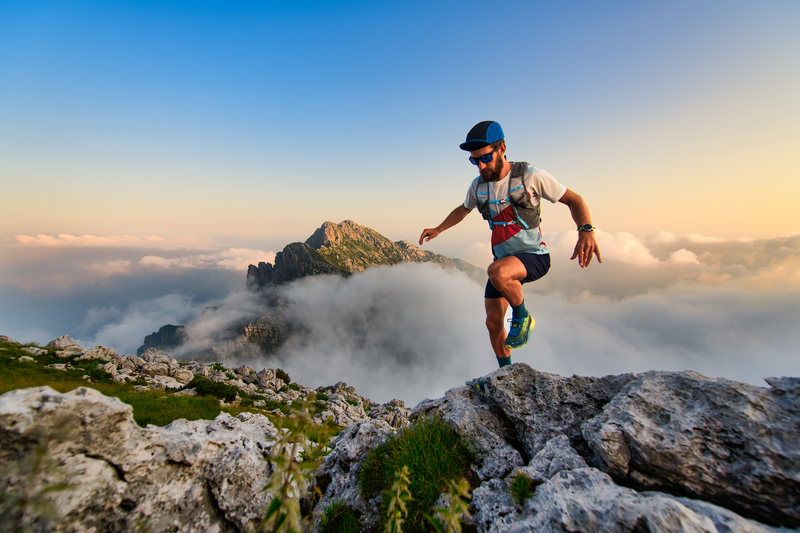
95% of researchers rate our articles as excellent or good
Learn more about the work of our research integrity team to safeguard the quality of each article we publish.
Find out more
REVIEW article
Front. Built Environ. , 09 November 2022
Sec. Building Information Modelling (BIM)
Volume 8 - 2022 | https://doi.org/10.3389/fbuil.2022.1013196
This study aims to evaluate the utilization of technology known as Digital Twin for fault detection in buildings. The strategy consisted of studying existing applications, difficulties, and possibilities that come with it. The Digital Twin technology is one of the most intriguing newly discovered technologies rapidly evolving; however, some problems still need to be addressed. First, using Digital Twins to detect building faults to prevent future failures and cutting overall costs by improving building maintenance is still ambiguous. Second, how Digital Twin technology may be applied to discover inefficiencies inside the building to optimize energy usage is not well defined. To address these issues, we reviewed 326 documents related to Digital Twin, BIM, and fault detection in civil engineering. Then out of the 326 documents, we reviewed 115 documents related to Digital Twin for fault detection in detail. This study used a qualitative assessment to uncover Digital Twin technology’s full fault detection capabilities. Our research concludes that Digital Twins need more development in areas such as scanner hardware and software, detection and prediction algorithms, modeling, and twinning programs before they will be convincing enough for fault detection and prediction. In addition, more building owners, architects, and engineers need substantial financial incentives to invest in condition monitoring before many of the strategies discussed in the reviewed papers will be used in the construction industry. For future investigation, more research needs to be devoted to exploring how machine learning may be integrated with other Digital Twin components to develop new fault detection methods.
The concept “Digital Twin” has emerged during the last decade in manufacturing, production, and operation as a computerized model that replicates the actual system (Jones et al., 2020; Sacks et al., 2020). This technology was first used in 2002 in aerospace production and product lifecycle management by NASA for the moon exploration mission Apollo 13 (Augustine, 2020).
The Digital Twin represents the physical and functional properties of a complex system such as a construction project. The Digital Twin has three main elements: a physical object, a virtual model, and the connection that binds these two together. This connection is an exchange of data, information, and knowledge made possible by advanced senses such as computer vision, the Internet of things, high-speed networks, and advanced analysis technologies. All data are collected from sensors located on the physical object, which is used to establish a virtual object (Sacks et al., 2020; Jiang et al., 2021).
The Digital Twin is later used for visualization, modeling, simulation, analysis, and further planning. For constructions, this means that you always have access to models that are constantly synchronized in real-time. This allows companies to monitor progress in a 4D BIM model at any time. In addition, it can be used to analyze different courses of action and estimate their probabilities to choose the most optimal solution (Hosamo et al., 2022a). The major components of the Digital Twin may be depicted in Figure 1, where the simulation model must be confirmed utilizing technologies such as laser scanners, drones, sensor data, and so on. Once the model has been verified, it may be used to make better decisions, streamline processes, and make predictions by providing insight into how the real thing behaves in various simulated settings.
More than 50 years of an facility asset’s overall life cycle is spent in the “Operation and Maintenance” (O&M) phase, which is typical for buildings and other types of civil infrastructure (National Research Council, 2007). During the O&M phase, one of the most difficult challenges is to achieve smart building management. It is necessary to save detailed information (such as historical records, performances of facilities, correct locations, etc.), and various technologies would be required to do so (e.g., sensors, cameras, etc.). During O&M management, one of the most important challenges is overcoming and maintaining the data’s integrity, validity, and interoperability (Wetzel and Thabet, 2015). As a consequence of this, it is necessary to have an O&M management system that is both efficient and intelligent to sustain dynamic information, support a variety of activities, and contribute to a healthy environment (Lu et al., 2018).
There is no integrated platform currently available to handle information scattered across many databases or support actions occurring throughout the O&M stages. Computerized Maintenance Management Systems (CMMS), Computer-Aided Facility Management (CAFM) systems, Building Automation Systems (BAS), and Integrated Workplace Management Systems (IWMS) are just some of the tools and systems that have been developed to improve O&M management (Keller, 2012). For example, CMMS is a computerized system for O&M management that may store daily work orders, historical data, service requests, and maintenance information. CMMS also can track service requests (Nojedehi et al., 2022). However, to extract the various O&M information that facilities management (FM) experts require (for example, data from CMMS and 3D models), they still have to put in a substantial amount of work and spend much time doing so (Wetzel and Thabet, 2015).
It is anticipated that building information modeling (BIM) developments would cut by 98% the time needed to update databases throughout the operation and maintenance phases (Ding et al., 2009). By modifying BIM and establishing technologies to increase data interoperability and integration, several integrated and complete solutions for O&M management have been presented. For instance, the team that worked on restoring the Sydney Opera House built a unified central data repository to enable efficient O&M management. This repository integrated a variety of various resources. However, on the whole, research is still being done to develop a complete and practical method for data integration based on BIM. This approach must be maintained and updated through the O&M phase (Ding et al., 2009; Parsanezhad and Dimyadi, 2013). An integrated, intelligent strategy or system is still necessary for ongoing development and improvement. This approach or system should be able to aid in the monitoring, updating, communicating, and integrating of O&M management concerns.
Anomaly detection for building assets is regarded as the procedure that requires the most human resources, takes the most time, and has the biggest impact throughout the operation and maintenance phase (Shi and O’Brien, 2019). Extensive research shows that prompt anomaly detection may significantly improve the safety, effectiveness, and quality of the processes involved in building operations (Shi and O’Brien, 2019). In its most basic form, it is a preventative and proactive action that ensures the assets continue to perform the original purpose they were designed throughout their lifecycle. However, one of the most critical issues is that the loads these assets are expected to carry are always shifting because of human needs. As a result, the assets’ performance, such as the pump’s vibration during the daily O&M, is not stationary. Conventional point-based anomaly detection algorithms cannot handle this situation very effectively, particularly in the constructed environments that are being targeted, since it is normal to be a lack of data that has been properly tagged (Qiuchen Lu et al., 2019). Digital Twin are regarded as an all-encompassing solution. Digital Twin originated as an all-encompassing method for managing, planning, predicting, and demonstrating the state of a building’s infrastructure assets (Fjeld, 2020; Chen et al., 2021; Deng et al., 2021).
This research explores the possibility of utilizing Digital Twin technology in the fault detection of service and monitoring systems, including:
• Data management and integration in facility maintenance.
• Real-time intelligent facility asset maintenance and monitoring.
• A well-established machine learning data analysis technique in fault diagnosis.
The following are the questions this study aims to address:
• What is the current use of Digital Twin?
• Can Digital Twin technology enhance the current fault detection in building practices?
• What are the challenges of Digital Twin technology implementation?
Figure 2 shows the service and monitoring systems.
Building information modeling (BIM) was introduced some time ago and is now essential in construction, architecture, and engineering (Seyis, 2019). It was mainly introduced to differentiate between architectural 3D modeling (BIM) with a lot of information and the established 2D drawings. BIM has been praised by its users because of its ability to detect errors in early stages, making the process more efficient (Pour Rahimian et al., 2020).
BIM allows the construction of virtual models with a lot of information. Digital models contain clear-cut geometry and appropriate data to aid in the construction, production, and acquisitions necessary to finalize the project as optimal as possible (Marzouk et al., 2018).
BIM covers every aspect of the building industry. The figure below (Figure 3) visually explains the process a BIM project will go through. The example below considers a project from restoration and every step on the way through the design phase, production phase, the operational phase, and finally demolition at the end of the building’s lifetime (Mijic et al., 2017).
In practice, BIM will be used by a group of engineers, architects, and contractors. They will use their chosen software to create a virtual model of the structure and share the model. This model will mainly be a 3D model of the structure, existing details and include drawings and specifications. By using this model, the group can share, identify and solve issues regarding the design and construction before the construction phase is ongoing. In addition, the model will also be used in the construction phase when new cases occur by using the model to find new alternative solutions (Eastman et al., 2011).
The Internet of things (IoT) connects physical objects to the Internet through sensing technologies and different types of communication (Yuan et al., 2016). These tools gather a lot of information to form connections between objects, as well as between things and people. IoT contains various technologies, but some are more central, such as:
• Sensing technology.
• Wireless communication technology.
• Cloud computing technology.
• Radio-frequency identification (RFID) intelligent identification technology.
• Internet Protocol version 6 (IPv6) technology.
RFID technology is the one that has been implemented the most so far, at least when we are looking into BIM and IoT together. This is because they are easily connectable, and RFID technology can be used in several different ways where BIM is already implemented (Chin et al., 2012; Srewil and Scherer, 2013).
One purpose of RFID technology is to use RFID tags in the helmet of construction workers so that they can be “traced” in BIM; this is beneficial for the construction workers’ safety as they can be monitored in real-time; however, a balance between workers safety and privacy should be considered. The same can be done on objects as they can be observed in transportation for logistic benefits, and in the case of a vast construction area, things can be found within the BIM model.
IoT sensors can also be a valuable tool in construction and operation since they provide construction data in fields such as temperature, humidity, stress, and strain. These values can prevent construction failure since they can give an early warning if critical values are reached (Plageras et al., 2018).
Devices that are involved with IoT are everywhere; even the simplest devices today may communicate in some ways. The technology is evolving and getting more familiar with time; this success is due to the availability of information to move around (Garramone et al., 2020).
The concept of Digital Twin technology is one of the segments in Industry 4.0 which is expanding the most (Boje et al., 2020; Opoku et al., 2021). A Digital Twin is a replica of the BIM model or a model from a 3D laser scan equipped with wireless sensors. The significant difference between the BIM model and the Digital Twin is that the BIM model is not dependent on real-time data to fulfill its function. The BIM model is mainly used to avoid any errors during the design and construction of a building. The digital model updates when the digital model of a physical object is changed; this is achieved through cloud-based software and the expanding diversity of IoT (Lu et al., 2020b). Figure 4 displays the process of completing a Digital Twin and highlights some positive uses: predictive maintenance, building operations improvement, and data analysis.
