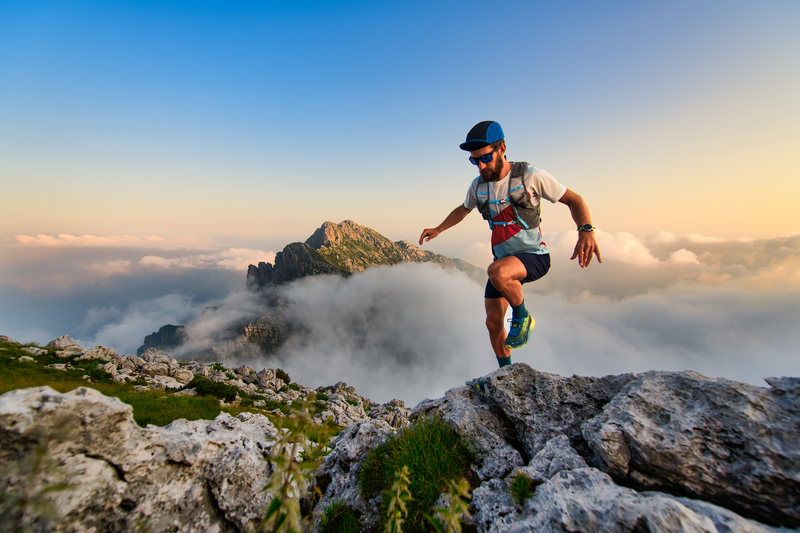
95% of researchers rate our articles as excellent or good
Learn more about the work of our research integrity team to safeguard the quality of each article we publish.
Find out more
ORIGINAL RESEARCH article
Front. Built Environ. , 27 September 2022
Sec. Transportation and Transit Systems
Volume 8 - 2022 | https://doi.org/10.3389/fbuil.2022.1000289
This article is part of the Research Topic Data Science Methods for Solving Real-World Problems in Transportation, Security and Beyond View all 6 articles
Increasingly more studies have implemented random regret minimization (RRM) as an alternative to random utility maximization (RUM) for modeling travelers’ choice-making behaviors. While for RUM, the focus is on utility maximization, for RRM the emphasis is on the regret of not selecting the best alternative. This study presented RRM and RUM for modeling actions made by drivers that resulted in crashes. The RRM method was considered in this study as the actions made before crashes might be the resultants of avoidance of regrets across the alternatives rather than the maximization of the utility related to the considered attributes. In addition, we extended the considered models to account for the unobserved heterogeneity in the datasets. Finally, we gave more flexibility to our model by changing the means of random parameters based on some observed attributes. This is one of the earliest studies, which considered the technique in the context of traffic safety for modeling drivers’ action while accounting for heterogeneity in the dataset by means of the random parameter. In addition, we considered the impact of inclusion of various predictors in the model fit of RRM and RUM. The results showed that while the standard RUM model outperforms the RRM model, the standard mixed models and the mixed models accounting for observed heterogeneity outperform the other techniques. As expected from the methodological structure of RRM, we found that the RRM performance is very sensitive to the included attributes. For instance, we found that by excluding the attributes of drivers’ condition and drivers under influence (DUI), the RRM model significantly outperforms the RUM model. The impact might be linked to the fact that when drivers are under abnormal conditions or influenced by drugs or alcohol, based on the sum of pairwise regret comparison, the inclusion of those attributes deteriorates the goodness-of-fit of the RRM model. It is possible that those parameters do not make a difference on regret pairwise comparison related to alternatives. The discussions at the end of this article examined possible reasons behind this performance.
Regret is a well-known measure in the evaluation of choice-making behaviors (Loomes, 1988). The regret theory (RT) was originally presented to describe the changes in the utility assessment product as the result of comparison across the obtained outcome with the outcome of non-chosen alternatives (Loomes and Sugden, 1982) (Bell, 1982).
The idea of the RRM model originated from the psychology concept that how much a position of a person would have been, if he decided and selected a choice differently (Loomes and Sugden, 1982) (Bell, 1982). In other words, in the model the attribute across various responses would be considered and would be mapped into regret.
