the runtime error:
In general, we obtain the following equation:
4.2.3 Consideration of other parameters
Other factors of site complexity can be a source of uncertainty on the deadline, such as delays in the payment of accounts, poor site management, and unsuitable methods used. Every construction contractor that follows the conditions of eligibility for bank loan faced a constant period for the loan file to be successful, depending on the bank involved. The impact of this duration on deadlines can be assumed to be zero if the contractor acts early enough.
5 Case application and results
This section is intended as a point of experimentation using the methodology developed. The model has been applied to a construction project of a 1250-m2 multisport gymnasium at Bafoussam town, Cameroon’s third largest city, whose architectural plans are shown in Figure 4.
Based on the structural calculations and general construction procedures adopted, the following site indicator matrix of quantities has been drawn up (Figure 5). Each row represents a sub-work (SW), and each column is the quantity of materials associated with the sub-structures.
Table 2 and Table 3 highlight extracts of information on potential suppliers and potential workforces that may be involved during construction. We realise that we have a maximum of 5,400 possible scenarios for choosing a set of combinations (material, supplier) at each stage.
In addition, we have 1,024 possible scenarios of choosing a team workforce of each trade. Combined with the previous scenarios, the maximum number of iterations is 5,529,600 to reach at the optimal solution.
For the programmed tasks, the heuristic algorithm starts by generating a random selection of the materials and labour options.
The supplier (price, delivery time, and efficiency) and teamwork are found (RDMT, MO, and reliability).
This minimizes uncertainties ∆C or ∆T.
It is then subjected to the constraint that the supplier and teamwork belong to the database.
The principle of refining a local optimum is that at the (i+1)-th iteration,
Update Tabu list with supplier(i) and teamwork(i)
When uncertainty (i+1)< uncertainty(i).
For construction stage 1 corresponding to earthworks (SW1) and footings and anchors (SW2), we have the following results.
Running the Tabu search algorithm, it is possible to explore millions of possible scenarios, calculating the uncertainty and displaying the relative uncertainty on Figure 6.
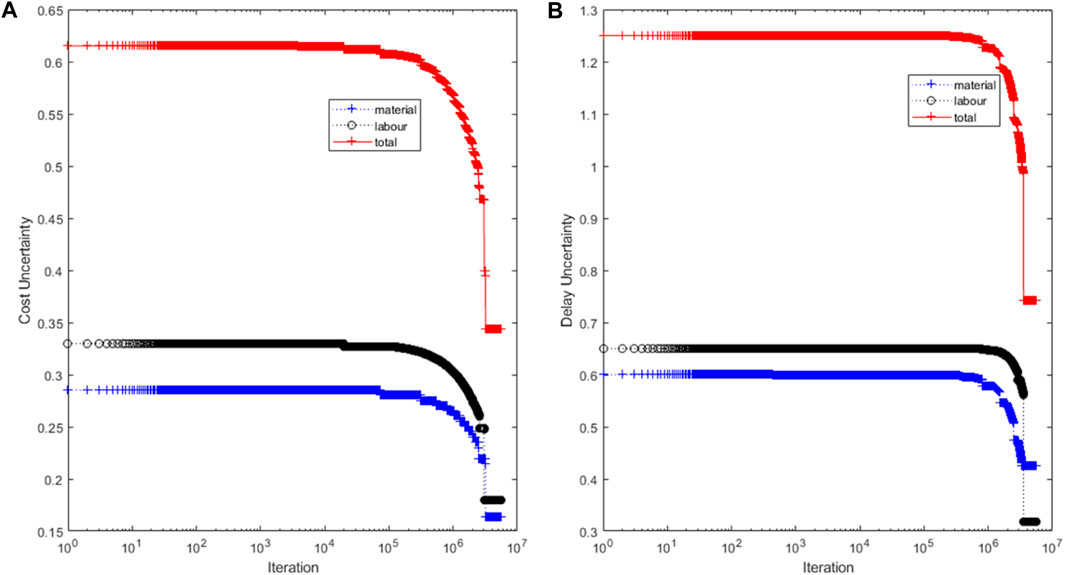
FIGURE 6. Convergence process of the relative uncertainties at construction stage 1, (A) cost uncertainty, and (B) delay uncertainty.
