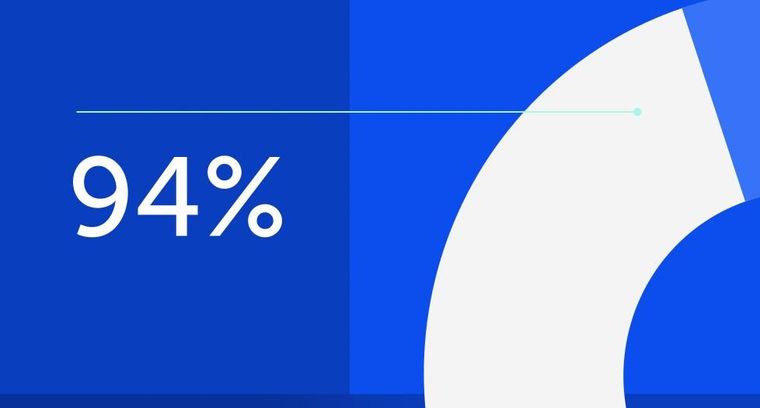
94% of researchers rate our articles as excellent or good
Learn more about the work of our research integrity team to safeguard the quality of each article we publish.
Find out more
EDITORIAL article
Front. Built Environ., 18 March 2022
Sec. Earthquake Engineering
Volume 8 - 2022 | https://doi.org/10.3389/fbuil.2022.876077
This article is part of the Research TopicArtificial Intelligence-powered Methodologies and Applications in Earthquake and Structural EngineeringView all 5 articles
Editorial on the Research Topic
Artificial Intelligence-Powered Methodologies and Applications in Earthquake and Structural Engineering
Earthquake disasters have caused enormous casualties and economic losses so far, threatening the social and economic development of humanity. At present, artificial intelligence (AI) is one of the frontiers and central issues in both academic research and engineering practice. AI refers to the branch of computer science that develops machines and software with human-like intelligence. In recent years, AI techniques are developing rapidly and have been widely adopted in several engineering disciplines. Among the different AI techniques, machine learning (ML), pattern recognition (PR), and deep learning (DL) have recently acquired considerable attention and are establishing themselves as a new class of powerful intelligent methods for use in the earthquake and structural engineering with proven effectiveness, as shown in recent studies. In the future, with the improvement of computational power and data accumulation, the feasibility and necessity of AI-driven technologies are expected to grow quickly. The Research Topic “Artificial Intelligence-Powered Methodologies and Applications in Earthquake and Structural Engineering” was proposed to collect cutting-edge research works combining AI with various scientific fields, such as seismic ground motion studies, structural and city-scale seismic risk, computational methods in structural engineering, structural system identification and damage detection, structural control under seismic action, structural health monitoring, among others.
Following these motivations, four papers have been selected and published in this Research Topic following a detailed peer-review process. These papers can be divided into two categories. Three of them employed convolutional neural networks (CNN) to obtain the high dimensional features of time-series data, to establish the mapping rules between the time-series input and the consequences/types of the shaking. Another paper used AI as a surrogate model to reduce the computational cost of physics-based modelling. Interestingly, these two categories cover the widest application fields of AI in the related disciplines.
Bamer et al. presented a new Monte Carlo simulation method enhanced by CNN and applied in non-linear structural dynamics. The sample set used for this Monte Carlo approach was provided by artificially generating site-dependent ground motion time histories using a non-linear Kanai-Tajimi filter. The CNN learns to extract the relevant input features and the structural response behaviour autonomously from the entire time histories, instead of learning from a set of hand-chosen intensity inputs. Training the neural network based on a chosen input sample set develops a meta-model that is, then used to predict the response of the total Monte Carlo sample set. This paper presents two CNN-enhanced strategies that allow for a practical design approach of seismically excited structures. The first strategy enables an accurate response prediction around the mean of the distribution. It is, therefore, useful regarding structural serviceability. The second strategy enables an accurate prediction around the tail end of the distribution. It is, therefore, beneficial for the prediction of the probability of failure.
