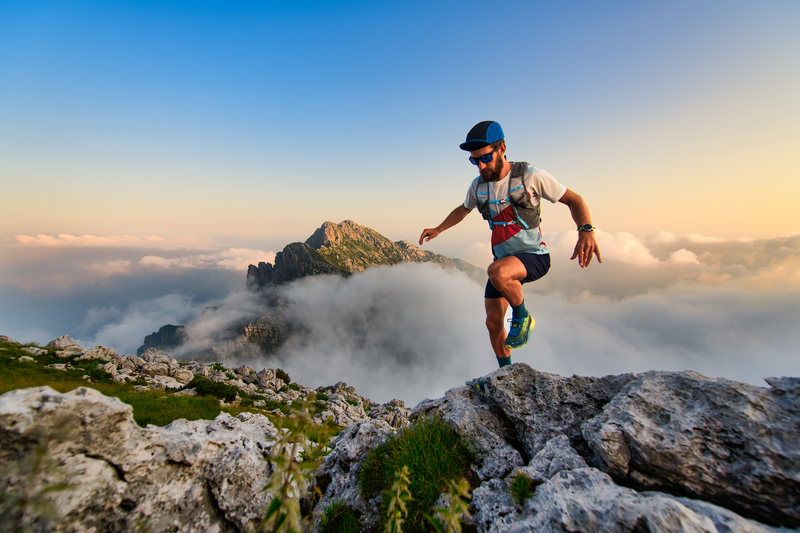
95% of researchers rate our articles as excellent or good
Learn more about the work of our research integrity team to safeguard the quality of each article we publish.
Find out more
ORIGINAL RESEARCH article
Front. Built Environ. , 26 July 2021
Sec. Indoor Environment
Volume 7 - 2021 | https://doi.org/10.3389/fbuil.2021.689388
This article is part of the Research Topic Acoustics in the Built Environment: a Challenge for Improving the Quality of Life View all 14 articles
Masking noise and reverberation strongly influence speech intelligibility and decrease listening comfort. To optimize acoustics for ensuring a comfortable environment, it is crucial to understand the respective contribution of bottom-up signal-driven cues and top-down linguistic-semantic cues to speech recognition in noise and reverberation. Since the relevance of these cues differs across speech test materials and training status of the listeners, we investigate the influence of speech material type on speech recognition in noise, reverberation and combinations of noise and reverberation. We also examine the influence of training on the performance for a subset of measurement conditions. Speech recognition is measured with an open-set, everyday Plomp-type sentence test and compared to the recognition scores for a closed-set Matrix-type test consisting of syntactically fixed and semantically unpredictable sentences (c.f. data by Rennies et al., J. Acoust. Soc. America, 2014, 136, 2642–2653). While both tests yield approximately the same recognition threshold in noise in trained normal-hearing listeners, their performance may differ as a result of cognitive factors, i.e., the closed-set test is more sensitive to training effects while the open-set test is more affected by language familiarity. All experimental data were obtained at a fixed signal-to-noise ratio (SNR) and/or reverberation time set to obtain the desired speech transmission index (STI) values of 0.17, 0.30, and 0.43. respectively, thus linking the data to STI predictions as a measure of pure low-level acoustic effects. The results confirm the consistent difference between robustness to reverberation observed in the literature between the matrix type sentences and the Plomp-type sentences, especially for poor and medium speech intelligibility. The robustness of the closed-set matrix type sentences against reverberation disappeared when listeners had no a priori knowledge about the speech material (sentence structure and words used), thus demonstrating the influence of higher-level lexical-semantic cues in speech recognition. In addition, the consistent difference between reverberation- and noise-induced recognition scores of everyday sentences for medium and high STI conditions and the differences between Matrix-type and Plomp-type sentence scores clearly demonstrate the limited utility of the STI in predicting speech recognition in noise and reverberation.