FIGURE 4. The components required to make a Digital Twin of a building and the distinction with BIM.
The importance of a Digital Twin can be described as follows: “Digital Twins will facilitate the means to monitor, understand, and optimize the functions of all physical entities, living as well as nonliving, by enabling the seamless transmission of data between the physical and virtual world.” (Fuller et al., 2020).
If the Digital Twin is applied in today’s architectural, construction, and engineering projects, some of the benefits one can expect are the following:
• The overall efficiency will increase throughout the entire life cycle of the service system (electrical, lighting, and HVAC systems) and monitoring system (CMMS, sensor system, AMS, and BMS systems).
• Real-time updates from the physical assets make it possible to predict the failure and required maintenance of the service and monitoring systems.
• Problems can be solved without having to visit the physical object. This means determining if the problem is related to indoor air quality, acoustic comfort quality, visual comfort quality, thermal comfort quality, envelope insulation quality, and HVAC capacity to meet the thermal load.
• The Digital Twin may also provide the possibility to provide insight into occupants’ behavior based on real-time data, which will result in further improvements as it is returned to the 3D simulations.
Digital Twin collect the real-time data transferred by sensors and utilize it to forecast how the process of a structure will perform during its lifetime. This can help detect eventual faults in the buildings. The detected defects could be minor errors that would only require small repairs, and potentially be more economical because it was seen in an early stage, compared to what would have been if Digital Twin were not used. It could also help detect numerous errors that could be crucial in terms of failure or total collapse. A Digital Twin is helpful for both small and bigger fault detection and will be more economical. One might avoid fatal outcomes by detecting structural issues before they appear. Digital Twin technology might be essential in areas with extreme weather and where it is familiar with natural disasters. These damages are harder to foresee since these damages are due to fatigue and will not be visible. It will be possible to predict the building’s process and development using Digital Twin technology, which will help prevent a potential building collapse.
A mixed review technique was used to assess the applications of Digital Twin in fault detection in buildings to comprehend the topic expertise completely. This approach entails the following steps:
• Choosing databases and keywords.
• Gathering and screening pertinent papers.
• Qualitative analysis.
• Identifying research gaps and future directions.
The Recommended Reporting Items for Systematic Reviews (PRISMA) standard was followed during the data collection and selection phase (Page et al., 2021). The method and important procedures used in the literature review are illustrated in Figure 5. To prevent omission, a search for the most pertinent papers was conducted in three different literature databases, including Scopus, Web of Science (WoS), and Google Scholar. Reference and citation monitoring was also done to lessen the possible bias from the chosen literature databases. Search principles were developed to find papers regarding Digital Twin uses in facility maintenance. The search covered all documents, including journal articles, conference papers, and proceedings. Without specifying a publication year range, a search on the “Title/Abstract/Keyword” of the literature was carried out in July 2022.
The keywords of the existing knowledge domain in the Digital Twin for fault detection in buildings are shown using VOSviewer in Figure 6. The VOSviewer shows a distance-based representation of the keywords network. In this network, each keyword is a node, and the linkages that connect them are known as links. A connection’s strength and weakness depending on the distance between two nodes. A weaker relationship between two keywords or nodes is shown by a larger distance, while a smaller distance indicates a stronger link (Perianes-Rodriguez et al., 2016). The connection strengths connected to a single node are added to the total link strength. Various colors denote different research years, and the size of the supplied nodes corresponds to the number of articles in which the phrase was first used (Oraee et al., 2017).
After deleting duplicates, the search returned 326 items. As part of the screening process, each article’s title and abstract were scrutinized for relevance to the scope of this study. Consequently, a total of 205 studies were included in the final assessment. The next stage was to determine whether or not these 205 studies could be included in the qualitative analysis. The number of citations, the year of publication, and the SCImago Journal Rank (SJR) were used to choose which articles could be used in the qualitative analysis. As a result, 115 articles were considered for inclusion in the qualitative study. Figure 7 depicts the literature trends in this study.
In order to assess the chosen papers, identify research issues, and offer suggestions for future study paths in the examined areas, qualitative analysis is used (Onwuegbuzie et al., 2012; Baldini, 2022). To fully automate fault detection and prediction, a comprehensive framework must be made available to reduce reliance on human knowledge and intervention while increasing productivity, dependability, and timeliness. Following this, we propose BIM and machine learning as fundamental pillars of a Digital Twin-enabled framework for charting a course toward automated fault detection and prediction in buildings. Results include a qualitative analysis of factors that have the potential to improve fault detection and prediction in buildings. Using the study’s findings, a road toward fault detection in buildings is drawn. In the following section, the results of this study will highlight significant applications and practical difficulties as well as potential future research areas.
A crucial part of answering the research questions of this paper is determining the feasibility of Digital Twin technology use for fault detection. Thus the following topics have been extracted from the literature review to reflect the benefits from Digital Twin in fault detection in buildings.
Several faults have been recognized based on the literature review (Zhao et al., 2015, 2017; Bortolini and Forcada, 2019; Cheng et al., 2020; Alavi et al., 2021; Nehasil et al., 2021; Hosamo et al., 2022b). For example, heating and cooling at the same time, risk of discomfort, heat unexpected on, faulty supply fan, envelope thermal insulation, widows to wall ratio, acoustic attenuator, etc. Dealing with those faults and showing the potential of Digital Twin to solve these issues is our focus in this study. The Digital Twin technology has huge potential in detecting faults in real-time that typical systems cannot detect. In addition, some researchers could accurately predict the faults using the Digital Twin (Hosamo et al., 2022b), making it easier for the facility managers to make the right decision and act before the failure happens. Moreover, using Digital Twin, one can address other buildings that may make occupants uncomfortable, like visual and acoustic issues leading to less energy consumption and making people feel comfortable in buildings. In this sense, the next building may be learned from the detected faults by Digital Twin to be considered in the following design.
The level of service a facility gives its inhabitants is determined by the assets within the building that is responsible for performing the building’s service functions. Consequently, building activities in the O&M phase are optimized by closely monitoring asset performance and quickly reporting abnormalities that arise. It is complicated to discover anomalies for asset monitoring because of the high degree of system complexity, the size of the integrated system, and the number of components. A frequent technique is looking for asset performance anomalies that depart from the anticipated patterns (Chandola et al., 2009; Hosamo et al., 2022b).
Digital Twin-based and traditional process flow scenarios are examined in literature studies (Lu et al., 2020a; Lu et al., 2020c; Motawa and Almarshad, 2013). There are two major flaws in the old procedure compared to Digital Twin-based anomaly identification: dispersed information and human query operations. It still takes a long time to search, query, verify and analyze the corresponding facility information from heterogeneous data sources, even though some data on maintenance and operation are managed in some facility information systems (e.g., BMS, AMS). As soon as the call service system (Figure 8) notifies facility management experts of a requirement for maintenance, they must first look for relevant asset management system information (such as historical or manufacturer information) before verifying the space management system’s location information. It could be required to query the BMS or use other methods if more details are needed.
As a result of this procedure, there may be mistakes and variances. In the typical method, there is much repetition of information searches. Overlapping data, such as historical records, locations, and the associated contractors’ information, can be stored in many databases (Lu et al., 2020a). In specific systems, such as AMS, BMS, and SMS, data sets of sites, buildings, and floors are saved repeatedly and redundantly. Manual query operations, in addition to the dispersed data, significantly contribute to the delay in anomaly identification. In a traditional procedure, as shown in Figure 8, the facility manager often serves as a central coordinator and makes decisions based on relevant data and expert expertise.
An intelligent and comprehensive platform is needed to efficiently search information and support semi-automated or automated operations to address these issues of the traditional approach. In light of the ease with which a DT-based system can be used to look up and validate facility information and automate anomaly detection, these issues can be alleviated.
Multi-domain and multi-layer information storage, manipulation, sharing, and interaction are required in the O&M phase to detect abnormalities in building assets. Through information sharing, effective anomaly detection may be achieved by eliminating change points induced by normal operating conditions to eliminate false alarms. Detection of anomalies in building O&M research relies on various data sources, including those discussed in previous section (such as building automation systems and building management systems, or BAS and CMMS). New O&M data sources remain within well-established communication protocols for storing and exchanging building data. Unusual operating behavior in a building’s HVAC system may be detected quickly using the BAS data generated by sensors and actuators (which may be Building Management Systems (BMS) for other scenarios) (Costa et al., 2013). Building sensing data (e.g., access control system and security camera for occupancy monitoring) should be incorporated to identify if a dramatic change in external temperature is the source of the supply air temperature drop of an AHU in heating mode. Anytime the AHU heating coil valve temperature falls below its mixed air temperature, an abnormality is likely to occur. The tenants’ service requests and work order issues are documented in the CMMS database (Motamedi et al., 2014). A CMMS’s inspection and maintenance data might give valuable information about the building, such as fault trees and linkages between components. A building’s root-cause detection capabilities can be bolstered by acquiring field expert rules. As a result, it is not easy to establish an effective anomaly detection strategy because of the fragmented nature of constructing data sources. The following section explains the Digital Twin approach for anomaly detection by integrating several data sources. Figure 9 shows the data that should be collected for building fault detection and prediction.
FIGURE 9. The necessary inputs for fault detection and prediction based on Digital Twin technology in existing buildings.
According to Digital Twin definitions, Digital Twins combine their components (e.g., AI, machine learning, and data analytics) to produce digital models that can learn and update from many sources, and to represent and forecast current and future conditions of their physical counterparts (Boje et al., 2020).
Every day, various O&M systems and databases are used (e.g., BMS and SMS) (Krämer and Beseny, 2018). In most cases, the O&M data are stored in multiple formats. As a result, gathering the diverse and dispersed O&M data necessitates significant time and effort from FM employees. In the O&M phase, data integration and intelligent asset management require a consistent and uniform data structure. The IFC data schema are the most appropriate and essential for BIM deployment and information integration because of its flexibility and consistency throughout the construction lifecycle. As a result, the present IFC must be expanded to meet O&M management needs (Cheng et al., 2020).
Furthermore, the O&M phase’s asset information is not static. When it comes to sensor data, for example, it is constantly changing, and maintenance events are logged one at a time. A single IFC file would be useless for decision-making because existing IFC files may only provide basic geometric information. In this regard, a centralized data model linking to dispersed data resources in day-to-day O&M management are a suitable and realistic approach for modeling the IFC schema and integrating information (Wetzel and Thabet, 2015; Burak Gunay et al., 2019).
As a result, the data structure used in building Digital Twins’ data integration layer are intended to exchange and interoperate external data connected to each BIM object on a semantic level, enabling IFC-based interoperability between BIM and other data sources. IFC is the primary data model, while other data resources are preserved in their original storage places, which are saved in several formats.
Recent studies show that FM is paying more and more attention to BIM development (Costa et al., 2013; Pärn and Edwards, 2017; Pärn et al., 2017; Pärn et al., 2018; Dixit et al., 2019). There is, however, several studies into IFC’s role in operations and maintenance. O&M-related information and actions are not represented in the current IFC4 schema (Chen et al., 2018). The Digital Twin data structure construction process should include additional subclass data entities, types, and parameters necessary for FM, where a broader range of data types and O&M tasks are required (Patacas et al., 2015; Wong et al., 2018). Additional FM-related attributes and relationships must be included in a data structure for the inspection process (Costa et al., 2013; Wong et al., 2018; Dixit et al., 2019). Maintenance and inspection procedures must be incorporated into the IFC schema, inspection events, activities required for maintenance, specification, regulation, cost schedule, and other requirements are all examples of control, which is an extension of the control or constraint concept. Even while IfcControl can partially represent the information necessary for the maintenance plan, schedule, and cost, these entities are not explicitly designed for O&M management and cannot be linked totally with O&M operations.
