- 1Department of Biology, New Mexico State University, Las Cruces, NM, United States
- 2Centro de Investigación en Neurociencias & Escuela de Biología, Universidad de Costa Rica, San José, Costa Rica
- 3Department of Biology, University of Pittsburgh at Johnstown, Johnstown, PA, United States
Introduction: Vocal dialects are a taxonomically widespread phenomenon which are typically only studied in a portion of a species’ range. Thus, it is difficult to infer whether a geographic pattern of vocal dialects observed in one part of a species’ range are typical across the range or whether local conditions influence their presence or absence. We examined the yellow-naped amazon, Amazona auropalliata, a parrot species with remarkable vocal learning capabilities. Although this species’ native range spans across Mesoamerica, only Costa Rican populations have been evaluated long-term. Previous studies have shown that these populations have geographically and temporally stable vocal dialect patterns. Without data on populations outside of Costa Rica, it is impossible to know whether vocal dialects are present in northern range populations, and whether they show similar geographic structure to southern range populations.
Introduction: We recorded yellow-naped amazon contact calls at 47 different sites across the species’ range between 2016 and 2019 and evaluated them for the presence of dialects. We visually classified 14 contact call types based on spectrographic similarity and used spectrographic cross-correlation, principal component analysis, and Mantel-based spatial autocorrelations to assess acoustic similarity; we also evaluated the robustness of our findings using simulated data.
Results and Discussion: The results from our study show that the vocal patterns previously seen in Costa Rica are also present in northern populations, supporting our hypothesis that this species has vocal dialects throughout its Mesoamerican range. Call types were regionally specific (e.g., vocal dialects occurred) across the range, and no call types were repeated across multiple regions. We did, however, observe distinctive structural characteristics that are found in multiple call types, suggesting that different call types stem from a common origin. Alternatively, similarity in the acoustic features of call types may also be a result of physiological and anatomical features that are common to all members of the species. Vocal dialects in this species are likely maintained through a tendency toward philopatry and matching call types to enhance social identification.
1 Introduction
Vocal learning, defined as the ability to replicate a sound produced by a conspecific or other species based on social experience (Bradbury and Balsby, 2016; Tyack, 2020), can generate high levels of variation in acoustic structure. Cultural transmission of vocal traits occurs when naïve individuals in a population match their vocal behavior to a template observed from conspecifics, such as parents or contemporaries (Whitehead, 2010; Whiten, 2019). Imperfect learning of these traits can lead to changes in the behaviors of a population, a phenomenon known as cultural drift (Podos and Warren, 2007). When acted upon in conjunction with geographic or reproductive isolation, cultural drift in the context of learned vocalizations can produce vocal dialects, a distinct mosaic pattern of geographic variation in acoustic signals (Wright et al., 2008; Martins et al., 2018). Research on vocal dialects in wild populations is usually limited to a portion of a species’ range (but see Kroodsma et al., 1999). Thus, it is generally unclear whether vocal dialect formation is intrinsic to a species or dependent on local conditions.
Since vocal dialects were first documented in the white-crowned sparrow, Zonotrichia leucophrys (Marler and Tamura, 1964), they have been identified in a much wider array of taxa, including whales (Deecke et al., 2000), hummingbirds (Wiley, 1971), seals (Casey et al., 2018), bats (Boughman and Wilkinson, 1998), and parrots (Wright, 1996). Despite this wide taxonomic distribution, songbirds have remained the focal point of research regarding both vocal learning (Beecher and Brenowitz, 2005; MacDougall-Shackleton, 2009) and vocal dialects (West and King, 1985; Maney et al., 2003; Pipek et al., 2018; Loo and Cain, 2021). Parrots are well-known for their vocal mimicry skills in captivity and there is increasing evidence that under natural conditions this open-ended learning results in a number of different patterns of variation, including vocal dialects, clinal variation, and individually-specific vocal signatures (Wright and Dahlin, 2018; Smith-Vidaurre et al., 2021; Smeele et al., 2023).
Yellow-naped amazons are large, social parrots that occur within lowland, seasonally dry tropical forests along both the Pacific and Caribbean coasts of Mesoamerica, ranging from southern Mexico to northern Costa Rica (Wright et al., 2018). They exhibit fission-fusion flock dynamics, communally roosting in the evenings and departing in smaller groups around sunrise to forage (Wright, 1999). The first evidence of vocal dialects in a parrot was documented in Costa Rican populations of this species (Wright, 1996). In 2005, Wright et al. (2008) recorded contact calls in 2005 at the same sites as previously recorded in Costa Rica and found that the acoustic variation discovered in 1996 was temporally and geographically stable. During both the 1996 and 2005 surveys, some birds were recorded using multiple dialects, known as bilingualism, at some sites along dialect boundaries (Wright, 1996; Wright et al., 2008). Genetic analysis showed that yellow-naped amazon vocal dialects are not genetically distinct (Wright and Wilkinson, 2001), suggesting that this species preferentially learns local call types (Wright et al., 2008), a conclusion supported by translocation experiments (Salinas-Melgoza and Wright, 2012). In addition, playback experiments showed that members of this species are less responsive to playbacks from different dialects (Wright and Dorin, 2001). These data indicate that yellow-naped amazon dialects may be maintained over time because using the proper dialect provides social benefits to individuals (Wright and Dahlin, 2018).
At present, there is little data to indicate whether yellow-naped amazon vocal dialects occur only in Costa Rica and neighboring populations or extend throughout the species’ range. Furthermore, it remains unclear whether patterns of geographic structure exist above the dialect level in this species. We hypothesized that vocal dialect patterns observed in the southern portion of the range would also be present in the northern portion. To address this question, we recorded contact calls from yellow-naped amazon populations in Costa Rica, Nicaragua, Honduras, Guatemala, and Mexico from 2016-2019. We expected to see a mosaic pattern of geographic variation in the contact calls, whereby calls within populations are more similar in their acoustic structure than between populations (Wright and Dahlin, 2018). Alternatively, variation in yellow-naped amazon contact calls could be clinal. In this case, we would expect to see the acoustic structure of calls progressively diverge from one end of the range to the other (Lee et al., 2019). We used spectrographic cross-correlation, PCA, and Mantel-based spatial autocorrelations to assess these two alternatives. In addition, we compared the fit of models predicting vocal variation based on our visual dialect classification to a model based on geographic distance alone, and evaluated the robustness of the conclusions using data simulating either clinal or dialectal variation.
2 Materials and methods
2.1 Data collection
We recorded the contact calls of unmarked and unsexed yellow-naped amazons occurring in and around the same roost sites at which population sizes were surveyed by Dupin et al. (2020). Contact calls are the most frequently emitted acoustic signal in this species, particularly in and around the roost (Wright, 1996). We recorded calls during the morning as individuals left night roosts or in the evening as they arrived. We recorded calls from both flying and perched birds as this species is known to use contact calls in both contexts (Wright, 1996). We aimed to record a minimum of 10 calls each from six different birds at each site. Calls were recorded using a Sennheiser ME67 shotgun microphone and a Marantz PMD660 solid state digital recorder at a frequency of 22.05 kHz, with an amplitude resolution of 16 bits. Individuals were differentiated in recordings via detailed dictation by the recordist. This project was approved by New Mexico State University’s Institutional Animal Care and Use Committee (IACUC protocols 2016-023 and 2018-009).
2.2 Sampling sites
We recorded contact calls from yellow-naped amazons during June and July of 2016, 2018, and 2019; both months fall just after the species’ breeding season (Matuzak and Brightsmith, 2007; Dahlin et al., 2018). Sites were chosen based on previous survey history, local anecdotes, information from local organizations working with yellow-naped amazons, and eBird reports. We attempted to record contact calls at as many locations as logistically possible where yellow-naped amazon populations were known to exist at the time of sampling, or were historically present (Dahlin et al., 2018). In 2016, we recorded calls at 22 sites in Costa Rica and 9 in Nicaragua, in 2018 and 2019 we recorded at 10 sites in southern Mexico, as well as 2 sites in Guatemala during 2019, and 4 sites in the Bay Islands, Honduras in 2019; one site in the Bay Islands was a private location which remains undisclosed at the owner’s request. In total, we recorded contact calls at 47 sites across the yellow-naped amazon range (Figure 1). We were unable to record calls from a reportedly small population on the Pacific coast of El Salvador, and larger populations reported to occur along the Caribbean coast of Honduras and Nicaragua due to logistical challenges.
