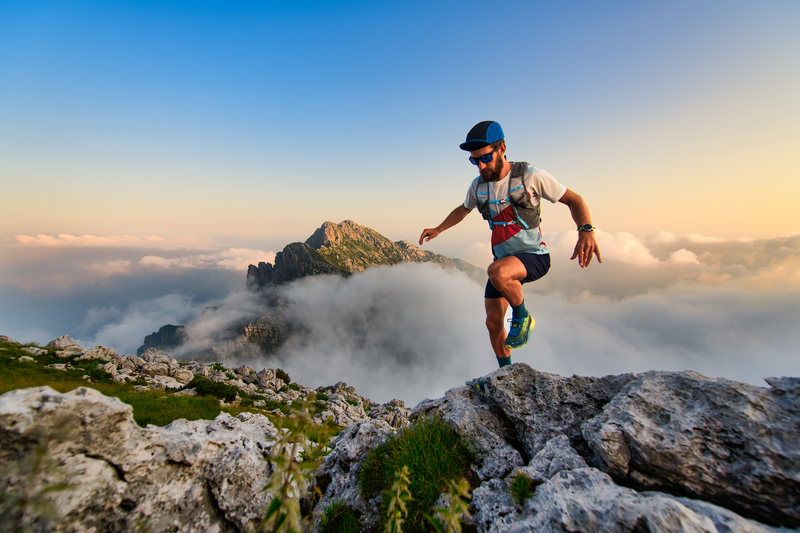
95% of researchers rate our articles as excellent or good
Learn more about the work of our research integrity team to safeguard the quality of each article we publish.
Find out more
ORIGINAL RESEARCH article
Front. Bird Sci. , 21 May 2024
Sec. Bird Conservation and Management
Volume 3 - 2024 | https://doi.org/10.3389/fbirs.2024.1385017
This article is part of the Research Topic Computational Bioacoustics and Automated Recognition of Bird Vocalizations: New Tools, Applications and Methods for Bird Monitoring View all 6 articles
Introduction: When studying bird intra- and inter-specific interactions it is crucial to accurately track which individual emits which vocalization. However, locating sounds of free moving birds (and other animals) in nature can be challenging, especially in situations when many individuals call in spatial and temporal vicinity.
Methods: In this paper, we will introduce the use of a hand-held acoustic camera which allows solving this problem. The system comprises 64 microphones each sampling a wide angle at a rate of 200kHz. The system can localize up to dozens of nearby callers and it displays them on a visual image. We applied the system to localize and record vocalizations of Arabian babblers (Argya squamiceps) during snake-mobbing behavior to demonstrate its potential. As the recorded babblers are ringed and therefore identifiable, we could use the calling order to analyze the vocalizing mob and to reconstruct a vocal social network depicting which individuals call after which individuals.
Results: Acoustic analysis revealed that Arabian babblers call in a periodic pattern and with age specific pause lengths between different individuals. Mobbing events with young babblers display a lower vocal network density compared to only adult groups.
Discussion: Our approach demonstrates how this novel technology can be applied to reveal new insight about vocal events in group living wild animals. The approach can be elaborated to study intra- and inter-specific animal vocalizations.
Locating vocalizations of animals in the wild is used to uncover the dynamics of intra- and inter-specific communication, identification of species, and abundance of species as well as movement patterns (Blumstein et al., 2011; Rhinehart et al., 2020). Tracking sounds emitted by free-moving individual animals in the field presents several challenges (Mizumoto et al., 2011; Rhinehart et al., 2020). When recording groups of animals, the close proximity between individuals complicates the identification of specific sound sources. The size of the individuals limits the use of on-board sound recorders which also necessitate the capturing and handling of wild animals (Anisimov et al., 2014; Greif and Yovel, 2019). To address these challenges, we introduce acoustic cameras which consist of large microphone arrays that allow accurate localization of multiple nearby sound sources. Acoustic cameras have been employed in various fields, including engineering, bioacoustics, and marine conservation biology (Mueller et al., 2006; Orman and Pinto, 2013; Beeck et al., 2022; Bocanegra et al., 2022). These cameras facilitate noise/sound detection and provide a visualization of the acoustic environment through phase array processing of acoustic signals (Erić, 2011). They consist of a microphone array and a video camera, enabling an overlay of the acoustic image on the recorded video to illustrate sound sources in space (Erić, 2011). Airborne acoustic camera systems have been particularly useful under laboratory conditions, for instance, recording typical and atypical ultrasound communication in mice, measuring the nasality of sounds in human speech, or capturing the diving sounds of Costa’s hummingbirds (Calypte costae) (Clark and Mistick, 2018; Lorenc et al., 2018; Rhinehart et al., 2020; Matsumoto et al., 2022). Additionally, an acoustic camera was used in a non-laboratory study with enclosed animals, but without following animal groups, to show that Asian elephants (Elephas maximus) produce coupled oral and nasal sound emission (Beeck et al., 2022). In the field, visualizing animal sounds remain challenging. Scientists have, for example, utilized sound-to-light conversion devices to assess call synchronization in Japanese tree frogs (Hyla japonica) at night (Mizumoto et al., 2011).
Vocalizations in social animals are crucial for various aspects such as cooperation, competition, maintaining group cohesion, decision making, parent–offspring interactions, reducing predation risk, and the regulation of social interactions (Bradbury and Vehrencamp, 1998; Raghuram et al., 2015). These communication systems significantly influence social relationships, survival, and reproduction (Fichtel and Manser, 2010; Griesser, 2013; Raghuram et al., 2015). For example, zebra finches utilize vocal cues for three-dimensional flock coordination, while meerkats (Suricata suricatta) demonstrate vocal turn-taking during low-conflict behavior, presumably to strengthen social bonds (Demartsev et al., 2018; Arnold et al., 2022). Competitive vocal behavior is observed in species like tungara frogs (Physalaemus pustulosus) where males attract mates through their calls in choirs (Bernal et al., 2009; Ryan et al., 2010). In a canopy katydid (Onomarchus uninotatus), signaling males are under less predation risk than searching females (Raghuram et al., 2015).
