- 1Sonny Astani Deptartment of Civil and Environmental Engineering, Viterbi School of Engineering, University of Southern California, Los Angeles, CA, United States
- 2Arup, London, United Kingdom
- 3Chan Division of Occupational Science and Occupational Therapy, University of Southern California, Los Angeles, CA, United States
- 4Institute for Creative Technologies, University of Southern California, Los Angeles, CA, United States
People spend most of their day in buildings, and a large portion of the energy in buildings is used to control the indoor environment for creating acceptable conditions for occupants. However, the majority of the building systems are controlled based on a “one size fits all” scheme which cannot account for individual occupant preferences. This leads to discomfort, low satisfaction and negative impacts on occupants' productivity, health and well-being. In this paper, we describe our vision of how recent advances in Internet of Things (IoT) and machine learning can be used to add intelligence to an office desk to personalize the environment around the user. The smart desk can learn individual user preferences for the indoor environment, personalize the environment based on user preferences and act as an intelligent support system for improving user comfort, health and productivity. We briefly describe the recent advances made in different domains that can be leveraged to enhance occupant experience in buildings and describe the overall framework for the smart desk. We conclude the paper with a discussion of possible avenues for further research.
Introduction
The office workplace has evolved over the past decades due to multiple factors, including changes in the nature of work, digitization, integration of ergonomics in space design, cost of building and maintaining office spaces, and the drive to improve employee productivity. For example, workspaces evolved from open floor factories in the 1920s to personal cubicles in the 1980s to more flexible and collaborative spaces in the twenty-first century. At the core of the workspace evolution is the design and layout of the office workstation (desk, chair and partitioning furniture), which has also changed over the years from supporting paperwork to supporting personal computers. With the recent advancements in Human–Computer Interaction (HCI), the office desk continues to evolve into an interactive device, supporting multiple inputs, such as touch, hand gestures, and voice commands, leading to faster information retrieval, reduced paperwork and enhanced collaboration (Arai et al., 1995; Coen, 1998; Mutlu et al., 2007; Wimmer et al., 2010; Gebhardt et al., 2014). Furthermore, efforts in injury prevention and health promotion have led to the development of ergonomic advances, such as sit-stand desks, adjustable chairs and walking and cycling workstations (Helander et al., 1987; Straker et al., 2009; Robertson et al., 2013). Although these developments help to improve worker productivity and health, there are other emerging novel approaches that support further improvements in the office workspace by focusing on the indoor environmental quality. In this paper, we present our vision for improving comfort, health and productivity by leveraging recent advancements in sensing technologies and Internet of Things (IoT) to create a smart desk for monitoring, controlling and personalizing the local indoor office environment.
We envision future workspaces that provide personalized experiences for each user and promote office worker productivity, health and well-being while also reducing overall energy consumption through direct interactions with their users to learn comfort requirements. Since the desk is an important part of any office space, where workers spend most of their time, we envision the desk acting as a point of contact between building systems and office users. By leveraging the current advancements in IoT, we believe a smart desk equipped with a variety of sensors and actuators can act as an agent for monitoring and controlling the local indoor environment. The field of IoT has seen enormous growth in recent years and is predicted to reach 24 billion devices by 2020, with smart buildings being a primary application domain (Gubbi et al., 2013). Potential applications of IoT in buildings include real time performance monitoring, fault diagnosis of equipment, data visualization, optimization of indoor environment for comfort and energy consumption, demand response and predictive controls (Gunay and Shen, 2017). To support these applications, smart desks could be used as distributed sensor networks throughout an office workplace to provide relevant sensor data. By collecting granular data in proximity to building occupants, the desks can enhance existing Building Management Systems (BMS) and Building Automation Systems (BAS), which currently only monitor the environment at the room or zone level. Moreover, smart desks provide a unique opportunity to improve the indoor environmental quality in buildings that do not have a BMS.
There is a large energy cost associated with maintaining comfortable indoor environments. Buildings consume about 40% of all energy in the U.S., U.K., and the EU, where more than half of the energy is used to maintain indoor thermal and lighting environments (Pérez-Lombard et al., 2008; US Department of Energy., 2015). The main focus of BMS or BAS has been to automate building operations to lower energy costs while maintaining adequate indoor conditions. However, post-occupancy evaluations have shown that about 43% of occupants are dissatisfied with their thermal environment (Karmann et al., 2018), and only about 11% of buildings met the ASHRAE 55 requirements of satisfying at least 80% of the occupants (Huizenga et al., 2006). Furthermore, there is very little difference in occupant satisfaction between green-certified buildings and conventional buildings (Paul and Taylor, 2008; Altomonte et al., 2017). This fact suggests that although centralized control of indoor environments may lower energy consumption, it leads to low occupant satisfaction (Hellwig and Boerstra, 2017). Current standards and guidelines for determining comfortable ranges for different indoor parameters are based on average responses from participants across various studies (Kim et al., 2018). However, individual occupant preferences can vary significantly from these average responses, leading to a mismatch between occupant preferences and indoor conditions (Kim et al., 2018). Considering individual preferences within the control loop of centralized HVAC systems can improve average satisfaction by 25% compared to the existing control methods; however, it is quite difficult to meet the ASHRAE 55 requirement of 80% satisfaction by using centralized systems alone (Aryal and Becerik-Gerber, 2018).
Completely automated control systems that take away the occupant's control over the environment lead to lower satisfaction (Vischer, 2007). Although a clear solution to improve comfort and satisfaction would be to provide local control (e.g., light switches, controllers for blinds), it is not clear that mere ability to control the environment will fully alleviate the problem. In fact, inaccessibility, poor location and negative user acceptability of the environmental controls can lead to further dissatisfaction (Day and O'Brien, 2017). Furthermore, occupants often use these controls in inefficient ways, such as leaving the systems on even when it is not needed, taking the easiest and quickest option rather than the best option for their health and environment, over-compensating for relatively minor annoyances, taking actions only after an event prompts them to do so or when they reach a very uncomfortable state (Leaman, 1999; Cole and Brown, 2009). Complete manual control of the environment given to occupants might also compromise efficiency because not all occupants make the best choices (Cole and Brown, 2009); for example leaving windows open can compromise security. A smart desk could provide the necessary behavioral support to occupants to use the building systems in more efficient ways.
In addition to efficiency in environmental management, local sensing and control through a smart desk can promote healthier workplaces and increased worker productivity. Indoor environmental quality (IEQ) parameters, such as air quality, ventilation, thermal environment, lighting and acoustics are associated with comfort, productivity, creativity, physiological and psychological health and well-being of building occupants (De Croon et al., 2005; Clausen and Wyon, 2008; Wong et al., 2009; Turunen et al., 2014; Allen et al., 2015; Cedeño-Laurent et al., 2018). The majority of the population in the U.S. spends about 90% of the time in enclosed buildings (Klepeis et al., 2001; Allen et al., 2015), and there are more than 81 million professional office workers in the U.S. (Department for Professional Employees., 2015) who spend most of their time working at a desk with little control over the thermal and lighting conditions. Unsuitable indoor conditions, in addition to low satisfaction with the environment, can lead to reduced job satisfaction and be a source of stress at the workplace (Vischer, 2007), which can lead to presenteeism or absenteeism (Vischer, 2007; Brager et al., 2015). Personalized Comfort Systems (PCS), which create a microclimate around the occupant, have the potential to provide comfortable conditions while reducing overall energy consumption (Amai et al., 2007; Veselý and Zeiler, 2014; Brager et al., 2015; Zhang et al., 2015). Furthermore, it has been estimated that potential productivity increases in the range of 0.5% to 5% are possible by improving the thermal and lighting conditions which translates to an annual productivity increase of $19 billion to $190 billion in the U.S. alone (Fisk, 2000). Several PCS devices have been studied in the past, such as a personal fan and foot warmers (Zhang et al., 2010), heater heated/cooled chair (Pasut et al., 2015) and radiant panels on the desk (He et al., 2017), where improvement in occupant comfort was observed due to the use of PCS. Prior studies focused on the improvement in occupant satisfaction using PCS, but relied on the users to manually control the devices. An intelligent system could reduce the burden of manual control and alleviate the inefficiencies that could result from manual control.
Apart from the unsatisfactory environmental conditions, office workers also spend ~11.6 hours per day in sedentary activities (Tudor-Locke et al., 2011), a number which includes 80% of their time spent working (Parry and Straker, 2013). Sedentary time leads to increased obesity, musculoskeletal disorders, cardiovascular disease, diabetes and other chronic conditions (Chau et al., 2013; Chu et al., 2016). There is emerging evidence that use of sit-stand desks can reduce sedentary time, while also improving worker satisfaction (Carr et al., 2016). At the same time, prolonged standing can lead to negative outcomes, such as lower back and leg pain, fatigue and discomfort (Waters and Dick, 2015). A structured sit-stand paradigm is necessary to reap the benefits of reduced sedentary time and avoid drawbacks of prolonged standing (Karakolis and Callaghan, 2014; Karol and Robertson, 2015). An intelligent support system, such as a smart desk, that automatically cues the user into proper use of sit-stand regimen could improve overall health of office workers.
Although an intelligent, user-centered automated system offers numerous benefits, determining the acceptable and preferred levels of automation is a foundational issue in the development of this solution (Ahmadi-Karvigh et al., 2017). Various sensors on the desk can enable it to be aware of the state of the environment around the user as well as the user's overall comfort and posture. This intelligent desk can then consider the degree of automation preferred by the user to use available controls to either make recommendations to the user or automatically adjust the local environment. If user preferences are not met, leading a user to override the automated controls, the desk can further utilize this feedback to adapt to the user for making future adjustments. Through this iterative and symbiotic learning process between the user and the desk, we believe that the intelligent desk can provide solutions to the issues of indoor working environments. Enabling occupants to control their environments with the support of an intelligent system might lead to positive behavioral changes, improving building efficiency and worker satisfaction, health and productivity.