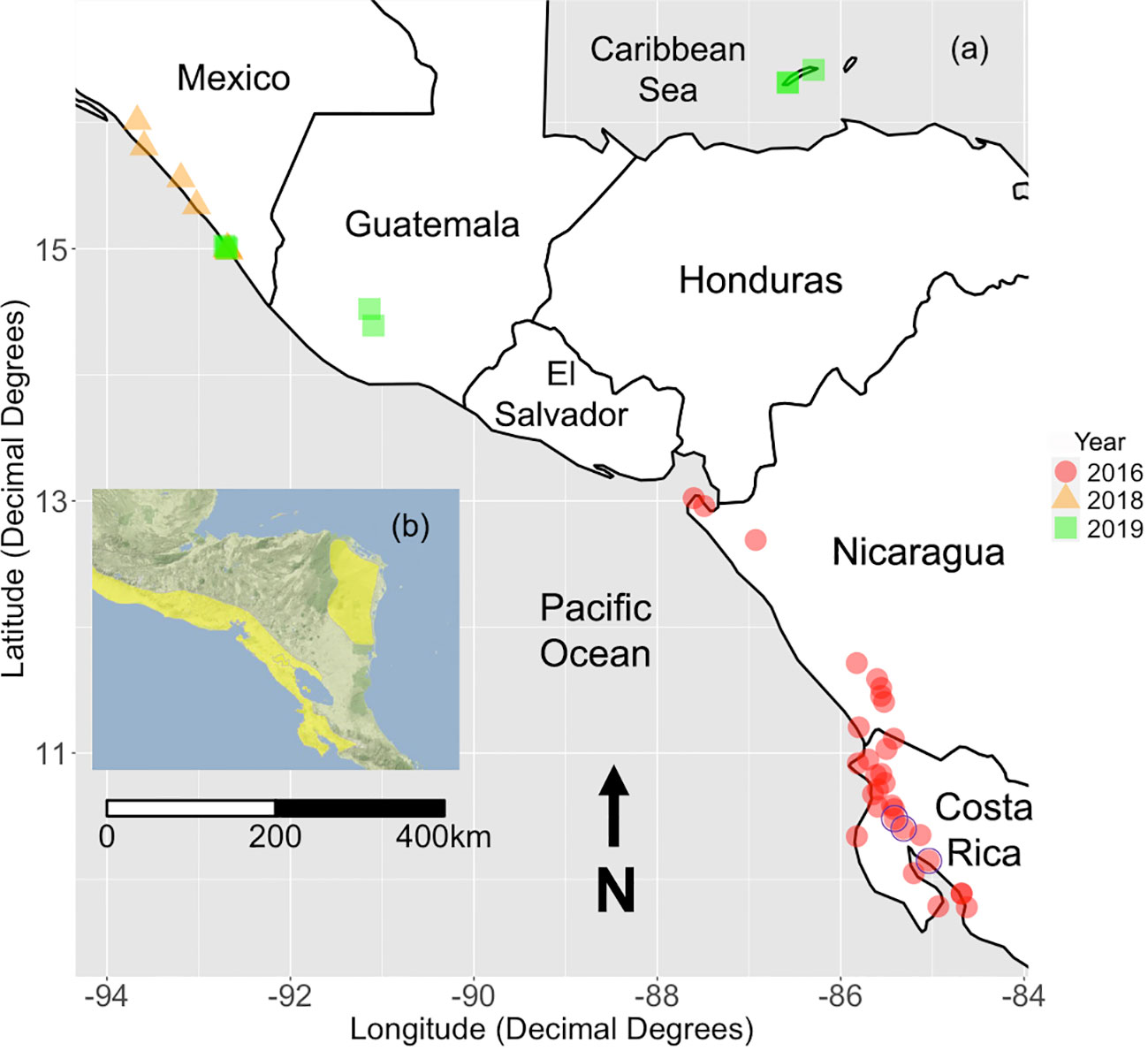
Figure 1 (A) A map of all sites where 3 or more birds were recorded during 2018 and 2019. Sites from the 2016 survey were also included (Wright et al., 2019). The color and shape of each point corresponds to the year the site was sampled. Purple circles represent sites with fewer than 3 birds that were removed from the dataset prior to analysis. The undisclosed site located in Honduras is not shown. (B) A species range polygon for the yellownaped amazon provided by BirdLife (BirdLife International and Handbook of the Birds of the World, 2019). Adapted from Dupin et al., (2020) under CC BY, and BirdLife International and Handbook of the Birds of the World (2019).
2.3 Data processing
Contact calls were processed digitally and visualized using the sound analysis program Raven Pro version 1.5. Calls from each field site were selected for analysis after a visual assessment of the original sound files and identification of one or more local types. Contact calls in yellow-naped amazons are typically short (0.2 - 0.5 seconds), monosyllabic, have a dominant frequency around 2 kHz, and are repeated in long strings by birds while perched and in flight; thus, calls across the range were identified as contact calls when they fit these patterns. After all contact calls within each file were identified, the highest quality calls were selected by manually annotating the start and end times on the spectrograms using Raven.
2.4 Visual assessment of call types
Annotations from Raven were imported into R for further analysis using the function imp_raven from the package Rraven (Araya-Salas, 2020). We visually assessed variation in call structure by creating a spectrogram catalog of all calls using the catalog function from the warbleR package (Araya-Salas and Smith-Vidaurre, 2017) (see Data Availability). To do this, we used a window length of 512, overlap of 85%, and a bandpass filter with low and high frequencies of 0.5 kHz and 2.5 kHz, respectively. We chose these limits to filter out low and high frequency background noise such as traffic, insects, and other bird species.
Each contact call was categorized as being of low, medium, or high signal quality by a single observer (MG). Low quality calls were those that had high levels of noise interference, overlap with another call, and/or had no visible harmonic bands in the signal; calls of medium quality had some visible bands but lacked higher-frequency harmonics and might contain some noise interference. High quality calls had multiple, dark harmonic bands and no noise interference. Each spectrogram was evaluated for the presence of overlap with other calls, and whether a 2.5 kHz upper frequency limit excluded any overlapping signal or background noise. All low-quality calls were removed from the dataset along with calls that overlapped with another signal. Call selections that included noise even after the bandpass filtering were examined individually to determine whether that noise would interfere with the analysis. If noise did not overlap substantially with the call, it was retained for further analysis.
After our visual assessment, we evaluated the number of remaining calls per bird at each site, and the number of birds at each site. Birds with fewer than 3 calls and sites with fewer than 3 birds were removed from the dataset (Supplementary Table 1). We adjusted the start and end times of the remaining calls using the seltailor function from the warbleR package in R (Araya-Salas & Smith-Vidaurre, 2017) (see Data Availability). Each call was then categorized based on its acoustic structure; calls with noticeably different acoustic structures were categorized as distinct call types. This method does require subjective judgement by observers, but has proven to be useful as a tool to assess the overall degree of difference between yellow-naped amazon call types, as described by Wright and colleagues in their previous studies on yellow-naped amazons (Wright, 1996; Wright et al., 2008). All calls were grouped by country for subsequent analyses to aid in visual assessment of results.
2.5 Statistical analyses
We used spectrographic cross-correlation (SPCC) to estimate pairwise dissimilarity across all calls using the cross_correlation function from the package warbleR in R (Araya-Salas & Smith-Vidaurre, 2017). Window length was set at 512, overlap was set at 85%, and low and high frequency bounds were set at 0.5 kHz and 2.5 kHz, respectively. Pairwise cross-correlation values were converted into vectors representing variation in acoustic structure using classic metric multidimensional scaling (hereafter, MDS) and Kruskal non-metric MDS plots. Kernel plots were then produced to show the distribution of each call type using concentric contour lines. The smallest circles indicate high concentrations of calls in one area of acoustic space. All code used for SPCC and MDS plots was modeled on code by Smith-Vidaurre et al. (2021) (see Data Availability).
We used principal component analysis on spectrographic features as an alternative approach to measure similarity between call types across the range. We accomplished this by first using the spectro_analysis function from the warbleR package in R (Araya-Salas and Smith-Vidaurre, 2017) to measure 26 acoustic parameters on each call, and then the prcomp function from the R stats package (R Core Team, 2021) to apply principal component analysis (PCA) to reduce dimensionality. We plotted the first 2 principal components for each region to compare to the MDS bidimensional plots (above). To test for equal variances, we used the function leveneTest() from the car package (Fox and Weisberg, 2019), and to evaluate the normality of our dataset we used the function shapiro.test() from the package MASS (Venables and Ripley, 2002). We evaluated differences among call types by performing Welch’s ANOVA using oneway.test() from the R stats package (R Core Team, 2021) on principle components 1 and 2 from our PCA output. To determine which call type comparisons showed significant differences, we conducted a Tukey post-hoc analysis on our ANOVA results using the posthocTGH() from the rosetta package (version 0.3.6) (Peters and Verboon, 2023).