In many of the above scenarios, acoustic communication is characterized by multiple individuals who are vocalizing in close spatiotemporal vicinity. From a technical point of view, these situations are difficult to monitor. The main goal of this paper is to introduce the use of acoustic cameras for the study of vocalizations in dense groups of birds in the field. To demonstrate the potential of this approach, we analyzed the vocalizations of a group of mobbing Arabian babblers (Argya squamiceps).
Mobbing behavior in birds involves gathering around a predator, vocalizing loudly, and displaying visually (Altmann, 1956; Francis et al., 1989). This behavior is typically social, involving members of a group, other conspecifics, and sometimes heterospecifics (Coomes et al., 2019; Francis et al., 1989; Ostreiher, 2003). The emission of loud calls is a fundamental characteristic of avian mobbing (Ficken and Popp, 1996; Carlson and Griesser, 2022; Dutour et al., 2024). Research often examines the variety and rate of mobbing calls in response to different predators, but the identification of individual callers is less frequently noted (Stone and Trost, 1991; Baker and Becker, 2002; Griesser and Ekman, 2005; Griesser, 2009). The call rate, a measure of perceived threat, with individuals often calling in quick succession, complicates caller identification (Baker and Becker, 2002; Dutour et al., 2022; Hernández et al., 2023). Mobbing, despite its risks, such as increased predation danger, serves to alert others and to disrupt or remove predators (Chandler and Rose, 1988; Maklakov, 2002; Ostreiher, 2003; Carlson and Griesser, 2022; Hernández et al., 2023). Factors like age, sex, and social status can influence mobbing behavior (Francis et al., 1989; Hernández et al., 2023), and predator recognition and response can evolve over an individual’s life and may be socially learned by juveniles (Curio, 1978; Francis et al., 1989; Maklakov, 2002).
Arabian babblers are a species of group-living cooperatively-breeding passerine, inhabiting deserts, known for using a large repertoire of acoustic communication signals for group coordination and predator avoidance (Zahavi and Zahavi, 1997). Arabian babbler groups are generally stable throughout most of the year. They are territorial and engage in cooperative mobbing behavior towards predators like snakes, raptors, or mammals (Naguib et al., 1999; Maklakov, 2002; Sommer et al., 2012). Snake mobbing behavior in Arabian babblers begins with vocalizations by an individual upon discovering the snake. Mobbing vocalizations are of a typical type, also referred to as “tzwicks” (Naguib et al., 1999). If the recruitment of conspecifics is successful, the birds form a circle around the predator, with several individuals emitting mobbing calls while hopping around the snake, frequently opening their wings (Maklakov, 2002). Individuals may call or not and often join or leave the mobbing circle at some point of the mobbing event.
We recorded vocal turn-taking in Arabian babblers during snake mobbing using an acoustic camera, and created social vocal networks based on the order in which birds vocally engaged in the mobbing. We used social networks as a tool to visualize how vocalizations of different individuals can impact the mobbing dynamics of the whole group (Farine and Whitehead, 2015). Since age significantly influences individual social behavior and learning propensity (Keynan et al., 2015, 2016; Dragić et al., 2021), and given that mobbing behavior may develop during individual ontogeny (Francis et al., 1989; Ostreiher, 2003), we focused on the ontogeny of the participants of fledgling babblers in vocal turn-taking during snake mobbing. Specifically, we hypothesized that fledgling babblers would differ from adults in their temporal vocal participation, which would also be expressed in different network densities for groups with participants of different ages.
Our research was carried out at the Shezaf Nature Reserve, a 40 km² area in the Arava region of Israel’s Negev Desert, characterized as a hyper-arid desert with an average annual rainfall of around 30mm (Anava et al., 2001; Keynan and Yosef, 2010). The Arabian babbler study population that this research is based on was established by Professors Amotz & Avishag Zahavi in 1971, and the population has been monitored continuously since then. The population currently contains approx. 120 individuals from 21 groups, and group size varies between 3–18 individuals. Each individual in the population is ringed with a unique combination of color rings for individual identification. The study population is habituated to the presence of human observers, allowing behavioral observations and data collection from close range (within 2–3m) without causing any perceivable behavioral change or stress (Zahavi and Zahavi, 1997). Typically, a group of Arabian babblers features one dominant breeding pair. The breeding season extends from February to July, with a single nest incubated at a time. All adult group members contribute to feeding the young and defending the nest against intruders and predators (Ridley, 2007). The incubation period lasts 14–15 days, and nestlings fledge after 14–16 days. However, the young remain reliant on adult feeding for an additional six weeks. By 12 months, juveniles reach adulthood, distinguishable by sexual dimorphism in beak shape and eye color dichromatism (Ostreiher, 1999; Anava et al., 2001; Ridley, 2007).
From January to September 2023, we studied the snake mobbing behavior of ten Arabian babbler groups, with each group comprising of five to ten individuals, totaling 59 individuals. During this period, some individuals dispersed or established new groups, particularly between February and April. Six of these groups successfully bred, producing 16 fledglings. Each group was recorded several times, averaging 6.2 ± 2.7 sessions. The free-roaming nature of the birds posed challenges in locating specific groups on certain days. Upon entering a group territory, we ensured that babblers were in visual or audible proximity before setting up a dummy snake model, either an Egyptian horned viper (Cerastes cerastes) or a painted saw-scaled viper (Echis coloratus) partially embedded in the sand 20 meters from the group. We randomly switched between the two viper models per group to avoid habituation to the snake model. In addition to the acoustic camera (see below), we positioned a high-resolution RGB (red, green, and blue wavelength) video camera approximately 3m from the snake model to enable clear identification of individual birds. Before attracting the babblers to the model with mealworms (one, up to three), we activated the acoustic camera (Figure 1). The wide angle of the SoundCam 2.0 required the observer to maintain a 3-meter distance from the babblers, a distance measured prior to recording and typical for observing these birds (Ostreiher, 2003). Recordings were conducted in 1-minute segments with 20 second pauses for data storage, and triggering was manual. Intense mobbing typically occurred in the initial three to five minutes, likely diminishing as the stationary snake model failed to maintain the babblers’ interest. Recording sessions were concluded when the group either moved beyond 3m from the dummy or stopped vocalizing for one minute.