Convergence was achieved here after the 2,404,357-th iteration. A maximum relative uncertainty of 61.52% was recorded for the cost, while the minimum total uncertainty was 34.4%. The uncertainty over the lead time also varied considerably, from 125% to 74%, demonstrating that it is indeed possible to limit uncertainties by making the best choices of suppliers and work teams, as summarised in Table 4.
The same operation is carried out for the subsequent phases of construction, construction stage 2 (paving
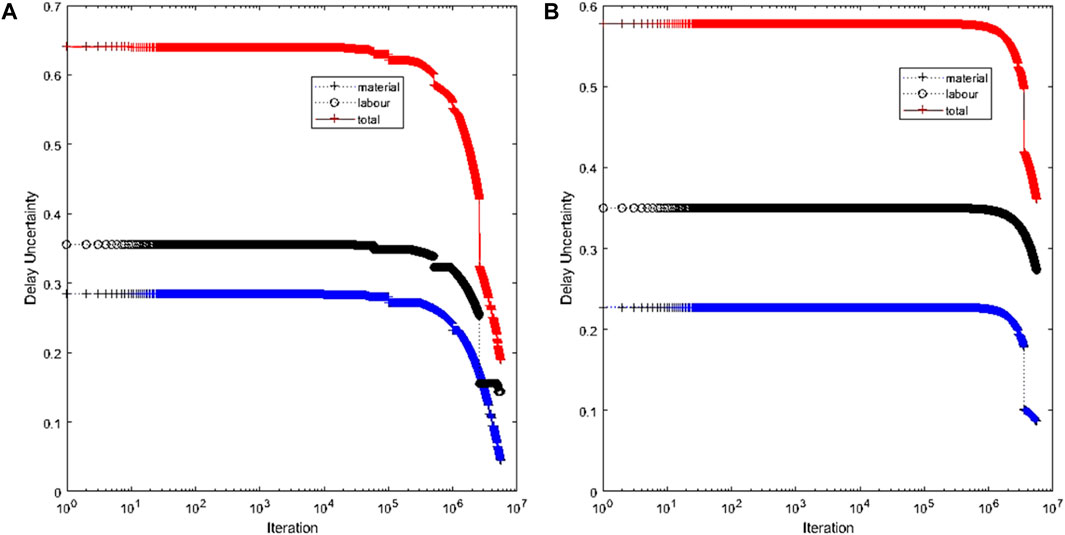
FIGURE 7. Convergence process of the relative uncertainty at construction stage 2, (A) cost uncertainty and (B) delay uncertainty.
As previously, the convergence of the best choices for construction stages 3 and 4 is illustrated in Figure 8 and Figure 9. Table 5 below resumes these choices for construction stages 2, 3, and 4.
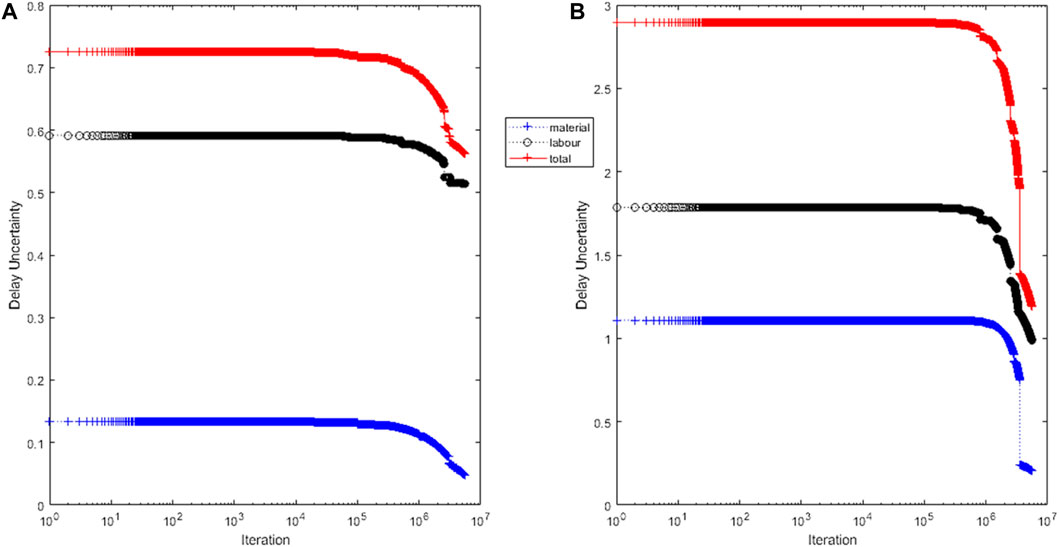
FIGURE 8. Convergence process of the relative uncertainty at construction stage 3, (A) cost uncertainty, and (B) delay uncertainty.
