- 1Sistema Estudios de Posgrado, Universidad de Costa Rica, San José, Costa Rica
- 2Laboratorio de Ecología Urbana y Comunicación Animal, Escuela de Biología, Universidad de Costa Rica, San José, Costa Rica
- 3Center for Avian Population Studies, Cornell Lab of Ornithology, Cornell University, Ithaca, NY, United States
Agriculture, which is spreading rapidly, is one of the major effectors on biodiversity – generally contributing to its decline. In the past few decades, most research efforts have focused on the impact of industrial agriculture on the environment and biodiversity. However, less attention has been paid on examining the impact of sustainable agricultural management practices on biodiversity. Challenges include the disruptive nature of some practices (e.g., agrochemical application) and the timing of others (e.g., tree pruning). Here, we highlight the value of passive acoustic monitoring in assessing the impact of agricultural management practices on biodiversity, using birds as indicators. We outline key considerations, including bird ecology and behavior, ARU sampling protocols, and data management. To demonstrate our approach, we present a case study from a coffee landscape in Costa Rica, where we analyzed the effects of pruning and pesticide application over two years. By focusing on selected focal species and using a subsample of the total hours recorded in combination with a mobile app for annotations, we found that pruning negatively impacted most species, while pesticide application adversely affected all species studied. Our methodology leverages technology to evaluate the impacts of agricultural management practices, offering insights to guide and assess sustainable agricultural strategies aimed at balancing biodiversity conservation with human well-being.
Introduction
Agriculture is indispensable for meeting the global demand for food and has impacted over 38% of the Earth’s surface (Ramankutty and Foley, 1999; Ramankutty et al., 2008; Dixon et al., 2020). Currently, habitat loss and degradation as a direct result of agriculture are signaled as the main drivers of biodiversity loss (Ruiz-Gutiérrez et al., 2010; Foley et al., 2011). However, not all agricultural production methods have the same impact on the environment (Laurance et al., 2014). Large-scale monocultures (e.g., bananas, pineapple, soybeans, palm oil, rice, and sugarcane) can transform significant tracts of land, increasing soil erosion, water consumption, pollution by agrochemicals, and loss of natural habitats (Fayle et al., 2010; Obando, 2017; Carlson and Garrett, 2018; Dhandapani et al., 2019). Perennial crops (e.g., coffee, cacao) can be grown mitigating habitat degradation but often still lead to water pollution and soil erosion due to the number of agrochemicals that are often used in production (Martin-Gorriz et al., 2020). One solution to offset the impacts of agriculture is agroforestry practices, which tend to incentivize agricultural production with native vegetative cover, most often native trees, and this helps mitigate habitat loss while often having co-benefits of reduced soil erosion, higher retention of soil nutrients, increased productivity, and reduced pesticide loads (Waldron et al., 2017).
However, inherent in most agricultural practices in the tropics is a decrease in the diversity and abundance of native species, given that even the most sustainable or regenerative agricultural practices can supplement but not completely replace the resources and conditions that species need and evolved with (Quinn et al., 2014; Kross et al., 2018; Evers et al., 2018). Agriculture also often generates novel, disturbed habitats that are more easily exploited by non-native or generalist species, which can often harm native species (Stanton et al., 2018; Sandoval, 2019; Olimpi et al., 2020). Other impacts of agricultural lands related to human disturbance can also have detrimental effects on species abundance, such as noise pollution from humans or farm equipment (Reijnen et al., 1996; Francis et al., 2012; Sánchez et al., 2022).
There is a wealth of knowledge on the impact of agricultural practices on species richness and abundance, even though there are often challenges related to how to best define a control or reference site (Daehler, 1998; Hazell et al., 2004; Fischer and Lindenmayer, 2007). Agricultural landscapes are often already degraded, and it is hard to identify a true community of reference (Mortelliti et al., 2010; Lindenmayer and Fischer, 2013). The source populations for species in agricultural lands are often those that are tolerant to disturbances already unless the landscape still has a significant amount of remnant habitat patches or it has not been modified for more than a few decades through small-scale agriculture (Weibull and Östman, 2003; Scales and Marsden, 2008; Duflot et al., 2017). However, to properly assess the impact of agricultural practices on biodiversity and evaluate and guide more sustainable, regenerative practices, we need to better define the reference landscape and species community to best determine which species might be suitable for use as indicators of sustainability.
In addition to defining what species might best serve as indicators of sustainable practices or higher quality habitat for a specific agricultural sector, there are spatial and temporal aspects to consider that are related to the nature and timing of agricultural management practices. For example, when crops are perennial, only the leaves or fruits are harvested (e.g., coffee, citrus, olives, cocoa). These types of crops allow plants to be partially pruned without removing the entire vegetation cover from the farm, and wildlife can often exploit vegetation remnants (Santos and Tellería, 2006; Estrada-Carmona et al., 2019). Aside from a few studies, not much is known about how the nature and timing of agricultural management practices influence species diversity and abundance. However, this knowledge is just as critical for measuring the impact of agricultural practices on biodiversity conservation (Malone et al., 2018; Yager et al., 2023). For example, in Malaysia, the selective pruning of oil palm canopy has been shown to increase the number of bird guilds driven by increased light penetration into the understory (Azhar et al., 2013).
Monitoring the effect of agricultural management practices is more challenging relative to monitoring the impact of an agricultural practice on its own. The timing of on-the-ground agricultural management practices often varies by farm and is influenced by factors that are difficult to predict such as weather or availability of farm workers. To properly monitor the impact of agricultural management practices, constant-effort approaches would be needed to collect data before, during, and after the practice given that the timing of these events is unknown. The increased popularity of non-invasive biological monitoring methodologies has opened up many avenues of opportunity for carrying out the types of intensive monitoring efforts that would be needed to measure the impact of agricultural management practices. For example, Pelletier-Guittier et al. (2020) used camera traps to identify what factors most influence the use of hedgerows by mammals from late spring to early fall, to best inform how these should be managed in agricultural landscapes. Such inferences would be difficult to obtain using more traditional field sampling methods.
The increased popularity of automatic recording units for monitoring species with high vocal activities, such as birds, has greatly reduced the cost of conducting more intensive monitoring efforts, both temporally and spatially (Shonfield and Bayne, 2017; Pérez-Granados and Traba, 2021). The increased cost-effectiveness of automated recording units (ARUs) allows researchers to collect inordinate amounts of data from just a handful of recorders in the field (Darras et al., 2019). However, there is a trade-off between the resources needed to properly store, manage, and process such large quantities of data and the total cost of generating the same information using more traditional field methods. Here, we use a case study using ARUs for monitoring the effect of agricultural practices related to coffee production using birds as indicators. First, we will provide an outline of the main factors to consider when monitoring the effect of agricultural management practices using ARUs and birds as indicators. Second, we present a case study using avian detections collected using ARUs to evaluate the impact of two agricultural management practices in coffee farms: pruning and pesticide application. Lastly, we present a mobile application that leverages crowdsourcing as a means for ARU recordings to generate species detection data.
Factors to consider when using ARUs and birds as indicators to evaluate the impact of agricultural management practices
There are several key factors to consider when using ARUs to measure the effects of agricultural practices on biodiversity using birds as indicators. We present these factors as part of three main categories: 1) factors for selecting the focal species, 2) factors for defining the sampling scheme for the ARUs, and 3) factors for determining how to most effectively analyze the recordings.
Factors for selecting focal species
When selecting focal species, the annual cycle needs to be considered. The large majority of bird species follow a breeding season cycle (Sharp, 1996; Carey, 2009), and vocal activity can vary throughout the year driven by seasonal differences in calling rates, such as during the breeding season (Catchpole and Slater, 2003). Some species have migratory periods (latitudinal or altitudinal), which result in all or most individuals abandoning a site during part of their annual life cycle (Barcante et al., 2017). This variation needs to be considered in the context of the timing of farm agricultural management practices of interest (i.e., pruning or harvesting) in order to associate changes in calling rates directly with a specific farm management practice (for more details on seasonal transitions, please see Supplementary Materials).
Duty cycle, the proportion of time that birds vocalize during the day compared to the total time for other activities (Staicer et al., 1996), should also be considered for selecting focal species. Most bird species have two peaks, i.e., an early dawn chorus and a chorus at dusk around sunset, and not all bird species exhibit both types of choruses (Staicer et al., 1996; Bruni et al., 2014; Farina and Ceraulo, 2017). Activity peaks during the dawn and dusk chorus have also been shown to vary depending on the weather conditions, daylight, or temperature (Hutchinson, 2002; Bruni et al., 2014; Marín-Gómez and MacGregor-Fors, 2021). For example, if the morning is cold, cloudy, and with high levels of precipitation, vocal activity has been shown to be delayed for up to an hour, and the timing of ARU recordings should account for that variation. Changes in daylight will also change the timing of vocal activity, even for tropical regions (Staicer et al., 1996; Hutchinson, 2002; Bruni et al., 2014; Marín-Gómez and MacGregor-Fors, 2021).
The differences in species presence and abundance and its fluctuation during the day in agricultural environments will likely influence the detection of certain species. Detectability and vocal activity will be affected also by weather, resource availability, and disturbances (e.g., coffee harvesting) (Thomas et al., 2020; Morelli et al., 2022). Information on natural history and behavior can be used as well as the informed criteria of an expert, but in general, species that tend to be quiet or only vocalize in very specific situations may not be useful, as well as species that can be detected at distances longer than the area of study where the agricultural management practices are being evaluated.
The species selected to evaluate the effects of a given management practice should represent the main foraging guilds (e.g., frugivores, carnivores, nectarivores, or insectivores) that are likely to be influenced by the specific management practice of interest. For example, foliage gleaners are insectivores and are more likely to be affected by the application of foliage agrochemicals such as fertilizers or insecticides, relative to fertilizers that are applied on the ground (Alharbi et al., 2016). The foraging strata (e.g., canopy, lower understory, leaf litter, tree trunks, or branches) should also be taken into consideration, given that species that forage on leaf litter or coffee branches are likely to be more affected by heavy pruning for example (Dietsch et al., 2007).