Figure 1 Setup of the snake mobbing experiment. Simultaneous recording of Arabian babbler snake mobbing with a high-resolution RGB video camera and a hand-held acoustic camera. Photos of the acoustic camera and the dummy snake are provided in the Supplementary Material.
The Soundcam 2.0 acoustic camera was used to locate sounds of Arabian babblers during snake mobbing. The acoustic camera, comprising 64 microphones, each sampling a wide aperture angle 70° (FoV horizontal) at a rate of 200kHz, stored with a 24-bit sound resolution, allowing a recording range of 20 meters for sounds as weak as 33dB and with beamforming-methods from 800 Hz; below this threshold, the resolution is decreased (SoundCam 2.0, CAE Software and Systems GmbH, Guetersloh, Germany). Arabian babbler mobbing calls cover frequencies from 2000Hz up to 8000Hz. Acoustic beam size and frequencies visualized in the black and white video were manually adjusted in the field in consideration of the soundscape of the surrounding during the recording. Beam size on screen was manually changed by regulating the dynamic range. Therefore, localization of filtered frequencies in the video were visualized even when masking sounds from wind, or other acoustic disturbances were present in the surrounding. While the video setting was adjusted for optimal call visualization, the full acoustic data was saved in a TDMS file, ensuring that no information was lost due to filtering. For the acoustic camera to effectively address our questions, we had to ensure following conditions: (1) The mobbing was set in a predictable location to synchronize and focus both, the acoustic camera, and an additional high-resolution video camera. The latter was used to ease individual recognition. (2) The wide angle of the acoustic camera, combined with the close proximity of the individuals during mobbing, required maintaining a three-meter distance, to prevent compromised video resolution. We tested the spatial resolution of the system by placing two speakers playing back Arabian babbler mobbing sequences of one group, with calls naturally alternating and overlapping in time. We placed the speakers at different distances from each other along a horizontal line and recorded this scenario according to the same guideline used in the experiment. Measured distances between speakers were: 5cm, 10cm and 15cm and we analyzed 50 calls per distance category. Call loudness was not normalized to copy natural conditions. We then manually annotated the broadcasting speaker by observing the speaker (as done with the babblers) and we validated this using the known ground truth.
We synchronized the acoustic camera (25fps) and video camera recordings (25fps) using adobe premium pro (Version 23.6.4), which also enabled us to place individual markers for each vocalizing babbler. The ring colors were distinguishable in the video’s RGB recordings, while the acoustic camera provided information on which individual was vocalizing and the timing of their calls (±40ms per frame) which were identified by the experimenter (Figure 2, upper). Due to the high resolution of RGB recordings, beak opening during vocalizations was occasionally used to reassure caller identity. The wide-angle setting of the acoustic camera also allowed us to capture vocalizations from individuals perched in nearby trees who later joined the mobbing circle (Figure 2, lower). We used Raven Pro 1.6.5 (K. Lisa Yang Center for Conservation Bioacoustics, 2024), to visualize snake mobbing vocalizations of 1–3-month-old juveniles joining the mobbing for the first time.
Figure 2 Video frames of the Arabian babbler mobbing circle and spectrogram of two mobbing calls. Video frames of both cameras are presented for the same scene. Videos of the high-resolution RGB video camera and the acoustic camera are synchronized, allowing the identification of the vocalizing individual in that moment (upper). The position of the dummy snake is marked with a blue cross in the RGB video. The acoustic camera visualizes locations with high sound pressure [dB] as a colorful beam in a black and white video (lower panels, left). Acoustic camera sound recording of two mobbing calls (lower panel, right).
To explore the vocal dynamics of snake mobbing in Arabian babblers and to examine if social–vocal network parameters differ across age categories, we analyzed each snake mobbing event by creating a directional network, yielding a total of 62 networks from the 10 groups (6.2 ± 2.7 recordings per group), excluding defective recordings or trials with only one or two individuals mobbing. To reconstruct the networks each edge i–j in the network was strengthened when individual i vocalized after individual j. We set a minimum threshold of 120ms for the interval between the calls of i–j because shorter intervals are unlikely to be a response due to the minimum reaction time. Therefore, if a respondent i called more than 120ms after the individual j, the preceding individual was designated as the source. This 120ms threshold was selected based on the auditory reaction time found for other species and the focal one (Thorpe, 1963). Thus, if individual i called only 40ms after j, it is unlikely that it was responding to j. Pause duration between calls of different individuals was analyzed by ignoring the minimal threshold of 120ms, but setting a maximal threshold at 1600ms to portrait behavior during mobbing and not when individuals start to join. 85.9% of the data lay between the pause durations of 0–160ms.
Networks were generated using the “igraph” package in R-studio 2022.12.0 (Csardi and Nepusz, 2006; Posit team, 2022; R Core Team, 2022). We focused on network density to assess the participation of group members during vocal turn taking for the whole mobbing sequence. The network density measures the ratio between the actual number of connections in a network and the maximum possible number of connections. It is therefore a measurement of the proportions of pairs that call after each other. Density was additionally examined as a function of group size.
To test differences of individual and group vocal turn-taking based on age category during snake mobbing, we used generalized linear mixed models (GLMMs) and a linear mixed model (LMM). We chose the three response variables: “Inter-individual pause duration[ms]”, “Graph density as function of youngest juvenile in the group”, and “Number of calls as function of youngest juvenile in the group”. For each model, “group identity” was set as random factor and for the model with group density as response variable, number of individuals was added as a fixed factor. The generalized linear mixed models (GLMMs) and the linear mixed model (LMM) were calculated using the R-packages “lme4” and “arm” in R-4.2.2 (Bates et al., 2015; Gelman and Su, 2020). For the LMM, we assumed a Gaussian-, and for all GLMMs a negative binomial error distribution. The model fit was assessed through visual inspection of the residuals and by applying the R-package “DHARMa” to assure model fit (Hartig, 2022).