In realistic room scenarios speech intelligibility is mainly determined by background noise and reverberation. This has been confirmed by various studies that investigated the detrimental effects of background noise and reverberation in listeners with different hearing status. To model the combined effect of these factors for arbitrary situations, objective measures have been developed based on the concept of the Speech Transmission Index (Steeneken and Houtgast, 1980). Several studies confirmed a strong relationship between STI predictions and empirical speech recognition data as well as the detrimental influence of noise, reverberation, and hearing status of the listeners (e.g., Duquesnoy and Plomp, 1980; George et al., 2010). Rennies et al. (2014) employed a similar paradigm as George at al. (2010) by measuring speech recognition scores in normal-hearing listeners for different combinations of noise and reverberation that produced the same STI values. In contrast to the data of George et al. (2010) for their group of normal-hearing listeners, Rennies et al. (2014) reported significant discrepancies between the measured data and STI predictions. While the STI correctly accounted for the influence of noise on speech recognition, but the influence of reverberation was overestimated compared to the empirical data, i.e., the predicted detrimental effect of reverberation was larger than in the measured data. Rennies et al. (2014) speculated that the differences between these two studies may be caused by the fact that speech material differed in talker (male German speaker used by Rennies et al., 2014, vs. female Dutch talker used in the study of George et al., 2010). Furthermore, different types of speech material were used which could be crucial for the different outcomes. However, it remains unclear which of these differences is mainly responsible for the observed discrepancy.1
In the current study we therefore investigate if the observed discrepancies between the studies of George et al. (2010) and Rennies et al. (2014) are due to the type of speech material used in the experiments: Rennies et al. (2014) used a closed-set matrix-type sentence test consisting of semantically unpredictably and syntactically fixed sentences which are generated from a base matrix consisting of 50 words (10 names, 10 verbs, 10 numerals, 10 adjectives, and 10 nouns, Wagener et al., 1999). Before the actual measurements, listeners are always trained with at least two test lists of 20 sentences to get familiar with the speech material and account for training effect (Wagener et al., 1999; Kollmeier et al., 2015). In contrast, George et al. (2010) used open-set everyday sentence test VU98 (Versfeld et al., 2000) consisting of sentences with different syntax and vocabulary. Hence, the listeners were not aware of the sentence content and were not trained with the same material that was used for testing.
It is investigated here if a priori knowledge about the speech material (i.e., training to the speech material resulting in the familiarity with the limited set of 50 words and information about the fixed grammatical sentence structure) is the reason for the observed robustness of matrix tests in reverberant conditions. Furthermore, the effects of noise only, reverberation only, and combinations of noise and reverberation are systematically investigated using the method adapted from Rennies et al. (2014), i.e., speech recognition measurements at a fixed signal-to-noise ratio (SNR) and/or reverberation time set to obtain the desired STI values of 0.17, 0.30, and 0.43 but with a different type of speech material, namely the German everyday sentences test (so-called Kollmeier and Wesselkamp, 1997). This type of speech material is comparable to the Plomp-type speech material used by George et al. (2010). The GÖSA was recorded with the same speaker as the German matrix sentence test used by Rennies et al. (2014) which allows for excluding the potentially large effects of speaker on speech recognition (Hochmuth et al., 2015). This way a direct comparisons and examination of the effect of speech material type was possible without confounding effects of talker differences.
If the type of speech material is indeed the main factor responsible for different outcomes in the studies reported by George et al. (2010) and Rennies et al. (2014), the speech recognition scores measured here with the German everyday sentence test should agree with the findings of George et al. (2010) and the measured scores should be constant along iso-STI contours, i.e., for different combinations of SNR and reverberation time that produce the same STI.
Furthermore, we investigated the reasons for the high robustness of the German matrix sentence test against reverberation observed by Rennies et al. (2014). We hypothesize that the high recognition scores in strongly reverberant conditions as measured by Rennies et al. (2014) arise from the a priori knowledge about the speech material which is given in the training session. In order to test this hypothesis, two additional conditions with the matrix test in reverberation were included. The main difference to the study of Rennies et al. (2014) was that the listeners were not familiarized with the speech material prior actual measurements and by that they were not aware of the fixed grammatical structure of the sentences and the limited number of words (which effectively made the matrix test similar to an open-set speech test).
By comparing the experimental results with GÖSA and the untrained matrix test to the trained matrix test data collected by Rennies et al. (2014) and George et al. (2010), we can assess the influence of top-down processing (i.e., knowledge-driven cognitive processes utilizing lexical-semantic cues) for speech recognition in reverberant environments. Furthermore, the comparison of the measured data to predictions of the STI will provide an estimate of the signal-driven, low-level, bottom-up processing contribution in the conditions considered. Hence, an estimate of the role of cognitive processes (with a focus on knowledge-driven cognitive processes utilizing lexical-semantic cues) in compensating for the detrimental effect of reverberation and background noise will become possible.