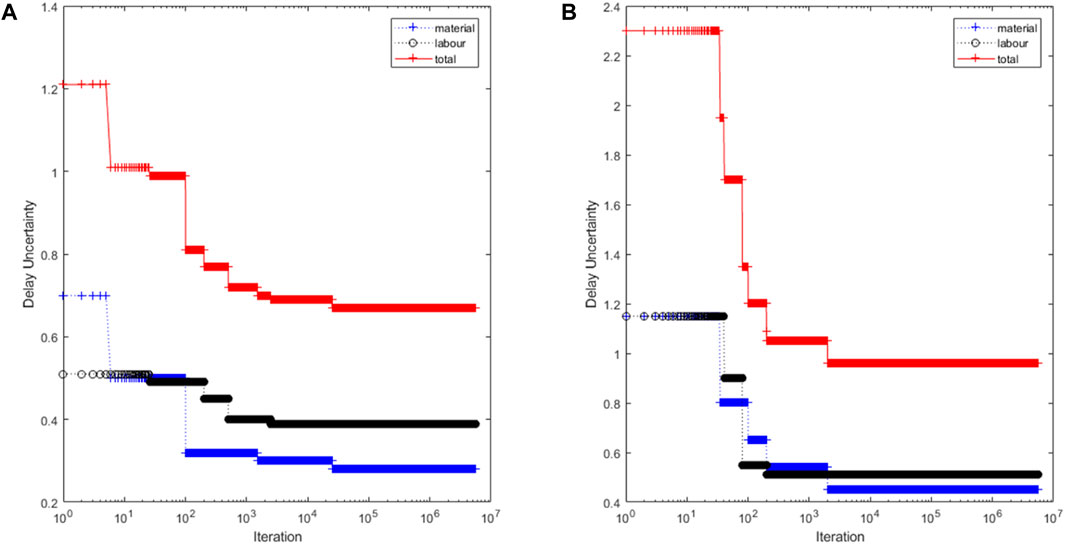
FIGURE 9. Convergence process of the relative uncertainty at construction stage 4, (A) cost uncertainty, and (B) delay uncertainty.
Let us note that the equipment was available and in good condition,
Also funding available, a bank completely financed the project
For the deployed management, we were working with a management and support team that was highly experienced in construction and management, and constantly communicating via social and mobile networks
In practice, we implemented each prediction with an aim in making the best possible choice to guarantee the best scenario for carrying out the planned activities. It is not a question of having the exact value of the extra cost and/or time overrun to come but mainly the minimum possible disorder since the informal sector is impossible to perfectly master with any certainty. It is the very nature of human behaviour that is erratic and divergent, which is sometimes irrational. These results are even more relevant as the uncertainties calculated per construction stage allow the contractor to address personalised solutions to each task to better control the complex environment related to it.
However, running the site as safely as possible has increased our chances of meeting deadlines and costs in the construction process. The actual site is shown in the photographs in Figure 10. Furthermore, it would be interesting to go beyond the simplifying hypotheses that limit the model, in particular the control of the uncertainties linked to the piece of equipment or funding.