We explored the relationship between geographic distance and acoustic similarity of contact calls using a custom routine based on the Mantel correlogram with the function mantel.correlog from the package vegan (version 2.5-7) (Oksanen et al., 2020). Mantel correlograms show the change in dissimilarity (in this case acoustic dissimilarity) as a function of spatial distance. Hence, they can be useful to assess patterns of variation in yellow-naped amazon calls across the species’ geographic range. Dissimilarity is typically measured over a fixed set of even spatial distances across a specified geographic range, but results can vary markedly for different spatial resolutions. Therefore, we calculated several Mantel correlograms with different spatial resolutions (6 distances from 25 to 250 km) and estimated the mean and standard deviations across the correlograms for each distance value after interpolation.
We tested whether distance or dialect assignment had a significant effect on call structure using a multiple regression model for distance matrices (Lichstein, 2007) in the function MRM from the R package ecodist (Goslee and Urban, 2007). This method uses a permutation approach to estimate statistical significance of the regression coefficients to account for the lack of independency of observations. A dissimilarity matrix based on the pairwise cross-correlation matrix (1 - spcc matrix) was used as a response variable while a pairwise distance matrix and a dialect membership binary matrix, were used as a predictor. The latter, created with the function binary_triangular_matrix in the R package PhenotypeSpace (Araya-Salas and Odom, 2022), uses 0s and 1s to represent whether two calls belong to the same dialect (0) or not (1), and therefore can be used to assess the variation in call structure explained by dialects.
Finally, we use simulations to evaluate whether our statistical approach could tease apart dialectal from clinal variation in call acoustic structure. For this, we created synthetic data sets with the same number of locations and calls per location as in the actual data set. Locations were equally spaced across the x axis (separated by one unit) in a bidimensional space. The position of each observation within each location was set randomly by adding random variation from a normal distribution (centered at 0 with standard deviation of 0.1) on both the x and y axis. For each pattern of acoustic variation we simulated a vector representing a hypothetical acoustic feature. The clinal geographic pattern was simulated by simply adding random variation (from a normal distribution centered at 0 with standard deviation of 0.2) to the x axis value of each observation. The dialectal geographic pattern was simulated by randomly assigning an integer number to each location (i.e. the same value to all observations within a location) and then adding random variation to each observation (from a normal distribution centered at 0 with standard deviation of 0.2). We also simulated a random variation pattern in which the acoustic feature value was assigned randomly regardless of locality of geographic distance to other observations. The three continuous simulated vectors (clinal, dialectal and random variation) as well as the geographic positions were converted into pairwise distance matrices using the function dist in R (R Core Team, 2021). Distances were zero-centered and transformed to unit variance to enable effect size comparisons between different models. As with the real data set, dialect membership was represented by a binary matrix that uses 0s and 1s to represent whether two calls belong to the same dialect (0) or not (1), created by the function binary_triangular_matrix from the package PhenotypeSpace (Araya-Salas and Odom, 2022). We also evaluated whether geographic distance and dialect membership (predictors) and the three acoustic feature variables (responses) were associated using multiple regression for distance matrices (Lichstein, 2007). Single response models were run for each acoustic feature variable. The simulation was replicated 100 times and the results were averaged across replicates.
3 Results
3.1 Contact calls and quality assessment
We surveyed for yellow-naped amazons at 72 locations in Mesoamerica where they are known to exist or have occurred historically. We observed this species at 56 of those sites and recorded 3 or more individuals at 47 sites (Supplementary Table 1). In 2016 our team recorded contact calls at 22 locations in Costa Rica and 9 in Nicaragua. We recorded calls at 10 sites in Mexico between 2018 and 2019. In 2019 we recorded contact calls at 2 sites in Guatemala and 4 in the Bay Islands of Honduras. We identified and selected 2,566 yellow-naped amazon contact calls from our field recordings and removed 116 of those during quality assessment due to the presence of noise overlap or low signal quality. In total, 24 birds were removed from the remaining dataset due to retention of fewer than three contact calls. Three sites from Costa Rica (Enseñada, Pelon Bajura, and Palo Verde) were removed as a result of having fewer than 3 birds (Supplementary Table 1). None of the other countries surveyed had sites removed during quality assessment.
We retained 2,338 yellow-naped amazon contact calls from our original dataset for analysis. We classified 21.5% of calls as medium quality, and 78.5% of calls as high quality. Contact calls from Mexico represented 23.3% of contact calls in the final dataset; Guatemala 1.5%; the Bay Islands, Honduras 9.3%; Nicaragua 23%; and Costa Rica 43% of all calls.
3.2 Call types across the range
Our visual classification of yellow-naped amazon contact calls yielded 14 different call types across the species range (Figure 2). We identified 4 types in Mexico and 2 types in Guatemala. In the Bay Islands Honduras, we found 2 distinct types. Previously, Wright et al. reported 3 call types in Costa Rica during a 1994 survey and the same 3 types and a new sub-type during a second survey in 2005 (Wright, 1996; Wright et al., 2008); our visual analysis of contact calls collected from these same regions during 2016 indicated that birds in Costa Rica gave 2 different call types, both of which were reported in the previous surveys. The third call type previously reported on the northern border of Costa Rica in 1994 and 2005 was not recorded there in 2016 but was seen in populations in the south of Nicaragua, along with 3 other call types across Nicaragua. Sixteen percent of the 243 birds in our dataset were bilingual. Overall, these observations were infrequent, and only 19 bilingual birds were retained in our dataset for analyses. These individuals were primarily concentrated in Costa Rica (N = 16), although bilingual birds were also recorded in Mexico (N = 2) and Guatemala (N = 1). It should be noted that we did observe and record bilingual birds in Nicaragua; however, none of those birds were preserved in our dataset due to low quality recordings or an insufficient number of recordings. Our visual assessment suggested that the mosaic patterns of vocal variation consistent with dialects was present throughout the range, rather than a clinal variation of call types. Dialect types in this species appear to result from discrete modifications to a basic call structure (Figure 3) that result in superficially similar call types appearing in different parts of the species range.