The STI is based on the concept of the modulation transfer function (MTF), which describes the changes in the temporal modulation of the signal due to its transmission through a system. A simplified STI calculation method was adopted here from the study of Rennies et al. (2014). This method considers the calculation of the MTF as a product of the factor mrev, which quantifies the convolutive distortion of the speech signal due to reverberation, and the factor mnoise, which characterizes the distortions of the speech signal due to the additive noise (IEC, 2003). If the room impulse response is approximated by an exponential decay, the factor mrev can be then described as:
where F is the modulation frequency in Hertz and T60 is the reverberation time in seconds (IEC, 2003).
For compatibility with the studies of George et al. (2010) and Rennies et al. (2014), the reverberation time was assumed to be frequency independent. Accordingly, all the room impulse responses (RIRs) used in this study were generated based on their broadband reverberation time. The factor mnoise is expressed as
where SNR is signal-to-noise ratio in dB (IEC, 2003).
Since the long-term spectra of the speech material and the masking noise were similar, it was assumed that the SNR also is constant across frequencies.
Fourteen normal-hearing listeners with a pure-tone threshold not exceeding 20 dB HL for octave frequencies between 125 Hz and 8 kHz participated in this study. They ranged in age from 21 to 27 years (mean age of 22.3 ± 2.2). None of them had previous experience with speech recognition measurements. All listeners were informed about the general purpose of the study, gave written informed consent, and were paid for their participation in the listening experiments. Ethical approval was obtained from the Research Ethical Committee of the Universität Oldenburg.
The Göttingen sentence test (Kollmeier and Wesselkamp, 1997) was used as a speech material in this study. It contains short, meaningful everyday sentences like the Plomp-type sentences (Plomp and Mimpen, 1979) and the HINT test (Nilsson et al., 1994), but employing word scoring and a numerical optimization procedure for homogenization across test items. The word corpus is rather large (1,194 words), the content of each sentence is unknown to the listener. Ten perceptually balanced lists of 20 sentences each are available. The lists were optimized for perceptual equivalence between lists, i.e., speech recognition scores do not depend on the test list used in the measurements.
In addition, two test lists of 20 sentences each from the German matrix sentence test (in Germany known as Oldenburg sentence test) were used to assess the influence of “a priori knowledge” about the speech material on speech recognition in reverberation. Speech recognition was measured without informing the listener about the structure of the test and with no training with the speech material. These sentences have a fixed grammatical structure and limited speech material of 50 words. Each word occurs in the test list exactly twice.
For the GÖSA, four different measurement conditions were included. In condition 1, only the influence of masking noise was considered. The noise had been generated by multiple, randomly time-shifted superpositions of sentences from the target talker and, hence, the long-term spectrum of the target material and the noise were very similar. Speech and noise were mixed at SNRs of −10, −6, and −2 dB to obtain the desired STI values of 0.17, 0.30, and 0.43, respectively. They were adapted from the study of Rennies et al. (2014) and corresponded to low, medium and high speech recognition scores. Conditions 2 and 3 included the combined influence of noise and reverberation. The signals were mixed at an SNR of 0 dB (condition 2) and 7 dB (condition 3), and the reverberation time was adapted to obtain the desired STI values (0.17 and 0.30, i.e., the same two lowest values as employed in condition 1). In condition 4, only reverberation was used as a detrimental factor. The reverberation times were chosen such that the same STI values were obtained as in the condition 1. The experimental settings are summarized in Table 1.
TABLE 1. Summary of the measurement settings including different combinations of signal-noise-ratio (SNR) and reverberation time (T60).
For the measurements with the German matrix test, two conditions with a reverberation time of 9.38 and 4.06 s were used resulting in the two lowest STI values used in this study (0.17 and 0.30, respectively). These conditions reflect the situations in which the robustness of the German matrix test was most prominent (Rennies et al., 2014). Pilot studies resulted in speech recognition scores for the reverberation time of 9.38 close to zero so that it can be assumed that presentation of one test list in this condition does not give sufficient possibility to get trained to the speech material. In other words, the second measurement with a reverberation time of 4.06 s can be considered as untrained measurement with no a priori knowledge about the sentence structure and linguistic content.
For all conditions containing reverberation, speech and noise (if applicable) were convolved with the desired RIR. To generate the RIR, white noise was multiplied with an exponential decay corresponding to the desired reverberation time. The length of the RIR was equal to the reverberation time. The same method of RIR generation was used by George et al. (2010) and Rennies et al. (2014) which makes it possible to define and vary the T60 in a systematic way.