6 Conclusion and perspectives
The construction sector has a pronounced randomness, with the various variables multiplying the uncertainties that need to be taken into account. These uncertainties are magnified in developing countries where the informal sector predominates. This work focused on the informal construction environment that governs 85% of projects in developing countries such as Cameroon. After presenting the sources of uncertainty common to informal building sites, we have put these uncertainties into equations under certain simplifying assumptions. We therefore propose a mixed approach based on the heuristic method and matrix and differential equations for the efficient control of uncertainties. Based on matrix calculations, this work led to the development of a model, assessing the impact of these uncertainties on the costs and delays which are the most significant parameters in the construction environment. This work integrates the envisaged fluctuations in materials, labour, and other parameters of the different tasks of the project in making their relative order of magnitude visible, to measure their amplitude, especially for every possible scenario of choosing the supplier and workforce. This optimization approach was based on the Tabu search algorithm which explores scenarios by seeking to minimise the uncertainties. This was applied to the example of the Bafoussam multisport gymnasium. We were able to reduce uncertainties from 61.52% to 34% for costs and from 125% to 74% for deadlines. The model would be used by both contractors and self-builders to help them in the decision-making process. This increase is not only the credibility of builders but also the confidence of forecasting times that could finally improve bad practices that have become common in the informal construction environment. The difficulty involved in the preliminary computations of the model can easily be overcome with the assistance of IT. Our main concern, apart from developing this model, is to collect more data on the errors and deviations observed on the construction sites and to improve the reliability of the predictions. In the order by organising a training course for contributing actors, a great improvement could be made in the construction sector and help well controlling, economic by realising good achievements in infrastructures.
Data availability statement
The raw data supporting the conclusion of this article will be made available by the authors, without undue reservation.
Author contributions
UP: conceptualization, methodology, validation, writing–original draft, and writing–review and editing. CF: formal analysis, methodology, software, and writing–original draft. PM: investigation and writing–original draft. FA: writing–review and editing, data curation, and validation. CP: writing–review and editing, conceptualization, project administration, resources, and supervision.
Funding
The author(s) declare that no financial support was received for the research, authorship, and/or publication of this article.
Acknowledgments
The authors acknowledge the contractor COMPLEXE OMEGAC CAM CONSTRUCTION for providing a platform for applying the theoretical concepts developed into real-life situations.
Conflict of interest
The authors declare that the research was conducted in the absence of any commercial or financial relationships that could be construed as a potential conflict of interest.
Publisher’s note
All claims expressed in this article are solely those of the authors and do not necessarily represent those of their affiliated organizations, or those of the publisher, the editors, and the reviewers. Any product that may be evaluated in this article, or claim that may be made by its manufacturer, is not guaranteed or endorsed by the publisher.
References
Abanda, F. H., Sibilla, M., Garstecki, P., and Anteneh, B. (2021). A literature review on BIM for cities distributed renewable and interactive energy systems. Int. J. Urban Sustain. Dev. 13 (2), 214–232. doi:10.1080/19463138.2020.1865971
Abanda, F. H., Tah, J. H. M., Pettang, C., and Manjia, M. B. (2011). An ontology-driven building construction labour cost estimation in Cameroon. J. Inf. Technol. Constr. (ITcon) 16 (35), 617–634.
