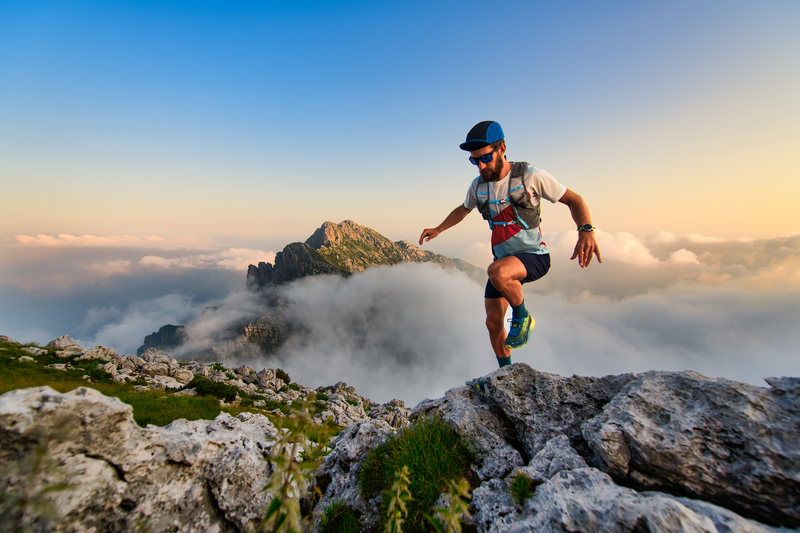
95% of researchers rate our articles as excellent or good
Learn more about the work of our research integrity team to safeguard the quality of each article we publish.
Find out more
ORIGINAL RESEARCH article
Front. Blockchain , 27 November 2024
Sec. Blockchain for Good
Volume 7 - 2024 | https://doi.org/10.3389/fbloc.2024.1440355
This article is part of the Research Topic Blockchain in the Age of AI View all articles
This study aims to identify and assess AI and blockchain solutions in relation to journalistic authenticity and integrity. Central to our exploration is the role of blockchain technology in verifying content provenance. As a key component of a global Web3 framework, blockchain could offer a foundation for authenticating the origins of content. In this article, we explore how blockchain, with its capacity for creating immutable and cryptographically signed data records, could be applied by journalists to verify photos, videos and documents. Our analysis identified nine blockchain-based solutions for content verification, with three platforms–Attestiv, OriginStamp, and Fact Protocol–showing particular promise for journalistic workflows. We conclude that while AI and blockchain solutions are currently available to journalists today, they require high-level technical expertise. Many media companies are now venturing into this field as well, thus affecting the professional role of journalists in general. In our study, it is evident that integrating AI and blockchain in journalism is not merely about adopting new tools but also about understanding their broader implications for journalism as a profession and the convergence in society. The focus must remain on enhancing journalistic integrity and public trust to ensure that these technological advances benefit the field of journalism and, by extension, the democratic processes it supports.
The term generative AI refers to artificial intelligence that is capable of generating text, images, sound or other data (Bäck, 2023). Artificial Intelligence (AI) could be both useful for journalism but also extremely challenging as it becomes increasingly difficult to distinguish genuine human-generated content from AI-produced content. Given the importance of journalistic integrity, distinguishing between AI-generated and human-generated content is emerging as a formidable challenge for journalism’s future.
The rise of generative AI has exacerbated issues related to misinformation and fake news with fake local news websites now outnumbering legitimate ones in the United States (Kunova, 2024). This development highlights the urgent need for reliable verification mechanisms, particularly for journalists. Blockchain has the potential to enhance verification through different approaches including by facilitating community-driven platforms where readers participate actively in news verification processes. This democratization of information has the potential to help build trust and accountability within journalism as an industry. The immutable nature of blockchain records ensures that once an article is published, it cannot be altered without leaving a trace, fostering transparency and integrity (Kunova, 2024).
This study aims to identify and assess blockchain solutions in relation to journalistic authenticity and integrity. The exploration presented in this article is both theoretical and practical and serves as an attempt to explore how blockchain could be used in journalism as generative AI begins to proliferate. Our inquiry is driven by a crucial question: How can we distinguish genuine human-generated content from AI-produced content in an era increasingly influenced by AI’s extensive content creation? Our research questions are as follows:
1. What existing blockchain-based solutions could help journalists distinguish genuine human-generated content from AI-produced news?
2. What are the opportunities for and challenges to using these solutions to both verify online content and certify own content?
Central to our exploration is the role of blockchain technology in verifying content provenance. As a key component of a global Web3 framework, blockchain could offer a foundation for authenticating the origins of content. In this article, we explore how blockchain, with its capacity for creating immutable and cryptographically signed data records, could be applied by journalists to verify photos, videos and documents.
While generative AI presents unprecedented capabilities in content creation, distinguishing this AI-generated content from human-created content poses significant challenges. This study explores blockchain as a potential solution to ensure the integrity and authenticity of content in journalism. The remainder of this paper is organized as follows: The background and literature review section provides historical context and current research on AI and blockchain in journalism. The theoretical framework section introduces the theory of professionalism and the theory of convergence which are valuable when discussing the implications of our results. The research methodology section details our mixed-methods approach to evaluating blockchain solutions. The results section presents our findings from analyzing nine blockchain platforms, with detailed examination of three promising solutions. The discussion section explores the implications of these technologies for journalistic practice, followed by our conclusions.
Since its inception in the 1950s, AI’s evolution has profoundly impacted multiple sectors, notably journalism where it has reshaped content creation and verification processes. British scientist Alan Turing was one of the first to explore the mathematical possibility of building intelligent machines and testing their intelligence (Turing, 1950). Five years later, other researchers initialized the proof of concept by developing a program designed to mimic the problem-solving skills of a human being. The program was presented at a conference at Dartmouth College in New Hampshire, United States and catalyzed the next 20 years of AI research. From 1957 to 1974 AI developed gradually, and computers could store more information and became faster, cheaper and more accessible. Machine-learning algorithms improved, becoming more useful in solving problems (Anyoha, 2017).
In the 1980s, research on AI received more funding, and deep learning techniques were developed, which allowed computers to “learn” by using their experience. In the 1990s and 2000s, large investments were made in AI, and many landmark goals in the field were met, such as when world chess champion Gary Kasparov was defeated by IBM’s chess playing computer program Deep Blue in 1997 (Anyoha, 2017). Another landmark was met in 2016 when a computer named AlphaGo, developed by Google DeepMind, defeated world champion Lee Sedol at the ancient and very popular strategy game Go (Willingham, 2023).
Subsequently, AI has permeated various aspects of society through diverse applications. Machine learning is the dominant field and includes neural networks, deep learning and other types of learning (Wärnestål et al., 2023). AI applications can be found in search algorithms, statistical analyses, and internet search history, among other things. Today, AI has applications in every way we use computers in society. In this study, we use the term AI when we refer to technologies that work independently or assist automation of media production. This includes robots that create news content (text, sound and images), algorithms that adapt news content to user preferences, and applications that compile and analyze large quantities of data.