Overall Smart Desk Framework
The overall framework of the smart desk consists of different application modules for enhancing various aspects of occupant comfort, well-being and productivity by providing recommended conditions for thermal comfort, visual comfort, sit-stand and posture. Each of the application modules consists of sensing, data analysis and actuation components. The sensing and actuation components rely on IoT devices, and the data analysis component leverages machine learning algorithms to model individual preferences. Each of the modules are independent from one another and can be implemented as needed. In this section, we briefly review some of the relevant literature and describe different smart desk modules that can enhance occupants' experience in office buildings. Furthermore, we identify previously developed methods that can be integrated into different modules with little or no modification and highlight modules where new methods need to be developed specifically for the desk because previous methods are not adequate. Some of the modules of the desk are categorized as supporting modules, and others, which are specific to certain applications, are categorized as application modules. Figure 1 shows the overall framework of the desk, where green color indicates components that have been implemented in a prototype. The prototype is shown in Figure 2 for illustration and is discussed in another publication (Aryal et al., 2018).
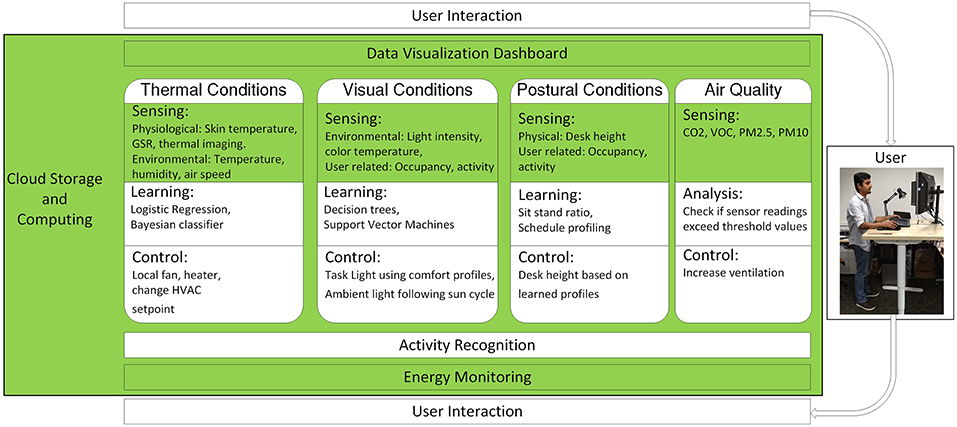
Figure 1. Overall Framework of the desk. Green color represents components already implemented in the prototype.
User Interaction
The user interaction module is essential for all aspects of the smart desk and is one of the supporting modules for other application modules that are focused on a single aspect of the indoor environment. The desk should support interactions with its user and gather feedback to learn his/her preferences. For instance, several studies have utilized smartphone-based interfaces to promote physical activity (Bort-Roig et al., 2014). There are several avenues for user interaction with the desk, from simple switches for controlling lights and other PCS, to more modern interfaces, such as gesture recognition or speech. Due to their ubiquitous nature, previous studies in the domain of human–building interaction (HBI) have utilized mobile phone and web interfaces to gather user feedback to learn their preferences (Erickson and Cerpa, 2012; Jazizadeh and Becerik-Gerber, 2012). In the field of human–computer interaction, several interfaces based on visual information, natural language, gesture, touch or haptic feedback have been developed in recent years to make the interaction more natural for the user (Mullet and Sano, 1995; Loper and Bird, 2002; Jaimes and Sebe, 2007). There is a potential to integrate more natural interaction interfaces into the desk. For instance, researchers have developed methods for tracking hand motions and gestures for an augmented desk surface using vision-based techniques, which can be implemented with low cost cameras (Sato et al., 2004). Other means, such as interaction via voice, may not be appropriate in shared office spaces as it can cause distraction to other occupants in the same room.
In addition to the physical interface for user interaction, the psychological aspects of interaction between the user and the desk are also important. We envision a bi-directional communication between the occupant and the desk where the occupant can indicate his/her preferences, and the desk can provide suggestions for the user to change their behavior. Past studies have explored different social influence methods for communication between a building agent and its occupants to increase compliance (Khashe et al., 2015). For instance, the study found that methods that apply the principal of reciprocity (i.e., the agent does something for the user first, and requests the user to take an action), or foot in the door (i.e., the agent makes a small request first, and then makes a bigger request to the user after the user complies with the smaller request) have a higher rate of user compliance compared to making a direct request (Khashe et al., 2015). Several studies have explored the intricacies of interaction between humans and robotic agents. For instance, personality traits of the user, their expectations from the agent and the user's perception of the agent's personality are important considerations for the development of better collaboration between virtual agents and the user (Dautenhahn, 2007). In addition to the direct messages or commands between the user and the desk, the interaction between the desk and the user also occurs via automated control of appliances. Depending on occupant's preferences toward automation, appliances can be automated to turn off when not in use. A study that explored automation preferences of occupants and developed algorithms to automate appliances based on these preferences (Ahmadi-Karvigh et al., 2018) is suitable for integrating into the desk.
Communication can also occur via the user interaction module by providing a dashboard with feedback about the impact of an occupant's choices on energy consumption, productivity and health. Several dashboards have been developed to promote energy efficient behavior in building occupants (Yun et al., 2013; Yun, 2014). In general, eco-feedback systems, which provide occupants with information regarding their current and historical energy consumption, lead to reduction in energy consumption (Paone et al., 2018). Furthermore, studies have found that adding social interaction (by providing comparisons of energy usage against other occupants or neighbors) and adding advice and tips on a dashboard can improve the effectiveness of an eco-feedback system and achieve greater energy savings (Cuddy et al., 2010; Yun, 2014; Paone et al., 2018). Furthermore, gamification of the feedback system by introducing game rewards and incentives can motivate change in occupant behavior (Cuddy et al., 2010). Due to their ubiquitous nature, mobile phones can be a suitable channel of communication between the user and the desk. Using a web interface on the occupant's computer or having an embedded screen on the desk are other options.
Another important aspect of the user interaction module is user identification, which provides information about the person who is using the desk to be able to personalize their experience across settings and desks. Due to increasing costs of providing office spaces and advances in technology that enable work to be performed outside of the office, there is an increasing tendency in the number of office workers working remotely (Felstead et al., 2005). There is also an increasing trend in the use of open plan offices and hot desking (i.e., workers do not have a fixed personal workspace and use any available desk as needed) (Felstead et al., 2005). In the context of a smart desk that learns and adapts to user preferences, it becomes important to identify the user because the desk they use may change. Several options exist for user identification, from simple password protected login using the user interface to more convenient methods, such as RFID (Radio Frequency IDentification) tags, fingerprint, face detection, NFC (Near Field Communication) authentication using cellphones, etc. (Braz and Robert, 2006); either of the user identification methods can be integrated into the desk depending on the level of security required and ease of authentication for the user.
Ultimately, the goal of user interaction is to increase acceptance of the smart desk's suggestions for thermal and lighting conditions and sit-stand regimen and posture. Research has shown that users accept machines' suggestions based on the extent to which they understand and agree with why the automation is operating (benevolence), how well the automation is operating (competence) and how the automation operates (integrity). As examples of benevolence, competence and integrity, understanding system goals are aligned with user goals helps users to accept the systems suggestions, people accept suggestions more when it is portrayed as a reputable or “expert” system and “explainable AI” increases acceptance by giving insight to users about how the AI made its decisions, respectively (Lerch et al., 1997; De Vries and Midden, 2008; Spain and Madhavan, 2009; Pak et al., 2012). The chosen interface—whether based on visual information, natural language, gesture, touch or haptic feedback—will follow these principles as closely as possible to encourage acceptance of the smart desk's suggestions regarding thermal and lighting conditions and sit-stand regimen. Furthermore, the smart desk would also go beyond traditional work in this area. Our novel recent work shows that imbuing machines with relational features can increase acceptance of a system's suggestions in an office context (Khashe et al., 2017, 2018a,b). To the extent possible based on the interface, the smart desk will also use relational features to increase acceptance. However, this prior work did not consider how to increase acceptance in automation beyond getting approval of users for a single automation request. The smart desk builds on this work to facilitate increasing levels of automation over time.
Activity Recognition
For the desk to be effective, it is important for it to be aware of the activities that the user is engaged in. A user's preferences for thermal and lighting conditions and sit-stand regimen could be highly influenced by the activity of the occupant. Different activities performed at the desk could be identified using a combination of occupancy sensors, use of electric appliances and ambient conditions. For example, if the user is engaged in focused work on their computer, the energy monitoring could identify if the computer is currently under use, and ambient sensors could identify that the noise levels are low. On the other hand, if the user is engaged in collaborative work with other occupants, the ambient noise levels could be used to identify conversations (Nguyen et al., 2012). In addition, monitoring of occupancy is crucial to identify if the user is at the desk or not and can guide the operation of other services. For instance, the lighting systems can be turned off if the user is away from the desk.
Past studies have developed several methods for activity recognition using a multitude of sensors including video, audio, computer interactions, Wi-Fi signals, wearable sensors, 3D depth cameras, energy plug meters and many more (Maurer et al., 2006; Wojek et al., 2006; Nguyen et al., 2012; Gaglio et al., 2015; Wang et al., 2015; Ahmadi-Karvigh et al., 2018). For instance, Karvigh et al. used power meters to identify different activities of occupants, such as working with a computer, working without a computer, etc. (Ahmadi-Karvigh et al., 2018). Laput et al. used a combination of sound, vibration and other sensors to identify the appliances currently being used as a proxy for the activity that the occupant was engaged in Laput et al. (2017). After identifying the activity of the occupant, the indoor environmental conditions can be controlled to suit the occupant's activities. Activity recognition plays a crucial part in automated control of different indoor conditions as described in the following sections. The sensors for detecting activities include power meters for appliances, occupancy sensors and ambient noise sensors. The algorithm previously developed by Ahmadi-Karvigh et al. (2018) can be used as a starting point for the desk because the original algorithm was developed specifically for activity recognition in office environments and focused on appliances typically found in office desks. However, since the algorithm mostly focuses on appliances' energy consumption data and is only able to identify a limited number of activities, it needs to be improved to identify more activities typically performed in an office environment.