The asset’s historical record is also critical for O&M, but neither IfcOwnerHistory nor IfcPerformanceHistory includes all of the necessary information for FM. Example: IfcChangeActionEnum lacks an enumeration for the function “FM”. The asset register criteria may be matched using IFC4 entities and the COBie spreadsheet. Some conditions in IFC4 cannot be tied to entities. Data gaps (such as costs, sources of components and spare parts, and consumption) and incompleteness are widespread in operations and maintenance (such as records of previous maintenance costs, pricing, and operations).
One of the most widely used national standards in the US, United Kingdom, and other nations is COBie, the most significant specification for integrating BIM and O&M systems (East, 2013). However, COBie can offer part of the O&M data that are required and still have several technical concerns that need to be addressed; for example, after the information transmission, model validation is required.
“Prediction” and “optimization” are frequently vague, imprecise terms. There are several sub-disciplines under the umbrella term “artificial intelligence,” such as “machine learning,” “data analytics,” and “logical” forms of “artificial intelligence.”
Artificial Intelligence (AI) has long been considered an essential component of Digital Twins to cope with the Internet of Things (IoT) (sensing and actuation). Several studies have found that artificial intelligence (AI) is crucial to the Digital Twin’s ability to forecast and optimize dynamically. There is still debate over how high a Digital Twin maturity level should be before it can accurately predict and issue warnings about the physical asset’s performance (Madni et al., 2019).
A typical requirement for Digital Twins is the ability to forecast future asset behavior or health condition. Predictions must be able to anticipate subsequent environmental states throughout time using measured input and output values, as well as beginning circumstances, in contrast to simulations, which can accurately duplicate the physical conditions utilizing input data and initial conditions (Kuster et al., 2017; Tongal and Booij, 2018)). Prediction should be employed for instant physical actuation as a reaction, according to phrases like “predictive modeling” and “structural life prediction” (Patterson et al., 2016; Qi and Tao, 2018; Madni et al., 2019; Hosamo et al., 2022b).
A common perspective of exploiting IoT utilization is “Big Data” fed prediction (Qi and Tao, 2018; Yusen et al., 2018). The phrase “Big Data” itself is vague, including not just quantity but also diversity, and stages of existence, ranging from unstructured to semi-structured to organized data (Gobble, 2013). As a result, managing vast volumes of data can significantly benefit the adoption of the Digital Twin (Qi and Tao, 2018). Big data prediction strategies frequently involve machine learning or data mining, with the former emphasizing finding new patterns and implicitly understanding the data itself, while the latter focuses on replicating general knowledge. Comparing data analysis to Digital Twins in-depth, Qi and Tao (Qi and Tao, 2018) emphasize the necessity for a future fusion between the two to increase Digital Twin self-reliance and provide general interoperability. The procedures used for acquiring, scrubbing, and organizing the data combined for greater significance and employed for processing intelligent tasks present a concern.
Concern should also be expressed about the validity of the data’s sources. Predictions based on real sensor data should be distinguished from forecasts based on simulated sensor data or a hybrid technique. Consideration must be given to confirming the accuracy of the prediction and its implications for actuating the physical component. In other words, can that judgment be made using existing AI techniques that are sufficiently “clever” to do so?
Like the preceding approaches that utilized AI, the term “optimization” is often used in the Digital Twin industry. Optimizing the Physical Twin’s production and operational expenses appears to be the joint driving force. When it comes to manufacturing, the essential use case is to ensure that resources are allocated in the most efficient way possible, like in the case of experimental testbeds that aim to optimize assembly algorithms (Schluse et al., 2018). For example, Alam and Saddik (Alam and El Saddik, 2017) employ Bayesian networks to describe the decision model in engineering systems design. Costs scale up dramatically during operation in the built environment and energy sector (Howell et al., 2017), where balancing consumption against the demand for energy and resources is the fundamental difficulty. Infrastructure and building construction has a significant influence on operating expenses throughout the course of their lifespan. Unfortunately, there are times when the aims of construction optimization diverge from those of operation optimization during the lifecycle.
Using deep learning to help facility managers make better decisions and perform more efficient maintenance has significantly impacted building facilities management. Usually, there are five basic forms of maintenance: corrective, preventive, predetermined, condition-based, and predictive (Sagnier, 2018). Depending on the nature of their business, several organizations employ various maintenance strategies; however, facility managers often handle building maintenance in one of two ways: preventative or reactive. These strategies have certain drawbacks since they cannot be used for prediction and cannot avoid breakdowns when mechanical, electrical, and plumbing (MEP) components need to be repaired in advance. Predictive maintenance solutions, including cutting-edge technologies like IoT, have become the go-to solution where deep learning technologies are more commonly seen to enhance facilities management and maintenance efficiency (Cheng et al., 2020; Hong et al., 2020). Python with TensorFlow (Abadi, 2016), Keras (Ketkar, 2017), and PyTorch (Paszke et al., 2019) are common tools for creating deep learning models, as are MATLAB with Deep Learning Toolbox (Teza et al., 2022) and R (Ciaburro and Venkateswaran, 2017). Figure 10 depicts the location of FM in the BIM domain of the construction sector.
The goal of predictive maintenance is to forecast equipment breakdowns to schedule advanced corrective maintenance and avoid unplanned downtime and raise service levels. To help with facility management and maintenance, Marzouk and Zaher (Marzouk and Zaher, 2020) suggested a proactive maintenance application to maintain, improve, and run the assets of three fire protection systems cost-effectively with a deep-learning pre-trained model. The suggested deep learning model could categorize Mechanical, electrical and plumbing (MEP) components in the fire prevention systems using supervised learning and a deep CNN utilizing image classification (Marzouk and Zaher, 2020). According to the following study, a computerized decision-support system incorporating CNNs for image recognition can quickly spot fractures or other signs of deterioration in three-dimensional (3D) models (Czerniawski and Leite, 2018). Analyzing many criteria in diverse settings, an automated decision support system using 3D geometry might help facility managers make the right interventions.
Images may be categorized into millions of predetermined categories using deep learning and computer vision algorithms (Marzouk and Zaher, 2020). To improve asset management, it is also feasible to interpret printed and handwritten text, read picture information, and build useful metadata for smart image catalogs (Czerniawski and Leite, 2018). Deep learning can automatically separate Red Green Blue-Depth (RGB-D) photos into different construction parts (Deng and Chen, 2020). A shared convolutional neural network (CNN) is used for feature extraction from pictures in a vision and learning-based indoor localization framework (Wei and Akinci, 2019). Instead of requiring the deployment of RFID tags, this system concurrently conducted localization and object recognition for facility management (Wei and Akinci, 2019).
Poor management of building resources, such as the HVAC and lighting systems, results from inaccurate occupancy estimation. Occupancy prediction models are created with the information gathered by occupancy sensors throughout the occupancy monitoring period. Due to the potential for very dynamic and contextual occupancy levels, these models are crucial for occupancy prediction. Advanced occupancy prediction methods employ assumption-free ANN algorithms to uncover hidden patterns in the sensor data gathered, increasing the accuracy of their predictions (Li et al., 2017). Assumptions regarding data distributions are often not made by ANN models before learning, which is consistent with their suitability for occupancy prediction.
The You Only Look Once (YOLO) deep CNN for multiple item identification and the multi-stream deep neural network are used by Mutis et al. (Mutis et al., 2020) to estimate occupancy counts in a room. The investigation had a positive result since using the technology for precise occupancy identification led to 10 and 15 percent energy savings, improving FM (Agarwal et al., 2010). Martani et al. (Martani et al., 2012) reported using Wi-Fi connections as a stand-in for occupancy level to analyze occupancy and measure occupant activities for energy consumption patterns (electricity, steam, and chilled water). Although just a small portion of power usage was connected to occupancy, the research findings also showed that the operation of HVAC systems depended on variables like the outside temperature and human occupancy (Martani et al., 2012). (Amato et al., 2017) presented a good CNN architecture for visual parking occupancy detection. The solution was then compacted for smart camera operation. Deep learning is recommended by Sonetti et al. (Sonetti et al., 2018) to study human behavior for intelligent and sustainable surroundings to reduce energy usage.
Occupancy prediction is critical since it influences energy use in real buildings, not just those under construction. Using IoT data, Kim et al. (Kim et al., 2019) developed a machine learning framework for HVAC that tested the performance of three occupancy estimate techniques, namely, decision trees, support vector machines, and ANNs. The study found that ANNs were more accurate in predicting occupancy than the other methods.
In order to construct the next generation of occupancy models that can reliably anticipate occupants’ behavior, new DL approaches are being employed. Hammad (Hammad, 2019) presented a strategy for reducing the difference between expected and real energy consumption rates by merging BIM with an ANN model. A deep neural network training to predict occupant behavior results in accurate BIM representations, further confirmed by energy simulations. On-site thermal cameras and deep learning were used by Lee et al. (Lee et al., 2019) to construct an adaptive comfort model. Because it considers the dynamic interactions between people and their surroundings, an adaptive comfort model could be employed to operate an air conditioning system successfully. Maintaining the temperature and humidity levels in commercial spaces is essential. Data gathered from various IoT sensors shows that manual work results in wasteful energy use. Customers are more satisfied, and the energy consumption is reduced by smart supermarkets that control the HVAC and refrigeration systems themselves. A building’s energy consumption is reduced as a result of maximizing the use of resources. As a result of this study, a firefly-based optimized Long short-term memory (FOLSTM) model for a supermarket was presented (Karthikeyan and Raghu, 2020). Forecasting crucial factors, such as temperature, was key to making the most available resources (Karthikeyan and Raghu, 2020).
Occupancy prediction is more important if occupant crowdedness can be forecast a day before to better facility management. Research thus far has focused on estimating the present population of a certain place, but this information might also help enhance decision-making processes in the future (Kumar et al., 2013; Zou et al., 2020). Schedule repair when foot traffic is low, i.e., during off-peak hours, to minimize disruption via deep learning-based time-series crowd prediction (Poon et al., 2022). For example (Poon et al., 2022), address the two fundamental restrictions where prediction accuracy drops as prediction time grows, and only the most recent input data are used by adopting a Long-Time Gap Two-Dimensional technique (LT2D). Predictions may be extended to 1 day with good accuracy using the LT2D technique, which uses long-time gap prediction with 2D inputs to leverage temporal trends from previous days. Adding the suggested LT2D approach to baseline models like Long short-term memory (LSTM), BiLSTM, and Gated recurrent units (GRUs) improves accuracy by about 22% (Poon et al., 2022).