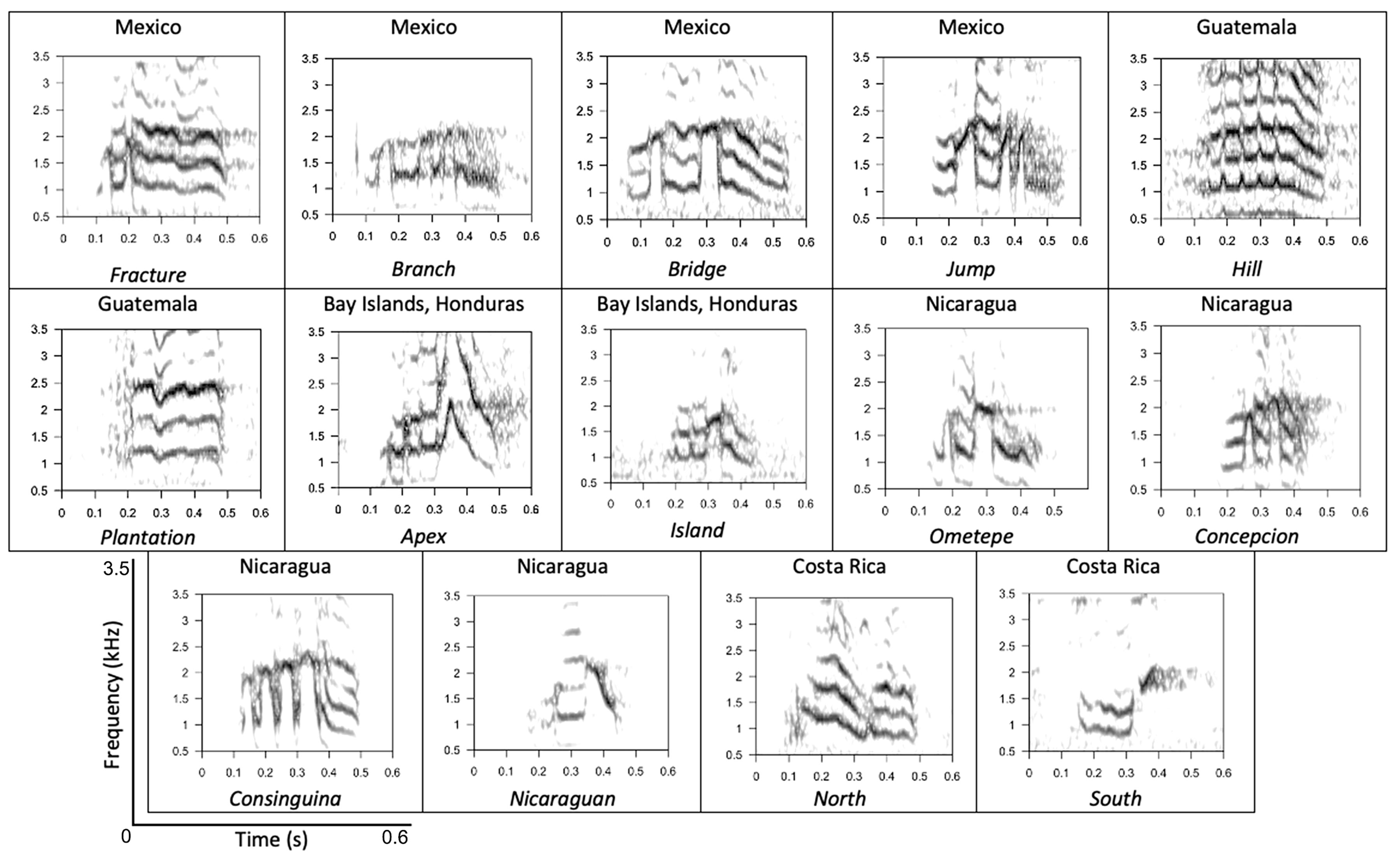
Figure 2 Spectrograms of each identified call type with the country of origin listed above and the call type name listed below the image. All images are shown on a 0.6 second timescale at 3.5 kHz frequency.
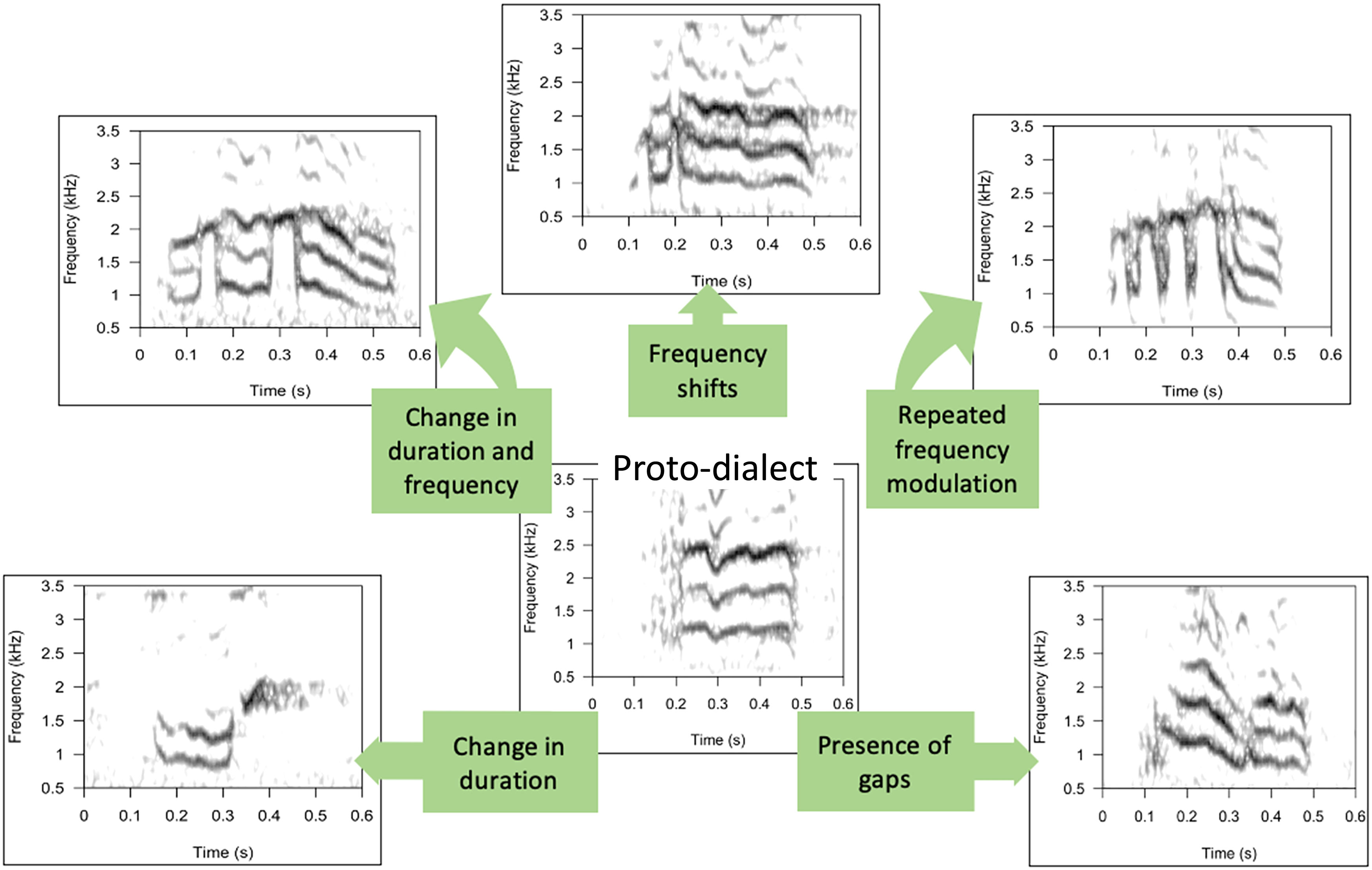
Figure 3 An image depicting typical structural variants of yellow-naped amazon contact calls and hypothesized routes by which one type might evolve into another through cultural drift.
3.3 Spectrographic cross-correlations
SPCC analyses for each country supported some, but not all of our call classifications for the yellow-naped amazon contact call types identified across the range (Figure 4). When all identified types from across the range were plotted in acoustic space, it was difficult to detect separate distributions as many overlapped to a significant extent. When we examined acoustic overlap on a country-level basis, we noticed that the types sampled in the northern (Mexico and Guatemala) and eastern (Bay Islands) portions of the range showed more acoustic separation among themselves than did call types in the southern (Nicaragua and Costa Rica) portion of the range, where dialects were first described in this species (see Supplementary Figure 1 for regional PCA plots).