Thermal Conditions
Recent advancements in sensing technologies have improved BAS and BMS through which indoor environments can be monitored at a zone level, enabling more efficient control of the building's HVAC and lighting systems. However, the zone level operation of HVAC systems is unable to meet the comfort requirements of most of the occupants due to variations in occupant preferences (Huizenga et al., 2006; Aryal and Becerik-Gerber, 2018). HVAC systems in buildings are operated based on the PMV (Predicted Mean Vote) model, developed by Fanger (1970), which specifies a narrow range of comfort conditions developed based on a heat transfer model from experiments with large numbers of participants (Hoyt et al., 2009; Roussac et al., 2011). Naturally ventilated buildings utilize the adaptive comfort model, which considers different ways occupants can adapt themselves or modify the environment to maintain comfort (De Dear et al., 1998). ASHRAE 55, the current standard upon which buildings are designed and operated, allows the use of the adaptive model for naturally ventilated buildings and recommends the use of the PMV model for all other types of buildings (ASHRAE., 2017). However, a study has shown that only 11% of the buildings meet the ASHRAE requirement of satisfying 80% or more occupants (Huizenga et al., 2006). Uncomfortable thermal conditions have been linked to lower productivity in office environments (Seppanen and Fisk, 2006). Furthermore, long term exposure to uniform sustained thermal conditions has been linked to negative health impacts, such as reduced metabolism and increased risk of cardiovascular diseases (van Marken Lichtenbelt et al., 2017). Using Personalized Control Systems (PCS) and providing direct control to the occupant has been shown to improve comfort and satisfaction (Zhang et al., 2015). Several such systems have been developed, such as heated and cooled chairs, hand warmers, foot warmers, personal air terminals, local fans and heaters, etc. (Zhai et al., 2013; Luo et al., 2018).
Thermal comfort of occupants depends on several factors, such as the air temperature, radiant temperature, humidity, air movement, metabolic rate and clothing levels (Fanger, 1970). In office settings, the metabolic rate is fairly constant, and clothing levels are also constant for each season. The other variables (i.e., air temperature, humidity, radiant temperature, and air movement) can vary based on daily weather, location of the occupant in a space, and operation of HVAC systems. In air-conditioned buildings, air movement is fairly constant, therefore it is not very important to monitor air speeds, but for naturally ventilated buildings, air speeds can vary depending on outdoor air speed. Although monitoring of air speeds is important, it is challenging because there is a high variation in air movement around the office desk, chairs and other furniture (Gao et al., 2017). However, as better methods to monitor air speeds are developed in the future, it should be one of the parameters that is monitored. In buildings with radiant heating systems, monitoring of radiant temperatures is important. However, for buildings without radiant heating systems, connecting to a model of sun movement and weather forecast might be sufficient. In addition, relative humidity has a small impact on thermal comfort in the range that is found in most indoor environments (Toftum et al., 1998), and might not be important to monitor in air-conditioned buildings where humidification/dehumidification is performed.
Several new methods have been developed to model personal thermal comfort preferences of occupants in recent years (Kim et al., 2018). The general procedure consists of using sensor data combined with periodic user feedback to build a comfort profile that indicates the environmental settings where the occupant is likely to be comfortable or uncomfortable. Once a preference profile for the user is learned, it can be used to control the HVAC systems or local PCS to improve user comfort. The user needs to provide frequent feedback until the system learns their preferences, but over time the system can automatically control the environment based on the learned comfort profiles without much feedback from the occupant. As the occupant's preferences change over time, the system can update the comfort profiles based on new feedback from the occupant. Several algorithms have been utilized in recent years to learn the thermal comfort preferences of occupants, such as logistic regression, decision trees, Bayesian networks, neural networks, etc. (Kim et al., 2018). Furthermore, emerging technologies, such as wearable devices and thermal imaging, could be used to learn comfort preferences more accurately (Burzo et al., 2014; Ghahramani et al., 2016; Li et al., 2018). Several ways to control the thermal environment based on occupant feedback have been developed, with some controlling the central HVAC system, such as comfy (www.comfyapp.com) and Thermovote (Erickson and Cerpa, 2012). Several PCS, such as heated chairs, personal air terminals, hand warmers, local cooling fans, etc., have been studied in recent years and can be used to control the local thermal environment around the user to improve their comfort and satisfaction (Zhang et al., 2007, 2010; Zhai et al., 2013). Although previous studies provide a good starting point to introduce local comfort systems as part of the desk (Zhang et al., 2007; Zhao et al., 2014), several improvements in learning algorithms are necessary to meet the vision of truly personalized environments. Current learning algorithms require a large amount of user feedback, and usually only consider the room temperature while learning personal comfort preferences. Furthermore, the learning algorithms have mostly focused on learning whole body thermal sensations in air-conditioned environments. New algorithms that consider the effects of local heating and cooling systems on user thermal comfort need to be developed for successful personalization of thermal comfort at the desk.
Visual Conditions
Lighting is another IEQ (indoor environmental quality) variable that is highly related to occupant comfort and productivity. Improper lighting can cause problems with visual acuity, cause distraction from the task, and lead to eyestrain (Boyce, 2010). Too much or too little light, variation in illuminance between work surface, glare, etc. can cause visual discomfort that leads to eye strain (Boyce, 2014). In addition to the direct impact on eye strain, lighting parameters also affect the posture of the user depending on the task (Rea et al., 1985). For instance, the user might hunch or move closer to the task while trying to read text that is affected by glare, reflection or poor contrast (Joines et al., 2015). Proper task lighting can help mitigate these negative effects on the posture, eye strain and visual comfort of the user (Newsham et al., 2005; Joines et al., 2015). Furthermore, studies have linked exposure to blue light during night to disruption in the circadian rhythm and increased risk of breast and colorectal cancer (Stevens, 2009; James et al., 2016). Exposure to bright white light during the day, and non-blue light at night can help reduce the impact on the natural circadian rhythm (Pauley, 2004). The circadian rhythm is also responsible for the regulation of core body temperature and can indirectly impact thermal comfort of the user (Pauley, 2004). Use of variable lighting conditions in offices could potentially improve mood (Zhang et al., 2015) and occupant satisfaction (de Kort and Smolders, 2010).
Lighting quality is commonly measured using light intensity (lux) and correlated color temperature (CCT) (Oi and Takahashi, 2007). In addition, a model of the daylight cycle can be used as an additional input to provide information about the variation of natural lighting in the location where the module is being implemented. Lighting standards define the typical range of lux and CCT values required in different environments depending on the primary task that is performed in that environment. However, studies have shown that the actual preferences of users can vary from those defined in the standards (Oi and Takahashi, 2007). Furthermore, lighting also has an impact on the human circadian rhythm and can have negative impacts on sleep, leading to reduced productivity and satisfaction. Although light settings—which are high in light intensity and CCT—can improve task-specific productivity in the short term (Badia et al., 1991), it can impact the circadian rhythm and could have a long term negative impact on the occupant's health (Pauley, 2004; Boyce, 2014). It is crucial to understand the positive and negative aspects of the lighting environment and find a balanced setting to promote productivity while reducing the negative impacts.
Several methods have been developed to learn lighting preferences of occupants. The methods primarily rely on the measurements from lighting sensors, user feedback and use of control options where available (Oi and Takahashi, 2007; Despenic et al., 2017; Sadeghi et al., 2018), using algorithms, such as k-means clustering (Despenic et al., 2017) and Bayesian classification (Oi and Takahashi, 2007; Sadeghi et al., 2018) to learn user preferences. Task-specific lighting can be used to promote productivity in the short term (Juslén et al., 2007), and lighting that is close to the natural light cycle can be used to reduce the negative impacts on the circadian rhythm (Pauley, 2004; Rea and Figueiro, 2018). Previous methods, such as the ones developed by Despenic et al. or Sadeghi et al. provide a good starting point for learning individual preferences of the user. However, these methods do not consider changing preferences of the user depending on the tasks that they are engaged in. For the desk to understand the tasks that the user is engaged in, methods for activity recognition, such as the ones described in section Activity Recognition are needed. New algorithms that can integrate user activity into lighting comfort profiles need to be developed. Modern lighting fixtures based on LEDs enable changes in both lux and CCT values (Hye Oh et al., 2014). Control algorithms should be able to recognize the task that the occupant is engaged in and provide suitable lighting settings. Furthermore, control algorithms should vary the ambient lighting in the room while taking the sun cycle into consideration to reduce disruption to the circadian rhythm.
Glare usually arises when direct sunlight enters the room and causes visual discomfort when it shines into the eyes of the occupant or reflects off of their surrounding surfaces (Osterhaus, 2005). Although daylight is typically considered good for occupants' health and wellbeing, occupants take corrective actions to eliminate daylight if it causes discomforting glare (Boyce et al., 2003). Glare is one of the major issues in lighting and daylighting design (Clear, 2013; Boyce, 2014) and it is typically avoided by controlling the blinds or other shading devices on the building façade (Haldi and Robinson, 2010). Since multiple occupants in the same room can be affected by glare, the elimination of glare needs to be achieved by controlling shading devices at a room level rather than a desk level. Although the glare control is beyond the scope of the desk, several methods have been developed to assess glare (Fisekis et al., 2003; Nazzal, 2005; Kim et al., 2009; Borisuit et al., 2010) and to control shading devices to reduce glare discomfort (Osterhaus, 2005; Ochoa et al., 2012; Chan and Tzempelikos, 2013; Gunay et al., 2017), which can be integrated into the BMS.
Postural Conditions and Sit-Stand Regimen
Proper use of sit-stand regimen can reduce sedentary time and improve productivity while reducing the negative health effects of prolonged sitting or prolonged standing. It is recommended to switch between sitting and standing positions at 30-min intervals (Thorp et al., 2014). The initial preferences of users might be more biased toward sitting or standing. Recent literature in ergonomics suggests the standing time of occupants should be gradually increased to gradually habituate the occupant to increased standing time (Thorp et al., 2014), and without proper intervention, it is difficult to promote long-term change in behavior (Laestadius et al., 2009; Thorp et al., 2014). The feedback system of the desk can cue the user to switch between sitting and standing positions. The data from the sensors that monitor the desk height can be used to analyze typical sitting and standing durations to create a sit-stand profile for the occupant. The user interaction module can cue the user to increase or decrease the standing durations depending on their sit-stand profiles. Since undesirable changes in desk height can create a nuisance to the occupant, it might be better to prompt the user regarding the change and wait for the occupant to change their position instead of automatically controlling the desk height.