Although the topic of fault detection is extensive, automated fault detection and diagnosis (FDD) is the one that concentrates on data processing and maintenance decision-making assistance. However, the language used in the FDD research is a little hazy. While some authors prefer the term “fault detection and diagnostics” (FDD) ((Yu et al., 2014)), others prefer the term “automated fault detection and diagnostics” (AFDD), emphasizing the methods’ automatic nature (While the processes are somewhat automated, they frequently depend on a human to perform the final verification of detected faults and to decide what maintenance measures to take) ((Kim and Katipamula, 2018)). Prognostics is also a component of specific research, which is referred to as “fault detection, diagnostics, and prognostics” (FDD&P) ((Yang et al., 2018)). Apart from the terminology aspects, specific analytical techniques are frequently used to extract characteristics from the given data and use those data for prognostics and diagnostics. This section examines the techniques used in Digital Twin-based automated fault detection, diagnostics, and prognostics.
Models for fault detection and diagnosis are created using data-driven techniques using previous data from BMS. Data-driven approaches can be classified as black-box depending on the models employed and their parameters; examples include polynomial regression, logistic regression, autoregressive (A.R.) models, and principal component analysis (PCA) (Cui and Wang, 2005; Radhakrishnan et al., 2006; Fisera and Stluka, 2012; Ploennigs et al., 2013; Yuwono et al., 2015) and gray-box methods (Nassif et al., 2008; Yu et al., 2011; Sun et al., 2014). The distinction between gray-box and black-box models is that gray-box models employ parameters that have a physical meaning, whereas black-box models do not.
The parameters of data-driven approaches must be tuned using non-trivial quantities of historical data, particularly in the case of artificial neural networks. Additionally, due to the operational variations, such a customized fault detection and diagnostic system cannot often be transferred to another comparable system. As a result, the techniques are not ideal for new structures or other locations without access to historical data. Additionally, even with gray box approaches, the extrapolation is restricted, and black-box methods often cannot extrapolate to find or diagnose errors in the data employed.
The majority of research on FDD for AHU either employ simulation-generated datasets (Padilla and Choinière, 2015; Allen et al., 2016; Granderson et al., 2016; Yan et al., 2016a) or datasets with manually added faults (Wang et al., 2012; Dong et al., 2014; Narayanaswamy et al., 2014; O’Neill et al., 2014; Dey and Dong, 2016). Such synthetic datasets indicate various constraints and difficulties. First, problems in buildings sometimes take arbitrary values on a scale rather than being binary, happening as defective or ordinary. A valve may allow various flows to leak, for instance, or a damper may be stuck halfway between fully open and fully closed (Li and Wen, 2014; Mulumba et al., 2015; Dey and Dong, 2016; Yan et al., 2016b). Secondly, artificial datasets typically oversimplify and make assumptions to be more practical, failing to adequately represent the range of errors that can exist in the real world. These details are required by particular FDD techniques that rely on a priori probability of different errors and prognostics. However, so-called natural occurrence datasets (Qin and Wang, 2005; Narayanaswamy et al., 2014), that were collected from the field have inherent limits. First, it takes time and effort to choose such datasets where the errors occur in statistically significant amounts. Furthermore, there is no assurance that all naturally occurring faults will be included in the information acquired during an observation period, regardless of how lengthy it is. Furthermore, it is challenging to confirm whether data from “regular” operations are faulty or not when data are collected from the field. There is always a chance that some faults are present; however, commissioning and other similar procedures can reduce the likelihood of concealed faults.
The steady and transient states of a system can be described in explicit mathematical models using quantitative model-based approaches (Weimer et al., 2012; O’Neill et al., 2014) A robust engineering design foundation is essential for quantitative methods since these methods demand vast and deep system understanding. The construction industry has seen a recent trend toward bridging the knowledge gap between design and usage by creating sophisticated simulation models throughout the design process. Data generated during the system’s design might lead to the high similarity between the system dynamics and diagnostic models. A precise understanding of the system’s physical workings and linkages can lead to sophisticated physical models (Kim and Katipamula, 2018). Partially differential equations based on mass and energy balances and the specific characteristics of the observed system can be used to construct such models (Katipamula and Brambley, 2005).
The ability to accurately simulate a system’s transient behavior is enhanced by using comprehensive physical models (Katipamula and Brambley, 2005). Instead of explaining the development of the system, physical models require only a few assumptions to mimic differential and algebraic equations with lumped parameters (Kim and Katipamula, 2018). Even simple physical models, need a great deal of expertise and time to develop (Kim and Katipamula, 2018).
Building models of both normal and abnormal behavior and comparing them with the measured features can help determine which model more correctly depicts the current behavior. This can be done by using either detailed or simplified models. There are more ways that employ residuals in the same way as previous methods. It is not uncommon for quantitative model-based approaches to necessitate a large amount of computer resources in order to simulate complicated systems correctly. In addition, the models are difficult to adapt to similar systems and need system characteristics that may not be readily available in the field. Another problem with such precisely calibrated models is their sensitivity to measurement or process noise.
The identification of many faults at the same time has become an increasingly important area of attention in current FDD technique research (Kim and Katipamula, 2018). In the past, the primary focus has been on identifying a single error when it occurs. However, in more recent research, the emphasis has been placed squarely on the identification of several errors simultaneously. This is a difficult task, and it becomes considerably more difficult when applied to the context of building systems because the symptoms of faults and the repercussions of those faults are typically not well understood or measured. Recent research has shown an increased interest in the utilization of ‘virtual sensors,’ which provide estimations of values that are not directly quantifiable (Ferretti et al., 2015; Mattera et al., 2018; Nehasil et al., 2021; Zhang et al., 2021; Chen J. et al., 2022; Zhang and Leach, 2022). Even though it can be challenging or expensive to measure specific parameters and values directly, indirect measurements and analytical approaches have made it possible to develop tools that are more effective in terms of their cost-effectiveness in estimating degradation signals and current conditions.
Even new approaches are constantly being considered and researched, it appears that more and more emphasis is being placed on the technology of Digital Twins (Chen K. et al., 2022; Halmetoja, 2022; Hosamo et al., 2022b; Xia et al., 2022). It is easy to comprehend why this would be the case, given that utilizing various approaches has the potential to assist mitigate the drawbacks of any one approach. In addition, using any historical, real-time, quantitative, or qualitative models that are now accessible makes it theoretically possible to combine expert knowledge with information from the past (Figure 11). It is generally agreed that Digital Twins offer a comprehensive solution (Batty, 2018; Haag and Anderl, 2018; Qi and Tao, 2018; Tao et al., 2019; Zheng et al., 2019). The idea of Digital Twins originated as an all-encompassing method for maintaining, forecasting, and proving the state of a building’s assets, allowing for improved services and FDD techniques.
Figure 12 depicts the Digital Twin-based fault detection structure, which is divided into two words, virtual and physical. Operational data, such as FM systems data for operation and servicing, is transmitted from the physical world to the Digital Twin. The Digital Twin is composed of three elements in the virtual world:
1) Data mapping between three systems, including BIM, BMS, and CMMS, so we have all the necessary information in one place. Data from sensors (temperatures from buildings rooms and HVAC systems, pressure, etc.), maintenance logs, and FM systems for facility operation and servicing are only a few examples of the operational information delivered from the real world to the Digital Twin via BIM. This can happen by building an application programming interface (API) over the sensor data system to transfer all the information using C# to BIM. Also, using COBie to integrate BIM data with BMS and CMMS. In this case, we have sensor data, maintenance records, space information, equipment information, and building material information in BIM. This information can be extracted from BIM using COBie to the MSSQL database, which can be updated every 5 min.
2) Simulation model. The simulation of the complete building based on the BIM model is the major purpose of this system component. By that, it would be possible to run thousands of simulations in combination to find the best design and operation for the building. The data from the MSSQL database and the simulation (which also can be stored in MSSQL) can be used as input for classification and regression models for fault prediction.
3) Services. This module is used to assist in building operations and maintenance. Services include fault diagnosis, real-time monitoring, and maintenance decision-making.
Even though it is mainly focused on the literature on the benefits of Digital Twin technology, some challenges must be addressed. Digital Twin runs parallel with artificial intelligence (AI) and the Internet of Things (IoT), which means they also have shared challenges. Some significant challenges of implementing Digital Twin for fault detection are listed below.
The first challenge is IT infrastructure. The significant growth of AI must be met with a well-utilized infrastructure for updated software and hardware to execute the algorithms. One of the biggest challenges within the infrastructure is the cost of installing and using these systems. For example, a Digital Twin for an office building of approx. 60,000 m2 can cost anywhere between 1.2 million and 1.7 million USD (Tao et al., 2019). For this technology to succeed and for companies to benefit from it, it is essential to have an IT infrastructure that is well connected and runs smoothly (Grieves, 2015; Magomadov, 2020).
The next challenge is the actual modeling of a Digital Twin, as there is no standardized approach to the procedure. Standardized methods create user understanding while ensuring the flow of information between each stage in developing and implementing a Digital Twin. Digital Twin also depends on IoT technologies regarding receiving data from smart devices. However, standards in the area of IoT require a lot of improvement, affecting the standardization of Digital Twin technology (Tuegel et al., 2011; Al-Qaseemi et al., 2016; Qi and Tao, 2018).
The success of Digital Twin technology depends on a real-time, two-way connection between the actual building and its Digital Twin. The challenges this entails are related to the resolution of sensor data, large data capacity, speed, etc. (Wu et al., 2022).
One of the most significant barriers to a Digital Twin is data sharing. This is because it is based on people’s attitudes towards this, which in turn impacts the company’s guidelines for what can be shared. The complexity of data sharing leads to developers being prevented from finding more integrated ideas (Magomadov, 2020).
Usually, BIM models utilized during the design phase are not appropriate for usage during the maintenance phase (Korpela et al., 2015). The problem starts when the person who places the order is frequently unaware of the appropriate use of the BIM models and the degree to which they should require modeling. Because of this, models can sometimes be too precise or leave out necessary information entirely.
The second issue with maintenance modeling is updating BIM models during maintenance. It is unknown who would be responsible for updating the model following major renovations or additions to the building (Quach and Wenström, 2022). No one who could keep the model and its data up to date is available. In addition, the upkeep of the model would need the expertise of the maintenance staff, which is typically unavailable.
The fact that no program can use the modeled information is the third obstacle to adopting BIM in maintenance. The FM software used in the process’s maintenance phase cannot yet read the information directly from the BIM models (Chen et al., 2018).
Finally, a model encompassing all the information required for fire safety, electro-technical repairs, and regulating potentially damaging situations would be too complicated for most users to implement effectively (Durdyev et al., 2022). The various maintenance models should be assigned to individual jobs, containing only the relevant data for that job.
The industry has to acknowledge all the advantages and overcome the obstacles for Digital Twins to realize their full potential. This must begin with the project owners and project managers at the top of the supply chain. Stakeholders must also make the required changes.
Information sharing is significantly hampered by the absence of universal standards for data exchange. Project management teams lose important data when they do not streamline procedures and hide data. Instead, everyone might have had access to this information. This hesitancy obstructs the two-way information exchange needed to create a Digital Twin. Digital twins are increasing in number and importance in the construction sector. However, improvements to this strategy must be made as quickly as possible if it is to ever succeed in large-scale enterprises.
This may be used to identify energy waste in a building and defect identification from the construction perspective. This can identify many inefficiencies in a building since these defects are also identified by IoT sensors and the deployment of Digital Twins. This indicates that reducing energy usage will be extremely important from an environmental standpoint. Reduced operational effects result in reduced potential emissions that might benefit the environment by reducing global warming.