The signals were calibrated to dB SPL using Brüel&Kjӕr instruments (artificial ear type 4153, microphone 4134, preamplifier 2669, and amplifier 2610). In the measurements, the speech level was fixed at 55 dB SPL and the level of the noise was varied to obtain the desired SNR. The masking noise was turned on 500 ms before and turned off 500 ms after sentence presentation. All signals were digitally preprocessed in MATLAB and the measurements were administered using the Oldenburg Measurement Application software (HörTech GmbH, Germany). The signals were run through an RME DIGI 96/8 PAD 24bit sound device and converted to analog signals (RME 4 ADI-8 Pro). The analog signals were then amplified by a TDT HB7 headphone amplifier and presented diotically through Sennheiser HD650 headphones in a sound attenuating booth (fulfilling the requirements of ANSI/ASA S3.1-1999, R2008).
A constant stimulus-level method was used in all measurements. For each measurement condition, one test list of 20 sentences was used. The order of the measurement conditions with GÖSA was fully randomized. The two tests conducted with the German matrix test were presented between the 3rd and the 7th measurement with GÖSA, the exact order was randomized, but the most difficult condition with a reverberation time of 9.38 s was always presented before the condition with reverberation time of 4.03 s. This was done to exclude the possibility of training to the speech material. Overall, 12 different conditions were tested (10 with GÖSA, 2 with the German matrix test). The listeners’ task was to repeat the understood words. The experimenter marked the correct responses. Word scoring was used meaning that each word in a sentence was judged separately as correct or incorrect. The percentage of correct responses was used as a measure of speech recognition.
Speech recognition data were transformed using the rationalized arcsine transform (Studebaker, 1985) since recognition scores for the lowest and highest STI were close to 0 and 100%, respectively. The statistical tests were done on the transformed data. Non-parametric Friedman rank tests and Wilcoxon tests for pairwise comparisons were used since, in some of the conditions, the data were not normally distributed as indicated by Kolmogorov-Smirnov tests. If appropriate, the Wilcoxon test was used for post-hoc analysis to further explore the sources of significance—in this case the significance level of 0.05 was adjusted using Bonferroni corrections.
The median rationalized arcsine unit (RAU) scores with corresponding interquartile ranges for all conditions (listed in Table 1) measured with everyday sentences are shown in Figure 1. As expected, speech recognition scores were lowest for the STI of 0.17 and highest for the STI of 0.43. No statistically significant differences were found across the measurement conditions for the lowest STI [χ2(3) = 3.51, p = 0.32]. The median scores for the lowest STI averaged across all four conditions was 13.6% with an interquartile range of 9.1%. For the highest STI, the median score and corresponding interquartile range were 87.3 and 8.1% in condition 1, and 69.4 and 11.2% in condition 4, respectively. These differences were statistically significant [Z = −3.3, p = 0.001]. Statistically significant differences were found also across measurement conditions for the medium STI value [χ2(3) = 28.11, p < 0.001], where median scores were 48.1, 31.1, 36.7, and 30.1% in conditions 1 to 4, respectively, and interquartile ranges varied from 7% (condition 1) to 14.7% (condition 2). Pairwise comparisons (with a significance level of 0.008) showed statistically significant differences in recognition score between condition 1 (noise only at an SNR of -6 dB) and condition 4 (reverberation only with T60 = 4.06 s; p = 0.001), condition 1 and condition 2 (SNR = 0 dB and T60 = 1.63 s, p = 0.001), condition 2 and condition 3 (SNR = 7 dB and T60 = 3.25 s, p = 0.004), and condition 3 and 4 (p = 0.002). Note that a consistent difference in speech scores across respective STI indicates that the measured effect of reverberation differs from the effect of noise on speech recognition even though no difference is predicted by the respective STI.
FIGURE 1. Median RAU speech recognition scores for the GÖSA with corresponding interquartile ranges for different STIs and the measurement conditions listed in Table 1. Stars indicate statistically significant differences across measurements conditions.