In addition to prolonged sitting, office workers are also at risk of developing musculoskeletal disorders due to improper posture while working at their computers (Fogleman and Lewis, 2002). Several studies indicate that providing postural feedback to users helps to improve posture and can help alleviate musculoskeletal discomfort (Vedsted et al., 2011; Park and Yoo, 2012). Several methods have been developed for posture recognition using techniques, such as Electromyography (EMG) (Park and Yoo, 2012), inertial sensors (Alahakone and Senanayake, 2010), pressure sensors, 3-D depth cameras (Alahakone and Senanayake, 2010) and computer vision-based methods (Moeslund and Granum, 2001). The desk can be equipped with sensors to monitor the posture of the user and provide appropriate feedback to correct the posture if needed. The methods that rely on 3-D depth cameras, computer vision, and pressure sensors are less intrusive to the user and have shown promising results. However, they can only provide information from the section of the human body that is captured by the sensors, typically the upper body from cameras and back and torso from pressure sensors. A combination of these methods is necessary to capture postural information from different parts of the body. Although several methods for identifying posture have been developed, methods to learn individual postural habits and provide tailored ergonomic feedback need to be developed.
Air Quality
Poor air quality is associated with reduced work performance and prevalence of Sick Building Syndrome (SBS). Dissatisfaction with air quality can arise after prolonged exposure to harmful levels of air pollutants. Carbon dioxide is a good proxy for indoor air quality because it can provide an indication of air exchange rate (Chatzidiakou et al., 2015). Additional indicators of indoor air quality, such as VOCs, PM2.5, etc., can be utilized. The World Health Organization (WHO) provides guidelines for safe levels of exposure to different air quality parameters (WHO., 2010). Furthermore, the Occupational Safety and Health Administration (OSHA) provides guidelines regarding indoor air quality in commercial and institutional buildings in the U.S. (OSHA., 2015). The air quality parameters can be monitored using appropriate sensors to measure CO2, VOC, PM10, PM2.5, etc. Measurement of CO2, respiratory suspended particles (such as PM10 and PM2.5), and VOCs can also be an effective way to predict other indoor pollutants, such as carbon monoxide, nitrogen dioxide, ozone, formaldehyde, etc. (Wong et al., 2006). The algorithm for this module can create a warning when the air quality measurements exceed the exposure levels indicated in the guidelines (WHO., 2010; OSHA., 2015). Furthermore, the control algorithm can increase the air flow rate through the HVAC system or open the windows to reduce the build-up of indoor pollutants by integrating with the BMS (Fisk et al., 2010).
Many of the indoor pollutants are a result of emissions from fuel combustion (e.g., while cooking), furnishing or from consumer products and construction materials (Zhang and Smith, 2003), whose exposure could be reduced by selection of better materials. Indoor CO2, on the other hand, results primarily from occupants' respiration (Satish et al., 2012; Persily and de Jonge, 2017). CO2 is one of the indoor air quality parameters that has a direct on impact occupants' health and work productivity (Seppanen et al., 1999; Satish et al., 2012). CO2 concentrations exceeding 950 ppm were found to cause significant declines in cognitive scores, even though the level of exposure is considered acceptable by ASHRAE 62.1, which provides ventilation guidelines for acceptable indoor air quality (Allen et al., 2016). Past studies have shown that the exhaled CO2 does not immediately dissipate with the surrounding air when occupants are sedentary and results in a buildup of a personal CO2 bubble around the occupant's head (Ozkaynak et al., 1996; Ghahramani et al., 2019). A recent study has shown that using a desk fan can dissipate the CO2 bubble and reduce the CO2 concentration by 177 ppm on average (Ghahramani et al., 2019). The use of personal fans can maintain comfortable thermal conditions while also reducing CO2 concentration and improving perceived air quality even when the air movement is from recirculated room air (Zhang et al., 2010; Ghahramani et al., 2019). Control algorithms that consider both thermal comfort and improvement in air quality need to be developed for control of PCS devices, such as fans or local air terminals.
Other Supporting Components
There are additional components of the desk that support the application modules discussed above. These components provide the necessary infrastructure for collecting and storing sensor data and monitoring the status of the desk. We briefly mention these components and some of the commercially available products in this section, but a detailed review is beyond the scope of this paper.
Energy Monitoring
Energy monitoring at the desk can provide granular data to help building managers understand how energy is consumed in the building. Several studies have used wired and wireless plug load meters to monitor energy consumption of different appliances (Lifton et al., 2007; Jiang et al., 2009). Furthermore, Non-Intrusive Load Monitoring (NILM) techniques have been developed to disaggregate the energy consumption of different appliances from an aggregated source (Rowe et al., 2010; Jazizadeh et al., 2014). If certain occupants are wasting energy, they can be identified and prompted about their wasteful habits. Different algorithms to evaluate energy waste in office settings have been developed. For instance, Ahmadi-Karvigh et al. (2018) developed an ontology-based approach for quantifying the energy waste of occupants. In addition to appliances used by individuals, Cheng et al. developed methods to attribute energy consumption of shared resources to individuals to calculate a per person energy footprint (Cheng et al., 2012). Several energy-monitoring plug meters are commercially available on the market, such as WeMo from Belkin, Kill A Watt from P3 international, Extech, etc., which can be used for monitoring appliance level energy consumption. The energy monitoring module can also provide input to the activity recognition module described in section Activity Recognition and provide feedback to the user using the interaction module described in section User Interaction.
Cloud Storage and Computing
Cloud computing is another supporting module that is required for all other modules. The sensor data that is gathered from the desk, along with logs of user interaction, can be stored in the cloud servers where different algorithms can be used to process the data to create meaningful actions. For a small number of desks, local servers may be set up for storage and data analysis. Different database systems, such as IRONdb and InfluxDB are available for storage of time series sensor signals and can easily be set up on local or cloud servers. For larger implementations, cloud computing is usually cheaper and more scalable than implementing local servers (Armbrust et al., 2010). Several service providers, such as Amazon, Google, Microsoft, IBM, etc., provide easy access to cloud computing with real time data analytics. The algorithms for each of the modules can run simultaneously on a cloud server, and they can be used for analyzing real time behaviors of the user to provide appropriate feedback. Given that different cloud service providers offer products with similar capabilities, any service can be adopted for storage and computing of data collected from the desk.
Data Visualization Dashboard
Visualization of data collected from the desk can be useful to identify use patterns, detect anomalies and increase awareness of building operations. Visualization dashboards can enable building managers to monitor the operation of building systems and take corrective actions in case of system malfunction or optimize building energy use (Chen et al., 2009). Current BMS and BAS are unable to provide information related to individual occupants since the monitoring of the environment usually occurs at the zone level. The desk monitors the local environment around the user and can provide relevant data at a much granular level, which can enable the dashboard to provide real time and historical information regarding comfort and IEQ-related parameters and energy consumption for each user in the building. Several frameworks have been developed by researchers to visualize sensor data for building management applications, such as WattDepot (Brewer and Johnson, 2010) and SAGA (Buevich et al., 2011). Indeed, several open source solutions exist for visualization of sensor data collected from IoT devices, such as Thingsboard (https://thingsboard.io), Freeboard (http://freeboard.io/), Grafana (https://grafana.com/), Graphite (https://graphiteapp.org/), etc. Furthermore, there are additional potential applications that could use the data gathered from the desk but are not directly linked to the occupant, such as demand response and failure prediction. Although these applications are beyond the scope of this paper, a visualization dashboard could be an important tool for these applications as well.
Discussion and Future Directions
We strongly believe that the smart desk can help improve productivity and well-being of office workers in the future. We see the greatest potential as the improvement of indoor environmental quality in buildings with centralized HVAC systems and poor daylighting. However, occupants in buildings that are naturally ventilated or have natural daylighting can also benefit from the increased local control. Since the desk modules are separated by application, it is possible to only select the modules that focus on a particular aspect of the indoor environment that needs improvement. This could lower the initial investment costs by reducing the number of sensing and actuation devices needed. Even though our current vision is focused on office buildings, the idea of personalizing indoor environments using current IoT technology and advancements in machine learning and control fields can be applied to other types of buildings as well. Furthermore, sensing and actuation devices can be implemented on other pieces of furniture, such as office chairs in order to provide similar functions. A pilot implementation of the smart desk is discussed in (Aryal et al., 2018).
Although ample related research has already been conducted, there are still gaps that need to be addressed to enable wide scale use of IoT devices for improving productivity and well-being of building occupants. Current methods for learning user preferences heavily rely on user feedback as discussed in section Overall Smart Desk Framework. Lack of user feedback can negatively impact the accuracy of learned preferences and therefore, lead to discomfort and dissatisfaction. To address this issue, better methods for sensing occupant comfort and robust methods for learning user preferences that work with low amount of user feedback need to be developed. Simultaneously, methods to increase a user's engagement and to sustain the level of engagement over time need to be developed using better design of the interaction between the desk and the user.
Another challenge lies in trying to identify the right optimization objective. The objectives of optimizing for comfort, productivity, health and well-being or energy efficiency may sometimes conflict with each other. For instance, prolonged exposure to a uniform thermal environment might be comfortable, but it could have adverse health impacts, such as reduced metabolism and increased risk of cardiovascular diseases (van Marken Lichtenbelt et al., 2017). Exposure to bright white light can increase immediate alertness and productivity (Badia et al., 1991) but it can affect the circadian rhythm and negatively impact the occupants' health over the long term (Pauley, 2004; Boyce, 2014). Slightly cooler temperatures may improve productivity but compromise comfort (Geng et al., 2017). Optimizing for comfort might lead to increased energy use in some climates (Aryal and Becerik-Gerber, 2018). It is difficult to quantify the tradeoffs between different objectives and it is important to find a balance between these different objectives. The potential economic benefits from improved work performance and reduced absenteeism due to improved indoor environmental quality are estimated to be two orders of magnitude larger than the increase in associated energy costs (Fisk et al., 2011). Occupants in commercial buildings are not responsible for the associated energy costs and energy consumption might not be a concern for building occupants. However, it is still important to improve the indoor environmental quality in energy efficient ways to reduce greenhouse gas emissions. Finding a balance between multiple objectives is challenging as it depends on the values of the stakeholders responsible for indoor environment control in each building. Further research is necessary to understand the potential tradeoffs between trying to optimize for different objectives and to find a balance.