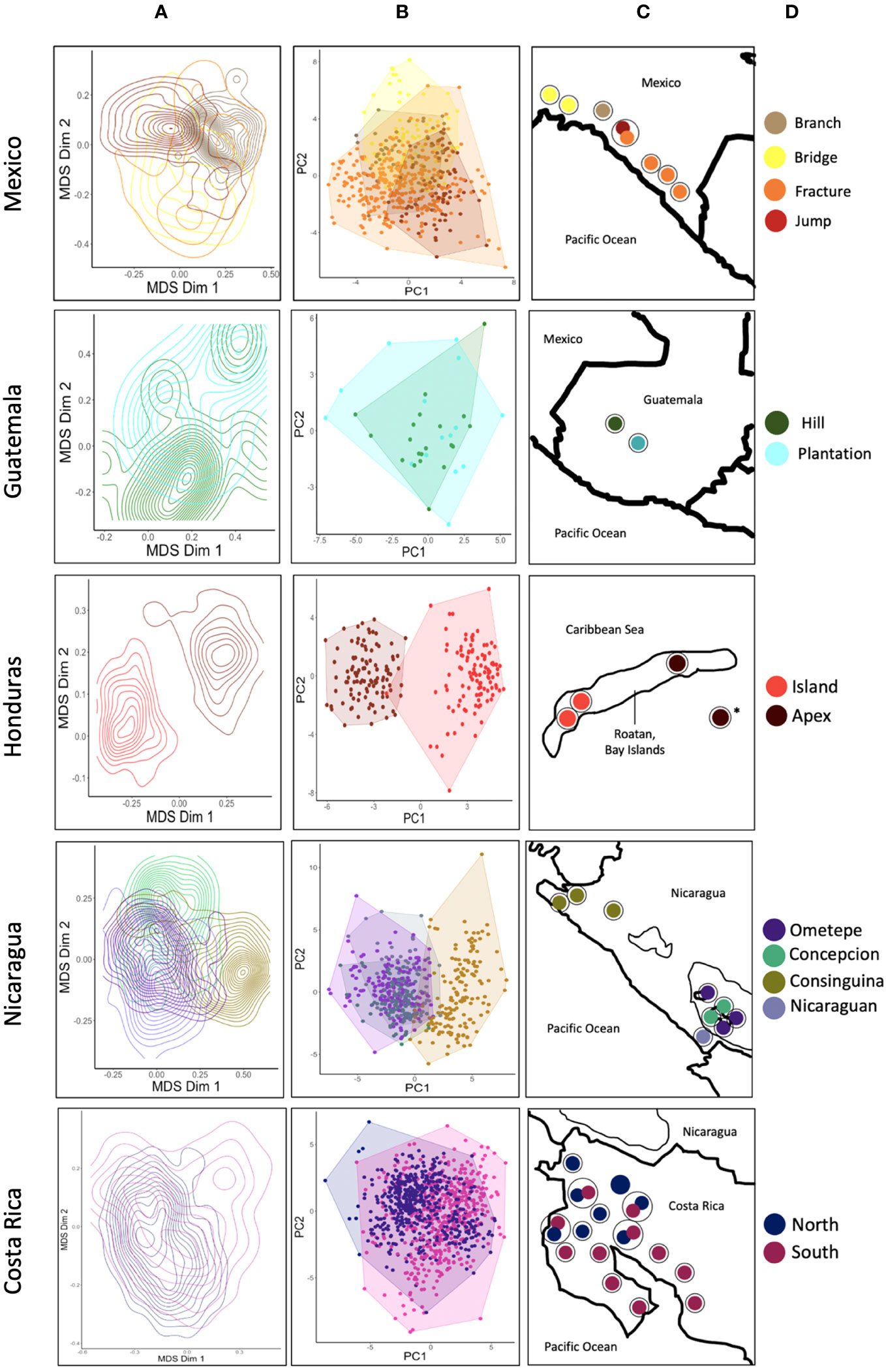
Figure 4 A multi-panel image with four columns showing SPCC plots (A), PCA plots (B), a geographic map (C), and a legend (D). Rows represent the sampled countries, arranged in order from north to south. The geographic map for Honduras depicts the call type for the undisclosed location using an asterisk (*). Black circles represent a single site.
3.4 Principal component analysis of spectral measures
We used PCA on spectral measures from all calls as an alternative measure of validation for our visually classified contact call types. The first 5 loadings from our analysis accounted for 77.4% of cumulative variation (Figure 4 and Supplementary Table 2). PC1 represented 26.7% of explained variation and primarily accounted for differences in frequency variables such as mean dominant frequency, and mean frequency (in kHz). PC2 explained 17.6% of variation and represented differences in temporal variables such as signal duration and duration of the call in the 75th quantile (Supplementary Table 2).
Plots of PC1 and PC2 for call types in each country generally reflected the patterns exhibited in the corresponding SPCC plots (Figure 4). When we created a PC plot for all call types identified across the range, there was little visual separation among call types (data not shown). An ANOVA on PC1 and PC2 for all call types combined did indicate significant differences between call types for both PC1 (F = 294.3, df = 299.41, p = < 0.001) and PC2 (F = 53.292, df = 300.36, p = < 0.001). Our Tukey post-hoc analysis on the ANOVA values from PC1 revealed that 74% of pairwise call type comparisons showed significant differences at the p < 0.001 level. Forty-one percent of pairwise call type comparisons from the Tukey post-hoc analysis on ANOVA values from PC2 showed significant differences at the p < 0.001 level (Supplementary Table 3).
3.5 Spatial patterns of acoustic similarity
The Mantel-based spatial autocorrelogram illustrated two distinct patterns in our data. First, within 250 km, yellow-naped amazon contact calls generally exhibit high similarity (Figure 5). Second, after 250km, acoustic similarity decreases markedly and remains at a low level for the remaining geographic distance range.
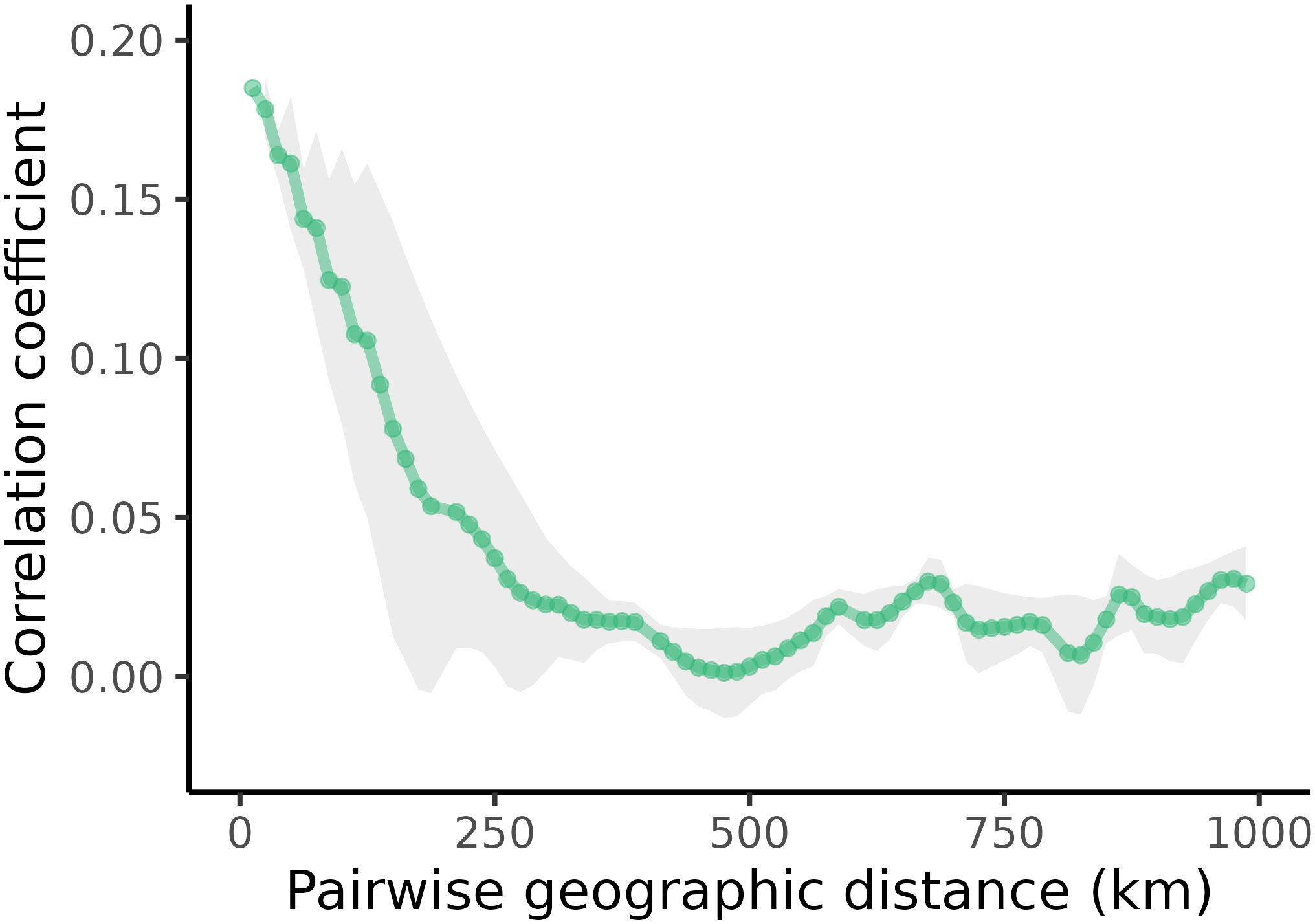
Figure 5 A Mantel-based spatial autocorrelogram depicting the acoustic similarity of calls against increasing geographic distance. Grey shading indicates the 95% confidence interval around the estimated correlation coefficient.
The multiple regression of distance matrices revealed a significant association between acoustic dissimilarity and dialect membership (p ≤ 0.0001) but no association with geographic distance (p = 0.281). Call dissimilarity was higher between calls from different dialects relative to calls from the same dialect. The effect size of dialect membership (0.65) was three orders of magnitude higher than the absolute effect size of geographic distance (-0.003).