There are more applications for the Digital Twin technology and advantages over those already stated. The Internet of Things and the real-time data gathered can be used to track building-related faults. Buildings may employ Digital Twin technology to detect faults since it has been used in numerous projects involving other structures. One can only anticipate considerable growth in the applications of the technology in construction as Digital Twins become increasingly sophisticated.
Our findings show that Digital Twin technology is frequently used for problem detection and maintenance savings despite a relatively new concept. Numerous successful cases in industries including manufacturing, shipping, mechatronic development, and offshore wind power generation, not to mention in the field of civil engineering itself, demonstrate the great potential of digital twinning processes for improving fault prediction and detection accuracy while also lowering cost and risk to human life. Being able to act on faults at the very earliest stages or even before they happen holds enormous potential and will be crucial to preventing further tragic losses of infrastructure and life. The successful development of algorithms to predict faults and errors in the maritime field with Trailing Suction Hopper Dredgers has already provided proof of concept.
There are difficulties in creating these technologies, despite the apparent promise and the necessity. First, inaccuracies in equipment constitute a substantial obstacle. While current laser measuring and scanning technology and software are pretty advanced, intrinsic imperfections are inescapable and play a considerable role in the correctness of a Digital Twin compared to the actual as-built object. As a result of our review, it is generally acknowledged that additional improvement of scanner hardware and software, detection and prediction algorithms, modeling, and twinning programs is required for Digital Twins to be compelling enough for fault detection (and other applications). Even though these are significant obstacles, it is essential to note that the idea of Digital Twins is now a heavily explored and investigated subject in several businesses. Development, case studies, testing, and subject improvement consume many resources. It is anticipated that the application of Digital Twins in several industries will develop quickly.
Second, a lot of condition-based maintenance research comes from industrial contexts and frequently makes the implicit assumption that equipment and context are not typically present in building systems. As a result, many of the techniques described in the reviewed articles could not be helpful in the construction sector until building owners and, by extension, designers have strong enough incentives to spend money on condition monitoring.
Many failures in building operations are not life-threatening. Consequently, the symptoms might not be obvious, or the residents could fail to address the problem with facility managers. People could respond by opening windows or putting on additional clothing without informing facility managers, for instance if the air conditioning malfunctions and cannot maintain a steady temperature within. This makes it challenging to gather and identify failure signs systematically.
The commissioning of building systems, including automation systems, often occurs when the facility is close to turn over to the owners or tenants. The sensors are often calibrated and verified only in the early stages of their lifetime, despite the trend of continual commissioning appearing to gain popularity. As a result, sensor readings may become less trustworthy over time, making it more challenging to operate systems and correctly gather reliable data for condition monitoring. While a flaw in, say, the air conditioning system is likely to have evident negative impacts, the flaw may go undetected for years. Consequently, it is challenging to relate known defects to BAS measurements. Contrarily, it may be difficult to ascertain the typical operating conditions because there may or may not have been an issue observed at any given time.
Additionally, data from BMS may be lost due to network, sensor, and power outages. Given that AHU systems vary throughout facilities, this is a concern. In reality, most research either employs recordings of manually caused errors in lab settings or databases of simulated failures. It can be challenging to simulate complex systems, such as air handling units with various controllable quantities and air flow mechanics, and it can be challenging to make decisions solely based on simulated data. Typically, a model developed using data from one system cannot readily apply to another. For instance, a machine learning model trained on sensor readings from normal operations and readings from various defects is unlikely to be an excellent match to identify and fix errors in a different environment. Assessing fault costs, including energy consumed and harm to indoor air quality, is made more difficult by a lack of understanding of the failure mechanism and its associated impacts. Evaluating the probable costs of failures vs the cost of preventative replacements would be extremely helpful in predictive maintenance of non-critical systems such as air handling units.
Another issue is that, even though buildings lose energy due to poor maintenance and operation, building owners lack strong incentives to invest in preventative maintenance. Monitoring and prognostics must be carried out with no additional expense or an articulated return on investment.
The inherent division of interests in the building sector presents another, less technical difficulty. A typical scenario is where one company owns the building, the occupants from a second company, and the facility mangers from a third company. Making money is the main goal for the building owner because the structure is often just one investment among many others. Maintenance may concentrate on keeping the systems functional to reduce future maintenance expenses and maximize energy expenditures while reducing maintenance costs. The building’s proper operation and the purity of the inside air are, nevertheless, what the residents care about. Maintenance companies are not compelled to provide services beyond what their clients, or building owners, want and pay for. In conclusion, building systems typically do not install sensors with condition monitoring in mind, and the current sensors may have only undergone a single calibration and verification after installation. Differentiating between normal operating conditions and malfunctioning operation is further complicated by the absence of historical data for both the particular system being monitored and other systems similar to it.
The Internet of Things system’s real-time monitoring data will be combined with the maintenance and operation management systems. By simulating all system-level operational components, fault detection enabled by Digital Twins in buildings can make confident predictions about how assets in facilities will behave in dynamic operational contexts. By formally documenting a building’s features and supplying important contextual information about an asset, BIM can promote data integration in a Digital Twin.
In addition, two of the many benefits of employing deep learning techniques are significant analytical powers in large data situations and the ability to identify complex defects in critical facilities in dynamic environments. There need to be more deep learning applications in predictive maintenance in civil infrastructure. IoT advancements hinder current predictive maintenance because conventional machine learning-based approaches require assistance evaluating HVAC large condition monitoring data. As a result, further work is needed to incorporate deep learning techniques into the field of HVAC predictive maintenance. Thus, it is crucial to create algorithms that require as little human involvement as possible in the pre-processing and analysis of HVAC condition monitoring data. Specifically, a CNN-based system can reliably detect faults without human knowledge by first transforming raw time-domain condition monitoring data into pictures. There is a need for greater study in this area due to high processing complexity, lengthy training times, and the possibility of reaching a local minimum.
More attention has to be paid to machine learning implementations in creating fault detection algorithms, which is a shortcoming of our study. Machine learning should be employed properly to forecast and select the best maintenance action by utilizing mathematical programming (e.g., mixed-integer), evolutionary computations, simulations, and logic-based models. As a result, routine maintenance may be automated even further. Predictive maintenance is a necessity, but it faces significant obstacles. These include the inability to accurately predict the behavior of building systems (such as faults and failures) in dynamic operational environments and the failure to account for system-level impacts in fault diagnosis analyses for real-world applications. Moreover, additional research is needed to improve interoperability across various data and information sources in BIM model operation and maintenance stages. IFC and COBie will be crucial in improving the integration of Internet of Things data with building maintenance and operation management systems in the BIM setting.
For fault detection, we used an analysis of 115 papers linked to the Digital Twin concept, and we found that an accurate description of the Digital Twin focuses on physical components, virtual models, connections between them, and a twin relationship between the two. From a physical standpoint, a Digital Twin may be used to model the physical aspects of a building or infrastructure. A wide range of modeling, simulation, calculation, and analytic tools, as well as complex algorithms, are used in digital twinning from the viewpoint of the virtual portion. Virtual and physical parts can be connected by using various technologies, such as laser scans and sensors to update geometric and non-geometric information on virtual parts to reflect the physical parts in time via the use of point cloud data and other data obtained from various sources such as sensors. Various Digital Twin applications for building failure detection are comprehensively covered in this paper. In light of the current study, this article provides some thoughts and ideas linked to Digital Twins in the building sector. Regarding defect identification and asset monitoring, the Digital Twin may play a significant role, and how to connect physical and virtual parts utilizing sophisticated connection technologies should be researched. It is also essential that the Digital Twin is used extensively in computation, analysis, optimization, and decision-making while employing a wide range of technologies. Finally, a Digital Twin is an effective tool for detecting and correcting faults. Civil engineering’s digital twinning will soon be elevated to a new level thanks to advances in AI, sensors, the Internet of Things (IoT), software, and hardware development.
HH: Conceptualization, Methodology, Software, Data curation, Formal analysis, Visualization, Writing—original draft, Writing—review and editing. HN: Supervision, Methodology, Resources, Writing—review and editing. AA: Conceptualization, Methodology, Visualization. PS: Supervision, Writing—review and editing. KS: Supervision, Writing—review and editing.
The work presented in this journal paper is funded by Scandinavian Sustainable Circular Construction (S2C) and the University of Agder.
The authors appreciate all of the contributors for their gracious support and input.
The authors declare that the research was conducted in the absence of any commercial or financial relationships that could be construed as a potential conflict of interest.
All claims expressed in this article are solely those of the authors and do not necessarily represent those of their affiliated organizations, or those of the publisher, the editors and the reviewers. Any product that may be evaluated in this article, or claim that may be made by its manufacturer, is not guaranteed or endorsed by the publisher.
Abadi, M. (2016). “TensorFlow: Learning functions at scale,” in Proceedings of the 21st ACM SIGPLAN International Conference on Functional Programming, Nara, Japan (New York, NY, USA: Association for Computing Machinery). ICFP 2016. doi:10.1145/2951913.2976746
Agarwal, Y., Balaji, B., Gupta, R., Lyles, J., Wei, M., and Weng, T. (2010). “Occupancy-driven energy management for smart building automation,” in Proceedings of the 2nd ACM Workshop on Embedded Sensing Systems for Energy-Efficiency in Building, Zurich, Switzerland, 2 November, 2010 (New York, NY, USA: Association for Computing Machinery), 1–6. BuildSys ’10. doi:10.1145/1878431.1878433
Alam, K. M., and El Saddik, A. (2017). C2PS: A digital twin architecture reference model for the cloud-based cyber-physical systems. IEEE Access 5, 2050–2062. doi:10.1109/ACCESS.2017.2657006
Alavi, H., Forcada, N., Bortolini, R., and Edwards, D. J. (2021). Enhancing occupants’ comfort through BIM-based probabilistic approach. Autom. Constr. 123, 103528. doi:10.1016/j.autcon.2020.103528
Allen, W. H., Rubaai, A., and Chawla, R. (2016). Fuzzy neural network-based health monitoring for HVAC system variable-air-volume unit. IEEE Trans. Ind. Appl. 52, 2513–2524. doi:10.1109/TIA.2015.2511160
Al-Qaseemi, S. A., Almulhim, H. A., Almulhim, M. F., and Chaudhry, S. R. (2016). “IoT architecture challenges and issues: Lack of standardization,” in 2016 Future Technologies Conference (FTC), San Francisco, 731–738. doi:10.1109/FTC.2016.7821686
Amato, G., Carrara, F., Falchi, F., Gennaro, C., Meghini, C., and Vairo, C. (2017). Deep learning for decentralized parking lot occupancy detection. Expert Syst. Appl. 72, 327–334. doi:10.1016/j.eswa.2016.10.055
Augustine, P. (2020). Chapter Four - the industry use cases for the Digital Twin idea. In Advances in computers, The digital twin paradigm for smarter systems and environments: The industry use cases. Editors. P. Raj, and P. Evangeline (Elsevier). 79–105. doi:10.1016/bs.adcom.2019.10.008
Baldini, G. (2022). Crisis leadership: Literature review and qualitative analysis of leadership behaviours in times of crisis. Università Ca’ Foscari Venezia (Accepted:02 21, 2022)
Batty, M. (2018). Digital twins. Environ. Plan. B Urban Anal. City Sci. 45, 817–820. doi:10.1177/2399808318796416
Boje, C., Guerriero, A., Kubicki, S., and Rezgui, Y. (2020). Towards a semantic construction digital twin: Directions for future research. Autom. Constr. 114, 103179. doi:10.1016/j.autcon.2020.103179
Bortolini, R., and Forcada, N. (2019). A probabilistic-based approach to support the comfort performance assessment of existing buildings. J. Clean. Prod. 237, 117720. doi:10.1016/j.jclepro.2019.117720
Burak Gunay, H., Shen, W., and Newsham, G. (2019). Data analytics to improve building performance: A critical review. Autom. Constr. 97, 96–109. doi:10.1016/j.autcon.2018.10.020
Chandola, V., Banerjee, A., and Kumar, V. (2009). Anomaly detection: A survey. ACM Comput. Surv. 41 (3), 1–58. doi:10.1145/1541880.1541882
Chen, W., Chen, K., Cheng, J. C. P., Wang, Q., and Gan, V. J. L. (2018). BIM-based framework for automatic scheduling of facility maintenance work orders. Automation Constr. 91, 15–30. doi:10.1016/j.autcon.2018.03.007
Chen, L., Xie, X., Lu, Q., Parlikad, A. K., Pitt, M., and Yang, J. (2021). Gemini principles-based digital twin maturity model for asset management. Sustainability 13, 8224. doi:10.3390/su13158224
Chen, J., Zhang, L., Li, Y., Shi, Y., Gao, X., and Hu, Y. (2022a). A review of computing-based automated fault detection and diagnosis of heating, ventilation and air conditioning systems. Renew. Sustain. Energy Rev. 161, 112395. doi:10.1016/j.rser.2022.112395
Chen, K., Zhu, X., Anduv, B., Jin, X., and Du, Z. (2022b). Digital twins model and its updating method for heating, ventilation and air conditioning system using broad learning system algorithm. Energy 251, 124040. doi:10.1016/j.energy.2022.124040
Cheng, J. C. P., Chen, W., Chen, K., and Wang, Q. (2020). Data-driven predictive maintenance planning framework for MEP components based on BIM and IoT using machine learning algorithms. Autom. Constr. 112, 103087. doi:10.1016/j.autcon.2020.103087
Chin, S., Yoon, S., Kim, Y.-S., Ryu, J., Choi, C., and Cho, C.-Y. (2012). Realtime 4D CAD = RFID for project progress management. CRC, 1–10. doi:10.1061/40754(183)33
Ciaburro, G., and Venkateswaran, B. (2017). Neural Networks with R: Smart models using CNN, RNN, deep learning, and artificial intelligence principles. Birmingham: Packt Publishing Ltd. Google-Books-ID: IppGDwAAQBAJ.