The main barrier that exists currently is the high level of effort that needs to be invested to carry out all of the annotations needed to detect all the species in a community, especially considering that it has been shown that manual detection has higher accuracy and is more time-efficient, in specific cases like for species that have several song types per individual, and song types varied between individuals and geographically (Venier et al., 2017; Joshi et al., 2017; Cole et al., 2022). Therefore, selecting a small number of focal species will be more informative and more likely to yield robust inferences.
Factors for defining an optimal ARU recording protocol
The factors for defining an optimal ARU recording protocol begin by answering the question of when to record; as previously discussed, different bird species in the community are likely to have different responses to the same management practice depending on their ecology and natural history (Vickery et al., 2001; Kirk et al., 2011; Alharbi et al., 2016). If the goal of the study is to understand the responses of a couple of species to agriculture practices, the programming of ARUs should be guided by previous knowledge on the vocal activity of those species. If the focal bird species tends to vocalize mostly during the dawn chorus, limiting the recording to only the dawn chorus will save 50% of storage capacity and battery life by reducing the total recording time from 5 h/day to 3 h/day. An advantage of focusing primarily on the dawn chorus for recording is that weather conditions tend to be more stable and predictable relative to the dusk chorus and daylight hours at sunset tend to be influenced by the degree of cloudiness, ultimately reducing the total sampling period for the dusk chorus (Staicer et al., 1996). In addition, more stable temperatures and lower average wind speeds at dawn have been found to increase the sound transmission of bird vocalizations (Catchpole and Slater, 2003) (For more details on when to record and the suggested duration of the recordings, please refer to the Supplementary Material).
The number of ARUs per unit of inference is also a matter of efficiency, and one ARU deployed per plot (1,000 m2 to 2,500 m2) should be sufficient to monitor the effects of agricultural practices on bird vocal activity If agricultural management practices and conditions differ between plots (e.g., large farms), multiple plots can be monitored. Care should be taken when using more than one ARU per plot, since placing ARUs too close to one another might cause the detection of the same vocalization more than once, especially species that vocalize loudly and can be heard for long distances or species with very large territories (>1 ha). In this case, an intensity threshold can be defined to select which sounds are taken into account when analyzing the data in a spectrogram. However, if the goal is to compare two treatments (e.g., two different pesticide active ingredients applied in similar plots of the same farm), or two vegetation types in the same plot (e.g., the plot matrix and the living fence), then paired ARUs could be used to detect differences in bird activity between those treatments or vegetation types.
Factors to determine how to best analyze the recordings
Finally, factors to determine how to best analyze the recordings include acoustic data storage. Acoustic monitoring with ARUs requires a significant investment in data management that can often be challenging. The size of a small-scale deployment can easily result in terabytes of recorded audio files. As a result, compression and transfer operations can often last multiple days and require expertise in big data management (e.g., parallel processing). The sound recordings should be first backed up to a single local hard drive as WAV files directly from the SD cards of the ARUs. Second, compressing these files to FLAC 1.4.3 is recommended, a format that maintains the audio properties without data loss and allows a file size compression of up to 70%. This type of compression rate makes it an ideal format for bioacoustics because it is commonly supported by bioacoustics data processing tools (MacPhail et al., 2024). Once the data are backed up in two hard drives using the two different formats (WAV and FLAC), the SD cards can be cleared and used again.
Analyzing large datasets of acoustic recordings from ARUs can be challenging, especially when the interest is being able to detect the entire avian community (Brandes, 2008; Digby et al., 2013; Darras et al., 2019). Overall, community-level analyses can involve a combination of computational methods, signal processing techniques, machine learning algorithms, and an efficient computing system to optimize the duration of processing and analysis (Brandes, 2008; Darras et al., 2019). In addition, analyzing ARU data often involves very specialized personnel, who identify and annotate all or a set of focal bird species present in the recordings, depending on the type of analysis that will be used: a trained detection algorithm or raw annotated data (Digby et al., 2013; Sugai et al., 2019). Both of these approaches can be time-consuming and are two of the main barriers for addressing research questions using ARU data.
The general type of data analysis is an approach most commonly used when researchers are interested in detecting all species in a bird community in the study area. The first step is to preprocess raw audio recordings to enhance the quality of the data and facilitate analysis. Second, audio recordings are segmented into smaller units to facilitate data management and analysis (e.g., Brandes, 2008; Priyadarshani et al., 2018). The last step is classification (annotation and labeling), which is conducted manually or semi-automatically by annotating specific vocalizations or just the presence of a species. There is a wealth of knowledge on guidelines and challenges on the topic (e.g., Zwerts et al., 2021; Stowell, 2022) of how classification is sorted and is critical for training machine learning models to properly detect species and increase the accuracy of the overall analysis and others.
The reduced type of data analysis uses a subsampling of all recordings and only targets focal species (see below for the selection criteria of those species). For this approach, raw audio recordings are preprocessed and segmented recordings into smaller units, as above. The main difference lies in the fact that annotation and labeling are typically conducted manually and restricted to focal species vocalizations, including calls and songs. This restricted analysis can usually increase the number of potential annotators, as the level of expertise required can be lower. Automated detections can also be used since focusing on training the algorithm to detect a handful of focal species can usually be done a lot more effectively.
The abovementioned reduced analysis includes manual annotation and identification of bird detections. For this analysis, 5% of all recordings in the same type of habitat for annotation are selected. The recordings come from ARUs with the same recording effort, avoiding those that fail or do not properly record the entire sampling period. If recordings lasted 5 or fewer days, all of the recordings can be used, if not, select 5 days randomly. For the selected 5 days, 1 h per day is analyzed, usually chosen to match the duty cycle peak of the focal species of interest. For that hour, all species present are annotated. Subsequently, different time windows from that hour are selected, and the species that are present within each time window are annotated (see Table 1 for time window recommendations). The list of species detected in each time window combination is then contrasted relative to the total species that was detected during the full 1-h file. This step can help identify which time window gives more than 75% of all species detections in community detection and 100% of the focal species.
Case study: evaluating the effects of agricultural management practices in coffee farms on biodiversity using birds as indicators
We present a case study where we considered the abovementioned factors for evaluating the effect of agricultural management practices in coffee using birds as indicators. Coffee production overlaps broadly with biodiversity hotspot regions worldwide (Jha et al., 2014). The presence of birds can reduce crop damage and pest abundance while increasing crop yield in perennial crops such as coffee (Díaz-Siefer et al., 2022). Birds have also been shown to be good indicators of sustainable practices in coffee and other agricultural systems (Estrada-Carmona et al., 2019; Alvarez-Alvarez et al., 2022; Moreau et al., 2022). There is a wealth of knowledge about how different types of coffee production systems (e.g., sun coffee, shade coffee) affect biodiversity and migratory bird species in particular (Perfecto et al., 2014). However, coffee production systems are often not easily categorized as either sun or shade coffee, since there is a wide range of density of trees, and a variety of species, used as shade in coffee plots (Toledo and Moguel, 2012). Although some of these differences have been explored, a lot less attention has been paid to other practices that also impact biodiversity.
For example, the timing of harvest season in coffee farms (e.g., February and April in Costa Rica) overlaps with the timing of when North American migratory birds pass through the isthmus on their way back to their breeding grounds (February–March). Harvest season also overlaps with the beginning of the reproductive season for most Neotropical resident bird species (April–June) (Skutch, 1950). During the harvest season, several different techniques of pruning coffee plants are used (e.g., pruning the entire plot, pruning every other row of plants in a plot, pruning only the branches, or pruning the entire plant at knee level). Each pruning method is likely to have a different impact on bird species that nest on coffee plants or use the coffee plot to forage. In Costa Rica, most plots prune plants every two rows, or they do so only selectively (e.g., only the plants that were assessed as damaged). Therefore, coffee plots are never stripped of their vegetation cover completely, unlike other regions where this is more commonplace (e.g., Colombia).
Another management practice in coffee farms that is likely to impact bird species on coffee farms is the application of pesticides, which can impact bird habitat use through the mechanical act of the application itself or through the impact of the pesticides that are being used. Some of the agrochemicals that are currently used in coffee systems are known to be highly detrimental to wildlife. For example, the harmful insecticide chlorpyrifos (Table 2) which is used in Costa Rica to combat the coffee borer, Hypothenemus hampei, has the potential to bioaccumulate in bees and fish, affecting development, fertility, and survival (Thomas and Mansingh, 2002; Arroyo Rivera, 2022). This insecticide can also impact migratory bird species such as white-crowned sparrows (Zonotrichia leucophrys) by impacting their ability to orient and navigate, due to the disruption of acetylcholine transmission and other neurotoxic effects (Eng et al., 2017). Fungicides, such as pyraclostrobin and epoxiconazole, are known to be easily washed into surface waters and aquifers when rains fall, resulting in the pollution of many other ecosystems (Soares et al., 2017; Hoff et al., 2019). In addition to the known direct impacts that agrochemicals have on birds, these also eliminate many other non-pest insects that are a vital food source for many groups of birds. Therefore, our objective with this case study is to estimate changes in bird habitat use in response to pruning and the application of agrochemicals, using ARUs as a method for evaluating and informing agricultural management practices. Additionally, we aimed to test an alternative tool for the identification and annotation of bird sound: the Euphonia app. If coffee pruning and agrochemical applications negatively affect bird habitat use, we expect to observe less occurrence of bird species after pruning and agrochemical application.