Although more recent studies have explored the application of the random regret minimization (RRM) technique, its application in drivers’ attitude and actions, especially from the perspective of traffic safety, is still very limited. But when drivers are on the roadway, they are constantly making choices regarding their next maneuvers. For instance, drivers make decisions about their speed, lane change, or avoiding improper maneuvers while evaluating the regret associated with each alternative or pairwise comparison. It is likely that those actions are influenced by various attributes, such as environmental factors or the driver’s emotional or demographic conditions.
The importance of regret in the decision-making process of drivers in the context of traffic safety has been studied in a limited number of studies. For instance, by surveying some people who drive to work, it was found that regret is one of the major factors dissuading drivers from speeding (Newnam et al., 2004). The importance of regret after involvement in crashes was evaluated (Peng et al., 2019), and the results suggested that road rage is caused by the loss of emotional control. Also, it was highlighted that regret is negatively associated with speeding intentions across the motorists.
A limited number of studies have been conducted in the field of traffic safety that examine drivers’ maneuvers related to crashes by the RRM model. The RRM model, a plausible behavioral approach for the driver’s avoidance maneuver choice in response to critical traffic events was employed (Prato et al., 2014). Five critical drivers’ behaviors such as “braking,” “steering,” and “other maneuvers” were used as the response. Various environmental, crash, and road characteristics were included in the analysis. The results highlighted that the RRM model slightly outperforms the RUM model. However, it should be noted that the study only considered the standard versions of the RRM and RUM models and did not account for the heterogeneity in the dataset. As most of the implemented RRM studies are related to other aspects of transportation problems, the next few paragraphs will outline a few of those studies.
The effects of latent satisfaction and uncertainty underlying car-sharing decisions were implemented with the help of the RRM-based hybrid model. Rho-square, the fraction of log-likelihood, was used as a means of evaluating the goodness-of-fit of the model. The results highlighted a relatively high explanatory power of the hybrid model (Kim et al., 2017).
The contrast between utility maximization and regret minimization in the presence of an opt-out alternative was used (Hess et al., 2014). The results found that the differences between the model performance across most studies are minor. In the context of a choice task involving an opt-out alternative, the differences are more clear-cut. In another study, the data from a stated choice experiment for identifying valuation of nature park characteristics were used (Thiene et al., 2012). A comparison was made across the performance of the RUM- and RRM-based techniques. The results highlighted that despite having a very small difference between models’ performances, the RUM model outperforms the RRM model in terms of the statistical fit.
In another study, the standard RUM and RRM models and models accounting for heterogeneities were considered and compared (Hensher et al., 2016). It was found that while there is not much difference between the two models, the inclusion of random parameters significantly enhances the model fit. In another study, based on the collection of studies in the literature, it was highlighted that the RRM model, in general, outperforms its RUM counterpart; however, the better goodness-of-fit is only marginal (Chorus et al., 2014).
The RRM model was used for evaluating the case study of a wildlife evacuation (Wong et al., 2020). The results highlighted that the linear utility models outperform the RRM counterpart. The RRM and RUM models were employed in the presence of preference heterogeneity (Hensher et al., 2016). The results highlighted that while the differences between RUM and RRM models are minor, where incorporating the random parameters into the model would widen the model variation in terms of goodness-of-fit. The social psychology of seat-belt use was investigated in another study (Şimşekoğlu and Lajunen, 2008). The result highlighted that the anticipated regret has no significant impact on the seat-belt use.
In the context of traffic safety, study has been conducted recently will be outlined here. The hybrid random utility–random regret model in the presence of preference heterogeneity was conducted to model drivers’ actions (Rezapour and Ksaibati, 2022). It was discussed that drivers differ in their choice-making process, and thus two modeling frameworks might be needed to account for heterogeneity in choice-making behaviors. The emotional conditions of drivers, environmental conditions, and gender were some of factors contributing to various drivers’ actions. It was also found that while the majority of attributes are processed by the RUM model, significant numbers of attributes are processed by the RRM model.