On the journalism front, Peña-Fernández et al. (2023) underscore the need to consider the societal impacts of AI, particularly generative AI, on journalists, audiences, and the profession at large. Pavlik (2023) examines the capabilities and constraints of generative AI, while Kothari and Cruikshank (2022) call for more research into its deployment in African newsrooms. The emergence of ChatGPT as a generative AI platform significantly influences journalism and media education with its capacity for instant text generation, albeit bounded by limitations and ethical considerations that warrant careful scrutiny (Karakose, 2023; Quintans-Júnior et al., 2023; Tsigaris and Teixeira Da Silva, 2023). Marconi (2020) suggests that AI could complement rather than supplant human journalism, highlighting the critical role of editorial oversight in ensuring content quality and integrity. This points to the essential need for a cautious approach to AI that fosters innovation while conscientiously addressing ethical concerns, including bias, privacy, and misinformation (Kenthapadi et al., 2023).
Current pressing challenges in the domain include the exponential growth of synthetic media, with deepfake videos doubling every 6 months and AI-generated news becoming increasingly sophisticated and believable (Kunova, 2024). This development is particularly concerning as traditional detection methods struggle to keep pace, making blockchain-based verification systems a viable solution or maintaining journalistic integrity from the source. The intersection of these challenges with the diminishing trust in the media (Newman et al., 2024) creates an urgent need for robust solutions.
Historically, blockchain technology is much younger than AI. It was first invented by the anonymous developer going under the pseudonym Satoshi Nakamoto, who introduced the Bitcoin protocol in a paper entitled Bitcoin: A peer-to-peer electronic cash system (Nakamoto, 2008). Blockchain is essentially a digital ledger system that operates over the Internet to create and maintain a decentralized digital ledger of transactions by individual members of the network to exchange anything of value, including electronic cash, without the need for a central authority, such as a bank or an exchange (Picha Edwardsson and Al-Saqaf, 2022).
Blockchain employs advanced cryptographic techniques and reward systems to maintain data integrity and security, while also encouraging participation by offering financial incentives. The idea that no central authority controls the data or ensures consistency is a paradigm shift in the field of digital financing and value sharing online, and it has the potential to change society in many ways (Al-Saqaf and Seidler, 2017). One of the areas in which blockchain could have a direct impact is tracking provenance and securing factual information, such as database entries of an encyclopedia or records of events (Picha Edwardsson and Al-Saqaf, 2022).
The increasing use of blockchain has led to the new concept Web3. The term gained traction with the creation of web3. js, the first JavaScript application programming interface (API) for Ethereum, the world’s second largest blockchain. This suite of libraries allows users to interact with Ethereum nodes via web protocols, which takes advantage of Ethereum’s smart contract capabilities (Buterin, 2015).
Liu et al. (2021) highlight blockchain’s potential in promoting press freedom, protecting digital rights, and combating fake news, though he acknowledges that research in this domain is still developing. Luo and Krishnamurthy (2022) investigate how blockchain could support political journalism by tackling the surplus of content, dwindling consumer willingness to pay, and reporting biases. However, Gupta et al. (2019) identify significant barriers, such as scalability and security, that blockchain needs to overcome. Kim and Yoon (2018) champion a blockchain-based model for journalism that seeks to restore lost revenue and trust by capitalizing on decentralization and the collective voice of the public.
Further exploration into blockchain reveals its utility in augmenting journalism’s fact-checking processes, as demonstrated by Picha Edwardsson and Al-Saqaf (2022). They devised a prototype for securely recording fact checks on a blockchain, making them universally accessible. Although their initiative proves the viability of a global fact-checking network, they also pinpoint considerable obstacles like cost, complexity, and the swift pace of technological progress, hinting that blockchain’s application in fact-checking is still evolving. Additionally, Al-Saqaf and Picha Edwardsson (2019) delve into the Civil project, which sought to enhance journalistic quality and sustainability through blockchain. They attribute Civil’s shortcomings to its dependency on nascent blockchain technology and various economic and governance problems, which collectively impeded its widespread adoption and practicality in journalism.
Within journalism, AI is used to autonomously generate textual content or other software that is designed to carry out specific tasks formerly associated with humans (Guzman and Lewis, 2020). As mentioned earlier, the term generative AI is used to describe software capable of generating text, images, sound or other data (Bäck, 2023).
There are many ongoing projects in the world exploring new ways of using AI in journalism. One such initiative was taken in the summer of 2023 when the American Journalism Project, a philanthropy working to rebuild local news, announced a partnership with OpenAI, the AI research and development company behind ChatGPT, to explore ways in which AI can support local news. The collaboration aims to establish lines of dialogue between the local news industry and OpenAI and develop tools that could assist local news organizations (OpenAI, 2023). Traditional news outlets, such as The New York Times, The Washington Post, and Associated Press, have also deployed AI in different newsroom projects (Chan-Olmsted, 2019).
Furthermore, Verdens Gang (VG), one of Norway’s premier news outlets, is using AI to help enhance its newsroom productivity. With a team of seven developers, VG is experimenting with various AI-driven tools built to meet the needs of the newsroom (Vijay, 2024). Another example of a collaboration project between a media company and university researchers is the Swedish study Exploring Communicative AI: Reflections from a Swedish Newsroom (Stenbom et al., 2023). The study explored AI’s ability to generate search engine-optimized headlines for the Swedish daily Aftonbladet.
Many of the recent scholarly studies within this area focus on the role of journalism, the role of the journalist, and the changing journalism profession in connection to AI. For example, Min and Fink (2021) investigate the changing role of journalists in relation to emerging technologies and what journalists think about this. They warn that new technologies such as AI and the automation of processes can be seen as the savior for everything within the media industry. Consequently, it is important to critically reflect on the ongoing development of AI within journalism.
Scholars de-Lima-Santos and Ceron (2022) argue that AI can take different forms in the news industry and suggest three major subfields that are more present in the news ecosystem: (1) machine learning/computer vision, (2) planning/scheduling, and (3) optimization. They conclude that machine learning algorithms are often used to strengthen news outlets’ business models and boost revenue streams (de-Lima-Santos and Ceron, 2022, p.11). Another area where AI could be used more frequently in the future is investigative journalism. According to Stray (2019), AI could be used in investigative journalism to cut costs and streamline work processes. While not a branch of journalism research, one key research domain, as demonstrated by the work of Fraga-Lamas and Fernández-Caramés (2020), Ki Chan et al. (2020), Patil and Chouragade (2021), Yazdinejad et al. (2020) among others, is the utilization of blockchain to help in the detection of deepfakes, which indirectly supports the work of journalists.