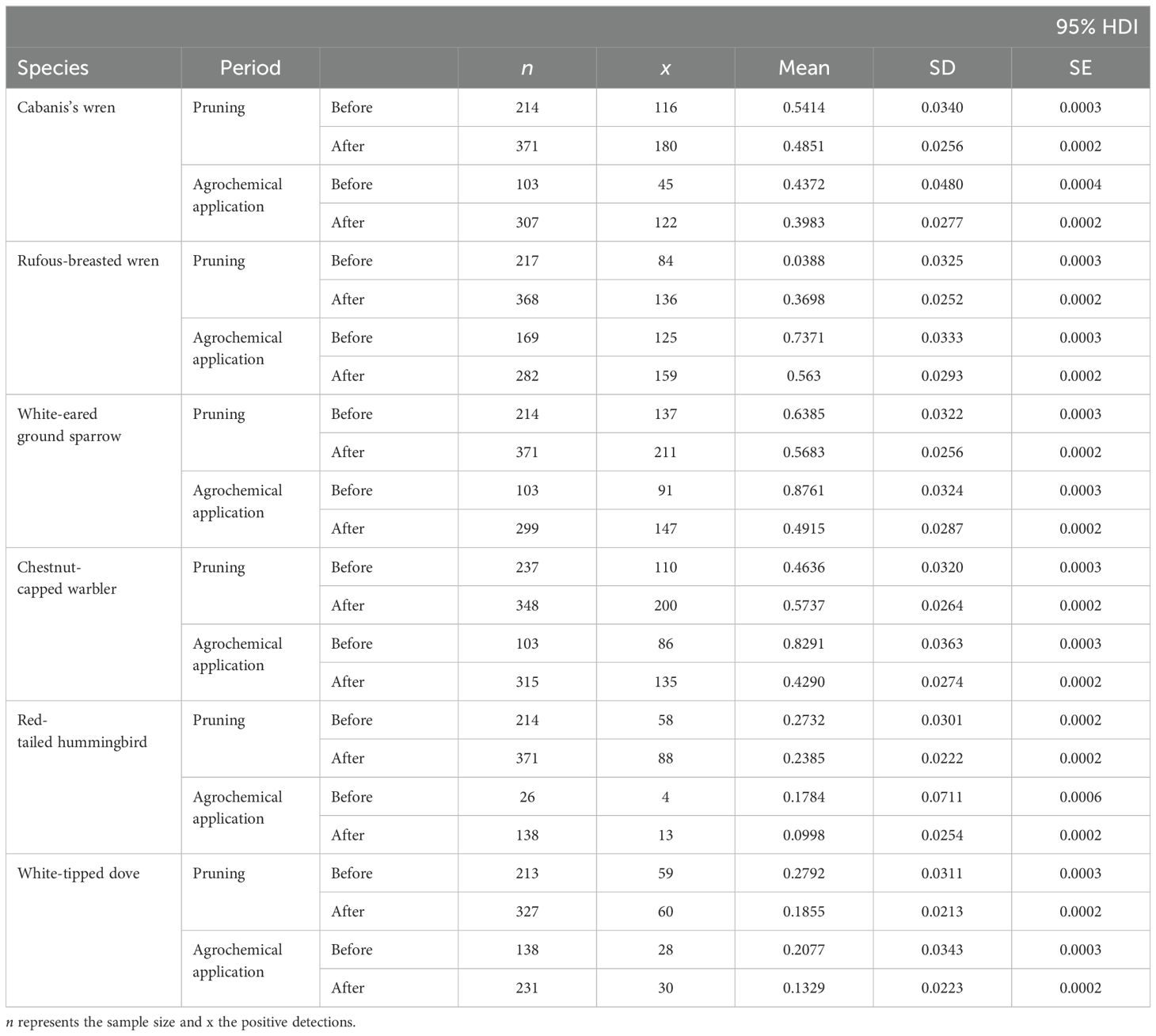
Table 2. Empirical detection mean, standard deviation, and standard error for six species of birds, before and after each deployment period.
Methodology
Study site
We carried out our study in a coffee landscape approximately 1,200 m elevation in the San Ramón Valley, Costa Rica, in 2021. All of the farms we selected were subject to similar incentives for regenerative agriculture and did not vary much in terms of elevation and other confounding factors. We selected 40 farms in this landscape using a phased stratified sampling design largely based on farm size (farm sizes are between 1 and 12.6 ha.), aboveground biomass at 30 m resolution (Baccini et al., 2012), and percentage of forest in the surrounding landscape with a resolution diameter of 25 m and an accuracy of 2.3 cm (Duncanson et al., 2020). We deployed one ARU per coffee plot per farm for all 40 farms during two 40+ day periods: 45 days between January and March during the pruning season and 41 days between July and August 2021 during the agrochemical application season.
ARU deployment
We selected one plot per farm since plots were management units within a farm and were physically divided by living fences and subjected to different agricultural management practices. A total of 40 ARUs were installed 3 m aboveground on tall trees in the middle of each plot, surrounded by a homogeneous coffee habitat (e.g., shade coffee, sun coffee, or organic coffee) of a radius of at least 50 m. To avoid border effects and disrupting noise being detected in the recorders, each plot selected was at least 200 m away from a main road and 100 m away from a secondary road. The trees in which the recorders were installed were not subject to any management practice. Each ARU was programmed to record during the morning chorus, 30 min before dawn and 3.5 h after, for a total of 4 h/day (from 4:30 to 8:30 h). To register bird vocalizations during seasons when different agricultural practices were applied, the ARUs were set to record for 45 days between January (pruning season) and March and 45 days between July and August 2021 (agrochemical application season). Farmers were asked to annotate the dates when each of the agricultural practices (e.g., pruning of coffee trees and application of agrochemicals) was conducted. The types of agrochemicals applied can be found in Table 3.
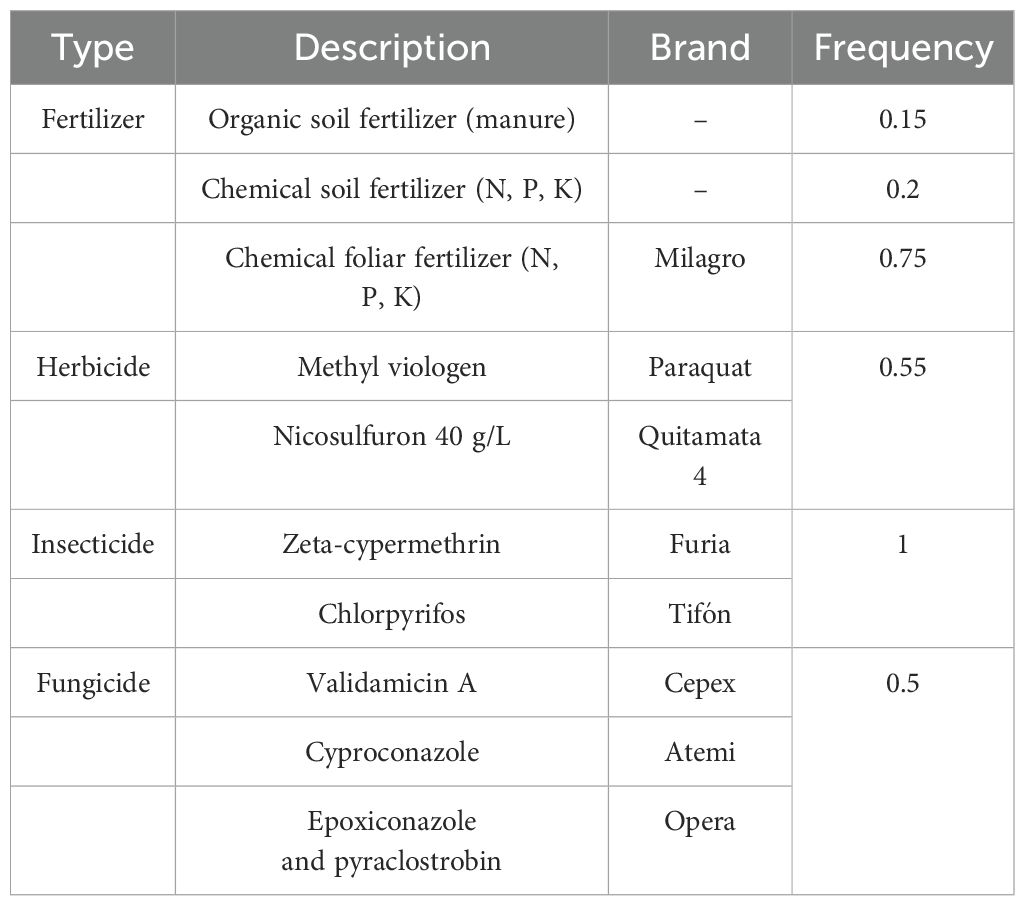
Table 3. List of agrochemicals applied during the study and the frequency they were mentioned by coffee producers.
Acoustic data storage
We developed and used the R package named soxr (Vega-Hidalgo, 2024) to compress the audio files to FLAC. We used soxr because it is executable on a per-deployment basis, instead of sox-o-matic that can only be used on a per-recorder basis (Center for Conservation Bioacoustics, Cornell Lab of Ornithology 2024). These tools not only assist in the compression process but also in the renaming of files (For more information on the storage method, see the complementary material).
