- 1Department of Civil Engineering, School of Engineering, University of Birmingham, Birmingham, United Kingdom
- 2School of Metallurgy and Materials, University of Birmingham, Birmingham, United Kingdom
- 3Polytechnic School, University of São Paulo, São Paulo, Brazil
When planning a new track or improving a track that is currently in-service, it is important to predict the probable rate of track deterioration as a function of the variables related to the train and its periodicity. This literature review reveals that there are currently no track deterioration methods available analyze the condition of a railway track. A prediction of deterioration requires accurate quantification of each track component and track geometry behavior and a better knowledge of interactions between components and geometry. This extended survey found more than 100 methods (or studies) related to track deterioration, including other literature reviews, but very few of these methods work when several track deterioration issues occur. This paper aims to build upon these, adopting a methodology for a systematic critical literature review that identifies, evaluates, and classifies these primary studies to predict the track deterioration according to criteria of assessment. Finally, it establishes the gaps and the challenges that will need to be overcome in future research.
Introduction
The effects of adverse traits on the railway track, which is known also as the permanent way, are cumulative. Railway materials such as the rail, sleepers, fastenings, and ballasts require routine attention and renewal at frequent intervals (Hay, 1982). If the permanent way is not impeccably aligned and leveled, roughness contributes to vibration (Yan et al., 2019) and oscillations of the train, which can cause discomfort to the passenger, damage to the freight (Tzanakakis, 2013), and in worst case scenarios, catastrophic accidents. The condition of the permanent way has an important function in the behavior of a railway system (i.e., maintenance, operational safety, and passenger comfort) and it is important to consider any irregularities and ascertain the phenomena that cause track deterioration, and to forecast irregularities (Gong et al., 2016).
Track conditions must be assessed by measurable degradation parameters (El-Sibaie and Zhang, 2004). These include the condition of railway components and geometry, which have a close relationship within the process of track degradation. According to Guler et al. (2011), if a component is in poor condition this will contribute to the deterioration of the component, and it will not be able to fit the desired track geometry. Each parameter must be weighed for its magnitude in impacting the permanent way activity (El-Sibaie and Zhang, 2004).
This literature investigation has shown that there are no ready methods of evaluating the deterioration and condition of the railway track. A prediction of track condition requires accurate quantification of each track component and each track geometry and a better knowledge of interactions between components and geometry. During this extended survey, more than 100 methods and studies related to track deterioration were found. Very few of these methods of inquiry covered more than one track deterioration issue.
This systematic critical review aims to identify, evaluate, and classify primary studies of track prediction into groups according to the complexity of both the method and the validation, whilst also taking into account the quality of the study. The application of tactics in evaluating the track deterioration and its elements (track components and track geometry parameters) are also evaluated. Consequently, it compares these track deterioration studies with a hypothetical study (the best one) and identifies gaps in research.
This paper is structured into five sections. Section “Background” presents a background of the permanent way and its environment. Following this, Section “Methodology” presents the methodology used to develop this systematic literature review. Section “Results and Discussions” then discussing the main findings, sharing these methods and assessing their complexities and qualities in order to group them. Lastly, Section “Conclusion” concludes the paper and outlines gaps in this research.
Background
The function of a railway track is to support the load of the railway vehicles (Hay, 1982) and to guide their movements (Iwnicki, 2006), enabling the railway vehicles to move without risk of derailment (Lichtberger, 2005). To investigate the specific load effect on the track, it is necessary to create a map of the different parts of the system.
A modern conventional track can be subdivided into seven components (rails, rail pads, sleepers, ballast, sub-ballast, geosynthetics, and subgrade), each having a specific function in trainload support (Le Pen, 2008). In turn, this same permanent way has a position in the space, called track geometry, which is the spatial position of the rail track. According to Faiz and Singh (2009), the “X-axis” of the track represents the distance along the direction of travel, “Y” is the axis parallel to the running top rail (surface), and “Z” defines the axis perpendicular to the running permanent way. Each rail has 2 degrees of freedom, and these 4 degrees of freedom are normally replaced by an equivalent system consisting of cant, level, alignment, gauge, and twist (Esveld, 2001), which represent track geometry. Descriptions and the function of these track components, including thresholds and geometry, are explained in detail by Hay (1982), Selig and Waters (1994), Esveld (2001), Lichtberger (2005), Profillidis (2006), Tzanakakis (2013), and Li et al. (2016).
A railway wheel causes vertical and horizontal forces on the permanent way. Additionally, the long-welded permanent way is subject to the influence of longitudinal forces arising because of changes in temperature (Lichtberger, 2005). The permanent way is stressed by quasi-static (low-frequency) and dynamic force components of higher frequency (Lichtberger, 2005). According to Iwnicki (2006), the principal difference between a railway vehicle and other types of wheeled vehicle is the guidance provided by the permanent way. The combination of vehicle and track should be regarded as one, because they are an integrated system. The separation between these subsystems is also the place where this interaction manifests: the wheel-rail contact, which enables vehicle bearing and guidance (Li et al., 2016).
Both the railway vehicle and the permanent way have irregularities, which produce different magnitudes of force due to the resonances they create within the permanent way components (Tzanakakis, 2013). To understand the relationship between permanent way failure behavior and track geometry, it is important to identify the forces on the rail created by a train traveling on it, and the responses made to those forces. It is also important to recognize the causes of these forces to be able to eradicate them and increase the longevity of the rail (Tzanakakis, 2013).
According to Li et al. (2016), wheel loads and train speeds have increased and lines have been optimized during the last decade, which has placed increased demands on the track structure. A combination of delayed permanent way maintenance together with more production (i.e., traffic, axle load, and speed) has caused the rate of permanent way degradation to increase (Martland, 2013). The most significant factor in degradation (wear, fatigue, and settlement) is therefore the dynamic load (De Man, 2002; Kaewunruen and Remennikov, 2008), which is related to the axle load and track geometry (Tzanakakis, 2013). Many factors can influence the permanent way and adequate methods must be applied in maintaining it (Jovanovic et al., 2014).
Track condition is divided into two groups of measurable parameters. The first contains the component deterioration parameters, which is the usual term used to describe the deterioration of each component in a permanent way (El-Sibaie and Zhang, 2004). In other words, it is which, how, when, and how much a component with a specific composition, form, dimension, and mechanical property loses its function as part of the permanent way. According to Guler et al. (2011), it is hard to apply a unique descriptor that records the status of all deterioration.
On the other hand, according to Vale and Ribeiro (2014) the second group of measurable parameters, namely geometry degradation is random by nature. The geometry of the permanent way is the position of the railway track in three-dimensional space (Faiz and Singh, 2009). According to Vale and Ribeiro (2014), track degradation is the decrease in quality of geometrical parameters (i.e., longitudinal and transversal level, gauge, twist, and cant) over a period of time. In another definition, track geometry degradation is which, how, when, and how much one or more than one geometric dimension in a finite space of the track fails to maintain their known standard characteristics. Component deterioration and geometry degradation are both affected by the environment, traffic, vehicle speed, construction methods, and maintenance history.
Bing and Gross (1983) discuss a common basic method of degradation analysis, which involves using an empirical model (i.e., correlation, variance, and regression) to assess a huge sample of observations about track parameters. However, alterations in data recording and explanation may undermine the outcomes. Simulating track degradation in this way makes it possible to allow for uncertainty in predictions of track degradation, as they are expected to increase with time, due to the imperfect methods of determination of the input parameters used in the model (Bing and Gross, 1983).
Additionally, in empirical modeling, a modern approach called Artificial Intelligence – AI (i.e., Artificial Neural Networks – ANNs and Neuro-Fuzzy Logic – NFL, a combination between ANN and fuzzy logic) is increasingly used among scientists, as discussed by Elkhoury et al. (2018). These methods are recognized to have high predictive accuracy. In multi-layered neural networks, the neurons are arranged in a layered fashion. The input and output layers are separated by a group of hidden layers in which the layer-wise architecture of the neural network is referred to as a feed-forward network (Aggarwal, 2018). Guler (2014) modeled railway track geometry degradation with ANN, considering the variables involved in track geometry degradation, which produced important findings on the relationships between the rate (deterioration) and independent variables.