Liao et al. proposed a crowdsensing-oriented vibration acquisition and identification method based on the input of time–frequency domain characteristics and deep transfer learning. The methodology can distinguish the responses of buildings during an earthquake event from vibration under serviceability conditions. The core classification process was performed using a combination of wavelet transforms and deep transfer networks. The latter were pre-trained using finite element models calibrated with the monitored seismic responses of the structures. The validation study confirmed the superior identification accuracy of the proposed method.
Sheibani and Ou proposed a data-driven method that runs the nonlinear time-history analysis for the distinct structures exclusively and inferred the damage and responses of other buildings using a surrogate model. Considering the skewed distribution of the buildings in a region, a novel informative sample selection method is proposed that is, designed for bimodal sampling of the input domain. The Gaussian process regression was used as the surrogate model and the performances of different sample selection methods were compared. The proposed method can approximate the results of the regional damage simulation regarding total economic loss estimation with 98.99% accuracy while reducing the computational demand to about 1/7th of the simulation processing time.
Yuan et al. proposed and developed a new image encoding technique for CNN-based seismic damage evaluation based on a specific code-conforming benchmark building. The method is based on time-series segmentation (TS) to transform acceleration (A), velocity (V), and displacement (D) ground motion records into a three-channel AVD image of the ground motion event. The proposed TS technique was compared with two time-series image encoding techniques, namely recurrence plot (RP) and wavelet transform (WT). The CNN trained through the TS technique was also compared with the IM-based machine learning approach. The CNN-based feature extraction has comparable classification performance to the one of the IM-based approach. Both the WT 1,000 × 100 and TS 100 × 100 three-channel AVD image encoding methods are promising for future studies of CNN-based seismic damage evaluation.
All authors listed have made a substantial, direct, and intellectual contribution to the work, and approved it for publication.
The authors declare that the research was conducted in the absence of any commercial or financial relationships that could be construed as a potential conflict of interest.
All claims expressed in this article are solely those of the authors and do not necessarily represent those of their affiliated organizations, or those of the publisher, the editors and the reviewers. Any product that may be evaluated in this article, or claim that may be made by its manufacturer, is not guaranteed or endorsed by the publisher.
We would like to thank all authors for their valuable contributions, the dedicated reviewers for their useful guidance and suggestions for improving the papers and the Editorial Team of Frontiers in Built Environment (“Earthquake Engineering” and “Computational Methods in Structural Engineering” sections) for the professional assistance and for giving us the valuable opportunity to launch this Research Topic.
Keywords: artificial intelligence, seismic risk, damage assessment, system identification, structural dynamics and control, structural health monitoring, damage detection
Citation: Lu X, Plevris V, Tsiatas G and De Domenico D (2022) Editorial: Artificial Intelligence-Powered Methodologies and Applications in Earthquake and Structural Engineering. Front. Built Environ. 8:876077. doi: 10.3389/fbuil.2022.876077
Received: 15 February 2022; Accepted: 01 March 2022;
Published: 18 March 2022.
Edited by:
Ehsan Noroozinejad Farsangi, Graduate University of Advanced Technology, IranReviewed by:
Abbasali Sadeghi, Islamic Azad University of Mashhad, IranCopyright © 2022 Lu, Plevris, Tsiatas and De Domenico. This is an open-access article distributed under the terms of the Creative Commons Attribution License (CC BY). The use, distribution or reproduction in other forums is permitted, provided the original author(s) and the copyright owner(s) are credited and that the original publication in this journal is cited, in accordance with accepted academic practice. No use, distribution or reproduction is permitted which does not comply with these terms.
*Correspondence: Xinzheng Lu, bHV4ekB0c2luZ2h1YS5lZHUuY24=
Disclaimer: All claims expressed in this article are solely those of the authors and do not necessarily represent those of their affiliated organizations, or those of the publisher, the editors and the reviewers. Any product that may be evaluated in this article or claim that may be made by its manufacturer is not guaranteed or endorsed by the publisher.
Research integrity at Frontiers
Learn more about the work of our research integrity team to safeguard the quality of each article we publish.