Selection of focal species
Approximately 6 focal species were selected for this study, out of 40+ species detected in the base recordings, to represent the effects of agricultural management practices on avian habitat use based on the following: 1) species frequently detected in coffee plots on previous surveys that represent different foraging guilds or foraging strategies (nectarivores, granivores, insectivores, understory gleaners, and soil scratchers), 2) species detected in the recordings vocalizing within the coffee plots and have distinctive calls and songs, and 3) resident species that were going to be present year-round during the execution of the different agricultural management practices. For this case study, we selected six species that met the criteria outlined above. The selected species were as follows: 1) rufous-tailed hummingbird (Amazilia tzacatl), a nectarivore which is a current nester on coffee plants; 2) chestnut-capped warbler (Basileuterus delatrii), an insectivore known for eating the coffee berry borer beetle and frequently detected on coffee farms (Chain-Guadarrama et al., 2019); 3) white-tipped dove (Leptotila verreauxi), a species that forages by scratching the ground surface between coffee rows; 4) rufous-breasted wren (Pheugopedius rutilus), an insectivore and gleaner that is not as common; 5) white-eared ground sparrow (Melozone leucotis), a species that feeds mostly of seeds on the ground and is territorial and commonly found in thicket habitats (Stiles et al., 1989); and 6) Cabanis’s wren (Cantorchilus modestus), a common insectivore in coffee farms.
Annotation of species detection
We used the Raven Pro 1.6 sound analysis software (Cornell University Laboratory of Ornithology) to annotate each time a species was detected on a 20-min sample within each hour of recording per day (reduced analysis approach). We used the unique six-digit species code for the annotations. Only the vocalizations evident in the spectrogram were included; signals that could be barely heard in the background were excluded. If the vocalization recurred at intervals shorter than 5 s, the entire sequence of calls was recorded within a single annotation box, capturing all occurrences of the vocalization. If the vocalization could not be effectively identified as one of the focal species but is suspected to be, a question mark was placed instead. All annotators used the same Raven presets to generate and navigate spectrograms.
Additionally, we designed a mobile application called Euphonia (see Supplementary Material). This approach also allows for more than one person to collaborate on annotating a recording at a time and shows promise in engaging with local stakeholders in the annotation process. The app allows for the annotation of recordings using a mobile device, and the same presets are available anywhere you can access an internet connection. The addition of having focal species as “presets” was time-efficient since it did not require the user to enter the species code manually when saving each annotation box.
Statistical analysis
To determine if there was a significant difference in habitat use by the target bird species before and after each management practice (e.g., comparison of two proportions or A/B test), we used a Bayesian test of proportions using Bayesian First Aid and Rjags packages in the R Statistical Computing Environment (R Core Team, 2023). The test of proportions is a robust approach that allows us to test for differences in habitat use, even when there are differences in sampling effort (Keysers et al., 2020). The test of proportions estimates the probability of an event (e.g., posterior mean) and 95% credibility interval. To run the test of proportions, we created a species-specific matrix of detection and non-detection events before (A) and after (B) each agricultural practice. We then estimated the posterior mean of the probability of detecting a species (Θ) in a coffee plot before (Θ1) or after (Θ2) the management intervention. We generated 5,000 MCMC samples for each test and presented the posterior mean and 95% credibility interval. We also estimated the difference between the posterior means (Θ1 − Θ2) to estimate the magnitude of the change in the probability of detecting a species, and the % value is the proportion of posterior estimates that are higher in a coffee plot before (posterior distribution of Θ1) the management practice (posterior distribution of Θ2), which can be interpreted as the probability that the management practice negatively influenced habitat use by a species, expressed as a percentage.
Results
We obtained 13,760 h of recordings during two sample periods and analyzed a subset of 1,720 h as per the reduced analysis approach (above). By reducing the data to be analyzed to 12.5% of all collected data, we increased the feasibility of the annotation process. The annotation process still amounted to approximately 1,590 h of annotations using Raven Pro. Our successful use of the Euphonia mobile application for annotations resulted in a reduction of total annotation time and divided the annotation into smaller periods of effort, achieving a more efficient use of time. Our results on the probability of detecting the focal species before and after the management practice showed a prevalent negative effect for most species for pruning and a more consistent negative effect for pesticide application (Figures 1, 2). We focused on the detection/non-detection of species before and after agricultural practices which means that our results are not affected by the number of vocalizations for each species, but our ability to detect an effect could be influenced by the sample size for each species. For example, the rufous-tailed hummingbird was detected in only four ARUs before the application of agrochemicals (Table 2); however, there is an 82% probability that there was an effect on this species (Figure 2). White-eared ground sparrows had the highest number of detections out of all focal species during all sampling events (Table 2).
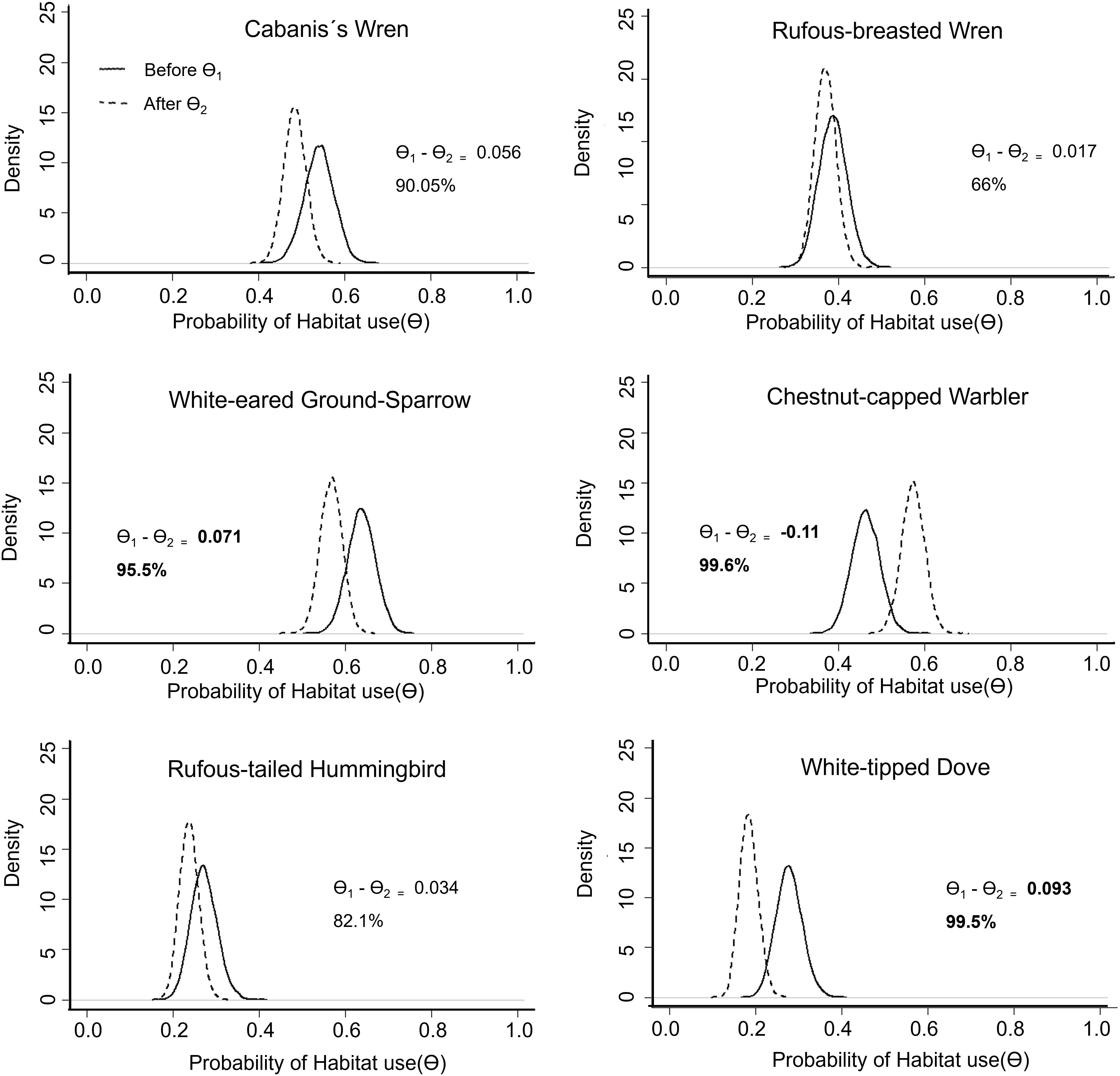
Figure 1. Density curve of the posterior distribution of the probability of habitat use (Θ) during the pruning season for each one of the six species of birds. Θ1 corresponds to the “before” values in a solid line and Θ2 to the “after” values in a dotted line. The difference in posterior mean values (Θ1 − Θ2) represents the magnitude of the change in the probability of detecting a species, and the % value is the proportion of posterior estimates that differ in Θ1 relative to Θ2, which can be interpreted as the probability that pruning did reduce or increased habitat use by a species.