Costa, A., Keane, M. M., Torrens, J. I., and Corry, E. (2013). Building operation and energy performance: Monitoring, analysis and optimisation toolkit. Appl. Energy 101, 310–316. doi:10.1016/j.apenergy.2011.10.037
Cui, J., and Wang, S. (2005). A model-based online fault detection and diagnosis strategy for centrifugal chiller systems. Int. J. Therm. Sci. 44, 986–999. doi:10.1016/j.ijthermalsci.2005.03.004
Czerniawski, T., and Leite, F. (2018). “3DFacilities: Annotated 3D reconstructions of building facilities,” in Advanced computing strategies for engineering. Lecture notes in computer science. Editors I. F. C. Smith, and B. Domer (Cham: Springer International Publishing), 186–200. doi:10.1007/978-3-319-91635-4_10
Deng, Z., and Chen, Q. (2020). Development and validation of a smart HVAC control system for multi-occupant offices by using occupants’ physiological signals from wristband. Energy Build. 214, 109872. doi:10.1016/j.enbuild.2020.109872
Deng, M., Menassa, C. C., and Kamat, V. R. (2021). From BIM to digital twins: a systematic review of the evolution of intelligent building representations in the AEC-FM industry. ITcon. 26, 58–83. doi:10.36680/j.itcon.2021.005
Dey, D., and Dong, B. (2016). A probabilistic approach to diagnose faults of air handling units in buildings. Energy Build. 130, 177–187. doi:10.1016/j.enbuild.2016.08.017
Ding, L., Drogemuller, R., Akhurst, P., Hough, R., Bull, S., and Linning, C. (2009). “Towards sustainable facilities management,” in Technology, design and process innovation in the built environment. Editors P. Newton, K. Hampson, and R. Drogemuller (United Kingdom: Taylor & Francis), 373–392.
Dixit, M. K., Venkatraj, V., Ostadalimakhmalbaf, M., Pariafsai, F., and Lavy, S. (2019). Integration of facility management and building information modeling (BIM): A review of key issues and challenges. Facilities 37, 455–483. doi:10.1108/F-03-2018-0043
Dong, B., O’Neill, Z., and Li, Z. (2014). A BIM-enabled information infrastructure for building energy Fault Detection and Diagnostics. Autom. Constr. 44, 197–211. doi:10.1016/j.autcon.2014.04.007
Durdyev, S., Ashour, M., Connelly, S., and Mahdiyar, A. (2022). Barriers to the implementation of building information modelling (BIM) for facility management. J. Build. Eng. 46, 103736. doi:10.1016/j.jobe.2021.103736
Eastman, C., Teicholz, P., Sacks, R., and Liston, K. (2011). BIM Handbook: A guide to building information modeling for owners, managers, designers, engineers and contractors. Hoboken, NJ: Wiley.
East, B. (2013). “Using COBie,” in BIM for facility managers (John Wiley & Sons), 107–143. doi:10.1002/9781119572633
Ferretti, N. M., Galler, M. A., Bushby, S. T., and Choinière, D. (2015). Evaluating the performance of diagnostic agent for building operation (DABO) and HVAC-cx tools using the virtual cybernetic building testbed. Sci. Technol. Built Environ. 21, 1154–1164. doi:10.1080/23744731.2015.1077670
Fisera, R., and Stluka, P. (2012). Performance monitoring of the refrigeration system with minimum set of sensors. zenodo. doi:10.5281/zenodo.1057169
Fjeld, T. M. B. (2020). Digital Twin - towards a joint understanding within the AEC/FM sector. NTNU (Accepted 09 20, 2021).
Fuller, A., Fan, Z., Day, C., and Barlow, C. (2020). Digital twin: Enabling technologies, challenges and open research. IEEE Access 8, 108952–108971. doi:10.1109/ACCESS.2020.2998358
Garramone, M., Moretti, N., Scaioni, M., Ellul, C., Re Cecconi, F., and Dejaco, M. C. (2020). “Bim and gis integration for infrastructure asset management: A bibliometric analysis,” in ISPRS annals of the photogrammetry, remote sensing and spatial information Sciences (Copernicus GmbH), VI-4-W1-2020, 77–84. doi:10.5194/isprs-annals-VI-4-W1-2020-77-2020
Gobble, M. M. (2013). Big data: The next big thing in innovation. Res. Technol. Manag. 56, 64–67. doi:10.5437/08956308X5601005
Granderson, J., Bonvini, M., Piette, M. A., Page, J., Lin, G., and Hu, R. L. (2016). “Can we practically bring physics-based modeling into operational analytics tools?,” in the Proceedings of the 2016 ACEEE Summer Study on Energy Efficiency in Buildings, Pacific Grove, CA, August 2016.
Grieves, M. (2015). Digital twin: Manufacturing excellence through virtual factory replication. J. Eng. Sci. Res. [NC-Rase 18], 6–15. doi:10.5281/zenodo.1493930
Haag, S., and Anderl, R. (2018). Digital twin – proof of concept. Manuf. Lett. 15, 64–66. doi:10.1016/j.mfglet.2018.02.006
Halmetoja, E. (2022). “The role of digital twins and their application for the built environment,” in Industry 4.0 for the built environment: Methodologies, technologies and skills. Editors M. Bolpagni, R. Gavina, and D. Ribeiro (Cham: Springer International Publishing), 415–442. Structural Integrity. doi:10.1007/978-3-030-82430-3_18
Hammad, A. W. (2019). Minimising the deviation between predicted and actual building performance via use of neural networks and BIM. Buildings 9, 131. doi:10.3390/buildings9050131
Hong, T., Wang, Z., Luo, X., and Zhang, W. (2020). State-of-the-art on research and applications of machine learning in the building life cycle. Energy Build. 212, 109831. doi:10.1016/j.enbuild.2020.109831
Hosamo, H. H., Imran, A., Cardenas-Cartagena, J., Svennevig, P. R., Svidt, K., and Nielsen, H. K. (2022a). A review of the digital twin technology in the AEC-FM industry. Adv. Civ. Eng. 2022, 1–17. doi:10.1155/2022/2185170
Hosamo, H. H., Svennevig, P. R., Svidt, K., Han, D., and Nielsen, H. K. (2022b). A Digital Twin predictive maintenance framework of air handling units based on automatic fault detection and diagnostics. Energy Build. 261, 111988. doi:10.1016/j.enbuild.2022.111988
Howell, S., Rezgui, Y., Hippolyte, J.-L., Jayan, B., and Li, H. (2017). Towards the next generation of smart grids: Semantic and holonic multi-agent management of distributed energy resources. Renew. Sustain. Energy Rev. 77, 193–214. doi:10.1016/j.rser.2017.03.107
Jiang, F., Ma, L., Broyd, T., and Chen, K. (2021). Digital twin and its implementations in the civil engineering sector. Autom. Constr. 130, 103838. doi:10.1016/j.autcon.2021.103838
Jones, D., Snider, C., Nassehi, A., Yon, J., and Hicks, B. (2020). Characterising the digital twin: A systematic literature review. CIRP J. Manuf. Sci. Technol. 29, 36–52. doi:10.1016/j.cirpj.2020.02.002
Karthikeyan, R. R., and Raghu, B. (2020). A firefly optimized lstm risk detection & prediction model for IoT enabled super market. Indian J. Comput. Sci. Eng. 11, 851–858. doi:10.21817/indjcse/2020/v11i6/201106216
Katipamula, S., and Brambley, M. R. (2005). Review article: Methods for fault detection, diagnostics, and prognostics for building systems—a review, Part I. HVAC. R Res. 11, 3–25. doi:10.1080/10789669.2005.10391123
Keller, C. (2012). “CAFM/IWMS—balancing technology, processes, and objectives,” in Technology for facility managers (John Wiley & Sons), 1–20. doi:10.1002/9781119572626
Ketkar, N. (2017). “Introduction to Keras,” in Deep learning with Python: A hands-on introduction. Editor N. Ketkar (Berkeley, CA: Apress), 97–111. doi:10.1007/978-1-4842-2766-4_7
Kim, W., and Katipamula, S. (2018). A review of fault detection and diagnostics methods for building systems. Sci. Technol. Built Environ. 24, 3–21. doi:10.1080/23744731.2017.1318008
Kim, S., Song, Y., Sung, Y., and Seo, D. (2019). Development of a consecutive occupancy estimation framework for improving the energy demand prediction performance of building energy modeling tools. Energies 12, 433. doi:10.3390/en12030433
Korpela, J., Miettinen, R., Salmikivi, T., and Ihalainen, J. (2015). The challenges and potentials of utilizing building information modelling in facility management: the case of the center for properties and facilities of the university of helsinki. Constr. Manag. Econ. 33, 3–17. doi:10.1080/01446193.2015.1016540
Krämer, M., and Besenyő, Z. (2018). Towards digitalization of building operations with BIM. IOP Conf. Ser. Mater. Sci. Eng. 365, 022067. doi:10.1088/1757-899X/365/2/022067
Kumar, K., Parida, M., and Katiyar, V. K. (2013). Short term traffic flow prediction for a non urban highway using artificial neural network. Procedia - Soc. Behav. Sci. 104, 755–764. doi:10.1016/j.sbspro.2013.11.170
Kuster, C., Rezgui, Y., and Mourshed, M. (2017). Electrical load forecasting models: A critical systematic review. Sustain. Cities Soc. 35, 257–270. doi:10.1016/j.scs.2017.08.009
Lee, C. K., Chan, H. Y. S., Poon, C. Y. C., Yip, K. M. G., and Yuen, P. H. F. (2019). Smart BIM-AM journey to green buildings. IOP Conf. Ser. Earth Environ. Sci. 290, 012050. doi:10.1088/1755-1315/290/1/012050
Li, S., and Wen, J. (2014). A model-based fault detection and diagnostic methodology based on PCA method and wavelet transform. Energy Build. 68, 63–71. doi:10.1016/j.enbuild.2013.08.044
Li, C., Ding, Z., Zhao, D., Yi, J., and Zhang, G. (2017). Building energy consumption prediction: An extreme deep learning approach. Energies 10, 1525. doi:10.3390/en10101525
Lu, Q., Chen, L., Lee, S., and Zhao, X. (2018). Activity theory-based analysis of BIM implementation in building O&M and first response. Autom. Constr. 85, 317–332. doi:10.1016/j.autcon.2017.10.017
Lu, Q., Parlikad, A. K., Woodall, P., Don Ranasinghe, G., Xie, X., Liang, Z., et al. (2020a). Developing a digital twin at building and city levels: Case study of west cambridge campus. J. Manage. Eng. 36, 05020004. doi:10.1061/(ASCE)ME.1943-5479.0000763