The simulated data revealed that our statistical approach is capable of discerning between the two hypothesized patterns of geographic variation. When modeling acoustic similarity in clinal variation, the effect of geographic distance on acoustic similarity was consistently higher (effect size mean = 1.000; sd = 0.001) than that of dialect membership (effect size mean = -0.024; sd = 0.005). The opposite pattern was observed when predicting acoustic similarity in data simulating dialectal variation: the effect size of dialect membership was consistently higher (mean = 1.440; sd = 0.257) than that of geographic distance (mean = 0.020; sd = 0.178). In both simulations 95% of the replicates produced significant effects for both predictors. Finally, for the random variation simulation, geographic distance and dialect membership both produce equally low effect sizes (mean = -0.001; sd = 0.009 and mean = 0.001; sd = 0.016; respectively). Effects were significant in fewer than 95% of these replicated models.
4 Discussion
Historically, variation in the contact calls of yellow-naped amazons in Costa Rica has been described as dialectical, with a mosaic pattern of variation in which calls within a locale are similar but change dramatically in acoustic structure at dialect boundaries (Wright, 1996). Based on visual categorization of sound spectrograms, we defined 14 different call types confirming that the pattern of dialects previously seen in Costa Rica is present throughout the Mesoamerican range of this species. Currently wild yellow-naped amazons in many parts of their range are isolated into discrete regional populations due to loss of habitat and declining populations (Dupin et al., 2020). In each region we sampled, we were able to visually detect more than one acoustically discrete call type with a distinct geographic distribution. Our SPCC and PCA analyses validated many, but not all, of our visually identified acoustic types. Importantly, multiple regressions of distance matrices from our data gave stronger support for dialect variation than clinal variation, with effect sizes of similar magnitude to the same analysis run on data simulating dialect variation. In general, our data indicate that conditions which lead to dialect formation are intrinsic to the social structure and communication system of yellow-naped amazons, which appears to depend heavily on vocal learning.
4.1 Global patterns of vocal variation
In 1996, Wright identified 3 distinct contact call types in Costa Rican populations (Wright, 1996), and later confirmed the geographic and temporal stability of those same call types (Wright et al., 2005). Our visual assessment of over 2000 contact calls recorded from 44 sites across the range of the yellow-naped amazon revealed the presence of 14 distinct call types, including those identified in the initial description of dialects in Costa Rica. Results from our SPCC analyses and PCA support many of our visual classifications; however, they also indicate that several call types were acoustically similar. Overall, the SPCC and PCA plots showed more separation in the northern and eastern portions of the range (e.g the Apex and Island call types from the Bay Islanda). The southern portion of the range had more call type overlap (e.g. South and North call types in Costa Rica). This pattern may result from the repetition of similar acoustic structures in different parts of the range, or a lack of resolution in our acoustic analysis approaches. While SPCC is widely-used method of assessing acoustic similarity (Cortopassi and Bradbury, 2000; Sharp and Hatchwell, 2006; Araya-Salas et al., 2019) and has been used in several previous studies of acoustic variation in parrots (Wright, 1996; Wright et al., 2008), the limits of its capabilities should be considered. The cross-corr function from the package warbleR in R generates correlation coefficients by sliding one spectrogram over another and comparing amplitudes for each frequency and time point across each call (Clark et al., 1987; Araya-Salas and Smith-Vidaurre, 2017; Sawant et al., 2022). Thus, when call types use the same bandwidths and have very similar fundamental frequencies, the cross correlation will indicate that the calls are acoustically similar, even if there are visually detectable temporal and frequency modulation differences in the overall structure (Supplementary Figure 2). Although SPCC is considered to be somewhat robust to noise, field recordings may contain considerable acoustic interference from insects, other birds, vehicles, and farm animals. We implemented a bandpass filter to remove high and low frequency noise and discarded those recordings with substantial overlapping noise within the frequency range as the calls themselves but retained calls with lower levels of masking noise.
Several other parrot species have been found to exhibit vocal dialects, including the Australian ringneck parrot, Barnardius zonarius (Baker, 2000); galah, Eolophus roseicapillus (Baker, 2003); Australian palm cockatoo, Probosciger aterrimus (Keighley et al., 2017); Cuban parrot, Amazona leucocephala (Reynolds and Hayes, 2010); and invasive populations of the monk parakeet, Myiopsitta monachus (Buhrman-Deever et al., 2007; but see Smith-Vidaurre et al., 2021) for evidence of different patterns in native populations. There is little understanding of the process by which parrot species form vocal dialects, but several hypotheses have been suggested to explain their function (Wright and Dahlin, 2018). Podos and Warren (2007) postulated that female preference for local call types is a driver of acoustic divergence. In this case, female sexual preference for males with a local trait would result in some level of genetic divergence between populations (Wilkins et al., 2013). A study by Wright and Wilkinson (2001) reported no genetic correlation with dialects in yellow-naped amazon contact calls, stating instead that individuals exhibited high dispersal across dialect boundaries. Parrots may also exhibit vocal dialect as a badge or signal of group affiliation and familiarity (Sewall et al., 2016). Alternatively, geographic variation in dialects could be a consequence of cultural drift, when individuals learning calls make copying errors, and then are themselves copied accurately (Podos and Warren, 2007).
Overall, yellow-naped amazon vocal dialects are most consistent with a process of cultural drift. In general, we noticed that yellow-naped amazon contact calls exhibit some similar spectrographic structures in different call types across the range, such as the presence of gaps within a call, frequency shifts, and differences in duration. We observed that neighboring call types exhibit subtle differences in acoustic structure as opposed to whole-structure change. In conjunction, these facts suggest that call types have a common origin in a ‘proto-dialect’ call type (Figure 3), and that subsequent variation is a result of cultural drift, possibly in isolated populations. It is also possible that call types share similar acoustic features due to anatomical and physiological characteristics of the vocal control system that are common across all members of the species, and constrain vocal system modulations that produce sounds. Long-term studies by Wright and colleagues on yellow-naped amazons in Costa Rica lend credence to the cultural drift hypothesis (Wright et al., 2008; C. Dahlin et al. in prep). Additionally, there is support for the social identification hypothesis when call types are examined on a smaller scale. Wright and Dorin (2001) found marked differences in behavioral responses to playback of calls from neighboring versus same dialects. If dialects were solely a result of cultural drift, neighboring call types would show a strong tendency to be more similar and show clinal variation across the species’ range. The absence of strong clinal patterns suggest that there is some selection for neighboring populations to produce acoustically distinct call types, consistent with the hypothesis that calls function for group identification (Buhrman-Deever et al., 2007).
Several birds in our dataset exhibited more than one call type. In most cases of bilingualism there appeared to be a dominant call type used with high frequency by an individual, and a secondary type that a bird would emit infrequently. Wright reported the occasional occurrence of bilingual birds in Costa Rica during his 1994 survey, and Wright et al. observed them infrequently in Costa Rica during their 2005 survey (Wright, 1996; Wright et al., 2005). Bilingualism may exist in these populations to facilitate individual movement between social groups during fission and fusion. If, during foraging, individuals encounter conspecifics that give a different call type, learning the additional call type might increase foraging success and enhance protection provided by group membership. It is also possible that bilingualism occurs primarily in dispersing individuals that have learned one dialect in their natal roost and then dispersed to a roost with a different dialect, and learned the new dialect while retaining the original one (Salinas-Melgoza and Wright, 2012). In this case, bilingualism would have no functional advantage.
4.2 Regional patterns and implications for cultural evolution
Yellow-naped amazon populations currently occur in regional clusters across their range with few or no birds observed in between, due to rapid population declines associated with habitat loss and fragmentation (Dupin et al., 2020). This habitat fragmentation in the birds’ geographic range may serve to enhance the mosaic pattern of call distribution. However, there is no evidence that the current call types have arisen due to this recent isolation, and many of these population clusters contain more than one call type. It seems more likely that call types predate this isolation, as some of the earliest recordings from this area show distinct regional call types (see Wright and Wilkinson, 2001). These regional call types may be maintained by a tendency toward philopatry and selection toward matching regional types to enhance group identification, as suggested by playback responses of dialect variants of another vocalization type, pair duets (Wright and Dorin, 2001). In southern Nicaragua and Costa Rica, where yellow-naped amazon populations are more contiguous, many call types are restricted to a certain geographic area, while others are currently more widespread. In the northern-most country of Mexico, yellow-naped amazons are much more patchily distributed, which may explain why call types exhibit almost no acoustic overlap (Sewall et al., 2016).