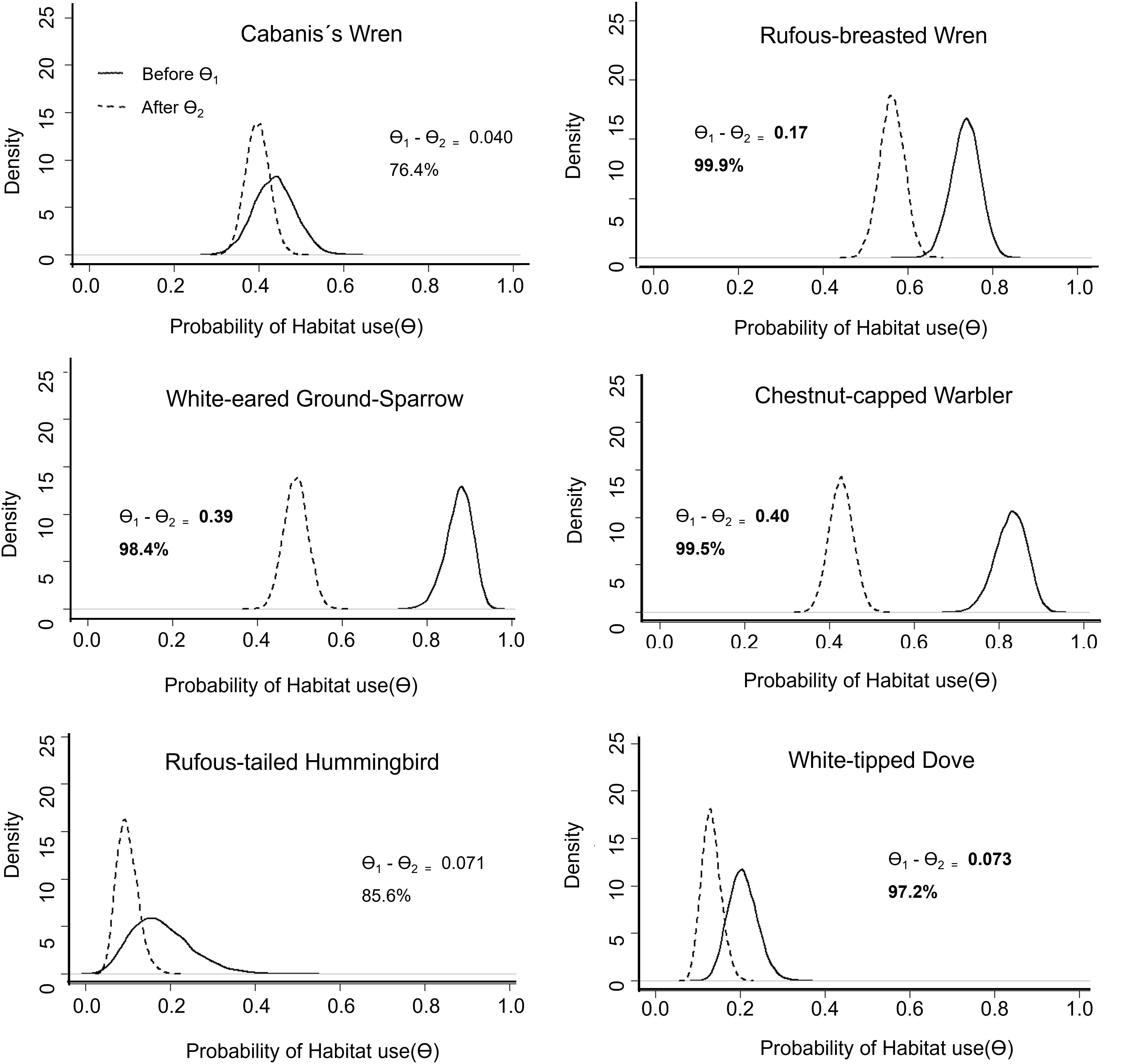
Figure 2. Density curve of the posterior distribution of the probability of habitat use (Θ) during the application of agrochemicals season for each one of the six species of birds. Θ1 corresponds to the “before” values in a solid line and Θ2 to the “after” values in a dotted line. The difference in posterior mean values (Θ1 − Θ2) represents the magnitude of the change in the probability of detecting a species, and the % value is the proportion of posterior estimates that differ in Θ1 relative to Θ2, which can be interpreted as the probability that pesticide application did reduce or increased habitat use by a species.
The estimated probability of habitat use (Θ) was lower after pruning for most species, except for the chestnut-capped warbler. For the Cabanis’s wren, white-eared ground sparrow, and white-tipped dove, the posterior means of the probability of habitat use before pruning (Θ1) were higher than the probability of habitat use after pruning (Θ2) with all probabilities of this effect above 90% (Figure 1). Rufous-breasted wren and rufous-tailed hummingbird had lower values, but the probabilities of the effect were lower (66% and 82%, respectively). For the chestnut-capped warbler, the probability of habitat use after pruning (Θ2) was higher than Θ1 (before pruning), with a 99.6% probability of this effect (Figure 1).
The estimated probability of habitat use (Θ) was lower after the application of agrochemicals for all species. For the rufous-breasted wren, white-eared ground sparrow, and chestnut-capped warbler, the differences in posterior means (Θ1 − Θ2) were much higher in magnitude relative to the effects we saw for pruning (Figure 2). For the rufous-breasted wren, white-eared ground sparrow, chestnut-capped warbler, and white-tipped dove, the posterior mean for the probability of habitat use before the pesticide application (Θ1) was higher for all species, with probabilities of 98% or higher. Rufous-tailed hummingbird was detected only in four of the 20 recorders during the second season, and the effect was still suggestive, but the probability of the effect was lower (85%). This type of visualization (Figures 1, 2) is easy to explain to stakeholders and, as opposed to frequentist approaches, easier to interpret by using probabilities of habitat use and of finding a difference, relative to basing inferences on p-values alone.
Discussion
Implications for agricultural management practices
We were able to evaluate the effect of two key coffee agricultural management practices on biodiversity—pruning and the application of agrochemicals, through the use of ARUs and using focal bird species as indicators. The six focal species showed different responses to both practices inside coffee farms, representing the complexity of bird community responses to coffee production. White-eared ground sparrow and chestnut-capped warbler are species that forage within the coffee plots (Stiles et al., 1989; Sandoval and Mennill, 2012) and are the two species that showed the greatest decrease in estimates of probability of habitat use (Θ) after the application of agrochemicals. Chestnut-capped warbler forages on the coffee plant branches looking for insects (Stiles et al., 1989); meanwhile, white-eared ground sparrow feeds mostly on seeds and small insects on the ground (Stiles et al., 1989; Sandoval and Mennill, 2012; Juárez et al., 2020). Our results indicate that habitat use by both species is likely to significantly decrease after the application of agrochemicals and is likely driven by the decline of insect abundance after the application of insecticides (BioQuim, 2015) and other chemicals (Table 3) that are toxic and irritating to humans and other animals.
White-eared ground sparrows and white-tipped doves showed a decrease in the estimates of probability of habitat use (Θ) after pruning. Vegetation density is often reduced in coffee plots after pruning, and most leaves and branches are commonly left on the ground. Given that both species forage mostly on the ground looking for seeds (Stiles et al., 1989), this practice increases the obstacles for foraging on the ground and opens the vegetation coverage, exposing these species to a more open area, leading birds to search for food in other sites. However, for the chestnut-capped warbler, probability (Θ) values are higher after pruning. This warbler often forages for insects and perches within the coffee branches; hence, the elimination of coffee branches may cause a disturbance on its foraging behavior indirectly affecting its detection on the recorders.
The rufous-breasted wren, which prefers tangled vines and scrubs at the coffee plot edges, showed similar estimates of habitat use before and after pruning but decreased after the application of agrochemicals. Conversely, Cabanis’s wren, a species that moves between the coffee plants and the surrounding bushes, live fences, and riparian forests (Stiles et al., 1989), showed similar detection before and after the application of agrochemicals and pruning. Rufous-tailed hummingbirds showed similar probability estimates (Θ) before and after pruning and a slight decrease after agrochemical applications, but it was not conclusive. This lack of an effect might be driven by a reduction in the ability to detect this hummingbird due to the territorial behavior around flower patches (Stiles et al., 1989). Lower patches are not that common within the coffee plots, and the blooming of coffee plants in this area is between April and June (a period not included in the recordings). In summary, our results showed that both agricultural management practices impact the ability of coffee farms to serve as supplemental habitats for the focal bird species and likely the avian community as a whole.
The comparison of the probability of each event aided in communicating these results with coffee producers and other stakeholders, as evidence of the effect that different agricultural management practices have on biodiversity in coffee farms. This is crucial especially for the chemicals reported (Table 3) such as the fungicide epoxiconazole, the use of which has been debated in Europe since 1990s, due to its high toxicity in water environments and hazardous effects on humans (European Commission, 2022; Cocco, 2002) and other vertebrates (Zhang et al., 2018), and it is used in Costa Rican coffee farms under government approval (Barquero, 2013; Ministerio de Agricultura y Ganadería, 2022).
Based on our results on six species that represent foraging guilds of common birds in coffee farms, we provided the following management suggestions: 1) consider using selective pruning or systematic cyclical pruning to maintain the structural complexity of the farm relative to pruning the entire coffee plot or farm (Azhar et al., 2013); 2) consider pruning coffee farms before April or after June, to avoid the peak of the breeding season for many resident bird species (Skutch, 1950), including the breeding peak for the rufous-tailed hummingbird (Lindell and Smith, 2003); 3) refrain from using herbicides to control weeds on access trails and trails running between coffee plots and use mowing as an alternative; and 4) use fungicides and fertilizers approved for sustainable practices and in the recommended amounts (Fuhrimann et al., 2019).
The potential for crowdsourcing data analysis in ARUs
Our use of the Euphonia mobile application shows promise in the potential leveraging of technology for community-driven bioacoustic annotations. This approach shows promise in leveraging local expertise with passive acoustic monitoring (PAM), thus deepening our understanding of biodiversity. This process of engaging with local communities could also help with establishing collective conservation goals and motivate community members to engage in bioacoustic annotation, a step that is especially important in tropical regions where species lists are not yet comprehensively supported by AI models for bioacoustics species identification (Sun et al., 2022). The invaluable local knowledge in species identification, when potentially incentivized, has been shown to serve as a cornerstone of conservation efforts (McElwee et al., 2020).
More importantly, integrating communities into conservation planning promotes the dissemination of conservation ethics and trust in its intrinsic value (Cetas and Yasué, 2017; Armitage et al., 2020). While AI-assisted species identification in apps like Merlin and BirdNET, developed by the Cornell Lab of Ornithology, represents significant progress by facilitating people’s interaction with the sounds of birds that surround them, these tools are currently primarily outreach and learning tools that help observers identify species in real time. However, mobilizing collective action through shared local bioacoustic species identification and annotation via a mobile app represents an exciting and novel path. Quantifying the potential efficiency boost for ARU projects and evaluating its usability by non-scientific audiences will provide guidance for the development toward an increase in inclusivity and efficiency in bioacoustics projects.