Drivers constantly make choices on roads, not only just to reach the destinations but also to minimize the regret associated with taking various actions, keeping in mind that those actions might have different consequences such as crashes. Despite the importance of considering the effect of regret on drivers’ choices before crashes, very limited studies have researched that factor in the context of traffic safety when applying the RRM model, while no study in the context implemented the method while accounting for the heterogeneity in the datasets.
The objective of this study was to explore the plausibility of the RRM model and compare it with the RUM model to unlock factors contributing to the choices of drivers before crashes. It should be emphasized that the objective was not to reject or accept one method in favor of another but to expand the understanding regarding the implementation of the RRM model in the context of traffic safety choice modeling.
In addition to comparison across various modeling frameworks, the exclusion of some attributes was evaluated to see how incorporating various attributes might impact the goodness-of-fit of the RRM compared with the RUM model. It is clear from the formulation of the RRM that those attributes which do not vary the regret functions across various alternatives would deteriorate the goodness-of-fit of the model, so it is important to discuss how inclusion or exclusion of various attributes impacts the goodness-of-fit of the RRM model in relationship to the RUM model.
In addition, we extended our models to account for the heterogeneity in taste to see the relationship of the standard RUM and RRM models in relation with the extended models. The remainder of this work is organized as follows: the next section presents the RRM and RUM frameworks. We then present the dataset used in this study followed by the Results section. In the Conclusion section, we summarize our findings.
Regret would be written as the sum of all possible pairwise regret comparisons. For instance, regret across two alternatives of i and j could be written as follows:
where m and n are indices of attribute and individual, respectively, and i and j are two arbitrary alternatives.
Again, for the aforementioned equation, similar
On the other hand, for the RUM model, the utility of each alternative is maximized by only using its related attributes, i.e.,
In the model parameter’s estimation of the RRM model, instead of minimizing the regret function, we maximized the negative of the regret function, which could be written as
It is clear from Eq. 3 that
where
The log likelihood of the aforementioned model with the consideration of random heterogeneity could be written as
where
As in some of our models we changed the means of the random parameters based on some observed attributes, it is worth discussing how that would be employed. For the standard random parameter, we have
where
The model parameter estimation is based on placing the related equations of Eqs 5, 1 in Eq. 6 and solving by maximum likelihood estimation. For Eq. 1, every pairwise comparison of alternatives would be included. The model is largely dependent on the implementation of the RUM model and creating the regret functions.
It is obvious that the random parameters would be added to the regret function and not the regret components. Also, due to having all individual-specific attributes, the regret function for one of the attributes would be set to 1. In addition, the random parameters would not be added to one of the regret functions for identification. After creating the regret functions, the final values would be multiplied by -1, similar to Eq. 4, and would be estimated by the maximum likelihood algorithm by using the assigned initial values.
The dataset was related to drivers’ actions or maneuvers, which resulted in crashes from 2015–2019. The dataset is maintained and provided by the Wyoming Department of Transportation (WYDOT). The dataset aggregated over three main datasets: crash, driver, and environment. The crash dataset includes various characteristics related to crashes, such as type of crashes, injury level, and number of involved vehicles. Vehicle characteristics include attributes such as type of vehicle and age, where the dataset was aggregated based on the common column of ID. A descriptive summary of important attributes which were found to be important in the main analyses is presented in Table 1.
Those drivers’ actions that did not account for a significant number of actions were categorized under other types of drivers’ action or “others.” Driver actions of others include mainly actions such as overcorrection, steering, improper backing, unknown, and avoiding animals. It should be noted that all attributes were converted into binary except for driver conditions, where categorical characteristics of this attribute was used. For this attribute based on the judgement of the cops, it was decided whether drivers were normal, had lack of attention, or were fatigued/sleepy.