We estimated correct positions of calls when speakers were 5cm apart with 76% accuracy, for speakers being 10cm apart with 72%accuracy and for speakers being 15cm apart with 80% accuracy. All but two errors occurred in cases when the calls played by the two speakers overlapped in time, especially when one call was louder than the other (Figure 3). We thus examined our accuracy specifically in overlapping calls and found that at 5cm we accurately identified the caller 44%, while it was 50% for 10cm and 40% for 15cm (Figure 3). In reality, mobbing babblers typically stand at least 10cm apart and rarely call simultaneously. Moreover, we know based on the spectrogram when calls are overlapping and could pay special attention to these cases. Therefore, these results demonstrate the validity of the acoustic camera for studying the phenomenon.
Figure 3 Test of the camera resolution. Percentage of accurate identification of sound source position as a function of the distance between sources. Categories were left speaker (left), right speaker (right), both speakers at the same time (overlapping), and over all accuracy (combined).
Juveniles younger than a month did not engage in snake mobbing. At this stage, they primarily follow caretakers and beg for food from the adults involved in mobbing, while ignoring the presence of the snake. The youngest mobbing individual that we observed was a 1–2-month-old, un-ringed individual. Although unable to produce a proper mobbing call, this juvenile exhibited attempts to mob the snake-model, showing vigilance and emitting shortened begging calls (Figure 4A). The youngest of the nine precisely ageable juveniles to participate in mobbing and emit accurate mobbing calls, was 48 ± 3 days old.
Figure 4 The mobbing vocalizations and inter individual pause duration. (A) Call spectrograms of snake mobbing events. The upper spectrogram shows a sequence of vocalizations emitted by three individuals (A–C) during a typical snake mobbing event with fully developed mobbing calls (recorded with the acoustic camera). The lower spectrogram visualizes a rare mobbing event, when a juvenile under 2 months old vocally joined one adult babbler during snake mobbing with a modified begging call (marked with grey squares), probably because it was not able to produce a mobbing call yet. The other calls in the spectrogram belong to the adults (A, B). White arrows mark two exemplar Inter-individual pause duration. (B) Frequency of data of Inter-individual pause durations. Pause durations shorter than the proposed reaction time are color-coded in brown and periodically occurring pause durations are highlighted in blue.
Analyzing the mobbing sequences revealed that Arabian babblers take turns vocalizing with preferred pause durations between consecutive calls. The most common pause is around 175ms long, which is in-line with the reaction time discussed above, and with additional peaks appearing at approximately integer multiplications of 175 ± 25ms (Figure 4B).
In order to explore the importance of learning the temporal coordination of snake mobbing, we examined how babblers of different ages time their pauses (Figure 5A). When examining pauses between calls emitted by individuals of different age groups, it can be seen that very young juveniles aged 1.5–3 months vocalize with significant shorter pauses when responding to each other compared to when responding to older individual such as adults (β=0.42, SE=0.12, z(280)=3.89, p<0.001, GLMM, Table 1, Figure 5B), or juveniles aged 4–6-month (β=0.45, SE=0.15, z(280)=3.07, p<0.01, GLMM, Table 1, Figure 5B). These shorter pauses may diminish the opportunity for other individuals to join the mobbing vocally.
Figure 5 Inter-individual pause durations between different age classes. (A) Heatmap of median pause durations between individuals of different age groups. (B) The response time of 1.5–3-month-old juveniles dependent on the previous caller.
Table 1 Generalized linear mixed model output comparing pause durations of 1.5–3-month-old juveniles dependent on the age category of the previous caller (n=280).
To investigate vocal turn-taking of mobbing babblers, we tested if the social–vocal network density and call rate differ across groups defined by the youngest participant. We found that groups containing very young juveniles aged 1.5–3 months exhibited reduced density compared to groups composed solely of adults (β=0.2, SE=0.06, z(62)=3.23, p<0.001, LMM, Figure 6A, Table 2). Groups with intermediate and older juveniles (4–6-month-old) exhibited intermediate network densities which did not differ statistically from each other, or from groups with 1.5–3-month-old juveniles (β<0.2, p>0.05, LMM, Figure 6A, Table 2). Therefore, the densities of networks follow an age gradient from low densities of groups with young babblers towards high densities in adult groups. Graph density was not correlated with group size (β=−0.02, SE=0.015, z(62)=−1.52, p>0.05, LMM, Table 2). The differences in network density could also not be explained by calling propensity. Groups composed of solely adults, actually called significantly less than groups with juveniles of any age category (β=−0.51, SE=0.24, z(62)=−2.17, p<0.001, GLMM, Figure 6B, Table 2). The mobbing events containing solely adults therefore show a higher vocal connectivity, even though the call rate of adult groups is lower than in groups with juveniles (Figure 6C).
Figure 6 (A) Network densities of groups with individuals of different age classes. Groups were categorized depending on the youngest participating juvenile during a mobbing event and were addressed to one of the three different age categories from 1.5 to 12 months. (B) Call rate of groups with individuals of different age classes. Letters a and b describe age categories that have significantly different parameter values from each other. (C) Example of two networks with the same number of babblers participating. The upper network describes a mobbing event with only adults, while the lower network shows an event including one juvenile.
Table 2 Linear and generalized linear mixed model output comparing the network density and number of calls of 62 networks categorized by groups dependent on the youngest individual participating during the snake mobbing event.
Arabian babbler mobbing–vocal networks generally exhibit symmetry between in-degree and out-degree edges (n=62 networks) (Figure 7B). This symmetry arises from alternating calls between two or more individuals. To illustrate the temporal dynamics of these networks, we visualized the social vocal networks of the same group during two different snake mobbing events, each lasting three minutes (Figure 7). The group consisted of 4 adults, one older juvenile (J1 ~8 months old) and two younger juveniles (J2–3 ~1.7 months old). These events were nine days apart and the differences were pronounced. In the first event only the older juvenile (J1) participated in mobbing, vocalizing after the subordinate female F2. However, in the subsequent event (9 days later) the younger siblings (J2–3) who previously only begged, now actively joined in, mostly calling in alternations with the dominant male (M1), the second ranked male (M2) and the second ranked female (F2) (Figure 7). The density of networks decreases from 0.7 to 0.57, after juveniles join the mobbing.