Acebes, F., Poza, D., González-Varona, J. M., and López-Paredes, A. (2022). Stochastic earned duration analysis for project schedule management. Engineering 9 (2022), 148–161. doi:10.1016/j.eng.2021.07.019
Adekunle, P., Aigbavboa, C., Akinradewo, O., Oke, A., and Aghimien, D. (2022). Construction information management: benefits to the construction industry. Sustainability 14, 11366. doi:10.3390/su141811366
Aibinu, A. A., and Jagboro, G. O. (2002). The effects of construction delays on project delivery in Nigerian construction industry. Int. J. Proj. Manag. 20 (8), 593–599. doi:10.1016/S0263-7863(02)00028-5
Albertini, J., Ismail, K., Poirier, A., and Terriau, A. (2020). Informal work in developing countries: a review of the literature. Fr. J. Econ. 2020, 139–182. doi:10.3917/rfe.201.0139
Badr Alsolami, M. (2022). Identifying and assessing critical success factors of value management implementation in Saudi Arabia building construction industry. Ain Shams Eng. J. 13 (6), 101804. doi:10.1016/j.asej.2022.101804
Bilal, M., Oyedele, L. O., Kusimo, H. O., Owolabi, H. A., Akanbi, L. A., Ajayi, A. O., et al. (2019). Investigating profitability performance of construction projects using big data: a project analytics approach. J. Build. Eng. 26, 100850. doi:10.1016/j.jobe.2019.100850
Buhammood, A. H., Abanda, F. H., Garstecki, P., Manjia, M. B., Pettang, C., and Abdullahi, A. M. (2020). Coupling BIM and game engine technologies for construction knowledge enhancement. Int. J. Gaming Comput. Mediat. Simulations 12, 38–63. doi:10.4018/ijgcms.2020100103
Challal, A. (2014). Conception d'un modèle d'estimation des coûts de projets de construction. La Rev. Sci. Gest. 267-268, 105–113. doi:10.3917/rsg.267.0105
Charitopoulos, V. M., Papageorgiou, L. G., and Dua, V. (2017). Nonlinear model-based process operation under uncertainty using exact parametric programming. Engineering 3 (2017), 202–213. doi:10.1016/J.ENG.2017.02.008
Deep, S., Singh, D., and Ahmad, S. (2017). A review of contract awards to lowest bidder in Indian construction projects via case based approach. Open J. Bus. Manag. 5, 159–168. doi:10.4236/ojbm.2017.51015
de Marcellis-Warin, N., Peignier, I., Leenhouts, R., Teodoresco, S., and des Chênes, M. (2014). Etude des facteurs de risques de dépassements de coûts dans les projets de construction de routes et de grands travaux au Québec. https://cirano.qc.ca/fr/sommaires/2014RP-13.
Doloi, H. (2013). Cost overruns and failure in project management: understanding the roles of key stakeholders in construction projects. J. Constr. Eng. Manag. 139, 267–279. doi:10.1061/(ASCE)CO.1943-7862.0000621
Fan, Y., Huang, G., Zhang, Y., and Li, Y. (2018). Uncertainty quantification for multivariate eco-hydrological risk in the xiangxi river within the three gorges reservoir area in China. Engineering 4 (2018), 617–626. doi:10.1016/j.eng.2018.06.006
Fandjio Yonzou, C. C., Pettang Nana, U. J. M., Manjia, M. B., and Pettang, C. (2021). Contribution to optimization of supplies programming of construction sites located in enclosed areas: case of Cameroon. WJERT 7 (4), 292–305.
Fandjio YonzouMbiada Mbiada, C. C. P. J., Nana Pettang, U. J. M., Manjia, M. B., Kouamou, G. E., and Pettang, C. (2022). Extending an ERP to improve decision-making in civil engineering companies in developing countries such as Cameroon. J. Decis. Syst. 25, 550–557. doi:10.1080/12460125.2016.1187425
Frimpong, Y., and Oluwoye, J. (2003). Significant factors causing delay and cost overruns in construction of groundwater projects in Ghana. J. Constr. Res. 04, 175–187. doi:10.1142/S1609945103000418
GhanemHamzeh, M. F., Seppänen, O., Shehab, L., and Zankoul, E. (2022). Pull planning versus push planning: investigating impacts on crew performance from a locationbased perspective. Front. Built Environ. 8, 980023. doi:10.3389/fbuil.2022.980023
Hanák, T., and Vítková, E. (2022). Causes and effects of contract management problems: case study of road construction. Front. Built Environ. 8, 1009944. doi:10.3389/fbuil.2022.1009944
Hong, Z., Jiang, X., Feng, Y., Tian, Q., and Tan, J. (2023). Reliability topology optimization of collaborative design for complex products under uncertainties based on the TLBO algorithm. Engineering 22 (2023), 71–81. doi:10.1016/j.eng.2021.06.027
Hou, L., Chi, H.-L., and Tan, Y. (2022). Editorial: digital technologies for construction management. Front. Built Environ. 8, 1017547. doi:10.3389/fbuil.2022.1017547
Iyer, K. C., and Jha, K. N. (2005). Factors affecting cost performance: evidence from Indian construction projects. Int. J. Proj. Manag. 23, 283–295. doi:10.1016/j.ijproman.2004.10.003
Jongo, J. S., Tesha, D. N. G. A. K., Teyanga, J. J., Mufaddal, G. H., and Sylvanus, M. S. N (2019). Factors affecting performance and time schedule for multi-unit residential building construction in Dar-es-Salaam, Tanzania. PARIPEX - INDIAN J. Res. 8 (1), 78–91.