Blockchain is a potentially useful technology in the fight against misinformation. By providing immutable records and tracking content provenance, blockchain helps detect and mark AI-generated content. This technology directly enhances the credibility of journalistic content and addresses misinformation challenges head-on. While AI alone faces challenges in accurately identifying and categorizing misinformation amid ethical concerns, integrating it with blockchain could theoretically offer a potent solution. However, it is critical to stress that this requires robust governance mechanisms and access controls by news organizations to ensure the accuracy of information before it is added to the immutable blockchain record. The combination of AI and blockchain could possibly aim to authenticate content and discern fake news effectively. Research by Patil and Chouragade (2021), Fraga-Lamas and Fernández-Caramés (2020), and Ki Chan et al. (2020) illustrates how blockchain can trace the origins of digital media, enhancing transparency and accountability. Buțincu and Alexandrescu (2023) introduce a platform leveraging collective intelligence and AI to more dynamically counter disinformation. Jaroucheh et al. (2020) propose TRUSTD, a system that enables content creators to verify their work and audiences to assess credibility. Despite these advancements, there is a notable research gap from a journalistic perspective, emphasizing the urgent need for further investigation into how these technologies can be pragmatically and ethically integrated into journalism to tackle disinformation and enhance trust.
It should be noted that content verification systems that initially employ blockchain technology may eventually transition to alternative technologies. A pertinent example is Truepic, accessible at truepic.com, which presently utilizes Digital Content Authenticity (DCA) to transparently track the origins and subsequent modifications of digital content. Initially, Truepic leveraged blockchain by generating a unique hash for each captured image or video and embedding it in the Bitcoin blockchain, thereby creating a permanent record for future reference. However, correspondence with a Truepic representative showed that the platform had shifted from a blockchain-centric approach to a Public Key Infrastructure (PKI)-based system for various reasons (Ibrahim, 2024). Recently, Truepic has broadened its capabilities to include the authentication of generative AI content through a collaboration with Revel. ai. This partnership facilitates the creation of authenticated deepfake videos, underscored by transparent technological processes.
As the distinction between genuine and synthetic content becomes increasingly blurred, it is imperative to understand how to merge AI and blockchain effectively to meet journalism’s requirements for authenticity, reliability, and transparency. Additionally, there should be a focus on the ethical implications of these technologies, such as potential biases in AI models and privacy concerns related to blockchain’s immutable records. This research aims to explore the frameworks and methodologies necessary to blend these technologies, addressing the pressing need for accuracy and accountability in news reporting. It also highlights the importance of collaborative efforts between technologists, journalists, and media organizations to ensure the successful implementation of these solutions. This study seeks to uncover the practicalities and ethical considerations of these technologies in journalism, a crucial step for identifying usability requirements and addressing the complexities of adopting AI and blockchain amid the challenges posed by generative AI content.
Figure 1 illustrates the interconnected relationship between blockchain technology, artificial intelligence, and journalism in the context of content verification. The overlapping areas represent key functionalities where these domains intersect: blockchain provides provenance and immutability, AI offers detection and generation capabilities, while journalism contributes the essential element of trust. At the center, where all three domains converge, lies the critical function of content verification and authentication that this study explores.
In this study, we mainly focus on the intersection of journalism, AI, and blockchain, by examining cases that use blockchain as a means to help journalists verify specific digital content they find on the web by extracting metadata such as creator, date of creation, whether it is human- or AI-generated, etc. We have also assessed the usability of blockchain technology for journalists to verify content provenance and create tamper-proof evidence of their own created work.
When exploring the intersection of journalism, AI, and blockchain, we mainly use two theories: professionalism theory and convergence theory. Because media companies are constantly under pressure to work more innovatively to create new products and services (Lowrey, 2011; Küng, 2017), inevitably, the meaning of professional journalism is affected. According to Waisbord (2013) p.6, professional journalism is a matter of permanent debate both within journalism and academia, but on a general level there is a normative set of ideas about what journalism is and should be. Ultimately, professional journalism stands for necessary quality standards and ideals that should guide press performance in a democracy.
Furthermore, Waisbord argues that journalists should be “insulated from market pressures and composed of truth-seeking news professionals” (2013, p.9). Professionalism is a way to define conditions and rules of work within certain political, economic, social and cultural settings. According to Waisbord (2013) p.11, professionalism refers to the ability of a field of practice to settle boundaries and avoid intrusion from external actors; “Professions do not exist in isolation; they are permanently engaged in relations with other social fields” (Waisbord, 2013, p.11). Thus, Waisbord argues that journalism should be understood as a field of practice that seeks to maintain separation from other fields, particularly politics and economics (p.13).
Nygren (2019) argues that journalistic professionalism results in journalists increasingly trying to keep a distance from politicians and other authorities in society. But the difficult balance in the media industry between the commercial side and the journalistic side remains (Nygren, 2019, p.61). This is especially true when introducing new innovative solutions in the media industry. In turn, Karlsson (2019) states that the work process of journalism consists of gathering/creating, choosing, processing, presenting and distributing information. In this process, the actual processing of information is a particularly important step, including verification of content, as a means of professional journalism.
The study of AI and blockchain technology in relation to journalism also touches on the theory of convergence. According to Henry Jenkins (2006), the merger of media production and consumption leads to a global convergence culture that is based on an increasingly participatory and interactive engagement between people and their media and professional and amateur media makers (Jenkins, 2006, p.172; Deuze, 2008, p.74). Mark Deuze argues that convergence culture plays into the hands of corporations that are seeking new ways to intensify customer relationships (2007, p.80). Furthermore, the impact of new technologies in the news industry increases the demand for and pressures on journalists who have to retool and diversify their skillset to produce more work in the same amount of time. Technology is used to supplement rather than radically change the work of journalists, and it takes a long time to change the working culture of a news organization. “The contemporary drive towards some kind of convergence across two or more media thus tends to offer little in terms of radically different forms of journalism or ways in which to gather, select, or report the news” (Deuze, 2008, p.155). Reflecting on the theory of convergence is thus valuable in our study when we explore specific cases where blockchain could potentially help journalists verify certain digital content.
In this study, we adopted a mixed-methods approach to explore the use of blockchain in verifying content for journalistic purposes. Our methodology integrates a systematic review of blockchain solutions targeting disinformation that is partially attributed to the rise of generative AI content and is complemented by testing the tools from a journalistic viewpoint. This approach aims to evaluate the technologies’ practical effectiveness, and usability in journalistic contexts when differentiating between authentic and AI-generated content is becoming more difficult over time.
The initial phase of our research focused on identifying solutions that work in the domain of blockchain in the context of AI-driven disinformation. We conducted a Google search with the query (Blockchain AI) (deepfake OR deepfakes OR fake OR fakes OR disinformation OR misinformation). This search was designed to identify the most relevant solutions that argue for or have implemented the use of blockchain in their operations, specifically targeting the issues of disinformation and the support of journalism.