Figure 7 Juveniles joining the mobbing network. (A) Visualization of the network and edge strength heatmap of a mobbing event without juveniles. (B) Visualization of the network and edge strength heatmap of a mobbing event with juveniles. The events are nine days apart and two young juveniles participate vocally only during the second event. Each node represents one individual of the group: F=female, M=male and J=juvenile; numbers from 1–3 after the letters J describe the age-related hierarchy of Arabian babbler groups. F1 and M1 are the breeding pair, while F2 and M2 are the subordinates. The oldest juvenile J1 is ~8 months old. Edges represent call response and the edge strength between individuals is displayed as color gradient from dark green (strong) to white (weak).
The acoustic camera can be useful for studying various animal systems where the localization of multiple vocalizing animals is necessary. Our setup can be used for recording the role of vocalizations during take-off and group flight in birds like flocking Tristram’s starlings (Onychognathus tristramii) and monk parakeets (Myiopsitta monachus, Figures 8A, B). The operational constraints of the acoustic camera, such as time and proximity, can be mitigated by connecting the SoundCam 2.0 to a laptop and mounting it on a tripod. When connected to a laptop, longer video and high-quality sound recordings can be performed, and the operator can maintain a greater distance from the camera to minimize disturbance. This setup is for example suitable to record animals in roosting, feeding, or courting sites such as our recordings of the Greater mascarene flying fox (Pteropus niger) in Mauritius (Figure 8C), or congregating Middle east tree frogs (Hyla savignyi) courting during the night (Figure 8D). The maximal distance to record animals is ~20 meters (for most species), which can limit the application of the camera especially in regard to flying wild birds.
Figure 8 SoundCam recordings of different animal vocalizations. Video recording frame images and cut outs of associated sound files of: (A) Tristram’s starlings (Onychognathus tristramii) during departure from a tree recorded in ein Gedi, Israel, (B) flocking Monk parakeets (Myiopsitta monachus) in Tel‐Aviv, Israel, (C) foraging Greater mascarene flying foxes (Pteropus niger) in Mauritius, and (D) congregating Middle east tree frogs (Hyla savignyi) in Tel‐Aviv. Locations with high sound pressure [dB] are visualized as a colorful beam in a black and white video.
This study provides examples and a detailed analysis of a case study using acoustic cameras for location of free-roaming vocalizing animals in the wild. Sound localization in natural environments presents several methodological challenges, which lead to limited research on the acoustic coordination of animals, as it often requires a complex setup that does not allow for following moving animal groups (Mizumoto et al., 2011). As Rhinehart et al. (2020) stated: “We find that the labor-intensive steps of processing recordings and estimating animal positions have not yet been automated.” To address some of these challenges, we introduced the SoundCam 2.0 acoustic camera to explore how Arabian babblers (Argya squamiceps) of different age groups perform vocal snake mobbing. We also demonstrate how this system might be useful for recording other animals like departing Tristram’s starlings (Onychognathus tristramii), flocking monk parakeets (Myiopsitta monachus), congregating Middle east tree frogs (Hyla savignyi), and foraging Greater Mascarene flying foxes (Pteropus niger).
The hand-held acoustic camera, equipped with 64 microphones, is portable and simple to set up. But note that the camera case has dimensions of 48 × 58 × 27 cm, which can make transportation into remote areas by foot somewhat difficult. The sound recordings can be analyzed separately from the video, and the unfiltered acoustic data could be utilized for frequency analysis. The camera, when not connected to a laptop, is ideal for recordings of up to 60 seconds. Due to the high information load, saving recordings can take up to 20 seconds. However, this effect was mitigated by the real-time visualization of sounds on the camera screen after recording started, and the option to save data retrospectively after the recording. This feature made it easier to capture desired sounds and minimized the loss of major call interactions. Still, long-term fully-continuous recordings are not possible by using the camera software. The system also allows for remote recordings of animals for up to 10 seconds pre-trigger setting. Synchronizing videos via acoustic cues from the acoustic camera with a high-resolution RGB video camera was necessary to identify individual ring colors in Arabian babblers, due to the black and white video of the acoustic camera. Recording animals in the dark can bear several additional challenges, like caller identification. Testing the video resolution of the acoustic camera revealed easy identification of beam sources when two sound sources are alternating, but we struggled to clearly identify overlapping callers that were standing right near each-other. To differentiate overlapping from non-overlapping calls when sound sources are less than 20cm apart, is challenging, mainly when one call is louder than the other. We minimized this effect, by also looking at beak opening in the high resolution RGB film, when the beam source was unclear. Moreover, the spectrogram of the sound file could be used to clearly identify overlapping calls and thus use additional information to identify the caller.
Using the acoustic camera for tracking the vocalizations of groups of Arabian babblers during snake mobbing revealed evidence for the importance of learning of vocal turn taking during mobbing:
(1) Learning plays an important role in the early development of Arabian babblers (Anava et al., 2001; Ridley, 2007; Keynan et al., 2015, 2016). Studies across various species reveal diverse juvenile responses to mobbing, ranging from independent mobbing without learning to observing and then joining experienced individuals (Carlson and Griesser, 2022). Our findings indicate that Arabian babblers start to engage in snake mobbing circles with mature mobbing calls at approximately 50 days old (about one month after fledging). We noted two younger juveniles using underdeveloped mobbing calls, alternating them with calls emitted by an adult. This anecdotal observation indicated the importance of the temporal alternation pattern alongside the development of adult mobbing calls in mobbing behavior. Juveniles might also need time to learn to associate a specific call with a specific predator as is proposed for Siberian jays (Griesser and Suzuki, 2016).