Past research has mostly focused on a single aspect of the indoor environmental quality (i.e., thermal comfort, visual comfort, air quality, ergonomics, etc.). However, one aspect of the indoor environmental quality might influence another aspect. For instance, the user might hunch or move closer while trying to read a text that is affected by glare, reflection or poor contrast caused by poor lighting, and thus also leading to poor posture (Joines et al., 2015). The circadian rhythm, which is influenced by the lighting environment, is also responsible for the regulation of core body temperature and can indirectly impact thermal comfort (Pauley, 2004). Many such influences may be discovered when different aspects are studied together. In order to improve our understanding of the interplays between different variables, there is a need for research that focuses on studying the indoor environment as a whole and is conducted in the real world rather than in controlled environments. Although new evidence is emerging on the negative impacts of poor indoor environments on health and productivity, there is a lack of consensus in what indoor conditions are ideal for promoting health and productivity. Long-term studies are needed to improve our understanding of the impacts of indoor environments on these two objectives. Large-scale studies are needed to explore the variations on the impact of the environment on health and productivity that is affected by individual differences. Such knowledge would greatly enhance our capabilities to tailor the environment to promote health while accounting for individual characteristics. There is also a need for standardized metrics for the measurement of productivity and health in indoor environments, which can be utilized to quantify improvements in health and productivity achieved by improving the indoor environment.
Conclusion
Centralized control of indoor environments is unable to cater to individual requirements of building occupants. Furthermore, existing BMS and BAS rely on zone level monitoring of an environment and do not consider individual preferences. In order to cater toward individual preferences and personalize the local environment around each user, the monitoring and control of the environment should occur around each individual. Personalizing the environment based on individual preferences can lead to increased satisfaction. In this paper, we described our vision of a smart desk that leverages recent advances in IoT to monitor the environment, machine learning to learn user preferences and predictive control algorithms to control the environment based on their preferences. Furthermore, we also described our vision where the smart desk acts as an intelligent support system which can motivate the user to change their preferences toward healthier, more productive and more energy efficient settings. We also provided a reference for the readers to look at an overview of our current prototypes for the smart desk and our work in progress (Aryal et al., 2018) and we discussed some research gaps and future research directions. We believe that there is great potential to enhance occupant comfort, productivity and health by using smart furniture, such as the smart office desk using the framework described in this paper.
Author Contributions
All authors had contribution toward the conception of different ideas in the manuscript. AA drafted the manuscript. Remaining authors contributed their ideas in different sections and provided critical feedback.
Funding
This work is partially based upon work supported by the ARUP 2017 Global Research Challenge and the National Science Foundation under grant #1763134. Any opinions, findings, and conclusions or recommendations expressed in this material are those of the authors and do not necessarily reflect the views of the National Science Foundation.
Conflict of Interest Statement
The authors declare that the research was conducted in the absence of any commercial or financial relationships that could be construed as a potential conflict of interest.
References
Ahmadi-Karvigh, S., Ghahramani, A., Becerik-Gerber, B., and Soibelman, L. (2017). One size does not fit all: understanding user preferences for building automation systems. Energy Build. 145, 163–173. doi: 10.1016/j.enbuild.2017.04.015
Ahmadi-Karvigh, S., Ghahramani, A., Becerik-Gerber, B., and Soibelman, L. (2018). Real-time activity recognition for energy efficiency in buildings. Appl. Energy 211, 146–160. doi: 10.1016/j.apenergy.2017.11.055
Alahakone, A. U., and Senanayake, S. M. N. A. (2010). A real-time system with assistive feedback for postural control in rehabilitation. IEEE ASME Trans. Mechatron. 15, 226–233. doi: 10.1109/TMECH.2010.2041030
Allen, J. G., MacNaughton, P., Laurent, J. G. C., Flanigan, S. S., Eitland, E. S., and Spengler, J. D. (2015). Green buildings and health. Curr. Environ. Heal. Rep. 2, 250–258. doi: 10.1007/s40572-015-0063-y
Allen, J. G., MacNaughton, P., Satish, U., Santanam, S., Vallarino, J., and Spengler, J. D. (2016). Associations of cognitive function scores with carbon dioxide, ventilation, and volatile organic compound exposures in office workers: a controlled exposure study of green and conventional office environments. Environ. Health Perspect. 124, 805–812. doi: 10.1289/ehp.1510037
Altomonte, S., Schiavon, S., Kent, M. G., and Brager, G. (2017). Indoor environmental quality and occupant satisfaction in green-certified buildings. Build. Res. Inf. 47, 255–274. doi: 10.1080/09613218.2018.1383715
Amai, H., Tanabe, S., Akimoto, T., and Genma, T. (2007). Thermal sensation and comfort with different task conditioning systems. Build. Environ. 42, 3955–3964. doi: 10.1016/j.buildenv.2006.07.043
Arai, T., Machii, K., Kuzunuki, S., and Shojima, H. (1995). “InteractiveDESK: a computer-augmented desk which responds to operations on real objects,” in Conference Companion on Human Factors in Computing Systems (CHI'95) (New York, NY: ACM Press), 141–142.
Armbrust, M., Stoica, I., Zaharia, M., Fox, A., Griffith, R., Joseph, A. D., et al. (2010). A view of cloud computing. Commun. ACM 53:50. doi: 10.1145/1721654.1721672
Aryal, A., Anselmo, F., and Becerik-Gerber, B. (2018). “Smart IoT desk for personalizing indoor environmental conditions,” in Proceedings of the 8th International Conference on the Internet of Things–IOT'18 (New York, NY: ACM Press), 1–6.
Aryal, A., and Becerik-Gerber, B. (2018). Energy consequences of comfort-driven temperature setpoints in office buildings. Energy Build. 177, 33–46. doi: 10.1016/j.enbuild.2018.08.013
ASHRAE. (2017). ASHRAE Standard 55-2017:“Thermal Environmental Conditions for Human Occupancy.” Available online at: https://www.ashrae.org/technical-resources/standards-and-guidelines/read-only-versions-of-ashrae-standards
Badia, P., Myers, B., Boecker, M., Culpepper, J., and Harsh, J. R. (1991). Bright light effects on body temperature, alertness, EEG and behavior. Physiol. Behav. 50, 583–588. doi: 10.1016/0031-9384(91)90549-4
Borisuit, A., Scartezzini, J.-L., and Thanachareonkit, A. (2010). Visual discomfort and glare rating assessment of integrated daylighting and electric lighting systems using HDR imaging techniques. Archit. Sci. Rev. 53, 359–373. doi: 10.3763/asre.2009.0094
Bort-Roig, J., Gilson, N. D., Puig-Ribera, A., Contreras, R. S., and Trost, S. G. (2014). Measuring and influencing physical activity with smartphone technology: a systematic review. Sport. Med. 44, 671–686. doi: 10.1007/s40279-014-0142-5
Boyce, P., Hunter, C., and Howlett, O. (2003). The Benefits of Daylight Through Windows. Troy, NY: Rensselaer Polytechnic Institute.
Boyce, P. R. (2010). Review: the impact of light in buildings on human health. Indoor Built Environ. 19, 8–20. doi: 10.1177/1420326X09358028
Boyce, P. R. (2014). Human Factors in Lighting. 3rd edn. Boca Raton, FL: CRC Press. doi: 10.1201/b16707
Brager, G., Zhang, H., and Arens, E. (2015). Evolving opportunities for providing thermal comfort. Build. Res. Inf. 43, 274–287. doi: 10.1080/09613218.2015.993536
Braz, C., and Robert, J.-M. (2006). “Security and usability: the case of the user authentication methods,” in Proceedings of the 18th International Conference on Association Francophone d'Interaction Homme-Machine–IHM'06 (New York, NY: ACM Press), 199–203.
Brewer, R. S., and Johnson, P. M. (2010). “WattDepot: an open source software ecosystem for enterprise-scale energy data collection, storage, analysis, and visualization,” in 2010 First IEEE International Conference on Smart Grid Communications (Gaithersburg, MD: IEEE), 91–95.
Buevich, M., Rowe, A., and Rajkumar, R. (2011). “SAGA: tracking and visualization of building energy,” in 2011 IEEE 17th International Conference on Embedded and Real-Time Computing Systems and Applications (Toyama: IEEE), 31–36.
Burzo, M., Abouelenien, M., Pérez-Rosas, V., Wicaksono, C., Tao, Y., and Mihalcea, R. (2014). “Using infrared thermography and biosensors to detect thermal discomfort in a building's inhabitants,” in ASME 2014 International Mechanical Engineering Congress and Exposition (Montreal, QC: American Society of Mechanical Engineers), V06BT07A015.
Carr, L. J., Leonhard, C., Tucker, S., Fethke, N., Benzo, R., and Gerr, F. (2016). Total worker health intervention increases activity of sedentary workers. Am. J. Prev. Med. 50, 9–17. doi: 10.1016/j.amepre.2015.06.022
Cedeño-Laurent, J. G., Williams, A., MacNaughton, P., Cao, X., Eitland, E., Spengler, J., et al. (2018). Building evidence for health: green buildings, current science, and future challenges. Annu. Rev. Public Health 39, 291–308. doi: 10.1146/annurev-publhealth-031816-044420
Chan, Y.-C., and Tzempelikos, A. (2013). Efficient venetian blind control strategies considering daylight utilization and glare protection. Sol. Energy 98, 241–254. doi: 10.1016/j.solener.2013.10.005
Chatzidiakou, L., Mumovic, D., and Summerfield, A. (2015). Is CO 2 a good proxy for indoor air quality in classrooms? Part 1: the interrelationships between thermal conditions, CO 2 levels, ventilation rates and selected indoor pollutants. Build. Serv. Eng. Res. Technol. 36, 129–161. doi: 10.1177/0143624414566244
Chau, J. Y., Grunseit, A. C., Chey, T., Stamatakis, E., Brown, W. J., Matthews, C. E., et al. (2013). Daily sitting time and all-cause mortality: a meta-analysis. PLoS ONE 8:e80000. doi: 10.1371/journal.pone.0080000
Chen, H., Chou, P., Duri, S., Lei, H., and Reason, J. (2009). “The design and implementation of a smart building control system,” in 2009 IEEE International Conference on e-Business Engineering (Macau: IEEE), 255–262.
Cheng, Y., Chen, K., Zhang, B., Liang, C.-J. M., Jiang, X., and Zhao, F. (2012). “Accurate real-time occupant energy-footprinting in commercial buildings,” in Proceedings of the Fourth ACM Workshop on Embedded Sensing Systems for Energy-Efficiency in Buildings–BuildSys'12 (New York, NY: ACM Press), 115.