Once the search results were obtained, we manually went through the pages to identify solutions that are available and positioned in the domain of disinformation, AI and blockchain. Once identified, we did a systematic literature review of the content on the official websites of y nine of these solutions, focusing on their objectives, methodologies, technologies used, outcomes, and their promise in combating disinformation and enhancing journalistic integrity. The solutions under review were meticulously chosen for their potential to mitigate the prevalent challenges in content verification faced by journalists, including the authentication of external sources and protection against content tampering. These solutions span a broad spectrum of blockchain technologies, offering varied approaches to the verification and authentication of journalistic content.
Our evaluation framework for the selected solutions is based on the following essential criteria, as encapsulated in our comparative analysis table:
• Blockchain Technology: The blockchain platform underpinning each solution.
• Verification of Digital Content: The mechanism each solution employs to ascertain the authenticity and provenance of digital content.
• Content Detection and Details: The solution’s proficiency in identifying the source of content that is already certified in the blockchain, including specifics about its creation, author and other metadata.
• Content Creation and Authentication: The availability of functionalities that empower journalists to authenticate their content.
• Integration with Journalistic Workflows: The degree of compatibility with existing journalistic practices and tools.
• User Experience for Journalists: The practicality and user-friendliness of the solution from a journalistic standpoint.
As part of the evaluation, we started with a thorough reviewing of official documentation and website content for each solution to extract detailed insights into their features, capabilities, and application scenarios. Based on our review, we identified the tools that we assessed to have a high emphasis on verification of digital content, and that are easy to integrate with journalistic workflows. At that point, we proceeded to do an in-depth journalistic evaluation of those tools.
Following the comprehensive evaluation of nine blockchain-based solutions aimed at enhancing journalistic practices in content verification and authentication, we selected a small subset of tools for more in-depth evaluation from a journalistic viewpoint to explore the practical impact of implementing such solutions in the field of journalism. The primary objective is to understand how these technologies could be integrated into journalistic workflows to authenticate content and distinguish real from fake news.
The evaluation was carefully formulated based on the data and insights obtained from our evaluation of the blockchain solutions. At this stage of the evaluation, we actively engaged with each of the shortlisted solutions to directly assess their functionality. Whenever possible, we accessed the services through web browsers to test usability, accessibility, and performance directly via their services (whether on the web or via their apps). The evaluation looked for perspectives on two critical use cases:
1) Detecting Content Authenticity: The utility of the solutions to help journalists discern the authenticity of external content, distinguishing between real and fake news.
2) Certifying Own Content: The utility of the solutions in enabling journalists to certify the authenticity of their own content, thereby bolstering credibility and trustworthiness.
We began each evaluation with an overview of the blockchain solutions evaluated, highlighting specific features and capabilities relevant to journalistic applications. Using our experience as former journalists, we then proceeded to test the solutions in real-life situations independently of each other and compiled their individual results into one single section.
We acknowledged potential limitations in our research, including the selection bias toward solutions that are publicly documented and accessible via Google, which uses a proprietary algorithm when showing results. However, since Google is the world’s largest search engine, it can be argued that it is a good measure of the perceived relevance and impact of those solutions. Furthermore, the rapidly evolving nature of technology might render some findings obsolete and is an inherent challenge in measuring the impact of these technologies on journalism and disinformation.
The study identified nine solutions that utilize blockchain technology to help detect fake or AI-generated content in some capacity and are available to journalists in their daily work. The details of each of those projects are provided below.
1. NOTARDEC https://www.notardec.com/Records forge-proof content that is anchored in time by leveraging fundamental properties of blockchain to help secure, prove, and timestamp data.
2. TrustApp https://trustapp.ai/Harnesses blockchain technology and natural language processing (NLP) to automatically sift through content on the web, informing users whether the information they see is trustworthy.
3. BlockCERTs https://www.blockcerts.org/Creates, views and verifies blockchain-based certificates. These digital records are registered on a blockchain, cryptographically signed, tamper-proof, and shareable.
4. Numbers Protocol https://www.numbersprotocol.io/Tracks digital provenance with blockchain technology by verifying the authenticity and traceability of online media, fostering a more transparent and trustworthy digital world for creators, businesses, and consumers.
5. Attestiv https://attestiv.com/Uses blockchain technology through a cloud-scale platform to ensure the authenticity and validity of digital assets utilizing patented AI technology.
6. Factom https://factom.pro/Allows users to create their own unique chains to group data specific to their application over a sustained period of time, thereby creating a transparent history of ordered state changes. This can be used, for example, to write tamperproof data and track the state of arbitrary content such as a file or database.
7. Fact Protocol https://fact.technology/Powers a suite of tools, also known as DApps in Web3 terms, that combats fake news, misinformation, and disinformation using native fact-checking applications, open APIs, and community contributions.
8. OriginStamp https://originstamp.com/Offers tamper-proof evidence of creation and ownership, which protects against plagiarism and data fabrication.
9. MultiversX https://multiversx.com/Provides an embeddable cryptographic seal to secure documents or media, establish historic authenticity, maintain digital integrity, and ensure effective deepfake countermeasures.
The review of projects and initiatives revealed a diverse range of applications—from enhancing the authenticity and transparency of news content to providing tools to verify information and combat deepfakes.
We analyzed the above solutions by distilling each platform’s distinct aspects into concise descriptors based on the public information provided on their respective websites. As shown in Table 1 below, there is an observable variance based on the provided descriptions, including blockchain technology application, verification of digital content, integration with journalistic workflows, and the notable features that set each platform apart.
This table provides a quick comparative overview and focuses on key differentiators and the general suitability of each solution for journalistic applications. It is important to note that the effectiveness and applicability of these solutions can vary based on specific journalistic needs, technical infrastructure, and the type of content being produced or verified. Further in-depth investigation and direct engagement with each platform may be necessary to fully assess their potential in specific journalistic contexts.
As the table shows, the solutions that stand out as particularly aligned with the needs of journalism by offering a high level of digital content verification and are easy to integrate into journalistic workflows are Attestiv, OriginStamp, and Fact Protocol. Hence, we proceed with an in-depth analysis as shown below to describe these three tools and test them in a journalistic manner. It is worth noting that the below results are condensed to point to concrete clear outcomes. The full raw results are available on Github at https://github.com/wsaqaf/blockchainai (Al-Saqaf, 2024).
Blockchain Technology Application: Attestiv utilizes blockchain and AI to identify suspicious elements in digital assets and detect alterations, addressing the growing concern over manipulated media and deepfakes in journalism.