Median scores with corresponding interquartile ranges for measurements in reverberation (no noise) with GÖSA and untrained matrix sentences (present study) as well as trained matrix sentences (data from Rennies et al., 2014, transformed in RAU) are shown in Figure 2. Statistically significant differences across measurement conditions were found for each STI with p < 0.001. For the lowest and medium STI values, pairwise comparisons (with a significance level of 0.016) showed statistically higher scores for the trained matrix sentences than for the untrained matrix sentences as well as for the GÖSA (all comparisons with p = 0.001). No differences between GÖSA and untrained matrix sentences were found for both STIs (with p = 0.041 for both comparisons). Higher scores for trained matrix sentences than for the GÖSA were also confirmed for the highest STI (p < 0.001). The median scores of trained matrix sentences were 45.2 percentage points higher than for the untrained matrix sentences at an STI of 0.17, and 47.7 percentage points higher at an STI of 0.30. This indicates a strong effect of a priori knowledge (top-down processes) on speech recognition in reverberant conditions. The results of speech recognition in reverberation with untrained matrix sentences (no a priori knowledge about the speech material) are comparable with the outcomes of everyday sentence test (GÖSA).
FIGURE 2. Median RAU recognition scores and corresponding interquartile ranges for everyday sentence test (GÖSA), trained German matrix test (data from Rennies et al., 2014), and untrained German matrix test including only reverberation as detrimental factor. Stars indicate statistically significant differences across measurements conditions.
Comparisons of the median recognition scores between trained matrix sentences and GÖSA for measurements in noise at different SNRs (no reverberation) are shown in Figure 3. The difference across the tests were significant for each STI. The median magnitude of this difference was 29.0% at an SNR of −10 dB (corresponding to an STI of 0.17, p < 0.001), 21.4% at an SNR of −6 dB (corresponding to an STI of 0.30, p < 0.001), and 5.1% at an SNR of −2 dB (corresponding to an STI of 0.43, p = 0.001). The relatively small difference at the highest STI probably resulted from ceiling effect observed at this SNR for both tests. The differences in speech recognition scores across the tests in noisy conditions were not expected since it is known from the literature that both tests have comparable reference speech recognition threshold in stationary noise (Kollmeier and Wesselkamp, 1997; Wagener et al., 1999; Brand et al., 2004; Warzybok et al., 2015). Possible reasons for this discrepancy will be elaborated in the discussion section.
FIGURE 3. Median RAU recognition scores and corresponding interquartile ranges for everyday sentence test (GÖSA) and trained German matrix test (data from Rennies et al., 2014) measured in stationary noise at different signal-to-noise ratios. Stars indicate statistically significant differences across measurements conditions.
The main aim of this research was to assess the role of different types of speech material on speech recognition in noise, in reverberation, and in combinations of noise and reverberation. The two speech material types used here, recorded with the same male talker, indicated significant differences in speech recognition even though the intelligibility should have been equal based on the STI predictions. The largest differences were observed in the conditions with reverberation as the only detrimental factor. In all reverberant conditions, the speech material of the German matrix test showed strong robustness (after training), i.e., the recognition scores were significantly higher than for the GÖSA. Moreover, comparing the outcomes of the measurements with the trained matrix test in noise and in reverberation, Rennies et al. (2014) found significantly higher speech recognition scores in reverberation (using the same white-noise RIRs as here) than in noise at STI values of 0.17 and 0.3, which is in disagreement with the STI predictions.
The outcomes of the present study indicate that the a priori knowledge and training to the speech material has a substantial contribution to the robustness of the matrix test against reverberation. The knowledge of the sentence structure and familiarity with the limited speech material consisting of 50 words obtained within the training session resulted in much higher speech recognition scores than expected based on the STI predictions. However, the robustness of this type of speech material was not observed when the listeners were not trained prior the actual measurements. In the measurements with untrained matrix sentences, the recognition scores did not differ from the scores of the everyday sentence test. This shows the importance and contribution of the high-level top-down processes to speech recognition, which cannot be predicted by the STI since its calculations are based on the acoustic cues of the signals without consideration of top-down processes. Since each test list of the GÖSA contains unique sentences, i.e., the vocabulary differs across the lists, no training effect that would be comparable with that of the matrix-type sentences is expected for the everyday sentences. However, a strong contribution of higher-level top-down processes will be observed when the same test list is used for the second time in a short period. This is due to the context in the everyday sentences which makes these sentences easy to memorize and, in addition, enables the listener (to some degree) to guess the complete sentence from recognizing a single word. In comparison to first-time use of a meaningful sentence test, this would result in incorrectly high recognition scores. This is not the case for the matrix-type sentences, since due to their semantically unpredictable content, the sentences are difficult to memorize and there is no benefit available from sentence context.