Here, few points should be highlighted regarding the choice of selected drivers’ actions. Drivers’ actions with the highest number of observations include “no improper driving (35%),” “drove too fast for conditions (11%),” “ran off road (8%),” “failed to yield row (8%),” “failed to keep proper lane (7%),” “following too close (6%),” and “unknown (5%).” However, the choice of keeping some of the variables was due to the clarity of those variables and the objectives of this study. For instance, “unknown” was not chosen due to the unclarity of variables. We also incorporated other insignificant/unimportant variables as “others” in the model. Other variables such as “erratic/reckless/careless/aggressive (3%)” were incorporated as others as they highlighted more of drivers’ attitudes and impairments more than an action.
The Results section is divided in two subsections. First, a comparison across considered models is presented. The last subsection presents the best performed model’s results.
It is clear that adjustment of the included attributes and removal or inclusion of attributes impacts the goodness-of-fit of the models. However, our objective here was to highlight the relative difference across the two types of model frameworks, RRM vs. RUM. As explained by Chorus (2012), the clear winner for the choice options results in an expected small regret and vice versa. Thus, by the adjustment of some attributes, for example, DUI and driver condition, the indifference of the drivers against the possible alternatives might be highlighted.
Based on the mathematical formulation of the RRM model, which highlights the relative performance of the alternative or pairwise comparison, it is expected that those attributes that do not change the competing alternatives result in the deteriorated RRM model fit, compared with the RUM model. In other words, the impact of some attributes on regret is expected to be minimal and as a result, inclusion of attributes would result in model fit deterioration, compared with the RUM model.
As can be seen from Table 2, we considered the adjustment/exclusion of various intuitive attributes that might impact the relative performance of the two standard models of RUM and RRM. Here, two attributes related to the mental and performance of drivers, which might impact the choice of drivers in regard to various alternatives, were considered.
As shown in Table 2, although by the removal of the driver condition alone, the RRM model did not outperform the RUM model, by exclusion of both DUI and drivers’ condition, the RRM model is superior to the RUM model. The changes in the result performance might be due to the indifferent DUI and those drivers being under some emotional conditions, which influence the drivers to be neutral for making the possible actions before crashes. Although it might be said that the impact could be seen from the fact that, for instance, some estimates of driver conditions for RRM are not different from zero, there is no consistency for this claim and the point could not be seen across the two attributes. Due to an increase in the model fits, in general, those attributes were not excluded from the final models.
Based on Table 2, it can be seen that despite the minor differences across the standard RRM and RUM models, the inclusion of the random parameters to account for the heterogeneity in the datasets resulted in a significant improvement in the model fit. For instance, while the standard RRM model slightly underperformed the RUM model, i.e., Akaike information criterion (AIC) = 185,485 vs. Bayesian information criteria (BIC) = 149,932, respectively, both mixed models outperformed the standard models. In the next step, we changed the means of the random parameters based on some observed attributes or observed heterogeneity, which resulted in minor but significant improvement in models fits, ID = 12. From Table 2, it can be seen that the final models accounting for observed heterogeneity outperformed both standard models.
As shown in Table 2, models accounting for unobserved and observed heterogeneity were found to outperform other considered models. Table 3 presents the estimation results, along with the standard error (SE) and t-ratio with the range of the significance of the final models, models’ ID = 11 and 12.
To build our regret functions, we used Eq. 2 by setting our fixed parameters to vary across the alternatives. However, we set similar coefficients across the random parameters for all alternatives. We also set the “others” alternative as constant for identification purpose for both RUM and RRM models. In addition, for identification, we did not incorporate the random parameters into one of the regret functions for the RRM model only, as it was observed that the inclusion of all random parameters for all functions for the RRM results in no change in log-likelihood estimates for those random parameters.
The interpretation of
The point estimates for the two considered models were consistent, being in similar directions, which will be discussed briefly in the next few paragraphs. Some form of distraction in cabin such as electronic device or passenger/pet in the cabin resulted in likelihood reduction of making all drivers’ actions. However, it should be noted that “other” types of drivers’ actions such as oversteering or braking were set as a reference.