Chu, A. H. Y., Ng, S. H. X., Tan, C. S., Win, A. M., Koh, D., and Müller-Riemenschneider, F. (2016). A systematic review and meta-analysis of workplace intervention strategies to reduce sedentary time in white-collar workers. Obes. Rev. 17, 467–481. doi: 10.1111/obr.12388
Clausen, G., and Wyon, D. (2008). The combined effects of many different indoor environmental factors on acceptability and office work performance. HVACandR Res. 14, 103–113. doi: 10.1080/10789669.2008.10390996
Clear, R. (2013). Discomfort glare: what do we actually know? Light. Res. Technol. 45, 141–158. doi: 10.1177/1477153512444527
Coen, M. H. (1998). “A prototype intelligent environment,” in Cooperative Buildings: Integrating Information, Organization, and Architecture, eds N. A. Streitz, S. Konomi, and H.-J. Burkhardt (Berlin; Heidelberg: Springer), 41–52.
Cole, R. J., and Brown, Z. (2009). Reconciling human and automated intelligence in the provision of occupant comfort. Intell. Build. Int. 1, 39–55. doi: 10.3763/inbi.2009.0007
Cuddy, A. J., Doherty, K. T., and Bos, M. W. (2010). OPOWER: Increasing Energy Efficiency through Normative Influence (A). Brighton, MA: Harvard Business School Case 911–016.
Dautenhahn, K. (2007). Methodology and themes of human-robot interaction: a growing research field. Int. J. Adv. Robot. Syst. 4, 103–108. doi: 10.5772/5702
Day, J. K., and O'Brien, W. (2017). Oh behave! Survey stories and lessons learned from building occupants in high-performance buildings. Energy Res. Soc. Sci. 31, 11–20. doi: 10.1016/j.erss.2017.05.037
De Croon, E., Sluiter, J., Kuijer, P. P., and Frings-Dresen, M. (2005). The effect of office concepts on worker health and performance: a systematic review of the literature. Ergonomics 48, 119–134. doi: 10.1080/00140130512331319409
De Dear, R. J., Brager, G. S., Reardon, J., and Nicol, F. (1998). Developing an adaptive model of thermal comfort and preference. ASHRAE Trans. 104:145.
de Kort, Y., and Smolders, K. (2010). Effects of dynamic lighting on office workers: first results of a field study with monthly alternating settings. Light. Res. Technol. 42, 345–360. doi: 10.1177/1477153510378150
De Vries, P., and Midden, C. (2008). Effect of indirect information on system trust and control allocation. Behav. Inf. Technol. 27, 17–29. doi: 10.1080/01449290600874956
Department for Professional Employees. (2015). The Professional and Technical Workforce–DPEAFLCIO. DPE Fact sheet. Available online at: http://dpeaflcio.org/programs-publications/issue-fact-sheets/the-professional-and-technical-workforce/#_ednref3 (accessed September 2, 2017).
Despenic, M., Chraibi, S., Lashina, T., and Rosemann, A. (2017). Lighting preference profiles of users in an open office environment. Build. Environ. 116, 89–107. doi: 10.1016/j.buildenv.2017.01.033
Erickson, V. L., and Cerpa, A. E. (2012). “Thermovote: participatory sensing for efficient building HVAC conditioning,” in BuildSys'12 (New York, NY: ACM Press), 9.
Fanger, P. O. (1970). Analysis and Applications in Environmental Engineering. Danish Technical Press, 244. Available at: https://www.cabdirect.org/cabdirect/abstract/19722700268 (accessed January 23, 2018).
Felstead, A., Jewson, N., and Walters, S. (2005). The shifting locations of work: new statistical evidence on the spaces and places of employment. Work. Employ. Soc. 19, 415–431. doi: 10.1177/0950017005053186
Fisekis, K., Davies, M., Kolokotroni, M., and Langford, P. (2003). Prediction of discomfort glare from windows. Light. Res. Technol. 35, 360–369. doi: 10.1191/1365782803li095oa
Fisk, W. J. (2000). Health and productivity gains from better indoor environments and their relationship with building energy efficiency. Annu. Rev. Energy Environ. 25, 537–566. doi: 10.1146/annurev.energy.25.1.537
Fisk, W. J., Black, D., and Brunner, G. (2011). Benefits and costs of improved IEQ in US offices. Indoor Air 21, 357–367. doi: 10.1111/j.1600-0668.2011.00719.x
Fisk, W. J., Sullivan, D. P., Faulkner, D., and Eliseeva, E. (2010). CO2 Monitoring for Demand Controlled Ventilation in Commercial Buildings, LBNL-3279E. Berkeley, CA: Lawrence Berkeley National Laboratory.
Fogleman, M., and Lewis, R. J. (2002). Factors associated with self-reported musculoskeletal discomfort in video display terminal (VDT) users. Int. J. Ind. Ergon. 29, 311–318. doi: 10.1016/S0169-8141(01)00071-3
Gaglio, S., Re, G., and Lo Morana, M. (2015). Human activity recognition process using 3-D posture data. IEEE Trans. Hum. Mach. Syst. 45, 586–597. doi: 10.1109/THMS.2014.2377111
Gao, Y., Zhang, H., Arens, E., Present, E., Ning, B., Zhai, Y., et al. (2017). Ceiling fan air speeds around desks and office partitions. Build. Environ. 124, 412–440. doi: 10.1016/j.buildenv.2017.08.029
Gebhardt, C., Rädle, R., and Reiterer, H. (2014). “Integrative workplace: studying the effect of digital desks on users' working practices,” in CHI'14 Extended Abstracts on Human Factors in Computing Systems (New York, NY: ACM Press), 2155–2160.
Geng, Y., Ji, W., Lin, B., and Zhu, Y. (2017). The impact of thermal environment on occupant IEQ perception and productivity. Build. Environ. 121, 158–167. doi: 10.1016/j.buildenv.2017.05.022
Ghahramani, A., Castro, G., Becerik-Gerber, B., and Yu, X. (2016). Infrared thermography of human face for monitoring thermoregulation performance and estimating personal thermal comfort. Build. Environ. 109, 1–11. doi: 10.1016/j.buildenv.2016.09.005
Ghahramani, A., Pantelic, J., Vannucci, M., Pistore, L., Liu, S., Gilligan, B., et al. (2019). Personal CO2 bubble: context-dependent variations and wearable sensors usability. J. Build. Eng. 22, 295–304. doi: 10.1016/j.jobe.2018.11.015
Gubbi, J., Buyya, R., Marusic, S., and Palaniswami, M. (2013). Internet of Things (IoT): a vision, architectural elements, and future directions. Futur. Gener. Comput. Syst. 29, 1645–1660. doi: 10.1016/j.future.2013.01.010
Gunay, B., and Shen, W. (2017). Connected and distributed sensing in buildings: improving operation and maintenance. IEEE Syst. Man, Cybern. Mag. 3, 27–34. doi: 10.1109/MSMC.2017.2702386
Gunay, H. B., O'Brien, W., Beausoleil-Morrison, I., and Gilani, S. (2017). Development and implementation of an adaptive lighting and blinds control algorithm. Build. Environ. 113, 185–199. doi: 10.1016/j.buildenv.2016.08.027
Haldi, F., and Robinson, D. (2010). Adaptive actions on shading devices in response to local visual stimuli. J. Build. Perform. Simul. 3, 135–153. doi: 10.1080/19401490903580759
He, Y., Li, N., He, M., and He, D. (2017). Using radiant cooling desk for maintaining comfort in hot environment. Energy Build. 145, 144–154. doi: 10.1016/j.enbuild.2017.04.013
Helander, M. G., Czaja, S. J., Drury, C. G., Cary, J. M., and Burri, G. (1987). Office technology and people an ergonomic evaluation of office chairs. Off. Technol. People Facil. Iss Facil. 3, 247–263. doi: 10.1108/eb022651
Hellwig, R., and Boerstra, A. (2017). Personal control over indoor climate disentangled, Part 2. Rehva J. 23–26. Available online at: https://www.rehva.eu/fileadmin/user_upload/20-23_RJ1804_WEB.pdf
Hoyt, T., Lee, K. H., Zhang, H., and Arens, E. (2009). “Energy savings from extended air temperature setpoints and reductions in room air mixing,” in Proceedings of the 13th International Conference on Environmental Ergonomics, ICEE 2009 (Boston, MA: ICCE).
Huizenga, C., Abbaszadeh, S., Zagreus, L., and Arens, E. A. E. (2006). Air quality and thermal comfort in office buildings : results of a large indoor environmental quality survey. Proc. Heal. Build. III, 393–397.
Hye Oh, J., Ji Yang, S., and Rag Do, Y. (2014). Healthy, natural, efficient and tunable lighting: four-package white LEDs for optimizing the circadian effect, color quality and vision performance. Light Sci. Appl. 3:e141. doi: 10.1038/lsa.2014.22
Jaimes, A., and Sebe, N. (2007). Multimodal human–computer interaction: a survey. Comput. Vis. Image Underst. 108, 116–134. doi: 10.1016/j.cviu.2006.10.019
James, P., Bertrand, K. A., Hart, J. E., Schernhammer, E. S., and Tamimi, R. M. (2016). Outdoor light at night and breast cancer incidence in the nurses' health study II. Environ. Health Perspect. 125:087010. doi: 10.1289/EHP935
Jazizadeh, F., and Becerik-Gerber, B. (2012). “Toward adaptive comfort management in office buildings using participatory sensing for end user driven control,” in Proceedings of the Fourth ACM Workshop on Embedded Sensing Systems for Energy-Efficiency in Buildings–BuildSys'12 (New York, NY: ACM Press), 1.
Jazizadeh, F., Becerik-Gerber, B., Berges, M., and Soibelman, L. (2014). “Unsupervised clustering of residential electricity consumption measurements for facilitated user-centric non-intrusive load monitoring,” in Computing in Civil and Building Engineering (2014) (Reston, VA: American Society of Civil Engineers), 1869–1876.
Jiang, X., Dawson-Haggerty, S., Dutta, P., and Culler, D. (2009). “Design and implementation of a high-fidelity AC metering network,” in 2009 International Conference on Information Processing in Sensor Networks (San Francisco, CA), 253–264.