Previous studies reported comparable speech recognition thresholds (SRTs), i.e., the SNRs corresponding to 50% speech recognition for GÖSA and the German matrix test (Kollmeier and Wesselkamp, 1997; Kollmeier et al., 2015). Hence, it was expected that the results of both tests would result in similar speech recognition scores in noise. However, the results showed higher scores for the matrix sentences than for the GÖSA. Rennies et al. (2014) reported that the good results observed in their study could be due to two extensively trained listeners participating in their experiments. To assess the impact of these listeners, we re-evaluated these data by excluding the two best listeners (corresponding to the two experienced listeners). However, the median speech recognition scores in different conditions only changed marginally (from 1 to maximally 5%) so that other reasons seem to be responsible for the good performance of the listeners in Rennies et al. (2014). Warzybok et al. (2015) and Brand et al. (2004) measured SRTs with a naïve group of normal-hearing listeners with the German matrix test and reported mean values of −6.7 and −6.8 dB, respectively. In the study of Rennies et al. (2014), the median speech recognition scores were 48% at an SNR of −10 dB and 84% at an SNR of −6 dB. This is considerably higher than the results obtained with naïve listeners by Brand et al. (2004) and Warzybok et al. (2015), supporting the assumption that listeners in the study of Rennies et al. (2014) were better than could be expected from a naïve listener panel. In contrast, Kollmeier and Wesselkamp (1997) reported a reference SRT of −6.2 dB for the GÖSA, which is in close agreement to the present data (median score of 48% at an SNR of −6 dB).
The measured data with the GÖSA can be also compared to the data from George et al. (2010) who adaptively measured SRTs with everyday sentences (VU98 corpus, Versfeld et al., 2000) in noise, in reverberation, and in combinations of noise and reverberation. George et al. (2010) assessed their listeners using sentence scoring. Because sentence scoring produces lower recognition rates than word scoring, the present data were re-calculated using sentence scoring in order to be directly comparable. Sentence-scored speech intelligibility was achieved by scoring a sentence as correct only if all the words of a sentence were repeated correctly. If the listener misunderstood one or more words, the answer was scored as incorrect. Then the number of correctly understood sentences was divided by the number of sentences presented to the listener (for GÖSA N = 20) and % correct responses were obtained. The re-calculation was possible since all the listener answers were digitally stored. Re-calculation was done for all conditions corresponding to STI values of 0.30 and 0.43 (the lowest STI was excluded from the comparisons since it resulted already in very low recognition scores for word scoring). For an STI of 0.30, sentence-scored medium recognition scores decreased similarly across measurement conditions and were on average 19.4% lower than scores obtained with word scoring. The median scores were 22.5% in condition 1 (SNR = −6 dB), 2.5% in condition 2 (SNR = 0 dB, T60 = 1.63 s) and 4 (T60 = 4.06 s), and 10.0% in condition 3 (SNR = 7 dB, T60 = 7.71 s). For an STI of 0.43, the median scores with sentence scoring were 90% in condition 1 (SNR = -6 dB) and 62.5% in condition 2 (T60 = 2.03 s). George et al. (2010) reported 50% speech recognition at an SNR of −3.9 dB when only noise was considered as a detrimental factor. For measurements in reverberation, 50% speech recognition was measured for T60 of 2.03 s. Considering the results of the present study, the 50% threshold in noise using sentence scoring can be estimated to be at about −4.4 dB (by interpolation) which is in line with the threshold measured by George et al. (2010) for the Dutch everyday sentence test. Sentence recognition scores in reverberation only at T60 of 2.02 s were 12.5% higher for GÖSA than the VU98 corpus, however, the significance of this difference remains unclear.
George at al. (2010) found a good correlation between the speech recognition data and STI predictions. They showed that the STI can account for the influence of noise, reverberation, and combination of both. In the study reported here, the recognition scores in reverberation only (condition 4) were significantly lower than in noise (condition 1) although the calculated STI was the same for both conditions. Hence, the detrimental influence of reverberation on speech recognition (using the same type of white-noise RIRs as used by George et al., 2010) was found to be greater than it was predicted by the STI. This effect occurred for both, sentence and word scoring methods, so that the scoring method does not seem to be the underlying reason for the observed discrepancies.