Conclusions
Although a wealth of knowledge exists on how to use ARUs, species recognition, and data analysis of those methods in recent years (Brandes, 2008; Digby et al., 2013; Darras et al., 2019; Sugai et al., 2019), our suggestions and case study provide a roadmap for effectively using ARUs for monitoring the effect of agricultural practices using birds as indicators. We discuss and compare how to maximize recording storage and energy on ARUs by recording only during the dawn chorus and the importance of knowing the seasonal and vocal diel patterns for the vocalizations of focal bird species. We also present a method to reduce the amount of time required for extracting species detection from the recordings for focal species although the recordings included all the bird community (Shonfield and Bayne, 2017; Drake et al., 2021). This method also allowed for testing the potential for crowdsourcing collaborations through apps such as Euphonia, given that the number of species for recognition is smaller, and consequently, the vocal learning recognition is easy and more feasible for non-expert collaborators (e.g., producers or volunteers). Finally, we provided recommendations on how ARU data on birds can be analyzed to facilitate communicating with decision-makers and coffee producers given the robust yet simple approach for data analysis.
As the demand to measure and mitigate the impact of food production steadily increases, from governments to producers and consumers, having a flexible approach that is applicable to multiple types of farm production is what allows this type of monitoring to be scaled across productive systems and regions. Our approach further increases the cost-effectiveness of such monitoring by addressing one of the main barriers for investing in monitoring birds—how to properly store data, and reducing the time needed to extract data from the recordings. We recommend that this approach be applied to other production systems such as cocoa, sugarcane, rice, pineapple, vineyards, olives, tobacco, and systems for vegetable production.
Data availability statement
The raw data supporting the conclusions of this article will be made available by the authors, without undue reservation.
Ethics statement
Ethical approval was not required for the study involving animals in accordance with the local legislation and institutional requirements because it was a passive acoustic monitoring (acoustic recording) without capture or manipulation of the studied birds.
Author contributions
IM-M: Data curation, Formal analysis, Investigation, Methodology, Writing – original draft. VR-G: Conceptualization, Data curation, Formal analysis, Funding acquisition, Methodology, Supervision, Writing – original draft. ÁV-H: Methodology, Software, Writing – original draft. LS: Conceptualization, Supervision, Writing – original draft.
Funding
The author(s) declare financial support was received for the research, authorship, and/or publication of this article. The K. Lisa Yang Center for Conservation Bioacoustics partially funded Euphonia app development. The Nespresso AAA Sustainable Quality™ Program supported all field work. LS was supported by Vicerectoría de investigación, Universidad de Costa Rica under project numbers C2705, C3025, and C3116. The Cornell Lab of Ornithology funded the publication fees. IM-M and all field work was supported by the Nespresso AAA Sustainable Quality™ Program. The funders were not involved in the study design, collection, analysis, interpretation of data, the writing of this article, or the decision to submit it for publication.
Acknowledgments
We thank each of the producers who allow us to set up the recordings in their farms and provide us with a detailed description of the practices and products they applied. Through the collaborative project “Our Coffee Our Birds” between The Cornell Lab of Ornithology and Nespresso, we were able to explore the relation between birds and agricultural practices. We thank Alejando Quesada who helped identify the birds and test the app via annotations, which were key to the development of the study. We gratefully acknowledge the K. Lisa Yang Center for Conservation Bioacoustics for their generous funding in support of the Euphonia app development. Special thanks to Laurel Symes and Holger Klinck for their invaluable feedback and ongoing support. We extend our gratitude to the Centro Nacional de Alta Tecnología (CENAT) of Costa Rica for their invaluable support. In particular, we thank Carlos Gamboa for his exceptional assistance with the IT requirements necessary for the efficient transfer and backup of big data.
Conflict of interest
The authors declare that the research was conducted in the absence of any commercial or financial relationships that could be construed as a potential conflict of interest.
The author LS declared that they were an editorial board member of Frontiers, at the time of submission. This had no impact on the peer review process and the final decision.
Publisher’s note
All claims expressed in this article are solely those of the authors and do not necessarily represent those of their affiliated organizations, or those of the publisher, the editors and the reviewers. Any product that may be evaluated in this article, or claim that may be made by its manufacturer, is not guaranteed or endorsed by the publisher.
Supplementary material
The Supplementary Material for this article can be found online at: https://www.frontiersin.org/articles/10.3389/fbirs.2024.1386759/full#supplementary-material
References
Alharbi H. A., Letcher R. J., Mineau P., Chen D., Chu S. (2016). Organophosphate pesticide method development and presence of chlorpyrifos in the feet of nearctic-neotropical migratory songbirds from Canada that over-winter in Central America agricultural areas. Chemosphere 144, 827–835. doi: 10.1016/j.chemosphere.2015.09.052
Alvarez-Alvarez E. A., Almazán-Núñez R. C., Corcuera P., González-García F., Brito-Millán M., Alvarado-Castro V. M. (2022). Land use cover changes the bird distribution and functional groups at the local and landscape level in a Mexican shaded-coffee agroforestry system. Agricult. Ecosyst. Environ. 330, 107882. doi: 10.1016/j.agee.2022.107882
Armitage D., Mbatha P., Muhl E. K., Rice W., Sowman M. (2020). Governance principles for community-centered conservation in the post-2020 global biodiversity framework. Conserv. Sci. Pract. 2, e160. doi: 10.1111/csp2.160
Arroyo Rivera C. (2022). Evaluación de la acumulación del insecticida clorpirifos en abejas domésticas (Apis mellifera) suministrado vía oral crónica, mediante métodos analíticos basados en cromatografía líquida de alta resolución. (Santiado de Chile: Especialidad en Química, Universidad de Chile).
(2015). Clorpirifos 5GR; MSDS No.PR-HS-PT-057 (San José CR: BioQuim). Available online at: https://guides.lib.monash.edu/c.php?g=219786&p=5937317 (Accessed Apr. 18, 2024).
Azhar B., Lindenmayer D. B., Wood J., Fischer J., Manning A., Mcelhinny C., et al. (2013). The influence of agricultural system, stand structural complexity and landscape context on foraging birds in oil palm landscapes. Ibis 155, 297–312. doi: 10.1111/ibi.12025
Baccini A. G. S. J., Goetz S. J., Walker W. S., Laporte N. T., Sun M., Sulla-Menashe D., et al. (2012). Estimated carbon dioxide emissions from tropical deforestation improved by carbon-density maps. Nat. Climate Change 2, 182–185. doi: 10.1038/nclimate1354
Barcante L., Vale M., S. Alves M. A. (2017). Altitudinal migration by birds: a review of the literature and a comprehensive list of species. J. Field Ornithology 88 (4), 321–335. doi: 10.1111/j.1466-8238.2007.00287.x
Barquero M. (2013). Recomendaciones para el combate de la roya en el cafeto (Instituto Nacional del Café). Available at: https://www.researchgate.net/publication/281625030_Recomendaciones_para_el_combate_de_la_roya_del_cafeto.
Brandes T. S. (2008). Automated sound recording and analysis techniques for bird surveys and conservation. Bird Conserv. Int. 18, S163–S173. doi: 10.1017/S0959270908000415
Bruni A., Mennill D. J., Foote J. R. (2014). Dawn chorus start time variation in a temperate bird community: relationships with seasonality, weather, and ambient light. J. Ornithol. 155, 877–890. doi: 10.1007/s10336-014-1071-7
Carey C. (2009). The impacts of climate change on the annual cycles of birds. Philos. Trans. R. Soc. B 364, 3321–3330. doi: 10.1098/rstb.2009.0182
Carlson K. M., Garrett R. D. (2018).Environmental impacts of tropical soybean and palm oil crops. In: Oxford Research Encyclopedia of Environmental Science (Accessed March 2020).
Catchpole C. K., Slater P. J. (2003). Bird song: biological themes and variations (Cambridge, United Kingdom: Cambridge university press).