Joshi, D. A., Radhika Menon, R. K. J., and Kulkarni, A. V. (2023). Deep learning based concrete compressive strength prediction model with hybrid meta-heuristic approach. Expert Syst. Appl. 233, 120925. doi:10.1016/j.eswa.2023.120925
Kouamou, G. E., Manjia, M. B., and Pettang, C. (2016). Extending an ERP to improve decision-making in civil engineering companies in developing countries such as Cameroon. J. Decis. Syst. 25 (1), 550–557. doi:10.1080/12460125.2016.1187425
Lautier, B. (2013). Education in underdeveloped countries. Third World Rev. 2013/2 (n°214) 214, 151–167. doi:10.3917/rtm.214.0151
Liu, S. Y., Wu, J. Y., Yue, Y., and Zhang, Y. Q. (2019). Analysis of factors affecting the hidden costs of construction projects factor analysis. Open Access Libr. J. 6, 1–8. doi:10.4236/oalib.1105665
Louzolo-Kimbembé, P., and Pettang, C. (2006). Contribution to the amelioration of the estimation method of construction costs’ mastering in developing countries. Int. J. Archit. Sci. 7 (1), 14–25.
Manjia, M. B., Abanda, F. H., and Pettang, C. (2014). “Using Markov decision process for construction site management in Cameroon,” in Proceedings of IFIP WG8.3 and ASIS SIG DSS Conference, France, June 2014, 2–5.
Manjia, M. B., Kedang, V. F., Louzolo-Kimbembe, P., and Pettang, C. (2010). A decision support tool for cost mastering in urban self-construction: the case of Cameroon. Open Constr. Build. Technol. J. 4 (1), 17–28. doi:10.2174/18748368010040100017
Manjia, M. B., Kouamou, G. E., and Pettang, C. (2012). Labor cost modeling to reduce project risk in urban self-building in Cameroon. Fusing Decis. Support Syst. into Fabr. Context, 365–376.
Manjia, M. B., Pettang, C., and Abanda, F. H. (2011). Urban self-building labour cost modelling in Cameroon. J. Constr. Dev. Ctries. 16 (2), 69–90.
Maranatha, W., Kukuh, L., Ellysa, N., and Dimas, I. L. (2022). The effect of economical phenomenon on informal construction workers earnings within Covid-19 pandemic: a mixed method analysis. Helyon 8 (8), e10321. doi:10.1016/j.heliyon.2022.e10321
Meagher, K. (2011). Identity economics: social Networks and the informal economy in Nigeria. Polit. Afr. 2011/2 (n°122), 199–216.
NdunguruNiyonyungu, D. F., and Yang, X. (2020). Quantification of the influence of factors causing time and cost overruns in Tanzanian construction projects. Open J. Bus. Manag. 8, 2133–2147. doi:10.4236/ojbm.2020.85130
Obodo, C., Xie, Z., Cobbinah, B., and Yari, K. (2021). Evaluating the factors affecting contractors tender for project construction: an empirical study of small scale indigenous contractors in awka, Nigeria. Open J. Soc. Sci. 9, 381–397. doi:10.4236/jss.2021.97028
OIT (2017). Enquête auprès des entreprises informelles du Cameroun. 164p. Sous la direction de Benjamin Fomba Kamga; Bureau international du Travail, Équipe d’Appui Technique de l’OIT au travail décent pour l’Afrique centrale. https://www.ilo.org/wcmsp5/groups/public/---africa/---ro-abidjan/---sro-yaounde/documents/publication/wcms_625014.pdf.