Though we were able to sample populations across the yellow-naped amazons’ range, there are several regions we were unable to survey due to time, manpower, and safety limitations. These regions include the country of El Salvador, the southeastern Caribbean region of Honduras, and the northwestern Caribbean region of Nicaragua. Although we were not able to record birds in these areas, our data are likely to be an accurate representation of the pattern of vocal variation across the range as we were able to sample populations at the northern- and southern-most bounds and on both the Pacific and Caribbean slopes of Mesoamerica. A study by Herrera et al. (2020) estimated a population of 250 yellow-naped amazons in El Salvador. It is also likely that some healthy populations remain in the large, unsampled regions of Nicaragua and Honduras where forests are relatively intact and human populations are smaller. Future yellow-naped amazon dialect studies should focus on evaluating the temporal and geographic stability of call types across the range, including those unsampled populations.
4.3 Summary and future directions
Our cultural atlas of vocal variation in contact calls across the range of the yellow naped amazon supports the hypothesis that vocal dialects are present across the range of this species. Contact call types exhibited separation by geographic region with only rare cases of bilingual birds, a trend seen in original studies on Costa Rican populations. These results suggest that the factors that give rise to and maintain vocal dialects are inherent to the social structure and communication system of this species. Our observation of repeated structural similarities in geographically distinct acoustic variants suggests that calls may have diversified from a shared basic structure through the occurrence of learning errors and their subsequent cultural transmission in populations. Recent isolation of populations may reinforce these tendencies. Future studies on yellow-naped amazons could examine functional responses to dialect variants of contact calls, potentially in reintroduced populations that lack local dialect types.
Data availability statement
The datasets presented in this study can be found in online repositories. The names of the repository/repositories and accession number(s) can be found below: https://doi.org/10.5061/dryad.9cnp5hqq7.
Ethics statement
The animal study was approved by New Mexico State University’s Institutional Animal Care and Use Committee. The study was conducted in accordance with the local legislation and institutional requirements.
Author contributions
MG: Conceptualization, Data curation, Formal Analysis, Investigation, Methodology, Software, Visualization, Writing – original draft, Writing – review & editing. MA-S: Formal Analysis, Methodology, Visualization, Writing – review & editing, Software, Validation. CD: Formal Analysis, Methodology, Writing – review & editing, Conceptualization, Funding acquisition, Investigation, Resources, Supervision. TW: Conceptualization, Formal Analysis, Funding acquisition, Investigation, Methodology, Resources, Supervision, Writing – review & editing, Project administration, Visualization.
Funding
The author(s) declare financial support was received for the research, authorship, and/or publication of this article. This research was funded by the World Parrot Trust (grant date April 2018), and New Mexico State University’s College of Arts and Sciences.
Acknowledgments
We are very grateful to the colleagues that aided in the collection field data between 2016 and 2019: Alyssa Trimeloni, Dr. Grace Smith-Vidaurre, Dominique Hellmich, Lorena Cabada-Gomez, Carlos Campos, Dr. James Gilardi, Sam Williams and the Macaw Recovery Network, Eric Anderson, Edith Belen Jimenez Diaz, Cristina Contreras Meda, Candelario Giron Montes, Martín Lezama Lopez, and Cara Dunbar. We would also like to extend a thank you to the following organizations which facilitated in data collection via permission to record on their land: Area de Conservación Guanacaste, La Biosfera de la Encrucijada, and Finca Patrocinio and One Earth Organization. Also, thank you to Theodore Muka, Sophia Lasche, and Adam Hopper for providing feedback and editing.
Conflict of interest
The authors declare that the research was conducted in the absence of any commercial or financial relationships that could be construed as a potential conflict of interest.
Publisher’s note
All claims expressed in this article are solely those of the authors and do not necessarily represent those of their affiliated organizations, or those of the publisher, the editors and the reviewers. Any product that may be evaluated in this article, or claim that may be made by its manufacturer, is not guaranteed or endorsed by the publisher.
Supplementary material
The Supplementary Material for this article can be found online at: https://www.frontiersin.org/articles/10.3389/fbirs.2023.1266420/full#supplementary-material
References
Araya-Salas M. (2020). Rraven: connecting R and Raven bioacoustic software. R package version 1.0.9.
Araya-Salas M., Odom K. (2022). PhenotypeSpace: an R package to quantify and compare phenotypic trait spaces R package version 0.1.0.
Araya-Salas M., Smith-Vidaurre G. (2017). warbleR: an R package to streamline analysis of animal acoustic signals. Methods Ecol. Evol. 8, 2. doi: 10.1111/2041-210X.12624
Araya-Salas M., Smith-Vidaurre G., Mennill D. J., González-Gómez P. L., Cahill J., Wright T. F. (2019). Social group signatures in hummingbird displays provide evidence of co-occurrence of vocal and visual learning. Proc. R Soc. B. 286, 1903. doi: 10.1098/rspb.2019.0666
Baker M. C. (2000). Cultural diversification in the flight call of the Ringneck Parrot in Western Australia. Condor. 102, 4. doi: 10.2307/1370319
Baker M. C. (2003). Local similarity and geographic differences in a contact call of the Galah (Cacatua roseicapilla assimilis) in Western Australia. Emu 103, 3. doi: 10.1071/MU02035
Beecher M. D., Brenowitz E. A. (2005). Functional aspects of song learning in songbirds. Trends Ecol. Evol. 20, 3. doi: 10.1016/j.tree.2005.01.004
BirdLife International and Handbook of the Birds of the World (2019). Bird species distribution maps of the world. Version 2019.1. Available at: http://datazone.birdlife.org/species/requestdis.
Boughman J. W., Wilkinson G. S. (1998). Greater spear-nosed bats discriminate group mates by vocalizations. Anim. Behav. 55, 6. doi: 10.1006/anbe.1997.0721
Bradbury J. W., Balsby T. J. S. (2016). The functions of vocal learning in parrots. Behav. Ecol. Sociobiol. 70, 3. doi: 10.1007/s00265-016-2068-4
Buhrman-Deever S. C., Rappaport A. R., Bradbury J. W. (2007). Geographic variation in contact calls of feral North American populations of the Monk Parakeet. Condor. 109, 2. doi: 10.1093/condor/109.2.389
Casey C., Reichmuth C., Costa D. P., Boeuf B. (2018). The rise and fall of dialects in northern elephant seals. Proc. R Soc. B. 285, 1892. doi: 10.1098/RSPB.2018.2176
Clark C. W., Marler P., Beeman K. (1987). Quantitative analysis of animal vocal phonology: an application to swamp sparrow song. Ethology. 76, 2. doi: 10.1111/j.1439-0310.1987.tb00676.x
Cortopassi K. A., Bradbury J. W. (2000). The comparison of harmonically rich sounds using spectrographic cross-correlation and principal coordinates analysis. Bioacoustics. 11, 2. doi: 10.1080/09524622.2000.9753454
Dahlin C. R., Blake C., Rising J., Wright T. F. (2018). Long-term monitoring of Yellow-naped Amazons (Amazona auropalliata) in Costa Rica: breeding biology, duetting, and the negative impact of poaching. J. Field Ornithol. 89, 1. doi: 10.1111/jofo.12240
Deecke V. B., Ford J. K. B., Spong P. (2000). Dialect change in resident killer whales: Implications for vocal learning and cultural transmission. Anim. Behav. 60, 5. doi: 10.1006/anbe.2000.1454
Dupin M. K., Dahlin C. R., Wright T. F. (2020). Range-wide population assessment of the endangered yellow-naped amazon (Amazona auropalliata). Diversity. 12, 10. doi: 10.3390/d12100377
Fox J., Weisberg S. (2019). An R Companion to Applied Regression. 3rd ed. (Thousand Oaks, CA: Sage).
Goslee S. C., Urban D. L. (2007). The ecodist package for dissimilarity-based analysis of ecological data. J. Stat. Software 22, 1-19. doi: 10.18637/jss.v022.i07
Herrera N., Lara K., Funes C. (2020). Estado poblacional de la Lora Nuca Amarilla (Amazona auropalliata) en El Salvador. Zeledonia. 24, 1.