Cetas E. R., Yasué M. (2017). A systematic review of motivational values and conservation success in and around protected areas. Conserv. Biol. 31, 203–212. doi: 10.1111/cobi.12770
Chain-Guadarrama A., Martínez-Salinas A., Aristizábal N., Ricketts T. H. (2019). Ecosystem services by birds and bees to coffee in a changing climate: A review of coffee berry borer control and pollination. Agricult. Ecosyst. Environ. 280, 53–67. doi: 10.1016/j.agee.2019.04.011
Cocco P. (2002). On the rumors about the silent spring: review of the scientific evidence linking occupational and environmental pesticide exposure to endocrine disruption health effects. Caderno Saúde Pública. 18, 379–402. doi: 10.1590/S0102-311X2002000200003
Cole J. S., Michel N. L., Emerson S. A., Siegel R. B. (2022). Automated bird sound classifications of long-duration recordings produce occupancy model outputs similar to manually annotated data. Ornithological Appl. 124 (2), duac003. doi: 10.1093/ornithapp/duac003
Daehler C. C. (1998). The taxonomic distribution of invasive angiosperm plants: ecological insights and comparison to agricultural weeds. Biol. Conserv. 84, 167–180. doi: 10.1016/S0006-3207(97)00096-7
Darras K., Batáry P., Furnas B. J., Grass I., Mulyani Y. A., Tscharntke T. (2019). Autonomous sound recording outperforms human observation for sampling birds: a systematic map and user guide. Ecol. Appl. 29, e01954. doi: 10.1002/eap.1954
Dhandapani S., Ritz K., Evers S., Sjögersten S. (2019). Environmental impacts as affected by different oil palm cropping systems in tropical peatlands. Agricult. Ecosyst. Environ. 276, 8–20. doi: 10.1016/j.agee.2019.02.012
Díaz-Siefer P., Olmos-Moya N., Fontúrbel F. E., Lavandero , Pozo B. R., Celis-Diez J. (2022). Bird-mediated effects of pest control services on crop productivity: a global synthesis. J. Pest Sci. 95, 567–576. doi: 10.1007/s10340-021-01438-4
Dietsch T. V., Perfecto I., Greenberg R. (2007). Avian foraging behavior in two different types of coffee agroecosystem in Chiapas, Mexico. Biotropica 39, 232–240. doi: 10.1111/j.1744-7429.2006.00248.x
Digby A., Towsey M., Bell B. D., Teal P. D. (2013). A practical comparison of manual and autonomous methods for acoustic monitoring. Methods Ecol. Evol. 4, 675–683. doi: 10.1111/2041-210X.12060
Dixon A. P., Baker M. E., Ellis E. C. (2020). Agricultural landscape composition linked with acoustic measures of avian diversity. Land 9, 145. doi: 10.3390/land9050145
Drake A., de Zwaan D. R., Altamirano T. A., Wilson S., Hick K., Bravo C., et al. (2021). Combining point counts and autonomous recording units improves avian survey efficacy across elevational gradients on two continents. Ecol. Evol. 11, 8654–8682. doi: 10.1002/ece3.7678
Duflot R., Ernoult A., Aviron S., Fahrig L., Burel F. (2017). Relative effects of landscape composition and configuration on multi-habitat gamma diversity in agricultural landscapes. Agricult. Ecosyst. Environ. 241, 62–69. doi: 10.1016/j.agee.2017.02.035
Duncanson L., Neuenschwander A., Hancock S., Thomas N., Fatoyinbo T., Simard M., et al. (2020). Biomass estimation from simulated GEDI, ICESat-2 and NISAR across environmental gradients in Sonoma County, California. Remote Sens. Environ. 242, 111779. doi: 10.1016/j.rse.2020.111779
Eng M. L., Stutchbury B. J., Morrissey C. A. (2017). Imidacloprid and chlorpyrifos insecticides impair migratory ability in a seed-eating songbird. Sci. Rep. 7, 1–9. doi: 10.1038/s41598-017-15446-x
Estrada-Carmona N., Martínez-Salinas A., DeClerck F. A. J., Vílchez-Mendoza S., Garbach K. (2019). Managing the farmscape for connectivity increases conservation value for tropical bird species with different forest-dependencies. J. Environ. Manage. 250, 109504. doi: 10.1016/j.jenvman.2019.109504
European Commission (2022). Amending Regulation (EU) No 649/2012 of the European Parliament and of the Council as regards the listing of pesticides, industrial chemicals, persistent organic pollutants and mercury and an update of customs codes. Official Journal of the European Union.
Evers C. R., Wardropper C. B., Branoff B., Granek E. F., Hirsch S. L., Link T. E., et al. (2018). The ecosystem services and biodiversity of novel ecosystems: A literature review. Global Ecol. Conserv. 13, e00362. doi: 10.1016/j.gecco.2017.e00362
Farina A., Ceraulo M. (2017). “The acoustic chorus and its ecological significance,” in Ecoacoustics: The Ecological Role of Sounds. Eds. Farina A., Gage S. H. (Oxford UK: John Wiley and Sons), 81–94.
Fayle T. M., Turner E. C., Snaddon J. L., Chey V. K., Chung A. Y., Eggleton P., et al. (2010). Oil palm expansion into rain forest greatly reduces ant biodiversity in canopy, epiphytes and leaf-litter. Basic Appl. Ecol. 11, 337–345. doi: 10.1016/j.baae.2009.12.009
Fischer J., Lindenmayer D. B. (2007). Landscape modification and habitat fragmentation: a synthesis. Global Ecol. biogeography 16 (3), 265–280. doi: 10.1111/jofo.12234
Foley J. A., Ramankutty N., Brauman K. A., Cassidy E. S., Gerber J. S., Johnston M., et al. (2011). Solutions for a cultivated planet. Nature 478, 337–342. doi: 10.1038/nature10452
Francis C. D., Kleist N. J., Ortega C. P., Cruz A. (2012). Noise pollution alters ecological services: enhanced pollination and disrupted seed dispersal. Proc. R. Soc. B 279, 2727–2735. doi: 10.1098/rspb.2012.0230
Fuhrimann S., Winkler M. S., Staudacher P., Weiss F. T., Stamm C., Eggen R. I., et al. (2019). Exposure to pesticides and health effects on farm owners and workers from conventional and organic agricultural farms in costa rica: protocol for a cross-sectional study. JMIR Res. Protoc. 8 (1), e10914. doi: 10.2196/10914
Hazell D., Hero J. M., Lindenmayer D., Cunningham R. (2004). A comparison of constructed and natural habitat for frog conservation in an Australian agricultural landscape. Biol. Conserv. 119, 61–71. doi: 10.1016/j.biocon.2003.10.022
Hoff R., Furtado R., Dos Santos J. M., Vogelmann E. S., Hoff G. D., Kneip R. C., et al. (2019). Removal of epoxiconazole and pyraclostrobin from highly contaminated effluent (grams per liter level): Comparison between ozone and solar still decontamination using real field conditions. Sci. Total Environ. 653, 597–604. doi: 10.1016/j.scitotenv.2018.11.043
Hutchinson J. M. (2002). Two explanations of the dawn chorus compared: how monotonically changing light levels favour a short break from singing. Anim. Behav. 64 (4), 527–539. doi: 10.1006/anbe.2002.3091
Jha S., Bacon C. M., Philpott S. M., Ernesto Méndez V., Läderach P., Rice R. A. (2014). Shade coffee: update on a disappearing refuge for biodiversity. BioScience 64, 416–428. doi: 10.1093/biosci/biu038
Joshi K. A., Mulder R. A., Rowe K. M. (2017). Comparing manual and automated species recognition in the detection of four common south-east australian forest birds from digital field recordings. Emu-Austral Ornithology 117 (3), 233–246. doi: 10.1080/01584197.2017.1298970
Juárez R., Chacón-Madrigal E., Sandoval L. (2020). Urbanization has opposite effects on the territory size of two passerine birds. Avian Res. 11, 1–9. doi: 10.1186/s40657-020-00198-6
Keysers C., Gazzola V., Wagenmakers E.-J. (2020). Using Bayes factor hypothesis testing in neuroscience to establish evidence of absence. Nat. Neurosci. 23, 788–799. doi: 10.1038/s41593-020-0660-4
Kirk D., Lindsay K., Brook R. (2011). Risk of agricultural practices and habitat change to farmland birds. Avian Conserv. Ecol. 6. doi: 10.5751/ACE-00446-060105
Kross S. M., Ingram K. P., Long R. F., Niles M. T. (2018). Farmer perceptions and behaviors related to wildlife and on-farm conservation actions. Conserv. Lett. 11 (1), e12364.
Laurance W. F., Sayer J., Cassman K. G. (2014). Agricultural expansion and its impacts on tropical nature. Trends Ecol. Evol. 29 (2), 107–116. doi: 10.1016/j.tree.2013.12.001
Lindell C., Smith M. (2003). Nesting bird species in sun coffee, pasture, and understory forest in southern costa rica. Biodiversity Conserv. 12, 423–440.
Lindenmayer D. B., Fischer J. (2013). Habitat fragmentation and landscape change: an ecological and conservation synthesis (Washinton DC, United States of America: Island Press).
MacPhail A. G., Yip D. A., Knight E. C., Hedley R., Knaggs M., Shonfield J., et al. (2024). Audio data compression affects acoustic indices and reduces detections of birds by human listening and automated recognisers. Bioacoustics 33 (1), 74–90. doi: 10.1080/09524622.2023.2290718
Malone L. A., Burgess E. P., Barraclough E. I., Poulton J., Todd J. H. (2018). Invertebrate biodiversity in apple orchards: agrichemical sprays as explanatory variables for inter-orchard community differences. Agric. For. Entomol. 20, 380–389. doi: 10.1111/afe.12269
Marín-Gómez O. H., MacGregor-Fors I. (2021). A global synthesis of the impacts of urbanization on bird dawn choruses. Ibis 163, 1133–1154. doi: 10.1111/ibi.12949
Martin-Gorriz B., Gallego-Elvira B., Martínez-Alvarez V., Maestre-Valero J. F. (2020). Life cycle assessment of fruit and vegetable production in the Region of Murcia (south-east Spain) and evaluation of impact mitigation practices. J. Clean. Product. 265, 121656. doi: 10.1016/j.jclepro.2020.121656
McElwee P., Fernández-Llamazares Á., Aumeeruddy-Thomas Y., Babai D., Bates P., Galvin K., et al. (2020). Working with Indigenous and local knowledge (ILK) in large-scale ecological assessments: Reviewing the experience of the IPBES Global Assessment. J. Appl. Ecol. 57, 1666–1676. doi: 10.1111/1365-2664.13705
Ministerio de Agricultura y Ganadería. (2022). Registro de plaguicidas. ministerio de agricultura y ganadería. Available at: https://app.sfe.go.cr/SFEInsumos/aspx/Insumos/ConsultaRegistroPlaguicida.aspx.
Moreau J., Rabdeau J., Badenhausser I., Giraudeau M., Sepp T., Crépin M., et al. (2022). Pesticide impacts on avian species with special reference to farmland birds: a review. Environ. Monit. Assess. 194, 790. doi: 10.1007/s10661-022-10394-0
Morelli F., Brlík V., Benedetti Y., Bussière R., Moudrá L., Reif J., et al. (2022). Detection rate of bird species and what it depends on: Tips for field surveys. Front. Ecol. Evol. 9, 671492. doi: 10.3389/fevo.2021.671492
Mortelliti A., Amori G., Boitani L. (2010). The role of habitat quality in fragmented landscapes: a conceptual overview and prospectus for future research. Oecologia 163. doi: 10.3389/fevo.2021.671492
Obando A. A. (2017). El estado detrás de la piña: el conflicto socioambiental del monocultivo de piña en los cantones de upala, guatuso y los chiles. Tesis Grado Universidad Costa Rica.