Lu, Q., Xie, X., Heaton, J., Parlikad, A. K., and Schooling, J. (2020b). “From BIM towards digital twin: Strategy and future development for smart asset management,” in Service oriented, holonic and multi-agent manufacturing systems for industry of the future. Studies in computational intelligence. Editors T. Borangiu, D. Trentesaux, P. Leitão, A. Giret Boggino, and V. Botti (Cham: Springer International Publishing), 392–404. doi:10.1007/978-3-030-27477-1_30
Lu, Q., Xie, X., Parlikad, A. K., and Schooling, J. M. (2020c). Digital twin-enabled anomaly detection for built asset monitoring in operation and maintenance. Autom. Constr. 118, 103277. doi:10.1016/j.autcon.2020.103277
Madni, A. M., Madni, C. C., and Lucero, S. D. (2019). Leveraging digital twin technology in model-based systems engineering. Systems 7, 7. doi:10.3390/systems7010007
Magomadov, V. S. (2020). The digital twin technology and its role in manufacturing. IOP Conf. Ser. Mater. Sci. Eng. 862, 032080. doi:10.1088/1757-899X/862/3/032080
Martani, C., Lee, D., Robinson, P., Britter, R., and Ratti, C. (2012). ENERNET: Studying the dynamic relationship between building occupancy and energy consumption. Energy Build. 47, 584–591. doi:10.1016/j.enbuild.2011.12.037
Marzouk, M., and Zaher, M. (2020). Artificial intelligence exploitation in facility management using deep learning. Constr. Innov. 20, 609–624. doi:10.1108/CI-12-2019-0138
Marzouk, M., Azab, S., and Metawie, M. (2018). BIM-based approach for optimizing life cycle costs of sustainable buildings. J. Clean. Prod. 188, 217–226. doi:10.1016/j.jclepro.2018.03.280
Mattera, C. G., Quevedo, J., Escobet, T., Shaker, H. R., and Jradi, M. (2018). A method for fault detection and diagnostics in ventilation units using virtual sensors. Sensors (Basel, Switz.) 18, 3931. doi:10.3390/s18113931
Mijic, N., Sestic, M., and Koljancic, M. (2017). “CAD—GIS BIM integration—case study of banja luka city center,” in Advanced technologies, systems, and applications. Lecture notes in networks and systems. Editors M. Hadžikadić, and S. Avdaković (Cham: Springer International Publishing), 267–281. doi:10.1007/978-3-319-47295-9_22
Motamedi, A., Hammad, A., and Asen, Y. (2014). Knowledge-assisted BIM-based visual analytics for failure root cause detection in facilities management. Automation Constr. 43, 73–83. doi:10.1016/j.autcon.2014.03.012
Motawa, I., and Almarshad, A. (2013). A knowledge-based BIM system for building maintenance. Autom. Constr. 29, 173–182. doi:10.1016/j.autcon.2012.09.008
Mulumba, T., Afshari, A., Yan, K., Shen, W., and Norford, L. K. (2015). Robust model-based fault diagnosis for air handling units. Energy Build. 86, 698–707. doi:10.1016/j.enbuild.2014.10.069
Mutis, I., Ambekar, A., and Joshi, V. (2020). Real-time space occupancy sensing and human motion analysis using deep learning for indoor air quality control. Autom. Constr. 116, 103237. doi:10.1016/j.autcon.2020.103237
Narayanaswamy, B., Balaji, B., Gupta, R., and Agarwal, Y. (2014). “Data driven investigation of faults in HVAC systems with model, cluster and compare (MCC),” in Proceedings of the 1st ACM Conference on Embedded Systems for Energy-Efficient Buildings, New York (New York, NY, USA: Association for Computing Machinery), 50–59. BuildSys ’14. doi:10.1145/2674061.2674067
Nassif, N., Moujaes, S., and Zaheeruddin, M. (2008). Self-tuning dynamic models of HVAC system components. Energy Build. 40, 1709–1720. doi:10.1016/j.enbuild.2008.02.026
National Research Council (2007). Core competencies for federal facilities asset management through 2020: Transformational strategies. National Academies Press.
Nehasil, O., Dobiášová, L., Mazanec, V., and Široký, J. (2021). Versatile AHU fault detection – design, field validation and practical application. Energy Build. 237, 110781. doi:10.1016/j.enbuild.2021.110781
Nojedehi, P., O’Brien, W., and Gunay, H. B. (2022). A methodology to integrate maintenance management systems and BIM to improve building management. Sci. Technol. Built Environ. 0, 1097–1114. doi:10.1080/23744731.2022.2052668
O’Neill, Z., Pang, X., Shashanka, M., Haves, P., and Bailey, T. (2014). Model-based real-time whole building energy performance monitoring and diagnostics. J. Build. Perform. Simul. 7, 83–99. doi:10.1080/19401493.2013.777118
Onwuegbuzie, A. J., Leech, N. L., and Collins, K. M. T. (2012). Qualitative analysis techniques for the review of the literature. Qual. Rep. 17, 1–28. doi:10.46743/2160-3715/2012.1754
Opoku, D.-G. J., Perera, S., Osei-Kyei, R., and Rashidi, M. (2021). Digital twin application in the construction industry: A literature review. J. Build. Eng. 40, 102726. doi:10.1016/j.jobe.2021.102726
Oraee, M., Hosseini, M. R., Papadonikolaki, E., Palliyaguru, R., and Arashpour, M. (2017). Collaboration in BIM-based construction networks: A bibliometric-qualitative literature review. Int. J. Proj. Manag. 35, 1288–1301. doi:10.1016/j.ijproman.2017.07.001
Padilla, M., and Choinière, D. (2015). A combined passive-active sensor fault detection and isolation approach for air handling units. Energy Build. 99, 214–219. doi:10.1016/j.enbuild.2015.04.035
Page, M. J., McKenzie, J. E., Bossuyt, P. M., Boutron, I., Hoffmann, T. C., Mulrow, C. D., et al. (2021). Updating guidance for reporting systematic reviews: Development of the PRISMA 2020 statement. J. Clin. Epidemiol. 134, 103–112. doi:10.1016/j.jclinepi.2021.02.003
Pärn, E. A., and Edwards, D. J. (2017). Conceptualising the FinDD API plug-in: A study of BIM-FM integration. Autom. Constr. 80, 11–21. doi:10.1016/j.autcon.2017.03.015
Pärn, E. A., Edwards, D. J., and Sing, M. C. P. (2017). The building information modelling trajectory in facilities management: A review. Automation Constr. 75, 45–55. doi:10.1016/j.autcon.2016.12.003
Pärn, E. A., Edwards, D. J., and Sing, M. C. P. (2018). Origins and probabilities of MEP and structural design clashes within a federated BIM model. Autom. Constr. 85, 209–219. doi:10.1016/j.autcon.2017.09.010
Parsanezhad, P., and Dimyadi, J. (2013). Effective facility management and operations via a BIM-based integrated information system. CIB Working Commission W070 Facilities Management in collaboration with W111 Usability and W118 Clients and Users. Available at: https://hdl.handle.net/10652/3034 (Accepted 09 24, 2015).
Paszke, A., Gross, S., Massa, F., Lerer, A., Bradbury, J., Chanan, G., et al. (2019). “PyTorch: An imperative style, high-performance deep learning library,” in Advances in neural information processing systems (Curran Associates, Inc.), 32.
Patacas, J., Dawood, N., Vukovic, V., and Kassem, M. (2015). BIM for facilities management: evaluating BIM standards in asset register creation and service life. J. Inf. Technol. Constr. 20, 313–331.
Patterson, E. A., Taylor, R. J., and Bankhead, M. (2016). A framework for an integrated nuclear digital environment. Prog. Nucl. Energy 87, 97–103. doi:10.1016/j.pnucene.2015.11.009
Perianes-Rodriguez, A., Waltman, L., and van Eck, N. J. (2016). Constructing bibliometric networks: A comparison between full and fractional counting. J. Inf. 10, 1178–1195. doi:10.1016/j.joi.2016.10.006
Plageras, A. P., Psannis, K. E., Stergiou, C., Wang, H., and Gupta, B. B. (2018). Efficient IoT-based sensor BIG Data collection–processing and analysis in smart buildings. Future Gener. Comput. Syst. 82, 349–357. doi:10.1016/j.future.2017.09.082
Ploennigs, J., Chen, B., Schumann, A., and Brady, N. (2013). “Exploiting generalized additive models for diagnosing abnormal energy use in buildings,” in Proceedings of the 5th ACM workshop on embedded systems for energy-efficient buildings, Roma, Italy (New York, NY, USA: Association for Computing Machinery), 1–8. BuildSys’13. doi:10.1145/2528282.2528291
Poon, K. H., Wong, P. K.-Y., and Cheng, J. C. P. (2022). Long-time gap crowd prediction using time series deep learning models with two-dimensional single attribute inputs. Adv. Eng. Inf. 51, 101482. doi:10.1016/j.aei.2021.101482
Pour Rahimian, F., Seyedzadeh, S., Oliver, S., Rodriguez, S., and Dawood, N. (2020). On-demand monitoring of construction projects through a game-like hybrid application of BIM and machine learning. Automation Constr. 110, 103012. doi:10.1016/j.autcon.2019.103012
Qi, Q., and Tao, F. (2018). Digital twin and big data towards smart manufacturing and industry 4.0: 360 degree comparison. IEEE Access 6, 3585–3593. doi:10.1109/ACCESS.2018.2793265
Qin, J., and Wang, S. (2005). A fault detection and diagnosis strategy of VAV air-conditioning systems for improved energy and control performances. Energy Build. 37, 1035–1048. doi:10.1016/j.enbuild.2004.12.011
Qiuchen Lu, V., Parlikad, A. K., Woodall, P., Ranasinghe, G. D., and Heaton, J. (2019). “Developing a dynamic digital twin at a building level: using cambridge campus as case study,” in International Conference on Smart Infrastructure and Construction 2019 (ICSIC) (ICE Publishing), 67–75. Cambridge Centre for Smart Infrastructure & Construction. doi:10.1680/icsic.64669.067
Quach, B., and Wenström, O. (2022). Research on barriers with BIM implementation in facilities management: An interview study to investigate the main challenges and prospects of BIM in FM. Available at: https://hdl.handle.net/20.500.12380/305540 (Accepted 09 05, 2022).