(2) Arabian babblers generally vocalize in a periodic pattern with individuals taking turns in calling. The occurrence of preferred pause duration of 150–200ms and repeated integer multiplications suggest a preferred rhythmic pattern during snake mobbing. Young juveniles – 1.5–3 months old – diverged from this pattern and vocalized after each other with shorter pauses then when calling after older juveniles of 4–6 months of age, and with extended pauses when calling after adults. This suggests that babblers have to learn how to coordinate vocalizations during snake mobbing. However, the observation that when calling after adults, the juveniles do wait longer suggests that shorter pauses might be advantageous for very young juveniles during collective mobbing. In other songbirds like house wrens (Troglodytes aedon chilensis), adults exhibit a stronger response to mobbing call playbacks with increased call rates (Fernández et al., 2023). Similarly, juvenile Richardson’s ground squirrels (Spermophilus richardsonii) increase call rate as predators’ approach, enhancing the vigilance of adult squirrels (Warkentin et al., 2001). Given that young Arabian babbler juveniles wait shorter pauses when vocalizing after each other and considering the post-fledging period is marked by high mortality rates for the young, a similar heightened response to call rate might occur in adult babblers (Ridley, 2007). This does not explain why juveniles show the longer pause durations when calling after an adult. As mobbing can be dangerous, juveniles might express less stress, when experienced adults are vocalizing, than when mobbing with other inexperienced juveniles, resulting in longer pause durations and a lower call rate. Still, the length of pause duration when calling after adults exceeds the length of pauses between adults themselves. Considering that older individuals show similar pause durations during mobbing, young babblers might learn the ideal call pattern by vocally adjusting to adults.
(3) Analyzing social vocal networks of babbler calls showed that, mobbing events including young babblers are characterized by lower network densities compared to groups comprising only adults. This outcome might be linked to the tendency of very young juveniles to use short pauses, possibly preventing other individuals from joining the mobbing vocally, which might lead to the lower vocal network density. Another possible interpretation for this result is that some adults call less after inexperienced juveniles. Considering that the call rate of the mobbing event is higher in groups including young juveniles, but the density of the network is lower, young juveniles might influence the vocal participation of other group members thus actually altering the social structure of mobbing.
In general, our results, which could only be obtained thanks to the acoustic camera, suggest that vocal turn taking during mobbing needs to be acquired by juveniles and might embed more social information than meets the eye at first analysis. Further research and larger sample sizes are required to unravel these hidden patterns and to determine what is the impact of call rate, in addition to caller identity, in mobbing behavior in babblers.
The raw data supporting the conclusions of this article will be made available by the authors, without undue reservation.
The animal study was approved by Israeli Nature and Parks Authority under the permit number 2022/43151. The study was conducted in accordance with the local legislation and institutional requirements.
MG: Writing – review & editing, Writing – original draft, Visualization, Software, Project administration, Methodology, Investigation, Formal analysis, Data curation, Conceptualization. AB: Writing – review & editing, Software, Methodology. OK: Writing – review & editing, Supervision, Resources, Methodology, Conceptualization. YY: Writing – review & editing, Validation, Supervision, Resources, Methodology, Conceptualization.
The author(s) declare that no financial support was received for the research, authorship, and/or publication of this article.
We want to thank Nikola Dragić for support in creating social networks. We are grateful for the hospitality of Dorit Narisna and Hazeva Field School during fieldwork. We are especially grateful to Yael Alon, Edgardo Santiago Rivera, Krista Oswald, and Alejandro Alaman for their support during and after fieldwork. Thanks also to Omer Yinon, who provided high quality recordings of the Greater Mascarene flying fox (Pteropus niger).
The authors declare that the research was conducted in the absence of any commercial or financial relationships that could be construed as a potential conflict of interest.
All claims expressed in this article are solely those of the authors and do not necessarily represent those of their affiliated organizations, or those of the publisher, the editors and the reviewers. Any product that may be evaluated in this article, or claim that may be made by its manufacturer, is not guaranteed or endorsed by the publisher.
The Supplementary Material for this article can be found online at: https://www.frontiersin.org/articles/10.3389/fbirs.2024.1385017/full#supplementary-material
Altmann S. A. (1956). Avian mobbing behavior and predator recognition. Condor 58, 241–253. doi: 10.2307/1364703
Anava A., Kam M., Shkolnik A., Degen A. A. (2001). Growth rate and energetics of Arabian Babbler (Turdoides squamiceps) nestlings. Auk 118, 519–524. doi: 10.1093/auk/118.2.519
Anisimov V. N., Herbst J. A., Abramchuk A. N., Latanov A. V., Hahnloser R. H., Vyssotski A. L. (2014). Reconstruction of vocal interactions in a group of small songbirds. Nat. Methods 11, 1135–1137. doi: 10.1038/nmeth.3114
Arnold F., Staniszewski M. S., Pelzl L., Ramenda C., Gahr M., Hoffmann S. (2022). Vision and vocal communication guide three-dimensional spatial coordination of zebra finches during wind-tunnel flights. Nat. Ecol. Evol. 6, 1221–1230. doi: 10.1038/s41559-022-01800-4
Baker M. C., Becker A. M. (2002). Mobbing calls of black-capped chickadees: effects of urgency on call production. Wilson Bull. 114, 510–516. doi: 10.1676/0043-5643(2002)114[0510:MCOBCC]2.0.CO;2
Bates D., Mächler M., Bolker B., Walker S. (2015). Fitting linear mixed effects models using lme4. J. Stat. Software 67, 1e14. doi: 10.18637/jss.v067.i01
Beeck V. C., Heilmann G., Kerscher M., Stoeger A. S. (2022). Sound visualization demonstrates velopharyngeal coupling and complex spectral variability in Asian elephants. Animals 12, 2119. doi: 10.3390/ani12162119
Bernal X. E., Akre K. L., Baugh A. T., Rand A. S., Ryan M. J. (2009). Female and male behavioral response to advertisement calls of graded complexity in túngara frogs, Physalaemus pustulosus. Behav. Ecol. Sociobiology 63, 1269–1279. doi: 10.1007/s00265-009-0795-5
Blumstein D. T., Mennill D. J., Clemins P., Girod L., Yao K., Patricelli G., et al. (2011). Acoustic monitoring in terrestrial environments using microphone arrays: applications, technological considerations and prospectus. J. Appl. Ecol. 48, 758–767. doi: 10.1111/j.1365-2664.2011.01993.x
Bocanegra J. A., Borelli D., Gaggero T., Rizzuto E., Schenone C. (2022). A novel approach to port noise characterization using an acoustic camera. Sci. Total Environ. 808, 151903. doi: 10.1016/j.scitotenv.2021.151903
Bradbury J. W., Vehrencamp S. L. (1998). Principles of Animal Communication (Sunderland/MA: Sinauer Associates).