An alternative to empirical models is mechanistic models, which involve establishing the mechanical properties of track components (Zhang et al., 2000). They are based on physical information, establishing the mechanical properties of all the elements of the track and railway vehicles (Sadeghi and Askarinejad, 2011). Track structure analysis methods based on mechanical models are used to calculate individual track components including forces, stresses, and defects. They are successful in calculating forces, tensions, and the probability of the development of failures in the individual components of the permanent way (Zhang et al., 2000).
According to Soleimanmeigouni et al. (2016) several researchers, including Sadeghi and Askarinejad (2010) and Rhayma et al. (2013), have also attempted to combine physical and empirical models to explore the best application of both methods. By complementing the mechanistic model with an empirical one, it is possible not only to study the structural behavior of the track components, but also to analyze the functional performance of the track geometry. Thus, this leads to the development of an empirical-mechanistic method, which allows a more dynamic and current interaction.
Shafahi and Hakhamaneshi (2009) have compared four models including one mechanistic model suggested by the Office for Research and Experiment of the International Union of Railways (ORE) and three empirical models: the Markov chain model, the ANN, and the Neuro-Fuzzy models. In this study, the Markov model proved to be robust in predicting the random behavior of the track deterioration process, and seems to be superior to conventional regression models, such as the ORE model.
The full railway track complex is preserved to deliver satisfactory track geometry. This is why there are track components. Repair decisions are frequently controlled by the geometry, and they are necessary not only when many track rail failure corrections ruin the geometry, but also in instances when track ballasts can no longer preserve the design geometry, or sleepers and fastenings cannot conserve the permanent way gauge. However, according to Esveld (2001), the process of determining whether, when, where, and how best to intervene is far more complex, as it involves evaluation of track condition and how much it is influenced by both track structure and track geometry, and consideration of the relationship between them. Systematically identifying the tactics (model and approach) that are used around the world to evaluate track deterioration is the first step in establishing the gaps in literature on this topic and proposing new techniques to fill them.
Methodology
Based on the systematic literature review method proposed by Kitchenham (2004) and Torres-Carrion et al. (2018), this research is divided into planning, conducting, and reporting the review. Figure 1 provides an overview of the macro-procedure of this methodology.
Planning
This systematic review aims to summarize existing information on track deterioration. Prior to conducting this review and following Torres-Carrion et al. (2018), it was ensured that the review itself was necessary, live reviews of the phenomenon of interest were identified. The current state of track degradation studies is the starting point of this review, before the development of research questions, and a contextualization of the scenario of research.
The following research questions are proposed in this paper:
• Research Question 1 (RQ-1): Which methods have been developed in predicting an integrated track deterioration in an in-service railway?
• Research Question 2 (RQ-2): How have these methods been designed?
• Research Question 3 (RQ-3): What predicting technologies have been applied to monitor the track deterioration processes?
One of the fundamental devices used to provide a good understanding of the issue was the “mentefacto conceptual,” a tool for addressing a complex situation, represented by an ideogram (Torres-Carrion et al., 2018). In this paper, the track deterioration is the issue, and the permanent way or track is the study. This is different from some kinds of regular interaction, for instance, track design, and track schedule. This process permits monitoring before a track component fails (Yan et al., 2019); it also provides information on downgrading the track quality. Figure 2 shows the “mentefacto conceptual” of this review.
The platform used for the first filtering is “Primo de Ex Libris,” under library license. This is a systematic search using a semantic sentence in the English language and identifying literature through specific words.
Conducting
In Kitchenham (2004), when the protocol has been accepted, the review can be developed. The steps are iterative/incremental, which means the research will run until the research questions are answered. The main idea of this type of review is to find as many primary sources related to the research question as possible avoiding, for example, a language bias. The “identification of research” is complementary to the protocol. Torres-Carrion et al. (2018) also suggest conducting a literature search in the Web of Science, Scopus, and Google Scholar. Once the relevant potential primary studies have been identified, they need to be assessed for their relevance (Kitchenham, 2004).
The sub-stage study assessment is supported by the criteria of inclusion and exclusion, the complexities of both the method and the validation, and the study quality, represented in criteria such as approach, systematics, extension, context, and peer review, among others. In this paper, it is proposed that the primary studies will be assessed according to three indicators: the complexity of the method, complexity of the validation, and study quality. A primary issue is that there is no agreed concept of study quality.
In this research the complexity of the method is used as an indicator of how much the method considers the requirements necessary for it to be complete. It has a rank of −5 to +5, which represents how much the study applies its potential to develop the research about track conditions. In order to assess the complexities of the methods of the primary studies previously listed, seven criteria are proposed: approach, type (or model), systematics, element, segment, scale, and extension. The maximum total value (35 points) and the minimum total value (seven points) are marked on a scale of −5 to +5, proportionality. The criteria used are described in Table 1.
A second indicator – the complexity of the validation – is also ranked from −5 to +5, according to whether the studies validate their respective research on track condition. In order to assess the complexities of the validations of the studies previously listed, six criteria are proposed: time period, reach, the error of measurement, context, correlation, and test-oriented. The maximum total value (30 points) and the minimum total value (6 points) also fit to a scale of −5 to +5. The criteria used are described in Table 2.
In this paper, study quality is the third proposed indicator, which is ranked from 1 to 5, representing how much the study applies the full potential to develop the research about track conditions. To assess the quality of the primary studies examined, eight criteria were used: peer review, thick description, analysis of the variables, omitted variable bias, credibility, transferability, reliability, and confirmability. The maximum total value (40 points) and the minimum total value (8 points) are fitted to a scale of 1–5. The criteria are presented in Table 3.
With the objective of identifying the groups in which the listed studies might be classified, it is that the values be plotted in a scatter bubble chart, showing on the X-axis, the complexity of the validation; on the Y-axis, the complexity of the method; and, on the diametric independent axis, the study quality. At this stage, the quality of the systematic review is defined (Torres-Carrion et al., 2018). This synthesis is descriptive, involving a quantitative summary that shows the principal findings and gaps.
Reporting the Review
According to Torres-Carrion et al. (2018), outcomes are published to the scientific community to gain opinion from other experts in the field. Systematic improvements to a review are always needed and this is a continuous process that has seen early benefits as a result of its implementation.
Results and Discussion
In this paper, the results and discussions are organized as follows: the related literature reviews of the issue, then the primary studies about track deterioration, and then a summary of these primary studies.
Related Literature Reviews
As described in the methodology section, the related literature reviews of track deterioration answer the research questions proposed in this paper. The answer to each of the six studies is no, at least partially. Table 4 presents these studies, charting whether they have answered the research questions.
The unanswered research questions are specified and labeled in Table 4. Although the literature reviews in these studies do not fully answer the research questions, they support this paper by complementing the survey.
Primary Studies
Using the methodology outlined above, 106 primary studies were initially selected. Most of these are concentrated in five countries, China, the UK, the US, Sweden, and Iran, however, other countries such as Germany, India, Japan, Spain, Austria, and Switzerland are also represented. The US is a highlight due to the research carried out by the Association of American Railroads – AAR through the Transportation Technology Center, Inc. (TTCi), and supported by the Federal Railroad Administration (FRA), a regulatory authority. Most of these studies examine high speed or cargo/passenger railway systems, and the papers were published in peer-reviewed journals during the between 2010 and 2019. Most studies have an observable approach (measured data from a recording vehicle, for instance), a supporting empirical formulation (statistical analysis such as regression and/or probability), and focus on track geometry. Figure 3 illustrates this data.