Moving to vehicle type of heavy truck, which was the only vehicle type incorporated into the analysis due to its significance. The results highlighted that while heavy trucks are more likely to be involved with drivers’ actions of run off the road, they are less likely to involve in other drivers’ actions. The results were intuitive and highlighted the challenges of controlling the truck under certain situations. Also, the experience of truck drivers prevents them from involving in other types of improper driving actions. Female drivers seem to be more cautious, so they are less likely to make improper driver actions than their male counterparts, except for the driver action of following too close.
Drivers tend to drive more cautiously under less-than-optimal road conditions. The results highlighted that under driving under an icy road condition, the likelihood of making various risky driver maneuvers would reduce. On the other hand, the presence of warning signs increases the likelihood of all driver actions. That might be due to the fact that those warning signs are installed at the locations with more challenging roadway characteristics, highlighting itself with drivers possibly making various improper actions.
Also, the predictor of being under the influence of drugs or alcohol (DUI) increases the odds of all drivers’ actions. Moving to the last fixed parameters of driver condition at the time of involving in various drivers’ actions, the results highlighted that having inattention and being fatigued decrease making various drivers’ actions, including no improper action, except for improper lane change. Also, being fatigued and tired increases the likelihood of running off the road.
Five attributes were considered random with significant standard deviations. Also, we changed the means of two random parameters based on some observed attributes, Eq. 7, where the means of random parameters of the day of the week changes based on the residency of drivers and lighting condition based on the day of the week. As can be seen from Table 3, the impact of all of those observed heterogeneities was found to be significant in influencing the means of random parameters.
Finally, Figure 1 is provided to visualize the associations between various variables and different drivers’ actions. In case of insignificant variables, the arrow was excluded. Also, due to a better fit of RUM, the result of that model is provided in Figure 1. Few points are worthy of discussion from Figure 1. As can be seen from the figure, heavy trucks are more likely to make drivers’ actions of improper lane change and run off the road. Female drivers are less likely to commit all drivers’ action, except for no improper driving. Locations with the presence of warning signs are likelier to be associated with all drivers’ actions, similar to DUI. Recall that the other types of drivers’ actions, “others,” were set as a reference category.
FIGURE 1. Associations between various considered variables and different drivers’ actions and RUM model, with other types of drivers’ actions as reference.
An extensive work has been carried out in the literature to evaluate the RRM model in comparison with the RUM model for choice modeling. The majority of those studies highlighted a minor but significant difference across the two modeling alternatives. Despite the importance of studying the drivers’ actions and maneuver before crashes, to the best knowledge of the authors of this study, only a single study considered the driver maneuver before crash occurrence, by just using the standard RRM and RUM models. That is despite the plausibility of the application of the RRM model in the choice of drivers’ action. In addition, very limited studies in the context of traffic safety considered the importance of accounting for heterogeneity in the dataset or adjusted the means of random parameters by observed attributes in the context of the RRM model. Also, there is a lack of understanding related to the psychological aspects of the drivers that might impact the goodness-of-fit of the RRM model.
Thus, this study was conducted to not only make a comparison across the two competing models but also highlight the impact of considering various attributes in the modeling frameworks on the RRM goodness-of-fit. Also, one of our main goals was to show the importance of accounting for the observed and unobserved heterogeneity of the dataset in the performances of the models.
The inclusion of the RRM model and making a comparison with the RUM model unlocked few observations that could not be revealed by just using the RUM model. For instance, we found that although the RUM model outperforms the RRM model by the exclusion of driver condition and DUI attributes, the RRM model outperforms the RUM model. The possible reason for the changes were discussed in the context of this article as those drivers, being under some emotional conditions or under the influence of alcohol or drug, might have a sense of indifference in making various driver actions, which, based on the RRM formulation, is due to the low variation across pairwise comparison resulted in the deterioration of the model fit.