Apart from the scoring method, these two studies differ also in other aspects including the talker (female Dutch talker vs. male German talker) or speaking style (more informal for the Dutch speech material). However, the influence and interaction of these factors on the observed differences and mainly on the relative susceptibility to reverberation is unclear and could be a subject of future studies. Furthermore, the STI calculation differs slightly between the two studies. George et al. (2010) used a modified STI version (Houtgast et al., 1980) including 18 modulation frequency bands instead of the classic 14 (used in the present study). They argued that the classical STI underestimates the adverse effect of reverberation on speech intelligibility when informal, conversational speech is concerned. Systematic investigation and comparisons of STI predictions with different number of modulation frequency bands and for different types of speech material could be investigated in future studies.
In summary, it was shown that the difference between robustness to reverberation observed in the study of Rennies et al. (2014) and of George et al. (2010) may be attributed to the speech material type (closed-set matrix type sentences with high familiarity/training effect vs. unfamiliar, short meaningful Plomp-type sentences) because our listeners basically exhibited the same difference for comparable speech materials. The impact of the speech material type seems to be stronger at low and medium STI values, corresponding to poor and medium speech intelligibility than for high STI resulting in very good speech intelligibility and being limited by a ceiling effect. The robustness of the closed-set matrix type sentences against reverberation disappeared when listeners had no a priori knowledge about the speech material (sentence structure and words used).
This provides some evidence about the relative importance of high-level, top-down processing strategies in difficult reverberation situations. It remains unclear if the same applies for situations with interfering noise without reverberation. Further studies are needed with a direct comparison within the same subjects to assess the importance of bottom-up and top-down processing across different acoustic conditions.
Nevertheless, the consistent difference between reverberation- and noise-induced recognition scores of everyday sentences for medium and high STI conditions and the differences between Matrix-type and Plomp-type sentence scores clearly demonstrate the limited utility of the STI for predicting speech recognition in conditions with varying susceptibility to noise and/or reverberation.
The datasets generated during and/or analysed during the current study are available from the corresponding author on reasonable request.
The studies involving human participants were reviewed and approved by the Research Ethical Committee of the Universität Oldenburg. The patients/participants provided their written informed consent to participate in this study.
AW contributed to the study design, data collection, statistical analyses, and paper draft. JR was involved in study design, data analysis and manuscript preparation. BK contributed to the study design and the manuscript.
The project was supported by the Deutsche Forschungsgemeinschaft (DFG, German Research Foundation)—Projektnummer 352015383—SFB 1330 A1 and A5 and research project “Model-based multilingual rehabilitative audiology” (No. 32543918).
The authors declare that the research was conducted in the absence of any commercial or financial relationships that could be construed as a potential conflict of interest.
All claims expressed in this article are solely those of the authors and do not necessarily represent those of their affiliated organizations, or those of the publisher, the editors and the reviewers. Any product that may be evaluated in this article, or claim that may be made by its manufacturer, is not guaranteed or endorsed by the publisher.
The authors thank Henning Schepker for the valuable comments and contribution to the experimental design.
1While both the study of Rennies et al. (2014) and of George et al. (2010) employed normal-hearing listeners, the age range differed slightly (20–41 years vs. 26–57 years), i.e., the listeners group of George at al. (2010) included some older normal-hearing listeners. This may have contributed to differences between the studies. However, the mean speech recognition threshold and corresponding standard deviation observed by George et al. (2010) was comparable to the reference data of young normal-hearing listeners for the speech material used (VU98 corpus, Versfeld et al., 2000). Hence it can be assumed that differences in listener groups are not the main contributor to the systematic differences discussed by Rennies et al. (2014).
ANSI/ASA S3.1-1999 (R2008). Maximum permissible ambient noise levels for audiometric test rooms. Washington, USA: American National Standards Institute.
Brand, T., Wittkop, T., Wagener, K., and Kollmeier, B. (2004). Vergleich von Oldenburger Satztest und Freiburger Wörtertest als geschlossenen Versionen (Comparison of Oldenburg Sentence test and Freiburger word test in the closed-set response format). Leipzig, Germany: Proceedings of 7th Congress of the German Society of Audiology.
Duquesnoy, A. J., and Plomp, R. (1980). Effect of reverberation and noise on the intelligibility of sentences in cases of presbyacusis. The J. Acoust. Soc. America 68, 537–544. doi:10.1121/1.384767
George, E. L. J., Goverts, S. T., Festen, J. M., and Houtgast, T. (2010). Measuring the effects of reverberation and noise on sentence intelligibility for hearing-impaired listeners. J. Speech Lang. Hear. Res. 53, 1429–1439. doi:10.1044/1092-4388(2010/09-0197)
Hochmuth, S., Jürgens, T., Brand, T., and Kollmeier, B. (2015). Talker- and language-specific effects on speech intelligibility in noise assessed with bilingual talkers: Which language is more robust against noise and reverberation? Int. J. Audiol. 54, 23–34. doi:10.3109/14992027.2015.1088174
Houtgast, T., Steeneken, H. J. M., and Plomp, R. (1980). Predicting speech intelligibility in rooms from the modulation transfer function: I. General room acoustics. Acustica 46, 59–72.