Carlson N. V., Griesser M. (2022). Mobbing in animals: a thorough review and proposed future directions. Adv. Study Behav. 54, 1–41. doi: 10.1016/bs.asb.2022.01.003
Chandler C. R., Rose R. K. (1988). Comparative Analysis of the Effects of Visual and Auditory Stimuli on Avian Mobbing Behavior (Análisis Comparativo del Efecto de Estímulos Visuales y Auditivos en Conducta de Tumultos en Aves). J. Field Ornithology 59, 269–277. https://www.jstor.org/stable/4513343
Clark C. J., Mistick E. A. (2018). Strategic acoustic control of a hummingbird courtship dive. Curr. Biol. 28, 1257–1264. doi: 10.1016/j.cub.2018.03.021
Coomes J. R., McIvor G. E., Thornton A. (2019). Evidence for individual discrimination and numerical assessment in collective antipredator behaviour in wild jackdaws (Corvus monedula). Biology Letters 15 (10), 20190380. doi: 10.1098/rsbl.2019.0380
Csardi G., Nepusz T. (2006). The igraph software package for complex network research. InterJournal Complex Syst. 1695, 1–9.
Curio E. (1978). The adaptive significance of avian mobbing: I. Teleonomic hypotheses and predictions. Zeitschrift für Tierpsychologie 48 (2), 175–183. doi: 10.1111/j.1439-0310.1978.tb00254.x
Demartsev V., Strandburg-Peshkin A., Ruffner M., Manser M. (2018). Vocal turn-taking in meerkat group calling sessions. Curr. Biol. 28, 3661–3666. doi: 10.1016/j.cub.2018.09.065
Dragić N., Keynan O., Ilany A. (2021). Multilayer social networks reveal the social complexity of a cooperatively breeding bird. Iscience 24. doi: 10.1016/j.isci.2021.103336
Dutour M., Fernandez G. J., Randler C. (2022). How great tits respond to urgency-based information in allopatric Southern house wren mobbing calls. Ethology 128, 676–683. doi: 10.1111/eth.13329
Dutour M., Lengagne T., Léna J. P. (2024). Relative divergence of mobbing calls and songs structures in passerine birds. J. Ornithology 165, 217–229. doi: 10.1007/s10336-023-02101-8
Erić M. M. (2011). Some research challenges of acoustic camera. 2011 19th Telecommunications Forum (TELFOR) Proceedings of Papers, IEEE. (Belgrade, Serbia: IEEE), pp. 1036–1039. doi: 10.1109/TELFOR.2011.6143725
Farine D. R., Whitehead H. (2015). Constructing, conducting and interpreting animal social network analysis. J. Anim. Ecol. 84, 1144–1163. doi: 10.1111/1365-2656.12418
Fernández G. J., Dutour M., Carro M. E. (2023). Information transfer during mobbing: call rate is more important than the number of callers in a southern temperate passerine. Behav. Ecol. Sociobiology 77, 78. doi: 10.1007/s00265-023-03357-z
Fichtel C., Manser M. (2010). “Vocal communication in social groups,” in Animal Behaviour: Evolution and Mechanisms. Ed. Kappeler P. (Springer, Berlin, Heidelberg). doi: 10.1007/978-3-642-02624-9_2
Ficken M. S., Popp J. (1996). A comparative analysis of passerine mobbing calls. Auk 113, 370–380. doi: 10.2307/4088904
Francis A. M., Hailman J. P., Woolfenden G. E. (1989). Mobbing by Florida scrub jays: Behaviour, sexual asymmetry, role of helpers and ontogeny. Anim. Behav. 38, 795–816. doi: 10.1016/S0003-3472(89)80112-5
Gelman A., Su Y. (2020) arm: Data Analysis Using Regression and Multilevel/Hierarchical Models. R package. version 1.11-2. Available online at: https://CRAN.R-project.org/package=arm.
Greif S., Yovel Y. (2019). Using on-board sound recordings to infer behaviour of free-moving wild animals. J. Exp. Biol. 222, jeb184689. doi: 10.1242/jeb.184689
Griesser M. (2009). Mobbing calls signal predator category in a kin group-living bird species. Proc. R. Soc. B: Biol. Sci. 276, 2887–2892. doi: 10.1098/rspb.2009.0551
Griesser M. (2013). Do warning calls boost survival of signal recipients? Evidence from a field experiment in a group-living bird species. Front. Zoology 10, 1–5. doi: 10.1186/1742-9994-10-49
Griesser M., Ekman J. (2005). Nepotistic mobbing behaviour in the Siberian jay, Perisoreus infaustus. Anim. Behav. 69, 345–352. doi: 10.1016/j.anbehav.2004.05.013
Griesser M., Suzuki T. N. (2016). Kinship modulates the attention of naïve individuals to the mobbing behaviour of role models. Anim. Behav. 112, 83–91. doi: 10.1016/j.anbehav.2015.11.020
Hartig F. (2022) _DHARMa: Residual Diagnostics for Hierarchical (Multi-Level/Mixed) Regression Models_. R package version 0.4.6. Available online at: https://CRAN.R-project.org/package=DHARMa.
Hernández M. C., Lara R. A., Redondo A. J. (2023). To mob or not to mob: habitat and time of day influence in mobbing behavior in the azure-winged magpie (Cyanopica cookii). Zoological Sci. 40, 273–277. doi: 10.2108/zs230004
Keynan O., Ridley A. R., Lotem A. (2015). Social foraging strategies and acquisition of novel foraging skills in cooperatively breeding Arabian babblers. Behav. Ecol. 26, 207–214. doi: 10.1093/beheco/aru181
Keynan O., Ridley A. R., Lotem A. (2016). Task-dependent differences in learning by subordinate and dominant wild Arabian babblers. Ethology 122, 399–410. doi: 10.1111/eth.12488
Keynan O., Yosef R. (2010). Annual precipitation affects reproduction of the Southern Grey Shrike (Lanius meridionalis). Wilson J. Ornithology 122, 334–339. doi: 10.1676/09-148.1
K. Lisa Yang Center for Conservation Bioacoustics (2024). Raven Pro: Interactive Sound Analysis Software (Version 1.6.5) [Computer software] (Ithaca, NY: The Cornell Lab of Ornithology). Available at: https://ravensoundsoftware.com/.