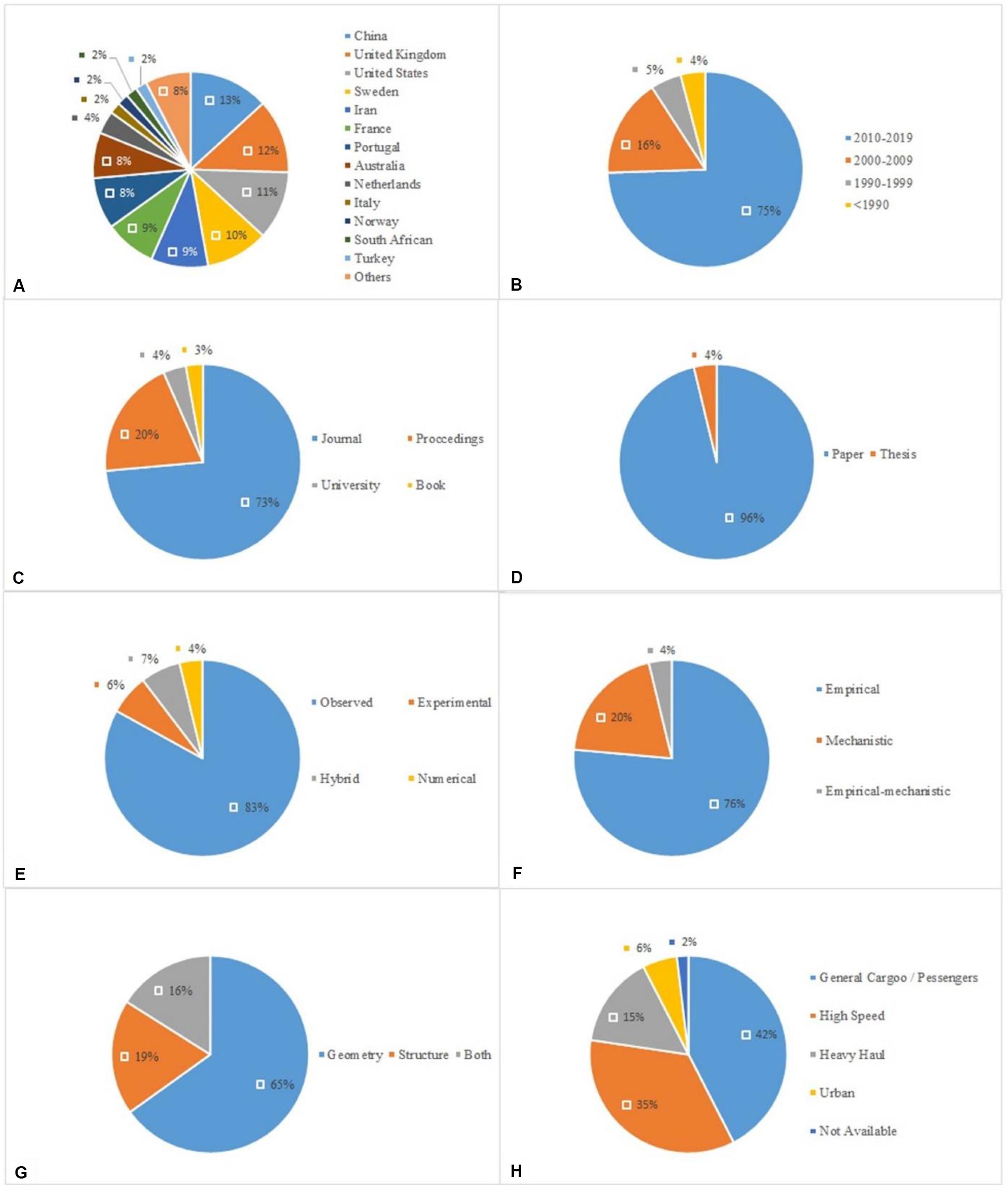
Figure 3. Primary studies: (A) applied country, (B) published in, (C) sort of publication, (D) document type, (E) approach, (F) model, (G) element studied, and (H) railway system.
An empirical model was built from a set of input and output variables. In modeling deterioration this can be used to deal with many descriptive factors that influence track conditions (Yousefikia et al., 2014). One of the advantages of this method is that, since actual data is used to build the deterioration process, an adequate estimate of the track condition can be obtained (Yousefikia et al., 2014). On the other hand, a major drawback is the lack of a physical basis for permanent way components and their interactions, which may result in some directionless outcomes (Sadeghi and Askarinejad, 2010).
The mechanistic model involves establishing the mechanical properties of track components. One of the advantages of this is that it can integrate the reaction of the track to production parameters. For instance, a unique defect on the fastening system may not cause any significant consequences, but several defects will cause the deterioration of other components and the full track. This indicates that these models cannot handle a range of operating, environmental, and maintenance conditions and do not allow for different degradation behaviors (Lovett et al., 2013).
Dahlberg (2001) and Guler et al. (2011) present other studies on the mechanistic formulation, discussing advantages and drawbacks. Guler et al. (2011) analyzed several mechanistic methods looking at the degradation of permanent way geometry, including the track damage model proposed by Sato (1995), the track degradation developed by British Rail Research, and the settlement model presented by the Technical University of Munich. Dahlberg (2001), comparing several important track prediction models, including those from Japan, the United States, the European Union, Africa, and Australia.
Sadeghi and Askarinejad (2010) have elaborated on a deterioration model combining mechanistic novel and statistics that took into account data on both track geometry and track components. The basic advantage derived from the use of an empirical-mechanistic model is that it allows the parameterization of geometry and basic properties. Therefore, these parameters can be altered and adapted to the different range requirements (Melo et al., 2019a), allowing for spatial variations, temporal effects, and fatigue of the elements.
Additionally, for the success of an empirical-mechanistic model, it is necessary to carry out laboratory and field experiments (examining track components) in building experimental models, using measurement data (track geometry) in the calibration process. Examples gathered in the field must take into account the sort of segment (straight, curve, switch, bridge, tunnel, ramp up/down, etc.) and build all the elements of the permanent way in the laboratory. Additionally, avoiding bias, and allowing for measurement errors and validation tests is essential (Melo et al., 2019b). This model is partially observed in studies by Guerin (1996) and Frohling (1997), and in models by Sadeghi and Askarinejad (2007) and Rhayma et al. (2013). These four studies approximate the hypothetical target when researching the track deterioration process (Melo et al., 2019b), because they apply specifically – but not only – the empiric-mechanistic model and the hybrid (numerical and experimental) approach to the study of track deterioration.
After analyzing and selecting these primary studies, they are classified in four different groups: Level III (L-III; low complexity of both the method and the validation), Level II-a (L-II-a; high complexity of the method and low complexity of the validation), Level II-b (L-II-b; low complexity of the method and high complexity of the validation), and Level I (L-I; high complexity of both the method and the validation). Additionally, the selected studies are assessed by their quality, as described in the methodology (Section “Methodology” above).
To offer one a more facetted assessment of these primary studies, an indicator of study quality was applied. The studies were classified into a range of 1–5, independently of the group previously described. This indicates whether the study has been subject to checks and controls, including a peer review. Most of the studies selected were evaluated as being 2 on the scale from 1 to 5. Figure 4 illustrates this data, and Figure 5 summarizes the study assessment.