For instance, considering the RRM model, especially for improper lane change against all other alternatives, it was found that the emotional condition of the driver is not an important factor for shaping the regret, which is despite the fact that those attributes were found to be important for the utility of the improper lane change alternative. However, our goodness-of-fit evaluation highlighted that just exclusion of the driver condition attribute will not result in superiority of the RRM model and the exclusion of the DUI attribute, which is significant across all alternatives, is also needed. This showed the importance of incorporation of attributes into the regret function.
The impact was also highlighted, to some extent, in uncertainty associated with those parameters, especially driver conditions’ estimates across few driver actions in the RRM model. Again, from the model’s mathematical formulation, the impact is expected: while the RUM model assumes that the drivers making an action with maximizing the utility, the RRM model assumes that the drivers minimize the regret by considering various pairwise attributes before making a decision, for example consider improper lane change vs. all other driver actions. The results of the previous study also highlighted the sensitivity of the RRM model for the inclusion of the opt-out choice (Hess et al., 2014).
Based on the goodness-of-fit measures, we included all attributes and found that by considering those attributes, while drivers making actions before involvement in crashes, they mostly consider maximization of the utility and not minimization of the regret. However, it should also be noted that it was obvious from the results that considering the heterogeneity in the dataset is more important than implementation of the RRM or RUM models.
In addition, accounting for unobserved heterogeneity resulted in a significant improvement in both RRM and RUM models. Although accounting for observed heterogeneity, compared with unobserved heterogeneity resulted in a minor improvement in model fit, the improvement is significant.
More attention and investigation are needed to confirm the finding of this study. The highlighted results are specific to the dataset used in this study, and more studies are needed to compare the methodological approaches used here.
Finally, based on the concept of the RRM model, it was noted that considering what variables to include makes a huge difference in the fit of the model. Therefore, in the context of traffic safety, special attention should be made regarding the considered variables, instead of just goodness-of- fit across the RRM or RUM models. The hybrid model might be considered to account for the heterogeneity in the decision-making behaviors of drivers.
In this study, we considered various drivers’ actions as our response. Also, our predictors, directly or indirectly, were all related to that factor. For instance, driving a heavy truck or driving on an icy roadway or presence of warning signs are all factors indirectly related to the drivers’ attitudes and perceptions about the hazards.
For the future studies, a hybrid version of the RUM and RRM models should be used to gain more robustness about the implemented method. In summary, the application of the RRM model is recommended to be used along with the RUM model, by taking into account the heterogeneity of the datasets to prevent unbiased model parameter estimates. Accounting for the RRM model is, especially important to highlight those attributes that deteriorate the RRM fit by showing indifferent choice across the alternatives.
The original contributions presented in the study are included in the article/Supplementary Material. Further inquiries can be directed to the corresponding author.
MR: writing and KK: supervision.
The authors declare that the research was conducted in the absence of any commercial or financial relationships that could be construed as a potential conflict of interest.
All claims expressed in this article are solely those of the authors and do not necessarily represent those of their affiliated organizations, or those of the publisher, the editors, and the reviewers. Any product that may be evaluated in this article, or claim that may be made by its manufacturer, is not guaranteed or endorsed by the publisher.
Bell, D. E. (1982). Regret in decision making under uncertainty. Operations Res. 30, 961–981. doi:10.1287/opre.30.5.961
Chorus, C. G. (2012). Logsums for utility-maximizers and regret-minimizers, and their relation with desirability and satisfaction. Transp. Res. Part A Policy Pract. 46, 1003–1012. doi:10.1016/j.tra.2012.04.008
Chorus, C., Van Cranenburgh, S., and Dekker, T. (2014). Random regret minimization for consumer choice modeling: Assessment of empirical evidence. J. Bus. Res. 67, 2428–2436. doi:10.1016/j.jbusres.2014.02.010
Hensher, D. A., Greene, W. H., and Ho, C. Q. (2016). Random regret minimization and random utility maximization in the presence of preference heterogeneity: An empirical contrast. Reston: American Society of Civil Engineers.