Joines, S., James, T., Liu, S., Wang, W., Dunn, R., and Cohen, S. (2015). Adjustable task lighting: field study assesses the benefits in an office environment. Work 51, 471–481. doi: 10.3233/WOR-141879
Juslén, H., Wouters, M., and Tenner, A. (2007). The influence of controllable task-lighting on productivity: a field study in a factory. Appl. Ergon. 38, 39–44. doi: 10.1016/j.apergo.2006.01.005
Karakolis, T., and Callaghan, J. P. (2014). The impact of sit–stand office workstations on worker discomfort and productivity: a review. Appl. Ergon. 45, 799–806. doi: 10.1016/j.apergo.2013.10.001
Karmann, C., Schiavon, S., and Arens, E. (2018). “Percentage of commercial buildings showing at least 80% occupant satisfied with their thermal comfort,” in Proceedings of 10th Windsor Conference: Rethinking Comfort. Available online at: https://cloudfront.escholarship.org/dist/prd/content/qt89m0z34x/qt89m0z34x.pdf?t=p9m07w
Karol, S., and Robertson, M. M. (2015). Implications of sit-stand and active workstations to counteract the adverse effects of sedentary work: a comprehensive review. Work 52, 255–267. doi: 10.3233/WOR-152168
Khashe, S., Becerik-Gerber, B., Lucas, G., and Gratch, J. (2018a). “Persuasive effects of immersion in virtual environments for measuring pro-environmental behaviors,” in Proceedings of the 35th International Symposium on Automation and Robotics in Construction (ISARC) (Berlin: IAARC), 1189–1195. doi: 10.22260/ISARC2018/0167
Khashe, S., Lucas, G., Becerik-Gerber, B., and Gratch, J. (2018b). Establishing social dialog between buildings and their users. Int. J. Hum. Computer Interact. 34, 1–12. doi: 10.1080/10447318.2018.1555346
Khashe, S., Heydarian, A., Carneiro, J., and Becerik-Gerber, B. (2015). Exploration of Building-Occupant Communication Methods for Reducing Energy Consumption in Buildings. Cham: Springer.
Khashe, S., Lucas, G., Becerik-Gerber, B., and Gratch, J. (2017). Buildings with persona: towards effective building-occupant communication. Comput. Human Behav. 75, 607–618. doi: 10.1016/j.chb.2017.05.040
Kim, J., Schiavon, S., and Brager, G. (2018). Personal comfort models–A new paradigm in thermal comfort for occupant-centric environmental control. Build. Environ. 132, 114–124. doi: 10.1016/j.buildenv.2018.01.023
Kim, W., Han, H., and Kim, J. T. (2009). The position index of a glare source at the borderline between comfort and discomfort (BCD) in the whole visual field. Build. Environ. 44, 1017–1023. doi: 10.1016/j.buildenv.2008.07.007
Klepeis, N. E., Nelson, W. C., Ott, W. R., Robinson, J. P., Tsang, A. M., Switzer, P., et al. (2001). The National Human Activity Pattern Survey (NHAPS): a resource for assessing exposure to environmental pollutants. J. Expo. Anal. Environ. Epidemiol. 11, 231–252. doi: 10.1038/sj.jea.7500165
Laestadius, J. G., Ye, J., Cai, X., Ross, S., Dimberg, L., and Klekner, M. (2009). The proactive approach—Is it worthwhile? A prospective controlled ergonomic intervention study in office workers. J. Occup. Environ. Med. 51, 1116–1124. doi: 10.1097/JOM.0b013e3181bae19d
Laput, G., Zhang, Y., and Harrison, C. (2017). “Synthetic sensors,” in Proceeding of 2017 CHI Conference on Human Factors in Computing Systems–CHI'17 (Denver, CO), 3986–3999.
Leaman, A. (1999). Window seat or aisle? Studies of buildings in use are showing the importance of personal control of one's environment, which includes windows. Archit. J. Available online at: https://www.architectsjournal.co.uk/home/window-seat-or-aisle-studies-of-buildings-in-use-are-showing-the-importance-of-personal-control-of-ones-environment-which-includes-windows/770070.article (accessed July 16, 2018).
Lerch, F. J., Prietula, M. J., and Kulik, C. T. (1997). “The Turing effect: the nature of trust in expert systems advice,” in Expertise in Context, eds P. J. Feltovich, K. M. Ford, and R. R. Hoffman (Cambridge, MA: MIT Press), 417–48.
Li, D., Menassa, C. C., and Kamat, V. R. (2018). Non-intrusive interpretation of human thermal comfort through analysis of facial infrared thermography. Energy Build. 176, 246–261. doi: 10.1016/j.enbuild.2018.07.025
Lifton, J., Feldmeier, M., Ono, Y., Lewis, C., and Paradiso, J. A. (2007). “A platform for ubiquitous sensor deployment in occupational and domestic environments,” in 2007 6th International Symposium on Information Processing in Sensor Networks (Cambridge, MA: IEEE), 119–127.
Loper, E., and Bird, S. (2002). “NLTK: the natural language toolkit,” in Proceedings of the ACL-02 Workshop on Effective Tools and Methodologies for Teaching Natural Language Processing and Computational Linguistics-Volume 1 ETMTNLP '02 (Philadelphia, PA: Association for Computational Linguistics), 63–70. doi: 10.3115/1118108.1118117
Luo, M., Arens, E., Zhang, H., Ghahramani, A., and Wang, Z. (2018). Thermal comfort evaluated for combinations of energy-efficient personal heating and cooling devices. Build. Environ. 143, 206–216. doi: 10.1016/j.buildenv.2018.07.008
Maurer, U., Rowe, A., Smailagic, A., and Siewiorek, D. (2006). Location and Activity Recognition Using eWatch: A Wearable Sensor Platform. Berlin; Heidelberg: Springer.
Moeslund, T. B., and Granum, E. (2001). A survey of computer vision-based human motion capture. Comput. Vis. Image Underst. 81, 231–268. doi: 10.1006/cviu.2000.0897
Mullet, K., and Sano, D. (1995). Designing Visual Interfaces : Communication Oriented Techniques. SunSoft Press. Available online at: https://dl.acm.org/citation.cfm?id=201011 (accessed September 26, 2018).
Mutlu, B., Krause, A., Forlizzi, J., Guestrin, C., and Hodgins, J. (2007). “Robust, low-cost, non-intrusive sensing and recognition of seated postures,” in Proceedings of the 20th Annual ACM Symposium on User Interface Software and Technology–UIST'07 (New York, NY: ACM Press), 149.
Nazzal, A. A. (2005). A new evaluation method for daylight discomfort glare. Int. J. Ind. Ergon. 35, 295–306. doi: 10.1016/j.ergon.2004.08.010
Newsham, G., Arsenault, C., Veitch, J., Tosco, A. M., and Duval, C. (2005). Task lighting effects on office worker satisfaction and performance, and energy efficiency. LEUKOS 1, 7–26. doi: 10.1582/LEUKOS.2004.01.04.001
Nguyen, T. A., Nguyen, T. A., and Aiello, M. (2012). Beyond indoor presence monitoring with simple sensors. Proc. PECCS 2012, 5–14. doi: 10.5220/0003801300050014
Ochoa, C. E., Aries, M. B. C., van Loenen, E. J., and Hensen, J. L. M. (2012). Considerations on design optimization criteria for windows providing low energy consumption and high visual comfort. Appl. Energy 95, 238–245. doi: 10.1016/j.apenergy.2012.02.042
Oi, N., and Takahashi, H. (2007). “Preferred combinations between illuminance and color temperature in several settings for daily living activities,” in Proceedings of the 2nd International Symposium on Design of Artificial Environments (Beijing), 214–215.
OSHA. (2015). Indoor Air Quality in Commercial and Institutional Buildings. Maroon Ebooks. Washington, DC: U.S. Occupational Safety and Health Administration.
Osterhaus, W. K. E. (2005). Discomfort glare assessment and prevention for daylight applications in office environments. Sol. Energy 79, 140–158. doi: 10.1016/j.solener.2004.11.011
Ozkaynak, H., Xue, J., Spengler, J., Wallace, L., Pellizzari, E., and Jenkins, P. (1996). Personal exposure to airborne particles and metals: results from the Particle TEAM study in Riverside, California. J. Expo. Anal. Environ. Epidemiol. 6, 57–78.
Pak, R., Fink, N., Price, M., Bass, B., and Sturre, L. (2012). Decision support aids with anthropomorphic characteristics influence trust and performance in younger and older adults. Ergonomics 55, 1059–1072. doi: 10.1080/00140139.2012.691554
Paone, A., Bacher, J.-P., Paone, A., and Bacher, J.-P. (2018). The impact of building occupant behavior on energy efficiency and methods to influence it: a review of the state of the art. Energies 11:953. doi: 10.3390/en11040953
Park, S., and Yoo, W. (2012). Effect of EMG-based feedback on posture correction during computer operation. J. Occup. Health 54, 271–277. doi: 10.1539/joh.12-0052-OA
Parry, S., and Straker, L. (2013). The contribution of office work to sedentary behaviour associated risk. BMC Public Health 13:296. doi: 10.1186/1471-2458-13-296
Pasut, W., Zhang, H., Arens, E., and Zhai, Y. (2015). Energy-efficient comfort with a heated/cooled chair: results from human subject tests. Build. Environ. 84, 10–21. doi: 10.1016/j.buildenv.2014.10.026
Paul, W. L., and Taylor, P. A. (2008). A comparison of occupant comfort and satisfaction between a green building and a conventional building. Build. Environ. 43, 1858–1870. doi: 10.1016/j.buildenv.2007.11.006
Pauley, S. M. (2004). Lighting for the human circadian clock: recent research indicates that lighting has become a public health issue. Med. Hypotheses 63, 588–596. doi: 10.1016/j.mehy.2004.03.020
Pérez-Lombard, L., Ortiz, J., and Pout, C. (2008). A review on buildings energy consumption information. Energy Build. 40, 394–398. doi: 10.1016/j.enbuild.2007.03.007
Persily, A., and de Jonge, L. (2017). Carbon dioxide generation rates for building occupants. Indoor Air 27, 868–879. doi: 10.1111/ina.12383
Rea, M., and Figueiro, M. (2018). Light as a circadian stimulus for architectural lighting. Light. Res. Technol. 50, 497–510. doi: 10.1177/1477153516682368
Rea, M. S., Ouellette, M. J., and Kennedy, M. E. (1985). Lighting and task parameters affecting posture, performance and subjective ratings. J. Illum. Eng. Soc. 15, 231–238. doi: 10.1080/00994480.1985.10748645
Robertson, M. M., Ciriello, V. M., and Garabet, A. M. (2013). Office ergonomics training and a sit-stand workstation: effects on musculoskeletal and visual symptoms and performance of office workers. Appl. Ergon. 44, 73–85. doi: 10.1016/j.apergo.2012.05.001
Roussac, A. C., Steinfeld, J., and de Dear, R. (2011). A preliminary evaluation of two strategies for raising indoor air temperature setpoints in office buildings. Archit. Sci. Rev. 54, 148–156. doi: 10.1080/00038628.2011.582390
Rowe, A., Berges, M., and Rajkumar, R. (2010). “Contactless sensing of appliance state transitions through variations in electromagnetic fields,” in Proceedings of the 2nd ACM Workshop on Embedded Sensing Systems for Energy-Efficiency in Building–BuildSys'10 (New York, NY: ACM Press), 19.