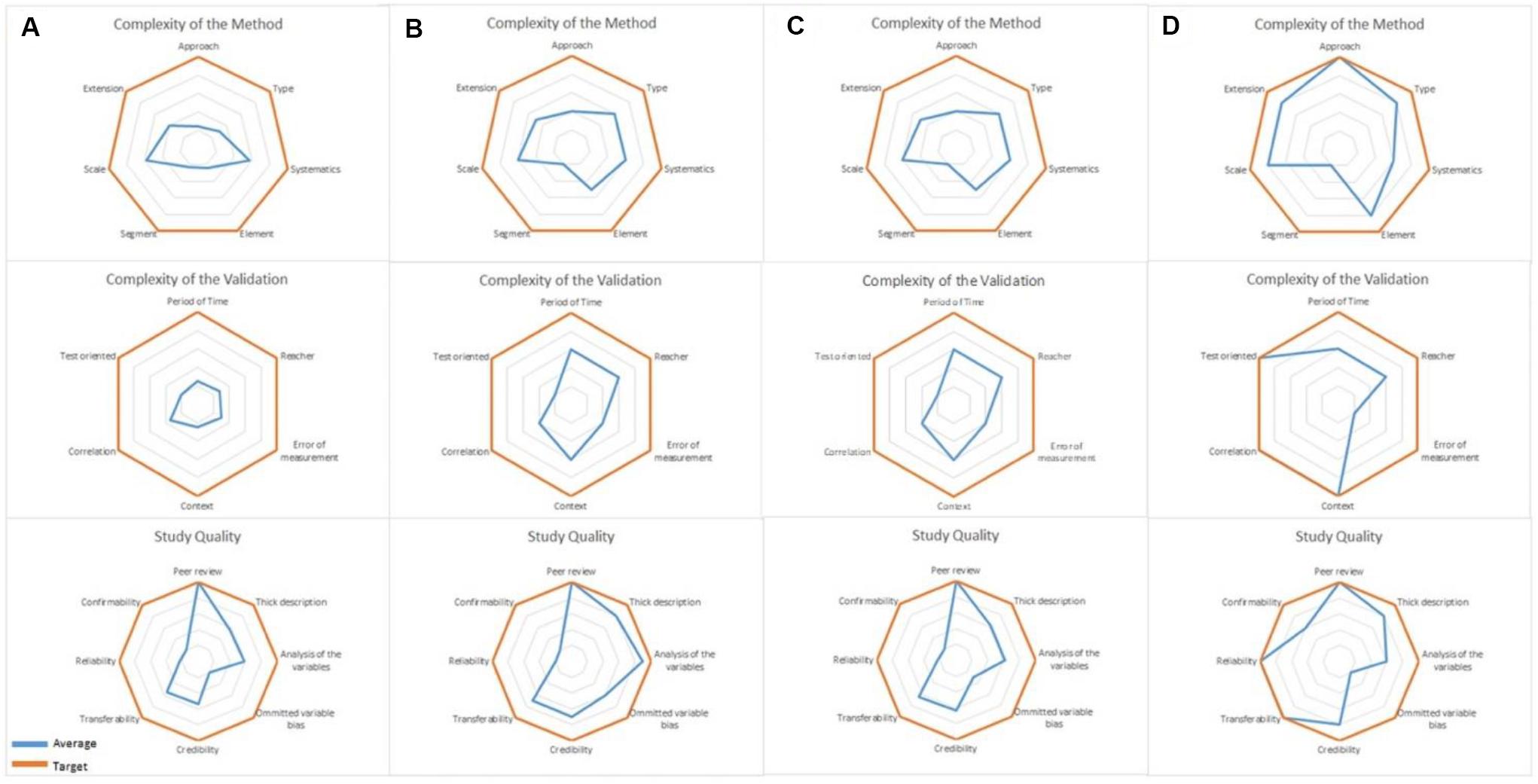
Figure 5. The average of complexity of both the method and the validation, and the study quality of the primary studies classified in groups: (A) L-I, (B) L-II-b, (C) L-II-a, and (D) L-III.
Level III (L-III) Group
L-III is the group where most of the studies are plotted (73%). Based on the criteria of the complexity of the method and the complexity of the validation, the L-III group includes studies that have low complexity in both the method and the validation. This means that the studies have not only applied a more observable (not experimental) approach and empirical analysis but also carried out a short validation process.
Level II-b (L-b-II) Group
The L-II-b is the second group where most of the studies are plotted (23%). This group includes studies that have low complexity in their method and high complexity in validation. This means that the studies have focused more on validating the method than on the method itself. In this case, there is more attention paid to the measurement of errors. In turn, these studies have applied a more empirical formulation.
Level II-a (L-a-II) Group
The L-II-a group represents studies that have high complexity in their method and low complexity in validation. This means that these studies have used more numerical and/or experimental approaches, and empirical mechanistic models. On the other hand, less attention has been given to the validation process.
Level I (L-I) Group
Lastly, there is a group named L-I, which has few studies classified into it (only two studies in total). The L-I group represents studies that have high complexity in both their method and validation. This means that the studies have not only applied more numerical and/or experimental approaches, empirical mechanistic models, and systematic research (field, laboratory, and office) but also, that they have developed an extended validation process. It is at this level that the best methods for studying track deterioration are located.
Summary of the Primary Studies
The primary studies included in this review are summarized in Figure 6 and Table 5, which illustrates an overview of all 106 selected studies. The figures show the concentration of these studies in the groups L-III and L-II-b, with low complexity of the method, varied complexity of the validation, with most of them ranked as a 2 for study quality. This means that most of the studies related to the track deterioration process do not have the necessary complexity and quality to deal with evaluating the track condition and how much it is influenced by both track structure and track geometry, as well as the relations between them. In other words, they do not have the accuracy to predict track deterioration. This may explain why, to some extent, this review largely found that studies were underpinned by empirical models (statistical, for example), which are more easily developed than, for instance, mechanistic or even empirical mechanistic ones.
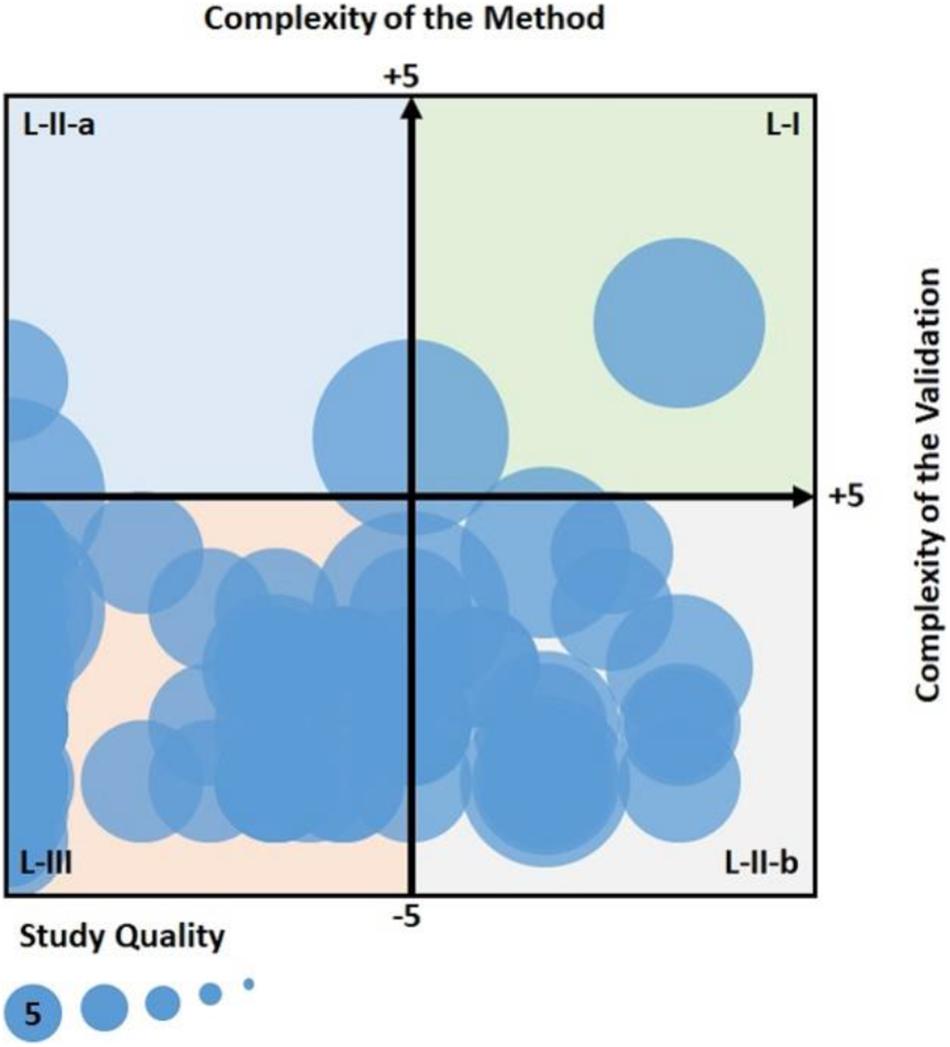
Figure 6. The study groups in according to the complexity of the validation, the complexity of the method, and the study quality.
Supported by these studies and findings, it is possible to identify which tactics – including model and approach – have been established by more than one peer review process. Table 6 shows that the most of valid tactics (established tactics) are related to both empirical (statistical) models and observable approaches (recording vehicles, for example) when these are applied to the elements of track components (except “all” elements together). This replicates an empiric model when it is associated with the elements of track geometry, being the most usual and less complex procedure. This may also clarify why most of the studies under investigation are related to the empirical formulation (both track components and track geometry), and also observable approaches (applied predominantly to the track geometry). In different circumstances, there are more complex techniques, termed empirical mechanistic models and hybrid (numerical and experimental) approaches. These are recognized as potential tactics and are applied in various studies, indicating potential opportunities in the development of more advanced investigations of track deterioration.