Hess, S., Beck, M. J., and Chorus, C. G. (2014). Contrasts between utility maximisation and regret minimisation in the presence of opt out alternatives. Transp. Res. Part A Policy Pract. 66, 1–12. doi:10.1016/j.tra.2014.04.004
Kim, J., Rasouli, S., and Timmermans, H. (2017). Satisfaction and uncertainty in car-sharing decisions: An integration of hybrid choice and random regret-based models. Transp. Res. Part A Policy Pract. 95, 13–33. doi:10.1016/j.tra.2016.11.005
Loomes, G. (1988). Further evidence of the impact of regret and disappointment in choice under uncertainty. Economica 55, 47–62. doi:10.2307/2554246
Loomes, G., and Sugden, R. (1982). Regret theory: An alternative theory of rational choice under uncertainty. Econ. J. 92, 805–824. doi:10.2307/2232669
Newnam, S., Watson, B., and Murray, W. (2004). Factors predicting intentions to speed in a work and personal vehicle. Transp. Res. Part F Traffic Psychol. Behav. 7, 287–300. doi:10.1016/j.trf.2004.09.005
Peng, Z., Wang, Y., and Chen, Q. (2019). The generation and development of road rage incidents caused by aberrant overtaking: An analysis of cases in China. Transp. Res. part F traffic Psychol. Behav. 60, 606–619. doi:10.1016/j.trf.2018.12.002
Prato, C. G., Rasmussen, T. K., and Kaplan, S. (2014). Risk factors associated with crash severity on low-volume rural roads in Denmark. J. Transp. Saf. Secur. 6, 1–20. doi:10.1080/19439962.2013.796027
Rezapour, M., and Ksaibati, K. (2022). Hybrid random utility-random regret model in the presence of preference heterogeneity, modeling drivers’ actions. Front. Built Environ. 8. doi:10.3389/fbuil.2022.972253
Şimşekoğlu, Ö., and Lajunen, T. (2008). Social psychology of seat belt use: A comparison of theory of planned behavior and health belief model. Transp. Res. part F traffic Psychol. Behav. 11, 181–191. doi:10.1016/j.trf.2007.10.001
Thiene, M., Boeri, M., and Chorus, C. G. (2012). Random regret minimization: Exploration of a new choice model for environmental and resource economics. Environ. Resour. Econ. (Dordr). 51, 413–429. doi:10.1007/s10640-011-9505-7
Wong, S. D., Chorus, C. G., Shaheen, S. A., and Walker, J. L. (2020). A revealed preference methodology to evaluate regret minimization with challenging choice sets: A wildfire evacuation case study. Travel Behav. Soc. 20, 331–347. doi:10.1016/j.tbs.2020.04.003
Keywords: random regret minimization, driver actions, traffic safety, emotional conditions of drivers, behavior, crash
Citation: Rezapour M and Ksaibati K (2022) Random regret minimization for analyzing driver actions, accounting for preference heterogeneity. Front. Built Environ. 8:1000289. doi: 10.3389/fbuil.2022.1000289
Received: 22 July 2022; Accepted: 24 August 2022;
Published: 27 September 2022.
Edited by:
Ampol Karoonsoontawong, King Mongkut’s University of Technology Thonburi, ThailandReviewed by:
Sajjakaj Jomnonkwao, Suranaree University of Technology, ThailandCopyright © 2022 Rezapour and Ksaibati. This is an open-access article distributed under the terms of the Creative Commons Attribution License (CC BY). The use, distribution or reproduction in other forums is permitted, provided the original author(s) and the copyright owner(s) are credited and that the original publication in this journal is cited, in accordance with accepted academic practice. No use, distribution or reproduction is permitted which does not comply with these terms.
*Correspondence: Mahdi Rezapour, cmV6YXBvdXIyMDg4QHlhaG9vLmNvbQ==
Disclaimer: All claims expressed in this article are solely those of the authors and do not necessarily represent those of their affiliated organizations, or those of the publisher, the editors and the reviewers. Any product that may be evaluated in this article or claim that may be made by its manufacturer is not guaranteed or endorsed by the publisher.
Research integrity at Frontiers
Learn more about the work of our research integrity team to safeguard the quality of each article we publish.