Verification of Digital Content: Offering a high level of verification, Attestiv could be used to ensure the integrity of digital media, an essential feature for journalists facing the challenge of verifying the authenticity of images and videos in their reporting. However, in tests, the tool displayed limitations, including some false positives where real videos were incorrectly flagged as suspicious.
Integration with Journalistic Workflows: Attestiv’s platform could be integrated easily with journalistic workflows, facilitating an efficient process for initial verification, although journalists may need to fingerprint content continuously for full traceability of modifications. This ease of use is critical for the rapid verification needs inherent in news reporting. According to the Attestiv team, the current urgency from media companies is verification of digital media. This need is ever more prevalent due to the rise of AI-generated video content. While the solution has not yet gained traction with journalists, Attestiv’s potential in the journalistic workflow could be in providing authentication for original photos and videos taken by journalists using their own cameras or mobile devices. Such content could be fingerprinted with blockchain to establish a chain of custody from the point of creation onwards. Furthermore, journalists could validate photos and videos provided by third parties by running them through Attestiv’s specialized AI detection software, thus providing web workflows that would allow journalists access to authentication and verification processes (Hamilton, 2024).
Notable Features: The application of AI technology for the detection of manipulations stands out as a key feature. It provides journalists with a powerful tool to verify content authenticity, enhancing the credibility of their work.
Challenges: While Attestiv is effective in initial verification, consistently tracking and fingerprinting modifications is challenging, which can be labor-intensive for journalists. For journalists, understanding how a photo has changed from the moment it was taken to publication is crucial. Attestiv’s focus on initial authenticity verification might not fully address the need to document and verify the lineage of edits and alterations unless sources ‘fingerprint’ their material continuously, making it less effective in contexts where the evolution of digital content is as significant as its origin.
Test results: The free trial was user-friendly to set up and use. There are different price levels to meet different needs. The solution in the trial tier aims to detect ‘suspicious’ aspects of deepfakes media content. A test was conducted for ten different videos, which is the maximum allowed per month in the trial plan. The test included five real and five AI-generated or manipulated videos. The tool successfully detected manipulated content but flagged some real videos as suspicious, indicating a need for improved accuracy. While the tool successfully identified most of the lip syncing and AI-generated aspects of the manipulated or AI-generated content, it was clear that it also had false positive when it marked real videos that were not altered with suspicious attributes. However, further improvements in user interface and ease of use are necessary to enhance its accessibility for journalists with limited technical backgrounds.
Blockchain Technology Application: OriginStamp leverages blockchain to provide tamper-proof timestamps, ensuring the integrity and originality of digital content. This functionality is crucial for certifying when a piece of journalistic content was created or modified.
Verification of Digital Content: OriginStamp offers a high level of verification, which could enable journalists to confidently establish the timing and authenticity of content. This capability is particularly useful in verifying the provenance of documents and other digital assets. However, OriginStamp does not verify the authenticity of the content itself, such as distinguishing between real and AI-generated media.
Integration with Journalistic Workflows: With its straightforward integration, OriginStamp has the potential to complement journalistic processes, allowing for a reliable way to timestamp and confirm document provenance, though not to authenticate media content. This ease of adoption ensures that journalists can leverage OriginStamp’s features without significant adjustments to their workflow.
Notable Features: The provision of tamper-proof timestamps is a significant feature, enhancing trust in digital documents and media by providing undeniable evidence of their creation and any subsequent changes.
Challenges: Although OriginStamp provides tamper-proof timestamps for digital content to ensure that the timing and originality of content are verifiable, it may not be sufficient for identifying the authenticity of the content itself, especially with AI-generated materials that are designed to be indistinguishable from real content at the point of their creation. While OriginStamp ensures timestamp integrity, it does not address media authenticity, leaving journalists without confirmation on whether a file is synthetic or real, focusing instead on the integrity of the content’s timestamp.
Test results: OriginStamp provides blockchain-backed timestamping to ensure document existence and integrity. From a journalistic perspective, the process of verifying timestamps is straightforward, but the platform does not directly support verifying content authenticity. Additionally, timestamp verification were independently confirmed using blockchain explorer services, which were utilized in testing to confirm the Bitcoin and Ethereum transactions. Intuitive instructions for document verification were insufficient, suggesting that the tool may not yet be accessible to non-technical users, who likely represent the majority of journalists inclined to utilize the service. Additionally, it should be noted that an email we sent to the OriginStamp Team was not attended, indicating inadequate customer support. This poses further challenges for first-time users.
Blockchain Technology Application: Fact Protocol’s vision is to leverage blockchain for decentralized fact-checking and provenance tracking, but its current functionality is limited and does not yet employ blockchain verification. This approach has the potential if fully implemented to harness the blockchain’s strength in security and trustworthiness to combat misinformation effectively.
Verification of Digital Content: Fact Protocol offers a mechanism for the verification of digital content, employing a decentralized network of fact-checkers. This system allows for the verification of content across various formats, making it particularly useful for journalists seeking to validate the authenticity and accuracy of information before publication. Currently, however, we were not able to confirm that the Fact Protocol incorporates cryptographic verification or blockchain anchoring, limiting its functionality to metadata tagging.
Integration with Journalistic Workflows: The decentralized fact-checking service provided by Fact Protocol, such as the 2FA News Fact Checker, is designed for easy integration into journalistic workflows. This ease of adoption empowers journalists with a powerful tool for verifying facts in real-time, directly enhancing the credibility and reliability of their reporting.
Notable Features: Fact Protocol’s fact-checking model is in early development, lacking Web3 integration, which limits its practical application in journalistic environments. By leveraging a broad network of contributors for fact verification, however, it can introduce a collaborative approach to establishing the truthfulness of information, which is crucial in the era of widespread misinformation.
Challenges: While Fact Protocol’s decentralized fact-checking introduces a novel method for verifying information, it encounters challenges due to the variability in the quality of contributions. The reliance on a broad network and token-based governance model prompts concerns regarding the consistency of verification standards and compatibility with traditional newsroom practices where journalistic expertise, not token ownership, should drive editorial decisions. The robustness of mechanisms that ensure the reliability and objectivity of the fact-checking process remains a key concern. Additionally, the simplicity of integrating and the effectiveness of decentralized fact-checking in identifying sophisticated AI-generated content warrant further investigation to fully understand its capabilities and limitations in journalistic contexts. The Fact Protocol team has acknowledged these challenges and implemented safeguards, including penalties for fact-checkers who repeatedly err. They are also developing precautionary guidelines and standards to ensure fact-checkers uphold practices aligned with traditional journalistic integrity (Agadi, 2024).
Test results: Fact Protocol demonstrates potential as a journalist-oriented fact-checking tool. It is also possible to integrate with Google, which is a strength because Google is well-known but also a weakness because it means the service is not completely independent. Currently, the service seems to be under construction and not completely ready for use. The test results indicate that Fact Protocol’s tag generator and checker are functional but limited to metadata verification without blockchain anchoring or FACT token usage. Given its current status as an early-stage proof-of-concept, Fact Protocol lacks full functionality and can therefore not be tested fully. Yet, it demonstrates potential for future use in journalistic content verification.