Olimpi E. M., Garcia K., Gonthier D. J., De Master K. T., Echeverri A., Kremen C., et al. (2020). Shifts in species interactions and farming contexts mediate net effects of birds in agroecosystems. Ecol. Appl. 30, e02115. doi: 10.1002/eap.2115
Pelletier-Guittier C., Théau J., Dupras J. (2020). Use of hedgerows by mammals in an intensive agricultural landscape. Agricult. Ecosyst. Environ. 302, 107079. doi: 10.1016/j.agee.2020.107079
Pérez-Granados C., Traba J. (2021). Estimating bird density using passive acoustic monitoring: a review of methods and suggestions for further research. Ibis 163, 765–783. doi: 10.1111/ibi.12944
Perfecto I., Vandermeer J., Philpott S. M. (2014). Complex ecological interactions in the coffee agroecosystem. Annu. Rev. Ecol. Evol. System. 45, 137–158. doi: 10.1146/annurev-ecolsys-120213-091923
Priyadarshani N., Marsland S., Castro I. (2018). Automated birdsong recognition in complex acoustic environments: a review. J. Avian Biol. 49, jav–01447. doi: 10.1111/jav.01447
Quinn J. E., Johnson R. J., Brandle J. R. (2014). Identifying opportunities for conservation embedded in cropland anthromes. Landscape Ecol. 29, 1811–1819. doi: 10.1007/s10980-014-0098-8
R Core Team. (2023). R: A language and environment for statistical computing (Vienna, Austria: R Foundation for Statistical Computing). Available at: https://www.R-project.org/.
Ramankutty N., Evan A. T., Monfreda C., Foley J. A. (2008). Farming the planet: 1. Geographic distribution of global agricultural lands in the year 2000. Global biogeochem. cycles 22. doi: 10.1029/2007GB002952
Ramankutty N., Foley J. A. (1999). Estimating historical changes in global land cover: Croplands from 1700 to 1992. Global biogeochem. cycles 13, 997–1027. doi: 10.1029/1999GB900046
Reijnen R., Foppen R., Meeuwsen H. (1996). The effects of traffic on the density of breeding birds in Dutch agricultural grasslands. Biol. Conserv. 75, 255–260. doi: 10.1016/0006-3207(95)00074-7
Ruiz-Gutiérrez V., Zipkin E. F., Dhondt A. A. (2010). Occupancy dynamics in a tropical bird community: unexpectedly high forest use by birds classified as non-forest species. J. Appl. Ecol. 47, 621–630. doi: 10.1111/jpe.2010.47.issue-3
Sánchez N. V., Sandoval L., Hedley R. W., St Clair C. C., Bayne E. M. (2022). Relative Importance for Lincoln’s Sparrow (Melospiza lincolnii) Occupancy of Vegetation Type versus Noise Caused by Industrial Development. Front. Ecol. Evol. 10. doi: 10.3389/fevo.2022.810087
Sandoval L. (2019). Variación mensual y anual de la riqueza y abundancia de aves en un mosaico agrícola periurbano tropical. Rev. Biol. Trop. 67, 298–314. doi: 10.15517/rbt.v67i2supl.37253
Sandoval L., Mennill D. J. (2012). Breeding biology of White-eared Ground-sparrow (Melozone leucotis), with a description of a new nest type. Ornitol. Neotropical. 23, 225–234.
Santos T., Tellería J. L. (2006). Pérdida y fragmentación del hábitat: efecto sobre la conservación de las especies. Rev. Ecosistemas 15.
Scales B. R., Marsden S. J. (2008). Biodiversity in small-scale tropical agroforests: a review of species richness and abundance shifts and the factors influencing them. Environ. Conserv. 35, 160–172. doi: 10.1017/S0376892908004840
Sharp P. J. (1996). Strategies in avian breeding cycles. Anim. Reprod. Sci. 42, 505–513. doi: 10.1016/0378-4320(96)01556-4
Shonfield J., Bayne E. M. (2017). Autonomous recording units in avian ecological research: current use and future applications. Avian Conserv. Ecol. 12. doi: 10.5751/ACE-00974-120114
Skutch A. F. (1950). The nesting seasons of Central American birds in relation to climate and food supply. Ibis 92, 185–222. doi: 10.1111/j.1474-919X.1950.tb01749.x
Soares A. S., Leão M. M. D., Neto M. V. (2017). Environmental fate of pesticides applied on coffee crops in southeast of Brazil. Afr. J. Environ. Sci. Technol. 11, 103–112. doi: 10.5897/AJEST2016.2187
Staicer C. A., Spector D. A., Horn A. G. (1996). “The dawn chorus and other diel patterns in acoustic signaling,” in Ecology and evolution of acoustic communication in birds. Eds. Kroodsma D. E., Miller E.H. (NY USA: Cornell University Press), 426–453.
Stanton R. L., Morrissey C. A., Clark R. G. (2018). Analysis of trends and agricultural drivers of farmland bird declines in North America: A review. Agricult. Ecosyst. Environ. 254, 244–254. doi: 10.1016/j.agee.2017.11.028
Stowell D. (2022). Computational bioacoustics with deep learning: a review and roadmap. PeerJ 10, e13152. doi: 10.7717/peerj.13152
Sugai L. S. M., Silva T. S. F., Ribeiro J. W. Jr., Llusia D. (2019). Terrestrial passive acoustic monitoring: review and perspectives. BioScience 69, 15–25. doi: 10.1093/biosci/biy147
Sun Y., Maeda T. M., Solís-Lemus C., Pimentel-Alarcón D., Buřivalová Z. (2022). Classification of animal sounds in a hyperdiverse rainforest using convolutional neural networks with data augmentation. Ecol. Indic. 145, 109621. doi: 10.1016/j.ecolind.2022.109621
Thomas C. N., Mansingh A. (2002). Bioaccumulation, elimination, and tissue distribution of chlorpyrifos by red hybrid Tilapia in fresh and brackish waters. Environ. Technol. 23, 1313–1323. doi: 10.1080/09593332308618324
Thomas A., Speldewinde P., Roberts J. D., Burbidge A. H., Comer S. (2020). If a bird calls, will we detect it? Factors that can influence the detectability of calls on automated recording units in field conditions. Emu-Austral Ornithol. 120, 239–248. doi: 10.1080/01584197.2020.1787848
Toledo V. M., Moguel P. (2012). Coffee and sustainability: the multiple values of traditional shaded coffee. J. Sustain. Agric. 36, 353–377. doi: 10.1080/10440046.2011.583719
Vega-Hidalgo. (2024). Sorx1.0.0. GitHub. Available at: https://github.com/alvarovegahd/soxr.
Venier L. A., Mazerolle M. J., Rodgers A., McIlwrick K. A., Holmes S., Thompson D. (2017). Comparison of semiautomated bird song recognition with manual detection of recorded bird song samples. ACE 1029, 120202. doi: 10.5751/ACE-01029-120202
Vickery J. A., Tallowin J. R., Feber R. E., Asteraki E. J., Atkinson P. W., Fuller R. J., et al. (2001). The management of lowland neutral grasslands in Britain: effects of agricultural practices on birds and their food resources. J. Appl. Ecol. 38, 647–664. doi: 10.1046/j.1365-2664.2001.00626.x
Waldron A., Garrity D., Malhi Y., Girardin C., Miller D. C., Seddon N. (2017). Agroforestry can enhance food security while meeting other sustainable development goals. Trop. Conserv. Sci. 10. doi: 10.1177/1940082917720667
Weibull A. C., Östman Ö. (2003). Species composition in agroecosystems: the effect of landscape, habitat, and farm management. Basic Appl. Ecol. 4, 349–361. doi: 10.1078/1439-1791-00173
Yager G. O., Wahab M. K. A., Agboola T. (2023). “Risk of Agrochemical on Biodiversity and Human Health: Conservation Implications and Sustainable Mitigations Strategies,” in One Health Implications of Agrochemicals and their Sustainable Alternatives (Springer Nature Singapore, Singapore), 181–209.
Zhang W., Cheng C., Chen L., Deng Y., Zhang L., Li Y., et al. (2018). Enantioselective toxic effects of cyproconazole enantiomers against Rana nigromaculata. Environ. Pollut. 243, 1825–1832. doi: 10.1111/csp2.568
Keywords: autonomous recording units, mobile app, citizen science, coffee farm, sampling protocol, species occurrence, vocalization analysis
Citation: Molina-Mora I, Ruíz-Gutierrez V, Vega-Hidalgo Á and Sandoval L (2024) The utility of passive acoustic monitoring for using birds as indicators of sustainable agricultural management practices. Front. Bird Sci. 3:1386759. doi: 10.3389/fbirs.2024.1386759
Received: 16 February 2024; Accepted: 29 July 2024;
Published: 23 August 2024.
Edited by:
Yizhar Lavner, Tel-Hai College, IsraelReviewed by:
Mário Gabriel Santiago Santos, University of Trás-os-Montes and Alto Douro, PortugalYossi Yovel, Tel Aviv University, Israel
Copyright © 2024 Molina-Mora, Ruíz-Gutierrez, Vega-Hidalgo and Sandoval. This is an open-access article distributed under the terms of the Creative Commons Attribution License (CC BY). The use, distribution or reproduction in other forums is permitted, provided the original author(s) and the copyright owner(s) are credited and that the original publication in this journal is cited, in accordance with accepted academic practice. No use, distribution or reproduction is permitted which does not comply with these terms.
*Correspondence: Ingrid Molina-Mora, bW9saW5hbS5pbmdyaWRAZ21haWwuY29t