This critical review has two main outcomes. In general, the studies included in this literature review do not deal with the interrelation between track components and track geometry when analyzing track deterioration. The most used and least complex techniques (empirical models and observable approach, not experimental) are “established tactics” while the less ordinary and more complex ones (empirical mechanistic models and the hybrid approach) are recognized generally as potential tactics. In other words, few studies have developed advanced investigation techniques for analyzing track deterioration with numerical and experimental modeling supported by statistic, probabilistic, and mechanistic models together.
Although it is recognized that this review does not include all existing studies, this critical systematic literature review does establish a reasonable understanding of how track deterioration is discussed. These studies provide insights that will aid in the development of this research over time, indicating that approaches to track deterioration must be updated. That is also why this kind of critical literature review is qualified as “systematic.” In response, it is recommended that future reviews revise this methodology and increase its accuracy to include other languages in the search, such as Spanish, French, German and Portuguese, and improving the “mentefacto conceptual” by including for example, the keywords “fouling” and “wear” as related to, respectively, the ballast and the rail.
Conclusion
This study has proposed a procedure for undertaking a systematic literature review identifying, evaluating, and classifying primary studies that predict the process of track deterioration (structure) and degradation (geometry) in an in-service railway. More than 100 studies that deal with this process were selected, most of which concentrated on five countries, namely China, the UK, the US, Sweden, and Iran, though it did identify some studies focused on other countries, including Germany, India, Japan, Spain, Austria, and Switzerland. Most of these discuss high speed or general cargo/passenger railway systems and were published in peer-reviewed journals during the last 10 years.
Although there are a wide variety of methodologies for evaluating track condition, generally they have focused on an observable approach (recording vehicle) of the track geometry, supported by empirical analysis (statistical), with a low degree of validation, as shown in Figure 6 (Level III group) and Table 6 (established tactic). It is recognized that this critical review has its limitations and it could be improved once other languages and countries are included in the search and reviewed using the “mentefacto conceptual.”
The figures reveal that despite the advances in statistic analytical methods there remains scope to improve existing track condition methodologies. One of these improvements is related to applying the best theoretical target in fully predicting track deterioration (Level I group), which includes associating the empirical mechanistic model (the best of statistic and mechanistic formulation) and the hybrid (numerical and experimental) approach. This is the main finding of this investigation.
In practice, unlike other techniques, a hybrid numerical and experimental approach and an empirical mechanistic model together may take into account the interactions between track elements (track components and track geometry) and the environmental effects through real measured data (experimental), and a complex mathematical calculation (numerical analysis and mechanistic model). Additionally, these tactics assume uncertainties (statistic and probabilistic model) in preventing even the best method being a perfect in model over time, due either to input parameters, for example axle loads, temperature variation, and material strengths, and outcomes such as displacements, forces, stresses, and strains. This systemic review has attempted to demonstrate that by using technological advances in computational methods and by incorporating these techniques, it is possible to fill the current gap in modeling, and create models that allow for the multiple processes that lead to track degradation.
Author Contributions
AM wrote the manuscript with support from other authors and developed the systematic research. SK, MP, LB, and RM gave very useful comments on this manuscript and contributed to the proposal methodology. All authors contributed to the article and approved the submitted version.
Funding
This project has received funding from the European Union’s Horizon 2020 Research and Innovation Programme Under Grant Agreement No. 691135. The Article Processing Charges (APC) was funded by the University of Birmingham Library’s Open Access Fund.
Conflict of Interest
The authors declare that the research was conducted in the absence of any commercial or financial relationships that could be construed as a potential conflict of interest.
Acknowledgments
AM gratefully appreciates the Brazilian National Council for Scientific and Technological Development (CNPq), Brazil, Project No. 200359/2018-5, for his Ph.D. scholarship. We are sincerely grateful to the European Commission for the financial sponsorship of the H2020-RISE Project No. 691135 “RISEN: Rail Infrastructure Systems Engineering Network,” which enables a global research network that tackles the grand challenge of railway infrastructure resilience and advanced sensing in extreme environments (Kaewunruen et al., 2016).
References
Ahac, M., and Lakusic, S. (2015). Tram track maintenance-planning by gauge degradation modelling. Transport 30, 430–436. doi: 10.3846/16484142.2015.1116464
Al-Jubooria, A., Zhua, H., Wexlera, D., Li, H., Lu, C., McCuskera, A., et al. (2019). Evolution of rail surface degradation in the tunnel: the role of water on squat growth under service conditions. Engin. Fract. Mechan. 209, 32–47. doi: 10.1016/j.engfracmech.2019.01.018
An, R., Sun, Q., Wang, F., Bai, W., Zhu, X., and Liu, R. (2018). “Railway track integral maintenance index and its application,” in Proceedings International conference on transportation engineering 2009. (China: ASCE), 2514–2519.
Andrade, A., and Teixeira, P. (2011). Uncertainty in rail-track geometry degradation Lisbon-Oporto line case study. J. Transpor Engin. 137, 193–200. doi: 10.1061/(ASCE)TE.1943-5436.0000206
Andrade, A., and Teixeira, P. (2012). A Bayesian model to assess rail track geometry degradation throughout its lifecycle. Res. Transpor. Econ. 36, 1–8. doi: 10.1016/j.retrec.2012.03.011
Andrade, A., and Teixeira, P. (2013a). Hierarchical Bayesian modeling of rail track geometry degradations. J. Rail Rapid Transit 227, 364–375. doi: 10.1177/0954409713486619
Andrade, A., and Teixeira, P. (2013b). Unplanned-maintenance needs related to rail track geometry. Proc. Institut. Civil Engin. 167, 400–410. doi: 10.1680/tran.11.00060
Andrade, A., and Teixeira, P. (2015). Statistical modelling of railway track geometry degradation using hierarchical Bayesian models. Reliab. Engin. Sys. Safe. 142, 169–183. doi: 10.1016/j.ress.2015.05.009
Andrews, J. (2012). A modelling approach to railway track asset management. J. Rail Rap. Trans. 227, 56–73. doi: 10.1177/0954409712452235
Andrews, J., Prescotta, D., and Rozieresb, F. (2014). A stochastic model for railway track asset management. Reliab. Engin. Sys. Safe. 130, 76–84. doi: 10.1016/j.ress.2014.04.021
Antoni, M., and Meier-Hirmer, C. (2008). Economic correlation between maintenance and regeneration – optimization of maintenance strategies for tracks, signaling equipment and overhead line components. Australia: World Congress on Railway Research.
Audley, M., and Andrews, J. (2013). The effects of tamping on railway track geometry degradation. J. Rail Rap. Trans. 227, 376–391. doi: 10.1177/0954409713480439
Bai, L., Liu, R., Sun, Q., Wang, F., and Feng, W. (2016). Classification-learning-based framework for predicting railway track irregularities. J. Rail Rap. Trans. 230, 598–610. doi: 10.1177/0954409714552818
Bai, L., Liu, R., Sun, Q., Wang, F., and Xu, P. (2015). Markov-based model for the prediction of railway track irregularities. J. Rail Rap. Trans. 229, 150–159. doi: 10.1177/0954409713503460
Berawi, A. R., Delgado, R., Calçada, R., and Vale, C. (2010). Evaluating track geometrical quality through different methodologies. Internat. J. Technol. 1, 38–47.
Bing, A. J., and Gross, A. (1983). Development of Railroad Track Degradation Models. Transport. Res. Rec. 939, 27–31.
Cardenas-Gallo, I., Sarmiento, C. A., Morales, G. A., Bolivar, M. A., and Akhavan-Tabatabaei, R. (2017). An ensemble classifier to predict track geometry degradation. Reliab. Engin. Sys. Safe. 161, 53–60. doi: 10.1016/j.ress.2016.12.012
Chang, H., Liu, R., and Li, Q. (2010a). Computer System Design and Operation in Railways and Other Transit Systems, Traffic and Transportation Studies. England, UK: WIT Press.