The insights gained in this article underscore the importance of a balanced approach that respects journalistic traditions while embracing innovation. The integration of blockchain into journalistic practices enhances content credibility and directly addresses misinformation challenges. While generative AI offers unprecedented capabilities for content creation, it faces significant challenges with reliability and robustness. Research has shown that large language models often lack the ability to provide uncertainty estimates, making it difficult for users to determine when the model’s output can be trusted (Kenthapadi et al., 2023). This uncertainty in model confidence, combined with the potential for fabricated outputs or ‘hallucinations,’ underscores why media organizations must implement robust verification processes and avoid blind trust in AI-generated content.
While AI and blockchain solutions are available to journalists, the challenges they face when adopting this emerging technology mirror historical patterns of technological integration in journalism. The key is not just developing user-friendly interfaces, but ensuring that journalists understand how these technologies serve their professional needs and journalistic principles, particularly in content verification and authentication workflows. We notice that many media companies are now venturing into this field, thus affecting the professional role of journalists.
As we delve into the potential application of blockchain to support journalists verify content authenticity, including AI-generated content, it is crucial to recognize that synthetic content is not inherently problematic. When properly verified and human-assessed, AI-generated content can actually benefit journalism by automating routine tasks like summarization, allowing journalists to focus more time on investigative reporting and complex analysis. The key is distinguishing between legitimate uses of synthetic content and actual disinformation. In this context, practical tools such as Attestiv, OriginStamp, and Fact Protocol can help establish content provenance and authenticity, regardless of whether the content is human or AI-generated. Each of these solutions offers unique insights into how blockchain can enhance the credibility and efficiency of journalistic practices while also introducing new challenges and ethical considerations.
Attestiv, for example, leverages blockchain and AI to authenticate digital assets and detect alterations. This tool exemplifies how technology can support journalists in verifying the authenticity of images and videos, a crucial capability in an era dominated by digital media. However, the reliance on Attestiv underscores a significant shift in the journalistic workflow, necessitating a deeper understanding of blockchain and AI technologies. This shift raises important questions about the training and adaptation required for journalists to effectively integrate such technology into their daily practices. Attestiv can apply a unique signature called ‘fingerprints’ to videos and digital media submitted to then be stored on an immutable ledger, which can be used to regenerate and compare fingerprints to assets at any time. However, testing revealed that Attestiv sometimes flagged real content as suspicious, indicating a need for refined accuracy in its AI detection mechanisms. For record timestamping, if an altered version of that content is uploaded, it will no longer match. This, however, necessitates that legitimate alterations of content created by journalists must continuously be fingerprinted even when minor edits are made.
OriginStamp, on the other hand, provides tamper-proof timestamps to certify the creation and modification of digital content. Testing confirmed its reliability for timestamping but also highlighted that it does not authenticate the originality of the content itself, such as distinguishing synthetic media from genuine material. While this tool strengthens the integrity of journalistic outputs by ensuring content originality, it also highlights the potential limitations of blockchain in addressing the authenticity of the content itself—particularly AI-generated materials designed to mimic real content seamlessly. For example, one can authenticate a video file claiming that it was captured at a particular location and upload it for timestamping. But this does not verify whether the video is real or fake. The example of OriginStamp invites a broader discussion about the need for journalistic diligence and the potential pitfalls of over-reliance on technological solutions without adequately understanding their limitations.
Similarly, Fact Protocol introduces a decentralized approach to fact checking, utilizing a network of contributors to verify information. Currently, the protocol lacks blockchain-based verification and remains more of a proof-of-concept than a complete decentralized solution, as per test results. This model not only enhances the verification process but also democratizes it, potentially increasing public trust in journalistic content. However, the decentralized nature of Fact Protocol’s fact-checking process also introduces variability in the quality and consistency of the fact-checking, posing challenges to maintaining standard journalistic accuracy and reliability.
Based on our analysis of Attestiv, OriginStamp, and Fact Protocol, we can map how each solution addresses different journalistic needs. Table 2 demonstrates the practical applications of each tool, along with specific examples from our testing. This mapping helps journalists identify which solution might best serve their particular requirements.
These tools and the challenges they address reflect the ongoing tension between innovation and tradition in journalism. The introduction of such technologies into newsrooms can be seen as a potential solution to the increasing complexity of news verification in the digital age. Yet, they also necessitate a critical evaluation of how these technologies alter the landscape of journalistic ethics, particularly concerning transparency, accountability, and the potential for misuse. Additionally, while such solutions enhance content authenticity, they raise privacy concerns as immutable records could expose sensitive source information. A balanced approach must be developed to protect source anonymity while ensuring content credibility. This is particularly relevant for investigative journalism where confidential sources are crucial. Private or permissioned blockchain implementations, rather than public ones, might be more appropriate for handling sensitive journalistic content.
In this article, we have offered a comprehensive understanding of how blockchain-verified data can ethically and reliably support journalists in verifying whether content they find online is AI-generated or not and certifying their own content for others to verify. Our research questions were:
1. What existing solutions could help journalists distinguish genuine human-generated content from AI-produced news?
2. What are the opportunities for and challenges to using these solutions to both verify online content and certify own content?
Our findings demonstrate that the convergence of AI and blockchain technologies is not just underway but is important for the future of credible journalism. By providing robust tools for content verification and provenance tracking, blockchain could act as the missing piece in the fight against misinformation, ensuring the integrity and reliability of journalistic content. For advanced users, the technology is ready but requires in-depth knowledge about both AI and blockchain. Consequently, the technology is not yet ready for journalists with limited technical know-how.
Another conclusion of our study is that the introduction of AI and blockchain into the journalistic work processes will definitely have an effect on the professionalism of journalists. This is usually the case when introducing new innovative solutions in the media industry, as suggested by Nygren (2019) and Waisbord (2013). In line with the theory of convergence, the impact of new technologies on the news industry increases the demand for and pressure on journalists to retool and diversify their skillset (Deuze, 2008), and this is true in our study as well.
In the results section, we have examined nine different solutions where AI and blockchain technologies are integrated for the purpose of increasing authenticity and credibility in journalistic content, and we looked especially into three separate services that stand out as particularly aligned with the needs of journalism by offering a high level of digital content verification and easy to integrate into journalistic workflows. These services have their own pros and cons, but the service offered by Attestiv seems to be the easiest of the three from a journalist’s perspective, even though it may be rather demanding to upload and fingerprint new legitimate content on a continuous basis.