Radhakrishnan, R., Nikovski, D., Peker, K., and Divakaran, A. (2006). “A comparison between polynomial and locally weighted regression for fault detection and diagnosis of HVAC equipment,” in IECON 2006 - 32nd Annual Conference on IEEE Industrial Electronics, Paris, France, 3668–3673. doi:10.1109/IECON.2006.347601
Sacks, R., Brilakis, I., Pikas, E., Xie, H. S., and Girolami, M. (2020). Construction with digital twin information systems. Cambridge University Press. Data-Centric Engineering. (Accepted 12 01, 2020).
Schluse, M., Priggemeyer, M., Atorf, L., and Rossmann, J. (2018). Experimentable digital twins—streamlining simulation-based systems engineering for industry 4.0. IEEE Trans. Ind. Inf. 14, 1722–1731. doi:10.1109/TII.2018.2804917
Seyis, S. (2019). Pros and cons of using building information modeling in the AEC industry. J. Constr. Eng. Manag. 145, 04019046. doi:10.1061/(asce)co.1943-7862.0001681
Shi, Z., and O’Brien, W. (2019). Development and implementation of automated fault detection and diagnostics for building systems: A review. Autom. Constr. 104, 215–229. doi:10.1016/j.autcon.2019.04.002
Sonetti, G., Naboni, E., and Brown, M. (2018). Exploring the potentials of ICT tools for human-centric regenerative design. Sustainability 10, 1217. doi:10.3390/su10041217
Srewil, Y., and Scherer, R. J. (2013). “Effective construction process monitoring and control through a collaborative cyber-physical approach,” in Collaborative systems for reindustrialization. IFIP advances in information and communication technology. Editors L. M. Camarinha-Matos, and R. J. Scherer (Berlin, Heidelberg: Springer), 172–179. doi:10.1007/978-3-642-40543-3_19
Sun, B., Luh, P. B., Jia, Q.-S., O’Neill, Z., and Song, F. (2014). Building energy doctors: An SPC and kalman filter-based method for system-level fault detection in HVAC systems. IEEE Trans. Autom. Sci. Eng. 11, 215–229. doi:10.1109/TASE.2012.2226155
Tao, F., Zhang, H., Liu, A., and Nee, A. Y. C. (2019). Digital twin in industry: State-of-the-Art. IEEE Trans. Ind. Inf. 15, 2405–2415. doi:10.1109/TII.2018.2873186
Teza, G., Cola, S., Brezzi, L., and Galgaro, A. (2022). Wadenow: A matlab Toolbox for early forecasting of the velocity trend of a rainfall-triggered landslide by means of continuous wavelet transform and deep learning. Geosciences 12, 205. doi:10.3390/geosciences12050205
Tongal, H., and Booij, M. J. (2018). Simulation and forecasting of streamflows using machine learning models coupled with base flow separation. J. Hydrol. 564, 266–282. doi:10.1016/j.jhydrol.2018.07.004
Tuegel, E. J., Ingraffea, A. R., Eason, T. G., and Spottswood, S. M. (2011). Reengineering aircraft structural life prediction using a digital twin. Int. J. Aerosp. Eng. 2011, 1–14. doi:10.1155/2011/154798
Wang, H., Chen, Y., Chan, C. W. H., and Qin, J. (2012). An online fault diagnosis tool of VAV terminals for building management and control systems. Autom. Constr. 22, 203–211. doi:10.1016/j.autcon.2011.06.018
Wei, Y., and Akinci, B. (2019). A vision and learning-based indoor localization and semantic mapping framework for facility operations and management. Autom. Constr. 107, 102915. doi:10.1016/j.autcon.2019.102915
Weimer, J., Ahmadi, S. A., Araujo, J., Mele, F. M., Papale, D., Shames, I., et al. (2012). “Active actuator fault detection and diagnostics in HVAC systems,” in Proceedings of the Fourth ACM Workshop on Embedded Sensing Systems for Energy-Efficiency in Buildings, Toronto, ON (New York, NY, USA: Association for Computing Machinery), 107–114. BuildSys ’12. doi:10.1145/2422531.2422551
Wetzel, E. M., and Thabet, W. Y. (2015). The use of a BIM-based framework to support safe facility management processes. Autom. Constr. 60, 12–24. doi:10.1016/j.autcon.2015.09.004
Wong, J. K. W., Ge, J., and He, S. X. (2018). Digitisation in facilities management: A literature review and future research directions. Autom. Constr. 92, 312–326. doi:10.1016/j.autcon.2018.04.006
Wu, S., Hou, L., Zhang, G. K., and Chen, H. (2022). Real-time mixed reality-based visual warning for construction workforce safety. Autom. Constr. 139, 104252. doi:10.1016/j.autcon.2022.104252
Xia, L., Lu, J., Zhang, H., Xu, M., and Li, Z. (2022). Construction and application of smart factory digital twin system based on DTME. Int. J. Adv. Manuf. Technol. 120, 4159–4178. doi:10.1007/s00170-022-08971-1
Yan, R., Ma, Z., Kokogiannakis, G., and Zhao, Y. (2016a). A sensor fault detection strategy for air handling units using cluster analysis. Autom. Constr. 70, 77–88. doi:10.1016/j.autcon.2016.06.005
Yan, R., Ma, Z., Zhao, Y., and Kokogiannakis, G. (2016b). A decision tree based data-driven diagnostic strategy for air handling units. Energy Build. 133, 37–45. doi:10.1016/j.enbuild.2016.09.039
Yang, C., Shen, W., Chen, Q., and Gunay, B. (2018). A practical solution for HVAC prognostics: Failure mode and effects analysis in building maintenance. J. Build. Eng. 15, 26–32. doi:10.1016/j.jobe.2017.10.013
Yu, D., Li, H., and Yang, M. (2011). A virtual supply airflow rate meter for rooftop air-conditioning units. Build. Environ. 46, 1292–1302. doi:10.1016/j.buildenv.2010.12.017
Yu, Y., Woradechjumroen, D., and Yu, D. (2014). A review of fault detection and diagnosis methodologies on air-handling units. Energy Build. 82, 550–562. doi:10.1016/j.enbuild.2014.06.042
Yuan, X., Anumba, C. J., and Parfitt, M. K. (2016). Cyber-physical systems for temporary structure monitoring. Autom. Constr. 66, 1–14. doi:10.1016/j.autcon.2016.02.005
Yusen, X., Bondaletova, N. F., Kovalev, V., and Komrakov, A. (2018). “Digital twin concept in managing industrial capital construction projects life cycle,” in 2018 Eleventh International Conference ”Management of large-scale system development” (MLSD), Russia, Moscow, 1–3. doi:10.1109/MLSD.2018.855186
Yuwono, M., Guo, Y., Wall, J., Li, J., West, S., Platt, G., et al. (2015). Unsupervised feature selection using swarm intelligence and consensus clustering for automatic fault detection and diagnosis in Heating Ventilation and Air Conditioning systems. Appl. Soft Comput. 34, 402–425. doi:10.1016/j.asoc.2015.05.030
Zhang, L., and Leach, M. (2022). Evaluate the impact of sensor accuracy on model performance in data-driven building fault detection and diagnostics using Monte Carlo simulation. Build. Simul. 15, 769–778. doi:10.1007/s12273-021-0833-4
Zhang, L., Leach, M., Bae, Y., Cui, B., Bhattacharya, S., Lee, S., et al. (2021). Sensor impact evaluation and verification for fault detection and diagnostics in building energy systems: A review. Adv. Appl. Energy 3, 100055. doi:10.1016/j.adapen.2021.100055
Zhao, Y., Wen, J., and Wang, S. (2015). Diagnostic Bayesian networks for diagnosing air handling units faults – Part II: Faults in coils and sensors. Appl. Therm. Eng. 90, 145–157. doi:10.1016/j.applthermaleng.2015.07.001
Zhao, Y., Wen, J., Xiao, F., Yang, X., and Wang, S. (2017). Diagnostic Bayesian networks for diagnosing air handling units faults – part I: Faults in dampers, fans, filters and sensors. Appl. Therm. Eng. 111, 1272–1286. doi:10.1016/j.applthermaleng.2015.09.121
Zheng, Y., Yang, S., and Cheng, H. (2019). An application framework of digital twin and its case study. J. Ambient. Intell. Humaniz. Comput. 10, 1141–1153. doi:10.1007/s12652-018-0911-3
Zou, H., Wu, Y., Zhang, H., and Zhan, Y. (2020). “Short-term traffic flow prediction based on PCC-BiLSTM,” in 2020 International Conference on Computer Engineering and Application (ICCEA), Guangzhou, China, 489–493. doi:10.1109/ICCEA50009.2020.00110
AHU Air handeling unit
AMS Advanced measurement systems
ANN Artificial neural network
APAR AHU performance assessment rule
API Application Programming Interface
BIM Building information modeling
BMS Building management system
CMMS Computerized maintenance management system
CNN Convolutional neural network
COBie Construction operations building information exchange
DL Deep learning
FM Facility management
HVAC Heating, ventilation, and air conditioning
IFC Industry foundation classes
IoT Internet of things
LR Linear regression
ML Machine learning
RF Random forest
SMS Smarter metering services
VAV Variable air volume
Keywords: BIM, fault detection, predictive maintenance, IoT, Digital Twin
Citation: Hosamo HH, Nielsen HK, Alnmr AN, Svennevig PR and Svidt K (2022) A review of the Digital Twin technology for fault detection in buildings. Front. Built Environ. 8:1013196. doi: 10.3389/fbuil.2022.1013196
Received: 06 August 2022; Accepted: 28 October 2022;
Published: 09 November 2022.
Edited by:
Abid Nadeem, Nazarbayev University, KazakhstanReviewed by:
Natasa Nord, Norwegian University of Science and Technology, NorwayCopyright © 2022 Hosamo, Nielsen, Alnmr, Svennevig and Svidt. This is an open-access article distributed under the terms of the Creative Commons Attribution License (CC BY). The use, distribution or reproduction in other forums is permitted, provided the original author(s) and the copyright owner(s) are credited and that the original publication in this journal is cited, in accordance with accepted academic practice. No use, distribution or reproduction is permitted which does not comply with these terms.
*Correspondence: Haidar Hosamo Hosamo, aGFpZGFyaEB1aWEubm8=
Disclaimer: All claims expressed in this article are solely those of the authors and do not necessarily represent those of their affiliated organizations, or those of the publisher, the editors and the reviewers. Any product that may be evaluated in this article or claim that may be made by its manufacturer is not guaranteed or endorsed by the publisher.
Research integrity at Frontiers
Learn more about the work of our research integrity team to safeguard the quality of each article we publish.