Chang, H., Liu, R., and Wang, W. (2010b). Multistage linear prediction model of track quality index. Bangalore: Traffic and Transportation Studies. doi: 10.1061/41123(383)112
Chaolong, J., Weixiang, X., Futian, W., and Hanning, W. (2012). Track irregularity time series analysis and trend forecasting. Discr. Dyn. Nat. Soc. 2012, 1–15. doi: 10.1155/2012/387857
Chen, R., Yang, K., Qiu, X., Zend, X., Wang, P., Xu, P., et al. (2017). Degradation mechanism of CA mortar in CRTS I slab ballastless railway. Construct. Build. Mater. 149, 921–933. doi: 10.1016/j.conbuildmat.2017.04.017
Chiachio, J., Chiachio, M., Prescotta, D., and Andrews, J. (2019). A knowledge-based prognostics framework for railway track geometry degradation. Reliab. Engin. Sys. Safe/ 181, 127–141. doi: 10.1016/j.ress.2018.07.004
Corriere, F., and Vincenzo, D. (2012). The rail quality index as an indicator of the “Global Comfort” in optimizing safety, quality and efficiency in railway rails. Amsterdam: Elsevier.
Dahlberg, T. (2001). Some railroad settlement models – a critical review. J. Rail Rap. Trans. 215, 289–300. doi: 10.1243/0954409011531585
Das, A., and Bajpai, P. K. (2018). A hypo-plastic approach for evaluating railway ballast degradation. Acta Geotecnh. 13, 1085–1102. doi: 10.1007/s11440-017-0584-7
De Man, A. P. (2002). A Survey of dynamic railway track properties and their quality. Ph.D. dissertation. The Netherlands: Delft University.
Dell’Orco, M., Ottomanelli, M., Caggiani, L., and Sassanelli, D. (2008). New decision support system for optimization of rail track maintenance planning based on adaptive neurofuzzy inference system. J. Transpor. Res. Board 2043, 49–54. doi: 10.3141/2043-06
Do, N. T., Nafari, S. F., and Gul, M. (2017). “Estimation of track modulus over long distances using artificial neural network,” in Proceedings International Conference on Structural Dynamics, (Amsterdam: Elsevier). doi: 10.1016/j.proeng.2017.09.482
Elkhoury, N., Hitihamillage, L., Moridpour, S., and Robert, D. (2018). Degradation prediction of rail tracks: a review of the existing literature. Open Transpor. J. 12, 88–104. doi: 10.2174/1874447801812010088
El-Sibaie, M., and Zhang, Y. (2004). Objective track quality indices. J. Transpor. Res. Board 1863, 81–87. doi: 10.3141/1863-11
Faiz, R. B. (2010). An empirical rail track degradation model based on predictive analysis of rail profile and track geometry. Ph.D. dissertation UK: Loughborough University.
Faiz, R. B., and Singh, S. (2009). “Time based predictive maintenance management of UK rail track,” in International Conference on Computing, Engineering and Information, (New Jersy: IEEE). doi: 10.1109/ICC.2009.70
Falamarzi, A., Moridpour, S., Nazem, M., and Hesami, R. (2018). Rail degradation prediction models for tram system: Melbourne case study. J Adv. Transpor. 2018:6340504 doi: 10.1155/2018/6340504
Ferreira, L., and Murray, M. H. (1997). Modelling rail track deterioration and maintenance: current practices and future needs. Transpor. Rev. 17, 207–221. doi: 10.1080/01441649708716982
Frohling, R. D. (1997). Deterioration of railway track due to dynamic vehicle loading and spatially varying track stiffness. Ph.D. dissertation South African: University of Pretoria.
Gong, C., Iwnicki, S., and Bezin, Y. (2016). The effect of railway vehicle dynamics on the lateral alignment of track. J. Rail Rap. Trans. 230, 258–270. doi: 10.1177/0954409714536548
Guerin, N. (1996). Approche experimental et numerique du comportement du ballast des voies ferrees. Ph.D. dissertation France: ParisTech.
Guler, H. (2014). Prediction of railway track geometry deterioration using artificial neural networks a case study for Turkish state railways. Struct. Infra. Engin. 10, 614–626. doi: 10.1080/15732479.2012.757791
Guler, H., Jovanovic, S., and Evren, G. (2011). Modelling railway track geometry deterioration. P. I. Civil Eng-Transp. 164, 65–75. doi: 10.1680/tran.2011.164.2.65
Guo, R., Han, B., and Wang, F. (2010). “Traffic and transportation studies,” in Proceedings of the 7th International Conference on Traffic and Transportation Studies, August 3-5, 2010, Kunming, China, (Reston: American Society of Civil Engineers).
Hamid, A., and Gross, A. (1981). Track-quality indices and track degradation models for maintenance-of-way planning. J. Transpor. Res. Board 802, 2–8.
He, Q., Li, H., Bhattacharjya, D., Parikh, D. P., and Hampapur, A. (2015). Track geometry defect rectification based on track deterioration modelling and derailment risk assessment. J. Operat. Res. Soc. 66, 392–404. doi: 10.1057/jors.2014.7
Higgins, C., and Liu, X. (2018). Modeling of track geometry degradation and decisions on safety and maintenance: a literature review and possible future research directions. J. Rail Rap. Trans. 232, 1385–1397. doi: 10.1177/0954409717721870
Hokstad, P., and Langseth, H. (2005). Failure modeling and maintenance optimization for a railway line. Internat. J. Perform. Engin. 1, 51–64.
Ivanov, Y. F., Morozovc, K. V., Peregudovc, O. A., and Gromovc, V. E. (2016). Degradation of rail-steel structure and properties of the surface layer. Steel Transl. 46, 567–570. doi: 10.3103/S0967091216080088
Iwnicki, S. (2006). Handbook of Railway Vehicle Dynamics. USA: CRC Press. doi: 10.1201/9781420004892
Jovanovic, S. (2004). “Railway track quality assessment and related decision making,” in International Conference on Systems, Man and Cybernetics, (Netherlands: The Hague).
Jovanovic, S., Bozovic, D., and Tomicic-Torlakovic, M. (2014). Railway infrastructure condition-monitoring and analysis as a basis for maintenance management. Gradevinar 66, 347–358.
Jovanovic, S., Guler, H., and Coko, B. (2015). Track degradation analysis in the scope of railway infrastructure maintenance management systems. Gradevinar 67, 247–257.
Kaewunruen, S., and Remennikov, A. M. (2008). Dynamic properties of railway track and its components: a state-of-the-art review. Australia: University of Wollongong.
Kaewunruen, S., Sussman, J. M., and Matsumoto, A. (2016). Grand challenges in transportation and transit systems. Front. Built Envir. 3:6. doi: 10.3389/fbuil.2016.00004
Karimpour, M., Hitihamillage, L., Elkhoury, N., Moridpour, S., and Hesami, R. (2017). Nonlinear estimation model for rail track deterioration. J. Transpor. Vehicle Engin. 11, 1591–1595.
Karttunen, K., Kabo, E., and Ekberg, A. (2016). Estimation of gauge corner and flange root degradation from rail, wheel and track geometries. Wear 366, 294–302. doi: 10.1016/j.wear.2016.03.030
Kawaguchi, A., Miwa, M., and Terada, K. (2005). Actual data analysis of alignment irregularity growth and its prediction model. J-STAGE 46, 262–268. doi: 10.2219/rtriqr.46.262
Kearsley, E. P., and As, S. C. (1995). Deterioration of Rail Track Geometry∗. J. South Afr. Institut. Civil Engin. 2, 121–145
Khouy, I. A., Larsson-Kraik, P., Nissen, A., and Kumar, U. (2016). Cost-effective track geometry maintenance limits. J. Rail Rap. Trans. 230, 611–622. doi: 10.1177/0954409714542859
Khouy, I. A., Schunnesson, H., Juntti, U., Nissen, A., and Larsson-Kraik, P. (2014). Evaluation of track geometry maintenance (Tamping) in Swedish heavy haul railroad – a case study. J. Rail Rap. Trans. 228, 496–503. doi: 10.1177/0954409713482239
Khouzani, A. H. E., Golroo, A., and Bagheri, M. (2014). Railway maintenance management using a stochastic geometrical degradation model. J Transpor. Engin. 143:04016002. doi: 10.1061/JTEPBS.0000002
Kitchenham, B. (2004). Procedures for Performing Systematic Reviews. Joint Technical Report. UK: Keele University.