In synthesizing these reflections, it becomes evident that the dialogue surrounding the integration of AI and blockchain in journalism is not merely about adopting new tools but also about understanding the broader implications of these tools for journalism as a profession and convergence in society. As journalists and technologists collaborate to navigate these challenges, the focus must remain on enhancing journalistic integrity and public trust to ensure that these technological advances benefit the field of journalism and, by extension, the democratic processes it supports.
Looking ahead, several key challenges require further research and development. The most notable is to focus on how solution providers could improve the user interfaces of blockchain tools for non-technical journalists. Additionally, efforts should be exerted to develop standardized governance frameworks for content verification and create hybrid solutions that can handle both public news content and sensitive materials. Future directions should focus on integrating reliable emerging technologies that help with authentication and verification of information such as blockchain into existing newsroom workflows while addressing the pressing need for better source protection mechanisms.
The original contributions presented in the study are included in the article/Supplementary Material, further inquiries can be directed to the corresponding author.
MP: Conceptualization, Formal Analysis, Investigation, Methodology, Validation, Writing–original draft, Writing–review and editing. WA-S: Conceptualization, Data curation, Formal Analysis, Investigation, Methodology, Writing–original draft, Writing–review and editing.
The author(s) declare that no financial support was received for the research, authorship, and/or publication of this article.
We wish to clarify that no specific funding was received for the research conducted in this study. We extend our gratitude to the representatives of Attestiv, Fact Protocol, and Truepic for their valuable cooperation through email exchanges. We are also thankful for the indirect support provided by our institution, which facilitated this research.
The authors declare that the research was conducted in the absence of any commercial or financial relationships that could be construed as a potential conflict of interest.
The author(s) declared that they were an editorial board member of Frontiers, at the time of submission. This had no impact on the peer review process and the final decision.
All claims expressed in this article are solely those of the authors and do not necessarily represent those of their affiliated organizations, or those of the publisher, the editors and the reviewers. Any product that may be evaluated in this article, or claim that may be made by its manufacturer, is not guaranteed or endorsed by the publisher.
The Supplementary Material for this article can be found online at: https://www.frontiersin.org/articles/10.3389/fbloc.2024.1440355/full#supplementary-material
Al-Saqaf, W. (2024). Wsaqaf/blockchainai. Available at: https://github.com/wsaqaf/blockchainai.
Al-Saqaf, W., and Picha Edwardsson, M. (2019). “Could blockchain save journalism? An explorative study of blockchain’s potential to make journalism a more sustainable business,” in Blockchain and Web 3.0: social, economic, and technological challenges. Editors M. Ragnedda,, and G. Destefanis 1st ed. (London: Routledge), 97–113.
Al-Saqaf, W., and Seidler, N. (2017). Blockchain technology for social impact: opportunities and challenges ahead. J. Cyber Policy 2 (3), 338–354. doi:10.1080/23738871.2017.1400084
Anyoha, R. (2017). The history of artificial intelligence. Sci. News. Available at: https://sitn.hms.harvard.edu/flash/2017/history-artificial-intelligence/.
Bäck, J. (2023). Vad är AI för något? Internetkunskap. Available at: https://internetkunskap.se/artiklar/grundkurs-i-ai/vad-ar-ai-for-nagot/.
Buterin, V. (2015). A next generation smart contract and decentralized application platform. Available at: https://www.semanticscholar.org/paper/A-NEXT-GENERATION-SMART-CONTRACT-%26-DECENTRALIZED-Buterin/0dbb8a54ca5066b82fa086bbf5db4c54b947719a.
Buțincu, C. N., and Alexandrescu, A. (2023). Blockchain-based platform to fight disinformation using crowd wisdom and artificial intelligence. Appl. Sci. 13 (10), 6088. doi:10.3390/app13106088
Chan-Olmsted, S. M. (2019). A review of artificial intelligence adoptions in the media industry. Int. J. Media Manag. 21 (3–4), 193–215. doi:10.1080/14241277.2019.1695619
de-Lima-Santos, M. F., and Ceron, W. (2022). Artificial intelligence in news media: current perceptions and future outlook. Journalism Media 3 (1), 13–26. doi:10.3390/journalmedia3010002
Fraga-Lamas, P., and Fernández-Caramés, T. M. (2020). Fake news, disinformation, and deepfakes: leveraging distributed ledger technologies and blockchain to combat digital deception and counterfeit reality. IT Prof. 22 (2), 53–59. doi:10.1109/MITP.2020.2977589
Gupta, A., Naahal, K., Deswal, A., and Yadav, S. (2019). Critical review of blockchain technology: opportunities and challenges. Available at: https://www.semanticscholar.org/paper/Critical-Review-of-Blockchain-Technology%3A-and-Gupta-Naahal/5546260e3cfab5b2edacc10ab6d357a3acab0ea4.
Guzman, A. L., and Lewis, S. C. (2020). Artificial intelligence and communication: a Human–Machine Communication research agenda. New Media Soc. 22 (1), 70–86. doi:10.1177/1461444819858691
Jaroucheh, Z., Alissa, M., and Buchanan, W. J. (2020). “Trust-based ecosystem to combat fake news,” in 2020 IEEE International Conference on Blockchain and Cryptocurrency (ICBC), Toronto, ON, Canada, 02-06 May 2020, 1–3. doi:10.1109/ICBC48266.2020.9169435
Jenkins, H. (2006). Convergence culture: where old and new media collide. New York: NYU Press. Available at: https://www.jstor.org/stable/j.ctt9qffwr.
Karakose, T. (2023). The utility of ChatGPT in educational research—potential opportunities and pitfalls. Educ. Process Int. J. 12 (2). doi:10.22521/edupij.2023.122.1
Karlsson, M. (2019). “Journalistikens algoritmisering,” in Handbok i journalistikforskning. Editors M. Karlsson,, and J. Strömbäck (Lund: Studentlitteratur).
Kenthapadi, K., Lakkaraju, H., and Rajani, N. (2023). “Generative AI meets responsible AI: practical challenges and opportunities,” in KDD ‘23: Proceedings of the 29th ACM SIGKDD Conference on Knowledge Discovery and Data Mining, Long Beach CA USA, August 6 - 10, 2023, 5805–5806. doi:10.1145/3580305.3599557
Ki Chan, C. C., Kumar, V., Delaney, S., and Gochoo, M. (2020). “Combating deepfakes: multi-LSTM and blockchain as proof of authenticity for digital media,” in 2020 IEEE/ITU International Conference on Artificial Intelligence for Good (AI4G), Geneva, Switzerland, 21-25 September 2020, 55–62. doi:10.1109/AI4G50087.2020.9311067
Kim, B., and Yoon, Y. (2018). Journalism model based on blockchain with sharing space. Symmetry 11 (1), 19. doi:10.3390/sym11010019
Kothari, A., and Cruikshank, S. A. (2022). Artificial intelligence and journalism: an agenda for journalism research in africa. Afr. Journal. Stud. 43 (1), 17–33. doi:10.1080/23743670.2021.1999840
Küng, L. (2017). Going digital: a roadmap for organisational transformation. Oxford: Digital News Project, Reuters Institute, University of Oxford. doi:10.60625/RISJ-XCE5-7E64
Kunova, M. (2024). Blockchain can help news publishers fight risks posed by fake news websites. Media News. Available at: https://www.journalism.co.uk/news/blockchain-can-help-news-publishers-fight-risks-posed-by-fake-news-websites/s2/a1171234/.