Sadeghi, S. A., Lee, S., Karava, P., Bilionis, I., and Tzempelikos, A. (2018). Bayesian classification and inference of occupant visual preferences in daylit perimeter private offices. Energy Build. 166, 505–524. doi: 10.1016/j.enbuild.2018.02.010
Satish, U., Mendell, M. J., Shekhar, K., Hotchi, T., Sullivan, D., Streufert, S., et al. (2012). Is CO2 an indoor pollutant? Direct effects of low-to-moderate CO2 concentrations on human decision-making performance. Environ. Health Perspect. 120, 1671–7. doi: 10.1289/ehp.1104789
Sato, Y., Oka, K., Koike, H., and Nakanishi, Y. (2004). “Video-based tracking of user's motion for augmented desk interface,” in Proceedings–Sixth IEEE International Conference on Automatic Face and Gesture Recognition (Seoul: IEEE), 805–809.
Seppanen, O., and Fisk, W. (2006). Some quantitative relations between indoor environmental quality and work performance or health. HVACandR Res. 12, 957–973. doi: 10.1080/10789669.2006.10391446
Seppanen, O. A., Fisk, W. J., and Mendell, M. J. (1999). Association of ventilation rates and CO2 concentrations with health andother responses in commercial and institutional buildings. Indoor Air 9, 226–252. doi: 10.1111/j.1600-0668.1999.00003.x
Spain, R. D., and Madhavan, P. (2009). The role of automation etiquette and pedigree in trust and dependence. Proc. Hum. Factors Ergon. Soc. Annu. Meet. 53, 339–343. doi: 10.1177/154193120905300437
Stevens, R. G. (2009). Light-at-night, circadian disruption and breast cancer: assessment of existing evidence. Int. J. Epidemiol. 38, 963–970. doi: 10.1093/ije/dyp178
Straker, L., Levine, J., and Campbell, A. (2009). The effects of walking and cycling computer workstations on keyboard and mouse performance. Hum. Factors J. Hum. Factors Ergon. Soc. 51, 831–844. doi: 10.1177/0018720810362079
Thorp, A. A., Kingwell, B. A., Owen, N., and Dunstan, D. W. (2014). Breaking up workplace sitting time with intermittent standing bouts improves fatigue and musculoskeletal discomfort in overweight/obese office workers. Occup. Environ. Med. 71, 765–771. doi: 10.1136/oemed-2014-102348
Toftum, J., Jørgensen, A. S., and Fanger, P. O. (1998). Upper limits for indoor air humidity to avoid uncomfortably humid skin. Energy Build. 28, 1–13. doi: 10.1016/S0378-7788(97)00017-0
Tudor-Locke, C., Leonardi, C., Johnson, W. D., and Katzmarzyk, P. T. (2011). Time spent in physical activity and sedentary behaviors on the working day: the American time use survey. Am. Coll. Occup. Environ. Med. 53, 1382–1387. doi: 10.1097/JOM.0b013e31823c1402
Turunen, M., Toyinbo, O., Putus, T., Nevalainen, A., Shaughnessy, R., and Haverinen-Shaughnessy, U. (2014). Indoor environmental quality in school buildings, and the health and wellbeing of students. Int. J. Hyg. Environ. Health 217, 733–739. doi: 10.1016/j.ijheh.2014.03.002
US Department of Energy. (2015). US Department of Energy Quadrennial Technology Review. Available online at: https://www.energy.gov/under-secretary-science-and-energy/quadrennial-technology-review (accessed April 17, 2018).
van Marken Lichtenbelt, W., Hanssen, M., Pallubinsky, H., Kingma, B., and Schellen, L. (2017). Healthy excursions outside the thermal comfort zone. Build. Res. Inf. 45, 1–9. doi: 10.1080/09613218.2017.1307647
Vedsted, P., Søgaard, K., Blangsted, A. K., Madeleine, P., and Sjøgaard, G. (2011). Biofeedback effectiveness to reduce upper limb muscle activity during computer work is muscle specific and time pressure dependent. J. Electromyogr. Kinesiol. 21, 49–58. doi: 10.1016/j.jelekin.2010.06.002
Veselý, M., and Zeiler, W. (2014). Personalized conditioning and its impact on thermal comfort and energy performance–A review. Renew. Sustain. Energy Rev. 34, 401–408. doi: 10.1016/j.rser.2014.03.024
Vischer, J. C. (2007). The effects of the physical environment on job performance: towards a theoretical model of workspace stress. Stress Heal. 23, 175–184. doi: 10.1002/smi.1134
Wang, W., Liu, A. X., Shahzad, M., Ling, K., and Lu, S. (2015). “Understanding and modeling of WiFi signal based human activity recognition,” in Proceedings of the 21st Annual International Conference on Mobile Computing and Networking–MobiCom'15 (New York, NY: ACM Press), 65–76.
Waters, T. R., and Dick, R. B. (2015). Evidence of health risks associated with prolonged standing at work and intervention effectiveness. Rehabil. Nurs. 40, 148–165. doi: 10.1002/rnj.166
WHO. (2010). WHO Guidelines for Indoor Air Quality. Copenhagen: World Health Organization Regional Office for Europe.
Wimmer, R., Hennecke, F., Schulz, F., Boring, S., Butz, A., and Hußmann, H. (2010). “Curve: revisiting the digital desk,” in Proceedings of the 6th Nordic Conference on Human-Computer Interaction Extending Boundaries–NordiCHI'10 (New York, NY: ACM Press), 561.
Wojek, C., Nickel, K., and Stiefelhagen, R. (2006). “Activity recognition and room-level tracking in an office environment,” in 2006 IEEE International Conference on Multisensor Fusion and Integration for Intelligent Systems (Heidelberg: IEEE), 25–30.
Wong, L. T., Mui, K. W., and Hui, P. S. (2006). A statistical model for characterizing common air pollutants in air-conditioned offices. Atmos. Environ. 40, 4246–4257. doi: 10.1016/j.atmosenv.2006.04.005
Wong, S.-K., Wai-Chung Lai, L., Ho, D. C.-W., Chau, K.-W., Lo-Kuen Lam, C., and Hung-Fai Ng, C. (2009). Sick building syndrome and perceived indoor environmental quality: a survey of apartment buildings in Hong Kong. Habitat Int. 33, 463–471. doi: 10.1016/j.habitatint.2009.03.001
Yun, R. (2014). “Persistent workplace plug-load energy savings and awareness through energy dashboards. in Proceedings of the extended abstracts of the 32nd annual ACM conference on Human factors in computing systems–CHI EA'14 (New York, New York, USA: ACM Press), 331–334.
Yun, R., Lasternas, B., Aziz, A., Loftness, V., Scupelli, P., Rowe, A., et al. (2013). Toward the Design of a Dashboard to Promote Environmentally Sustainable Behavior among Office Workers. Berlin; Heidelberg: Springer.
Zhai, Y., Zhang, H., Zhang, Y., Pasut, W., Arens, E., and Meng, Q. (2013). Comfort under personally controlled air movement in warm and humid environments. Build. Environ. 65, 109–117. doi: 10.1016/j.buildenv.2013.03.022
Zhang, H., Arens, E., Kim, D., Buchberger, E., Bauman, F., and Huizenga, C. (2010). Comfort, perceived air quality, and work performance in a low-power task–ambient conditioning system. Build. Environ. 45, 29–39. doi: 10.1016/j.buildenv.2009.02.016
Zhang, H., Arens, E., and Zhai, Y. (2015). A review of the corrective power of personal comfort systems in non-neutral ambient environments. Build. Environ. 91, 15–41. doi: 10.1016/j.buildenv.2015.03.013
Zhang, J., and Smith, K. R. (2003). Indoor air pollution: a global health concern. Br. Med. Bull. 68, 209–225. doi: 10.1093/bmb/ldg029
Zhang, Y. F., Wyon, D. P., Fang, L., and Melikov, A. K. (2007). The influence of heated or cooled seats on the acceptable ambient temperature range. Ergonomics 50, 586–600. doi: 10.1080/00140130601154921
Keywords: personalized environments, smart desk, internet of things (IoT), smart buildings, indoor environmental quality (IEQ)
Citation: Aryal A, Becerik-Gerber B, Anselmo F, Roll SC and Lucas GM (2019) Smart Desks to Promote Comfort, Health, and Productivity in Offices: A Vision for Future Workplaces. Front. Built Environ. 5:76. doi: 10.3389/fbuil.2019.00076
Received: 13 December 2018; Accepted: 20 May 2019;
Published: 12 June 2019.
Edited by:
Fadi Alsaleem, University of Nebraska-Lincoln, United StatesReviewed by:
Kristen S. Cetin, Iowa State University, United StatesRalph T. Muehleisen, Argonne National Laboratory (DOE), United States
Copyright © 2019 Aryal, Becerik-Gerber, Anselmo, Roll and Lucas. This is an open-access article distributed under the terms of the Creative Commons Attribution License (CC BY). The use, distribution or reproduction in other forums is permitted, provided the original author(s) and the copyright owner(s) are credited and that the original publication in this journal is cited, in accordance with accepted academic practice. No use, distribution or reproduction is permitted which does not comply with these terms.
*Correspondence: Burcin Becerik-Gerber, YmVjZXJpayYjeDAwMDQwO3VzYy5lZHU=