Kumar, S., Espling, U., and Kumar, U. (2008). Holistic procedure for rail maintenance in Sweden. J. Rail Rap. Trans/ 222, 331–334. doi: 10.1243/09544097JRRT177
Le Pen, L. (2008). Track behaviour: the importance of the sleeper to ballast interface. Ph.D. dissertation (UK: University of Southampton).
Li, H., and Xiao, T. (2014). Improved generalized energy index method for comprehensive evaluation and prediction of track irregularity. J. Stat. Comput. Sim. 84, 1213–1231. doi: 10.1080/00949655.2013.797420
Li, D., Meddah, A., Hass, K., and Kalay, S. (2006). Relating track geometry to vehicle performance using neural network approach. J. Rail Rap. Trans 220, 273–281. doi: 10.1243/09544097JRRT39
Lobo-Guerrero, S., and Vallejo, L. E. (2006). Discrete Element Method Analysis of Railtrack Ballast Degradation during Cyclic Loading. Granular Matt. 8, 195–204. doi: 10.1007/s10035-006-0006-2
Lovett, A. H., Dick, C., Tyler, Ruppert, C. Jr., Saat, M. R., and Barkan, C. (2013). “Development of an integrated model for the evaluation and planning of railroad track maintenance,” in Joint Rail Conference, JRC 2013 [JRC2013-2407] (2013 Joint Rail Conference, JRC 2013), Italy. doi: 10.1115/JRC2013-2407
Luber, B., Haigermoser, A., and Grabner, G. (2010). Track geometry evaluation method based on vehicle response prediction. Vehicle Sys. Dyn 48, 157–173. doi: 10.1080/00423111003692914
Lyngby, N. (2009). Railway track degradation shape and influencing factors. J. Performab. Engin. 5, 177–186.
Mahmoud, E., Papagiannakis, A. T., and Renteria, D. (2016). Discrete element analysis of railway ballast under cycling loading. Internat. Conferen. Transport. 143:1068-1076 doi: 10.1016/j.proeng.2016.06.221
Martland, C. (2013). Introduction of heavy axle loads by the North American rail industry. J. Transport. Res/ Forum 52, 103–125. doi: 10.5399/osu/jtrf.52.2.4162
Meier-Hirmer, C., Riboulet, G., Sourget, F., and Roussignol, M. (2009). Maintenance optimization for a system with a gamma deterioration process and intervention delay: application to track maintenance. J. Risk Reliab. 223, 189–198. doi: 10.1243/1748006XJRR234
Meier-Hirmer, C., Senee, A., Riboulet, G., Sourget, F., and Roussignol, M. (2006). Computer System Design and Operation in the Railway and Other Transit Systems. UK: WIT Press.
Melo, A., Kaewunruen, S., and Papaelias, M. (2019a). “Parameters and Boundary Conditions in Modelling the Track Deterioration in a Railway System,” in World Multidisciplinary Civil Engineering – Architecture – Urban Planning Symposium – WMCAUS, (Prague: Czech Republic).
Melo, A., Kaewunruen, S., and Papaelias, M. (2019b). “Hybrid approach to predict the track deterioration in a railway in-service: a conceptual design,” in World Multidisciplinary Civil Engineering – Architecture – Urban Planning Symposium – WMCAUS, (Prague: Czech Republic).
Mercier, S., Meier-Hirmer, C., and Roussignolc, M. (2012). Bivariate Gamma wear processes for track geometry modelling, with application to intervention scheduling. Struct. Infra. Engin. 8, 357–366. doi: 10.1080/15732479.2011.563090
Minbashi, N., Bagheri, M., Golroo, A., and Khouy, I. A. (2017). Geometrical degradation analysis of railway turnouts using power spectral density. J Transport. Engin. 143:04017035. doi: 10.1061/JTEPBS.0000056
Ngamkhanong, C., Kaewunruen, S., and Costa, B. J. A. (2018). State-of-the-art review of railway track resilience monitoring. Infrastructures 3:3. doi: 10.3390/infrastructures3010003
Nguyen, K., Villalmanzo, D. I., Goicolea, J. M., and Gabaldon, F. (2016). A computational procedure for prediction of ballasted track profile degradation under railway traffic loading. J. Rail Rap. Trans. 230, 1812–1827. doi: 10.1177/0954409715615374
Nielsen, J. C. O., and Li, X. (2018). Railway track geometry degradation due to differential settlement of ballast-subgrade – Numerical prediction by an iterative procedure. J. Sound Vibrat. 412, 441–456. doi: 10.1016/j.jsv.2017.10.005
Nimbalkar, S., Indraratna, B., and Biabani, M. M. (2016). “Deformation and degradation of railroad granular layers under high frequency cyclic loading and the benefits of using geosynthetics,” in Geo-Chicago 2016: Sustainable Materials and Resource Conservation. eds K. R. Reddy, N. Yesiller, D. Zekkos, A. Farid and A. De (Reston, US: American Society of Civil Engineers), 222-232. doi: 10.1061/9780784480151.023
Oberg, J., and Andersson, E. (2009). Determining the deterioration cost for railway tracks. J. Rail Rap. Trans. 223, 121–129. doi: 10.1243/09544097JRRT222
Osman, M. H. B., and Kaewuruen, S. (2018). Uncertainty propagation assessment in railway-track degradation model using bayes linear theory. J. Transpor. Engin. 144:04018026. doi: 10.1061/JTEPBS.0000146
Peralta, D., Bergmeir, C., Krone, M., and Galende, M. (2018). Multiobjective Optimization for Railway Maintenance Plans. J. Comput. Civil Engin. 32:04018014. doi: 10.1061/(ASCE)CP.1943-5487.0000757
Prescott, D., and Andrews, J. (2013). A track ballast maintenance and inspection model for a rail network. J. Rail Rap. Trans. 227, 251–266. doi: 10.1177/1748006X13482848
Prescott, D., and Andrews, J. (2015a). Investigating railway track asset management using a Markov analysis. J. Rail Rap. Trans. 229, 402–416. doi: 10.1177/0954409713511965
Prescott, D., and Andrews, J. (2015b). Modelling maintenance in railway infrastructure management, Proceedings Annual Reliability and Maintainability Symposium (RAMS). Florida, USA: RAMS.
Qian, J., Gu, J., Gu, X., Huang, M., and Mu, L. (2016). “DEM Analysis of Railtrack Ballast Degradation under Monotonic and Cyclic Loading,” in International Conference on Transportation Geotechnics, (Portugal: Guimaraes). doi: 10.1016/j.proeng.2016.06.137
Quiroga, L., and Schnieder, E. (2010). “Modelling high speed railroad geometry ageing as a discrete-continuous process,” in Stochastic Modeling Techniques and Data Analysis International Conference, (Chania: Greece).
Quiroga, L., and Schnieder, E. (2011a). Heuristic forecasting of geometry deterioration of high speed railway tracks. Spain: Springer.
Quiroga, L., and Schnieder, E. (2011b). Monte Carlo simulation of railway track geometry deterioration and restoration. J. Risk Reliab. 226, 274–282. doi: 10.1177/1748006X11418422
Rhayma, N., Bressolette, P. H., Breul, P., Fogli, M., and Saussine, G. (2013). Reliability analysis of maintenance operations for railway tracks. Reliab. Engin. Sys. Safe. 114, 12–25. doi: 10.1016/j.ress.2012.12.007
Sadeghi, J. (2010). Development of railway track geometry indexes based on statistical distribution of geometry data. J. Transport. Engin. 136, 693–700. doi: 10.1061/(ASCE)0733-947X(2010)136:8(693)
Sadeghi, J., and Askarinejad, H. (2007). Influences of track structure, geometry and traffic parameters on railway deterioration. Internat. J. Engin. 20, 291–300.