Pettang, C., Manjia, M. B., and Abanda, F. H. (2016). Decision support for construction cost control in developing countries. Hershey, PA: IGI Global, 1–325. doi:10.4018/978-1-4666-9873-4
Pettang, C., Mbumbia, L., and Foudjet, A. (1997). Estimating building matérials cost in urban housing construction projects, based on matrix calculation: the case of Cameroon. Constructions and Building Materials. Amsterdam, Netherlands: Elevier Science Ltd, 47–55.
Pettang, C., and Vermande, P. (1995). L’impact du secteur informel dans la production de l’habitat au Cameroun. Cah. Sci. Hum. 31 (4), 883–903.
Québec, I. (2015). Guide méthodologique – La gestion des risques dans les grands projets d’infrastructure publique. http://www.bv.transports.gouv.qc.ca/mono/consultéle15/06/2020.
Shakeel Faridi, A., and Monir El-Sayegh, S. (2006). Significant factors causing delay in the UAE construction industry. Constr. Manag. Econ. 24 (11), 1167–1176. doi:10.1080/01446190600827033
Shoar, S., Chileshe, N., and Edwards, J. D. (2022). Machine learning-aided engineering services’ cost overruns prediction in high-rise residential building projects: application of random forest regression. J. Build. Eng. 50 (2022), 104102. doi:10.1016/j.jobe.2022.104102
Silver, E. A., Victor, R., Vidal, V., and de Werra, D. (1980). A tutorial on heuristic methods. Eur. J. Operational Res. 5 (3), 153–162. doi:10.1016/0377-2217(80)90084-3
Sohrabi, H., and Noorzai, E. (2022). Risk-supported case-based reasoning approach for cost overrun estimation of water-related projects using machine learning. Eng. Constr. Archit. Manag., doi:10.1108/ECAM-05-2022-0450
Tawanda, S. (2015). “An investigation of the causes and effects of construction delays on zimbabwean construction projects”. Bulawayo, ZIMBABWE: Department of Quantity Surveying, Faculty of The Built Environment, National University of Science and Technology, 80.
Vu, H. A., Wang, J., Min, L., Mai, S., and Nguyen, H. (2016). Research on cost overrun risk of construction phase of vietnam highway international contracting project. Engineering 8, 86–98. doi:10.4236/eng.2016.83011
Wm Chan, D., and Kumaraswamy, M. (1997). A comparative study of causes of time overruns in Hong Kong construction projects. Int. J. Proj. Manag. 15 (1), 55–63. doi:10.1016/S0263-7863(96)00039-7
Keywords: fuzzy environment, construction informal sector, uncertainties, heuristic approach, delays, costs
Citation: Pettang Nana UJM, Fandjio Yonzou CC, Mbiada Mbiada PJ, Abanda FH and Pettang C (2024) A heuristic approach of modelling developing countries' construction sector uncertainties to improve the building environment. Front. Built Environ. 10:1302467. doi: 10.3389/fbuil.2024.1302467
Received: 26 September 2023; Accepted: 08 January 2024;
Published: 08 February 2024.
Edited by:
Amir Mahdiyar, University of Science Malaysia, MalaysiaReviewed by:
Muneera Esa, University of Science Malaysia (USM), MalaysiaNehal Elshaboury, Housing and Building National Research Center, Egypt
Copyright © 2024 Pettang Nana, Fandjio Yonzou, Mbiada Mbiada, Abanda and Pettang. This is an open-access article distributed under the terms of the Creative Commons Attribution License (CC BY). The use, distribution or reproduction in other forums is permitted, provided the original author(s) and the copyright owner(s) are credited and that the original publication in this journal is cited, in accordance with accepted academic practice. No use, distribution or reproduction is permitted which does not comply with these terms.
*Correspondence: Ursula Joyce Merveilles Pettang Nana, pettangursu@gmail.com; Cédric Cabral Fandjio Yonzou, cabralfandjio@outlook.fr