Lorenc A., Król D., Klessa K. (2018). An acoustic camera approach to studying nasality in speech: The case of Polish nasalized vowels. J. Acoustical Soc. America 144, 3603–3617. doi: 10.1121/1.5084038
Maklakov A. A. (2002). Snake-directed mobbing in a cooperative breeder: anti-predator behaviour or self-advertisement for the formation of dispersal coalitions? Behav. Ecol. Sociobiology 52, 372–378. doi: 10.1007/s00265-002-0528-5
Matsumoto J., Kanno K., Kato M., Nishimaru H., Setogawa T., Chinzorig C., et al. (2022). Acoustic camera system for measuring ultrasound communication in mice. Iscience 25. doi: 10.1016/j.isci.2022.104812
Mizumoto T., Aihara I., Otsuka T., Takeda R., Aihara K., Okuno H. G. (2011). Sound imaging of nocturnal animal calls in their natural habitat. J. Comp. Physiol. A 197, 915–921. doi: 10.1007/s00359-011-0652-7
Mueller R. P., Brown R. S., Hop H., Moulton L. (2006). Video and acoustic camera techniques for studying fish under ice: a review and comparison. Rev. Fish Biol. Fisheries 16, 213–226. doi: 10.1007/s11160-006-9011-0
Naguib M., Mundry R., Ostreiher R., Hultsch H., Schrader L., Todt D. (1999). Cooperatively breeding Arabian babblers call differently when mobbing in different predator-induced situations. Behav. Ecol. 10, 636–640. doi: 10.1093/beheco/10.6.636
Orman M., Pinto C. T. (2013). Usage of acoustic camera for condition monitoring of electric motors. In Proceedings of the IEEE Region 10 Annual International Conference, Proceedings/TENCON, Xi’an, China, 22–25.
Ostreiher R. (1999). Nestling feeding-space strategy in Arabian babblers. Auk 116, 651–657. doi: 10.2307/4089326
Ostreiher R. (2003). Is mobbing altruistic or selfish behaviour? Anim. Behav. 66, 145–149. doi: 10.1006/anbe.2003.2165
Posit team (2022). RStudio: Integrated Development Environment for R (Boston, MA: Posit Software, PBC). Available at: http://www.posit.co/.
Raghuram H., Deb R., Nandi D., Balakrishnan R. (2015). Silent katydid females are at higher risk of bat predation than acoustically signalling katydid males. Proc. R. Soc. B: Biol. Sci. 282, 20142319. doi: 10.1098/rspb.2014.2319
R Core Team (2022). R: A language and environment for statistical computing (Vienna, Austria: R Foundation for Statistical Computing). Available at: https://www.R-project.org/.
Rhinehart T. A., Chronister L. M., Devlin T., Kitzes J. (2020). Acoustic localization of terrestrial wildlife: Current practices and future opportunities. Ecol. Evol. 10, 6794–6818. doi: 10.1002/ece3.6216
Ridley A. R. (2007). Factors affecting offspring survival and development in a cooperative bird: social, maternal and environmental effects. J. Anim. Ecol. 76, 750–760. doi: 10.1111/j.1365-2656.2007.01248.x
Ryan M. J., Bernal X. E., Rand A. S. (2010). Female mate choice and the potential for ornament evolution in túngara frogs Physalaemus pustulosus. Curr. Zoology 56, 343–357. doi: 10.1093/czoolo/56.3.343
Sommer C., Todt D., Ostreiher R., Mundry R. (2012). Urgency-related alarm calling in Arabian babblers, Turdoides squamiceps: predator distance matters in the use of alarm call types. Behaviour 149 (7), 755–773. doi: 10.1163/1568539X-00003003
Stone E., Trost C. H. (1991). Predators, risks and context for mobbing and alarm calls in black-billed magpies. Anim. Behav. 41, 633–638. doi: 10.1016/S0003-3472(05)80901-7
Thorpe W. H. (1963). Antiphonal singing in birds as evidence for avian auditory reaction time. Nature 197, 774–776. doi: 10.1038/197774a0
Warkentin K. J., Keeley A. T., Hare J. F. (2001). Repetitive calls of juvenile Richardson’s ground squirrels (Spermophilus richardsonii) communicate response urgency. Can. J. Zoology 79, 569–573. doi: 10.1139/z01-017
Keywords: acoustic camera, sound imaging, vocal mobbing, social animal, bioacoustics, vocal turn-taking
Citation: Guggenberger M, Boonman A, Keynan O and Yovel Y (2024) Using acoustic cameras to study vocal mobbing reveals the importance of learning in juvenile Arabian babblers. Front. Bird Sci. 3:1385017. doi: 10.3389/fbirs.2024.1385017
Received: 11 February 2024; Accepted: 15 April 2024;
Published: 21 May 2024.
Edited by:
Cristian Pérez-Granados, University of Alicante, SpainReviewed by:
Paul Rose, University of Exeter, United KingdomCopyright © 2024 Guggenberger, Boonman, Keynan and Yovel. This is an open-access article distributed under the terms of the Creative Commons Attribution License (CC BY). The use, distribution or reproduction in other forums is permitted, provided the original author(s) and the copyright owner(s) are credited and that the original publication in this journal is cited, in accordance with accepted academic practice. No use, distribution or reproduction is permitted which does not comply with these terms.
*Correspondence: Marie Guggenberger, Z3VnZ2VuYmVyZ2VyQG1haWwudGF1LmFjLmls
Disclaimer: All claims expressed in this article are solely those of the authors and do not necessarily represent those of their affiliated organizations, or those of the publisher, the editors and the reviewers. Any product that may be evaluated in this article or claim that may be made by its manufacturer is not guaranteed or endorsed by the publisher.
Research integrity at Frontiers
Learn more about the work of our research integrity team to safeguard the quality of each article we publish.