Sadeghi, J., and Askarinejad, H. (2009). An investigation into the effects of track structural conditions on railway track geometry deviations. J. Rail Rap. Trans. 223, 415–425. doi: 10.1243/09544097JRRT266
Sadeghi, J., and Askarinejad, H. (2010). Development of improved railway track degradation models. J. Struct. Infrastruct. Engin. 6, 675–688. doi: 10.1080/15732470801902436
Sadeghi, J., and Askarinejad, H. (2011). Development of track condition assessment model based on visual inspection. J. Struct. Infrastruct. Engin. 7, 895–905. doi: 10.1080/15732470903194676
Sadeghi, J., and Askarinejad, H. (2012). Application of neural networks in evaluation of railway track quality condition. J. Mechan. Sci. Technol. 26, 113–122. doi: 10.1007/s12206-011-1016-5
Sadeghi, J., Fathali, M., and Boloukian, N. (2009). Development of a new track geometry assessment technique incorporating rail cant factor. J. Rail Rap. Trans. 223, 255–263. doi: 10.1243/09544097JRRT237
Sadri, M., Lu, T., Zoeteman, A., and Steenbergen, M. (2018). “Railway track design & degradation,” in International Conference on Vibration Engineering and Technology of Machinery, (Lisbon: Portugal). doi: 10.1051/matecconf/201821111006
Sadri, M., and Steenbergen, M. (2018). Effects of railway track design on the expected degradation parametric study on energy dissipation. J. Sound Vibrat. 419, 281–301. doi: 10.1016/j.jsv.2018.01.029
Sato, Y. (1995). Japanese studies on deterioration of ballasted track. Vehicle Sys. Dynam. 24, 197–208. doi: 10.1080/00423119508969625
Selig, E. T., and Waters, J. M. (1994). Track Geotecnology and Substructure Management. UK: Thomas Telford. doi: 10.1680/tgasm.20139
Shafahi, Y., Masoudi, P., and Hakhamaneshi, R. (2008). Track D Degradation Prediction Models, Using Markov Chain, Artificial Neural and Neuro-Fuzzy Network. Tehran, Iran: Sharif University of Technology.
Shafahi, Y., and Hakhamaneshi, R. (2009). Application of a maintenance management model for Iranian railways based on the Markov chain and Probabilistic Dynamic Programming. Sci. Iranica 1, 87–97.
Shafiee, M., Patriksson, M., and Chukova, S. (2016). An optimal age–usage maintenance strategy containing a failure penalty for application to railway tracks. J. Rail Rap. Trans. 230, 407–417. doi: 10.1177/0954409714543337
Simson, S., Ferreira, L., and Murray, M. (1999). “Modeling rail track maintenance planning using simulation,” in The Australian Society for Operations Research Inc. 15 National Conference, (Australia: Brisbane).
Soleimanmeigouni, I., Ahmadi, A., and Kumar, U. (2016). Current Trends in Reliability, Availability, Maintainability and Safety: an Industry Perspective. USA: Springer.
Soleimanmeigouni, I., Ahmadi, A., and Kumar, U. (2018). Track geometry degradation and maintenance modelling: a review. J. Rail Rap. Trans. 232, 73–102. doi: 10.1177/0954409716657849
Tolppanen, P., Stephansson, O., and Stenlid, L. (2002). 3D Degradation Analysis of Railroad Ballast. Bull. Engin. Geolo. Environ. 61, 35–42. doi: 10.1007/s100640100140
Torres-Carrion, P. V., Gonzalez-Gonzalez, C. S., Aciar, S., and Rodríguez-Morales, G. (2018). “Methodology for systematic literature review applied to engineering and education,” in Global Engineering Education Conference, Santa Cruz de Tenerife, (Spain: IEEE). doi: 10.1109/EDUCON.2018.8363388
Tzanakakis, K. (2013). The Railway Track and Its Long Term Behaviour. USA: Springer. doi: 10.1007/978-3-642-36051-0
Vale, C., and Calçada, R. (2011). Tools for evaluating and predicting track geometrical quality evolution over time. France: World Congress on Railway Research.
Vale, C., and Lurdes, S. M. (2013). Stochastic model for the geometrical rail track degradation process in the portuguese railway northern line. Reliab. Engin. Sys. Safe. 116, 91–98. doi: 10.1016/j.ress.2013.02.010
Vale, C., and Ribeiro, I. M. (2014). Railway condition-base maintenance model with stochastic deterioration. J. Civil Engin. Manag. 20, 686–692. doi: 10.3846/13923730.2013.802711
Wei, X., and Liu, F. (2014). “Track quality prediction based on center approach Markov-Grey,” in International Conference on Information and Automation, (China: Hailar).
Wei, Z., Núñez, A., Li, Z., and Dollevoet, R. (2017). Evaluating degradation at railway crossings using axle box acceleration measurements, Sensors 17:2236. doi: 10.3390/s17102236
Westgeest, F. P., Dekker, R., and Fischer, R. H. (2012). Predicting rail geometry deterioration by regression models. Adv. Safe. Reliab. Risk Manag. 2012, 926–933. doi: 10.1201/b11433-130
Xin, T., Famurewa, S. M., Gao, L., Kumar, U., and Zhang, Q. (2016). Grey-system-theory-based model for the prediction of track geometry quality. J. Rail Rap. Trans. 230, 1735–1744. doi: 10.1177/0954409715610603
Xu, P., Liu, R., Wang, F., and Sun, Q. (2011). A novel description method for track irregularity evolution. Int. J. Comput. Intell. Sys. 4, 1358–1366. doi: 10.1080/18756891.2011.9727886
Xu, P., Liu, R., Wang, F., Wang, F., and Sun, Q. (2013). Railroad track deterioration characteristics based track measurement data mining. Mathemat. Probl. Engin. 2013:970573. doi: 10.1155/2013/970573
Yan, Y., Li, T., Liu, J., Wang, W., and Su, Q. (2019). Monitoring and early warning method for rockfall a along railways based on vibration signal characteristics. Sci. Rep. 9:6606. doi: 10.1038/s41598-019-43146-1
Yousefikia, M., Moridpour, S., Setunge, S., and Mazloumi, E. (2014). Modeling degradation of tracks for maintenance planning on a tram line. J. Traffic Logistics Engin. 2, 86–91. doi: 10.12720/jtle.2.2.86-91
Zarembski, A. M., Einbinder, D., and Attoh-Okine, N. (2016). Using multiple adaptive regression to address the impact of track geometry on development of rail defects. Construct. Build. Mater. 127, 546–555. doi: 10.1016/j.conbuildmat.2016.10.012
Zhang, Y. J., Murray, M., and Ferreira, L. (1997). Track degradation predication: criteria, methodology and models. Australia: Australasian Transport Research Forum.
Zhang, Y. J., Murray, M. H., and Ferreira, L. (2000). Modelling rail track performance: an integrated approach. Transport 141, 187–194. doi: 10.1680/tran.2000.141.4.187
Zhu, M., Cheng, X., Miao, L., Sun, X., and Wang, S. (2015). Advanced stochastic modeling of railway track irregularities∗. Advances in Mechanical Engineering 2013:401637. doi: 10.1155/2013/401637
Keywords: railway track, permanent way, track components, track geometry, track deterioration, track degradation, track monitoring, systematic literature review
Citation: de Melo ALO, Kaewunruen S, Papaelias M, Bernucci LLB and Motta R (2020) Methods to Monitor and Evaluate the Deterioration of Track and Its Components in a Railway In-Service: A Systemic Review. Front. Built Environ. 6:118. doi: 10.3389/fbuil.2020.00118
Received: 21 April 2020; Accepted: 26 June 2020;
Published: 10 September 2020.
Edited by:
Sanjay Shrawan Nimbalkar, University of Technology Sydney, AustraliaReviewed by:
Marco Guerrieri, University of Trento, ItalyPatric Mak, Network Rail, United Kingdom
David Robert Michael Milne, University of Southampton, United Kingdom
Copyright © 2020 de Melo, Kaewunruen, Papaelias, Bernucci and Motta. This is an open-access article distributed under the terms of the Creative Commons Attribution License (CC BY). The use, distribution or reproduction in other forums is permitted, provided the original author(s) and the copyright owner(s) are credited and that the original publication in this journal is cited, in accordance with accepted academic practice. No use, distribution or reproduction is permitted which does not comply with these terms.
*Correspondence: Andre L. O. de Melo, YW5kcmVsdWlzbWVsb0B1b2wuY29tLmJy; Sakdirat Kaewunruen, cy5rYWV3dW5ydWVuQGJoYW0uYWMudWs=; c2FrZGlyYXRAZ21haWwuY29t