Liu, L., Zhang, W., and Han, C. (2021). A survey for the application of blockchain technology in the media. Peer-to-Peer Netw. Appl. 14 (5), 3143–3165. doi:10.1007/s12083-021-01168-5
Lowrey, W. (2011). Institutionalism, news organizations and innovation. Journal. Stud. 12 (1), 64–79. doi:10.1080/1461670X.2010.511954
Luo, R., and Krishnamurthy, V. (2022). Mitigating misinformation spread on blockchain enabled social media networks. ArXiv. Available at: https://www.semanticscholar.org/paper/Mitigating-Misinformation-Spread-on-Blockchain-Luo-Krishnamurthy/3e38ad279dcf8d8cd5824a989e7e2b17fba922cb.
Marconi, F. (2020). Newsmakers. New York: Columbia University Press, 55–128. doi:10.7312/marc19136-005
Min, S. J., and Fink, K. (2021). Keeping up with the technologies: distressed journalistic labor in the pursuit of “shiny” technologies. Journal. Stud. 22 (14), 1987–2004. doi:10.1080/1461670X.2021.1979425
Nakamoto, S. (2008). Bitcoin: a peer-to-peer electronic cash system. Available at: https://bitcoin.org/bitcoin.pdf.
Newman, N., Fletcher, R., Robertson, C. T., Arguedas, A. R., and Nielsen, R. K. (2024). Reuters Institute digital news report 2024. Oxford: Reuters Institute for the Study of Journalism.
Nygren, G. (2019). “Journalistikens professionalisering,” in Handbok i journalistikforskning. Editors M. Karlsson, and J. Strömbäck (Lund: Studentlitteratur).
OpenAI (2023). Partnership with American Journalism Project to support local news. OpenAI.Com. Available at: https://openai.com/blog/partnership-with-american-journalism-project-to-support-local-news.
Patil, U., and Chouragade, P. M. (2021). Blockchain based approach for tackling deepfake videos. Int. J. Sci. Res. Comput. Sci. Eng. Inf. Technol., 342–347. doi:10.32628/CSEIT217372
Pavlik, J. V. (2023). Collaborating with ChatGPT: considering the implications of generative artificial intelligence for journalism and media education. Journalism and Mass Commun. Educ. 78 (1), 84–93. doi:10.1177/10776958221149577
Peña-Fernández, S., Meso-Ayerdi, K., Larrondo-Ureta, A., and Díaz-Noci, J. (2023). Without journalists, there is no journalism: the social dimension of generative artificial intelligence in the media. El Prof. La Inf., e320227. doi:10.3145/epi.2023.mar.27
Picha Edwardsson, M., and Al-Saqaf, W. (2022). Drivers and barriers for using blockchain technology to create a global fact-checking database. Online J. Commun. Media Technol. 12 (4), e202228. doi:10.30935/ojcmt/12381
Quintans-Júnior, L. J., Gurgel, R. Q., Araújo, A. A. D. S., Correia, D., and Martins-Filho, P. R. (2023). ChatGPT: the new panacea of the academic world. Rev. Soc. Bras. Med. Trop. 56, e0060–e2023. doi:10.1590/0037-8682-0060-2023
Stenbom, A., Wiggberg, M., and Norlund, T. (2023). Exploring communicative AI: reflections from a Swedish newsroom. Digit. Journal. 11 (9), 1622–1640. doi:10.1080/21670811.2021.2007781
Stray, J. (2019). Making artificial intelligence work for investigative journalism. Digit. Journal. 7 (8), 1076–1097. doi:10.1080/21670811.2019.1630289
Tsigaris, P., and Teixeira Da Silva, J. A. (2023). The role of ChatGPT in scholarly editing and publishing. Eur. Sci. Ed. 49, e101121. doi:10.3897/ese.2023.e101121
Turing, A. M. (1950). I.—computing machinery and intelligence. Mind LIX (236), 433–460. doi:10.1093/mind/LIX.236.433
Vijay, A. (2024). Norway’s VG finding success with six AI-related newsroom tools. WAN-IFRA. Available at: https://wan-ifra.org/2024/04/norways-vg-finding-success-with-six-ai-related-newsroom-tools/.
Waisbord, S. R. (2013). Reinventing professionalism: journalism and news in global perspective. Cambridge: Polity Press.
Wärnestål, P. (2023). “AI-drivna tjänster och medier,” in Tekniska mediestudier: En introduktion till metoder och teknologier. Editors M. Berg, M. Engberg, and S. Leckner (Lund: Studentlitteratur), 217–247.
Willingham, E. (2023). AI’s victories in Go inspire better human game playing. Sci. Am. Available at: https://www.scientificamerican.com/article/ais-victories-in-go-inspire-better-human-game-playing/.
Keywords: journalism, blockchain, artificial intelligence, verification, provenance, generative AI, content authentication, digital trust
Citation: Picha Edwardsson M and Al-Saqaf W (2024) Blockchain solutions for generative AI challenges in journalism. Front. Blockchain 7:1440355. doi: 10.3389/fbloc.2024.1440355
Received: 29 May 2024; Accepted: 07 November 2024;
Published: 27 November 2024.
Edited by:
Bhagwan Chowdhry, Indian School of Business, IndiaReviewed by:
Raul Zambrano, Independent Researcher, New York, United StatesCopyright © 2024 Picha Edwardsson and Al-Saqaf. This is an open-access article distributed under the terms of the Creative Commons Attribution License (CC BY). The use, distribution or reproduction in other forums is permitted, provided the original author(s) and the copyright owner(s) are credited and that the original publication in this journal is cited, in accordance with accepted academic practice. No use, distribution or reproduction is permitted which does not comply with these terms.
*Correspondence: Malin Picha Edwardsson, bWFsaW4ucGljaGEuZWR3YXJkc3NvbkBzaC5zZQ==
†These authors have contributed equally to this work
Disclaimer: All claims expressed in this article are solely those of the authors and do not necessarily represent those of their affiliated organizations, or those of the publisher, the editors and the reviewers. Any product that may be evaluated in this article or claim that may be made by its manufacturer is not guaranteed or endorsed by the publisher.
Research integrity at Frontiers
Learn more about the work of our research integrity team to safeguard the quality of each article we publish.