IEC (2003). “Sound System Equipment - Part 16: Objective rating of speech intelligibility by speech transmission index,” in International Standard IEC 60268-16 (Geneva, Switzerland: International Electrotechnical Commission)).
Kollmeier, B., Warzybok, A., Hochmuth, S., Zokoll, M., Uslar, V. N., Brand, T., et al. (2015). The multilingual matrix test: principles, applications and comparison across languages – a review. Int. J. Audiol. 54 (Suppl. 2), 3–16. doi:10.3109/14992027.2015.1020971
Kollmeier, B., and Wesselkamp, M. (1997). Development and evaluation of a German sentence test for objective and subjective speech intelligibility assessment. J. Acoust. Soc. America 102, 2412–2421. doi:10.1121/1.419624
Nilsson, M., Soli, S. D., and Sullivan, J. A. (1994). Development of the Hearing in Noise Test for the measurement of speech reception thresholds in quiet and in noise. J. Acoust. Soc. America 95, 1085–1099. doi:10.1121/1.408469
Plomp, R., and Mimpen, A. M. (1979). Speech‐reception threshold for sentences as a function of age and noise level. J. Acoust. Soc. America 66, 1333–1342. doi:10.1121/1.383554
Rennies, J., Schepker, H., Holube, I., and Kollmeier, B. (2014). Listening effort and speech intelligibility in listening situations affected by noise and reverberation. J. Acoust. Soc. America 136 (5), 2642–2653. doi:10.1121/1.4897398
Steeneken, H. J. M., and Houtgast, T. (1980). A physical method for measuring speech‐transmission quality. J. Acoust. Soc. America 67, 318–326. doi:10.1121/1.384464
Studebaker, G. A. (1985). A "Rationalized" Arcsine Transform. J. Speech Lang. Hear. Res. 28, 455–462. doi:10.1044/jshr.2803.455
Versfeld, N. J., Daalder, L., Festen, J. M., and Houtgast, T. (2000). Method for the selection of sentence materials for efficient measurement of the speech reception threshold. J. Acoust. Soc. America 107, 1671–1684. doi:10.1121/1.428451
Wagener, K., Brand, T., and Kollmeier, B. (1999). Entwicklung und Evaluation eines Satztests für die deutsche Sprache Teil III: Evaluation des Oldenburger Satztests (Development and evaluation of a German speech intelligibility test. Part III: Evaluation of the Oldenburg sentence test). Z. Audiol. 38, 86–95.
Keywords: speech transmission index, speech in noise, reverberation, speech perception, matrix sentence test, everyday sentence test
Citation: Warzybok A, Rennies J and Kollmeier B (2021) Contribution of Low-Level Acoustic and Higher-Level Lexical-Semantic Cues to Speech Recognition in Noise and Reverberation. Front. Built Environ. 7:689388. doi: 10.3389/fbuil.2021.689388
Received: 31 March 2021; Accepted: 21 June 2021;
Published: 26 July 2021.
Edited by:
Arianna Astolfi, Politecnico di Torino, ItalyReviewed by:
Rosario Romano, University of Naples Federico II, ItalyCopyright © 2021 Warzybok, Rennies and Kollmeier. This is an open-access article distributed under the terms of the Creative Commons Attribution License (CC BY). The use, distribution or reproduction in other forums is permitted, provided the original author(s) and the copyright owner(s) are credited and that the original publication in this journal is cited, in accordance with accepted academic practice. No use, distribution or reproduction is permitted which does not comply with these terms.
*Correspondence: Anna Warzybok, YS53YXJ6eWJva0B1b2wuZGU=
Disclaimer: All claims expressed in this article are solely those of the authors and do not necessarily represent those of their affiliated organizations, or those of the publisher, the editors and the reviewers. Any product that may be evaluated in this article or claim that may be made by its manufacturer is not guaranteed or endorsed by the publisher.
Research integrity at Frontiers
Learn more about the work of our research integrity team to safeguard the quality of each article we publish.