Keighley M. V., Langmore N. E., Zdenek C. N., Heinsohn R. (2017). Geographic variation in the vocalizations of Australian palm cockatoos (Probosciger aterrimus). Bioacoustics. 26, 1. doi: 10.1080/09524622.2016.1201778
Kroodsma D. E., Byers B. E., Halkin S. L., Hill C., Minis D., Bolsinger J. R., et al. (1999). Geographic variation in black-capped chickadee songs and singing behavior. Auk. 116, 2. doi: 10.2307/4089373
Lee J. H., Podos J., Sung H. C. (2019). Distinct patterns of geographic variation for different song components in Daurian Redstarts Phoenicurus auroreus. Bird Study. 66, 1. doi: 10.1080/00063657.2019.1614144
Lichstein J. W. (2007). Multiple regression on distance matrices: a multivariate spatial analysis tool. Plant Ecol. 188, 117-131. doi: 10.1007/s11258-006-9126-3
Loo Y. Y., Cain K. E. (2021). A call to expand avian vocal development research. Front. Ecol. Evol. 9. doi: 10.3389/fevo.2021.757972
MacDougall-Shackleton S. A. (2009). The importance of development: What songbirds can teach us. Can. J. Exp. Psychol. 63, 1. doi: 10.1037/a0015414
Maney D. L., MacDougall-Shackleton E. A., MacDougall-Shackleton S. A., Ball G. F., Hahn T. P. (2003). Immediate early gene response to hearing song correlates with receptive behavior and depends on dialect in a female songbird. J. Comp. Physiol. A. 189, 667-674. doi: 10.1007/s00359-003-0441-z
Marler P., Tamura M. (1964). Culturally transmitted patterns of vocal behavior in sparrows. Science, 146, 365. doi: 10.1126/science.146.3650.1483
Martins B. A., Rodrigues G. S. R., de Araújo C. B. (2018). Vocal dialects and their implications for bird reintroductions. Perspect. Ecol. Conserv. 16, 2. doi: 10.1016/j.pecon.2018.03.005
Matuzak G. D., Brightsmith D. J. (2007). Roosting of Yellow-naped Parrots in Costa Rica: estimating the size and recruitment of threatened populations. J. Field Ornithol. 78, 2. doi: 10.1111/j.1557-9263.2007.00099.x
Oksanen J., Blanchet F. G., Friendly M., Kindt R., Legendre P., McGlinn D., et al. (2020) Vegan: Community Ecology Package. Available at: https://CRAN.R-project.org/package=vegan.
Peters G.-J., Verboon P. (2023) Rosetta: Parallel use of statistical packages in teaching. Available at: https://r-packages.gitlab.io/rosetta/.
Pipek P., Petrusková T., Petrusek A., Diblíková L., Eaton M. A., Pyšek P. (2018). Dialects of an invasive songbird are preserved in its invaded but not native source range. Ecography. 41, 2. doi: 10.1111/ecog.02779
Podos J., Warren P. S. (2007). The evolution of geographic variation in birdsong. Adv. Stud. Behav. 37, 403-458. doi: 10.1016/S0065-3454(07)37009-5
R Core Team (2021). R: A Language and Environment for Statistical Computing (Vienna, Austria: R Foundation for Statistical Computing).
Reynolds M. B. J., Hayes W. K. (2010). Geographic variation in the flight call of the Cuban Parrot (Amazona leucocephala) and its taxonomic relevance. J. Caribbean Ornithol. 23, 1.
Salinas-Melgoza A., Wright T. F. (2012). Evidence for vocal learning and limited dispersal as dual mechanisms for dialect maintenance in a parrot. PloS One 7, 11. doi: 10.1371/journal.pone.0048667
Sawant S., Arvind C., Joshi V., Robin V. V. (2022). Spectrogram cross-correlation can be used to measure the complexity of bird vocalizations. Methods Ecol. Evol. 13, 2. doi: 10.1111/2041-210X.13765
Sewall K. B., Young A. M., Wright T. F. (2016). Social calls provide novel insights into the evolution of vocal learning. Anim. Behav. 120, 163-172. doi: 10.1016/j.anbehav.2016.07.031
Sharp S. P., Hatchwell B. J. (2006). Development of family specific contact calls in the Long-tailed Tit Aegithalos caudatus. Ibis. 148, 4. doi: 10.1111/j.1474-919X.2006.00568.x
Smeele S. Q., Senar J. C., Aplin L. M., McElreath M. B. (2023). Evidence for vocal signatures and voice-prints in a wild parrot. R. Soc Open Sci. 10, 230835. doi: 10.1098/rsos.230835
Smith-Vidaurre G., Araya-Salas M., Wright T. F. (2021). Individual signatures outweigh social group identity in contact calls of a communally nesting parrot. Behav. Ecol. 31, 2. doi: 10.1093/BEHECO/ARZ202
Tyack P. L. (2020). A taxonomy for vocal learning. Philo T R Soc. B. 375, 1789. doi: 10.1098/rstb.2018.0406
Venables W. N., Ripley B. D. (2002). Modern Applied Statistics with S. 4th ed. (New York: Springer).
West M. J., King A. P. (1985). Studying dialects in songbirds: Finding the common ground. Behav. Brain Sci. 8, 1. doi: 10.1017/S0140525X0001997X
Whitehead H. (2010). Conserving and managing animals that learn socially and share cultures. Learn Behav. 38, 3. doi: 10.3758/LB.38.3.329
Whiten A. (2019). Cultural evolution in animals. Annu. Rev. Ecol. Evol. S. 50, 27-48. doi: 10.1146/annurev-ecolsys-110218-025040
Wiley R. H. (1971). Song groups in a singing assembly of little hermits. Condor. 73, 1. doi: 10.2307/1366121
Wilkins M. R., Seddon N., Safran R. J. (2013). Evolutionary divergence in acoustic signals: Causes and consequences. Trends Ecol. Evol. 28, 3. doi: 10.1016/j.tree.2012.10.002
Wright T. F. (1996). Regional dialects in the contact call of a parrot. Proc. R Soc. B. 263, 1372. doi: 10.1098/rspb.1996.0128
Wright T. (1999). Vocal communication in wild populations of the yellow-naped amazon (Amazona auropalliata). AFA Watchbird. 26, 7–9.
Wright T. F., Dahlin C. R. (2018). Vocal dialects in parrots: Patterns and processes of cultural evolution. Emu. 118, 1. doi: 10.1080/01584197.2017.1379356
Wright T. F., Dahlin C. R., Salinas-Melgoza A. (2008). Stability and change in vocal dialects of the yellow-naped amazon. Anim. Behav. 76, 3. doi: 10.1016/j.anbehav.2008.03.025
Wright T. F., Dorin M. (2001). Pair duets in the yellow-naped amazon (Psittaciformes: Amazona auropalliata): responses to playbacks of different dialects. Ethology. 107, 2. doi: 10.1046/j.1439-0310.2001.00632.x
Wright T. F., Lewis T., Lezama-Lopez M., Smith-Vidaurre G., Dahlin C. (2018). Yellow-naped amazon (Amazona auropalliata) populations are markedly low and rapidly declining in Costa Rica and Nicaragua. Bird Conserv. Int. 29, 2. doi: 10.1017/S0959270918000114
Wright T. F., Rodriguez A. M., Fleischer R. C. (2005). Vocal dialects, sex-biased dispersal, and microsatellite population structure in the parrot Amazona auropalliata. Mol. Ecol. 14, 4. doi: 10.1111/j.1365-294X.2005.02466.x
Keywords: contact call, cultural atlas, geographic variation, vocal dialect, vocal learning, yellow-naped amazon
Citation: Genes MK, Araya-Salas M, Dahlin CR and Wright TF (2023) A cultural atlas of vocal variation: yellow-naped amazons exhibit contact call dialects throughout their Mesoamerican range. Front. Bird Sci. 2:1266420. doi: 10.3389/fbirs.2023.1266420
Received: 24 July 2023; Accepted: 30 October 2023;
Published: 17 November 2023.
Edited by:
Jiang Feng, Northeast Normal University, ChinaReviewed by:
Stefano Filacorda, University of Udine, ItalyHeather Williams, Williams College, United States
Copyright © 2023 Genes, Araya-Salas, Dahlin and Wright. This is an open-access article distributed under the terms of the Creative Commons Attribution License (CC BY). The use, distribution or reproduction in other forums is permitted, provided the original author(s) and the copyright owner(s) are credited and that the original publication in this journal is cited, in accordance with accepted academic practice. No use, distribution or reproduction is permitted which does not comply with these terms.
*Correspondence: Timothy F. Wright, d3JpZ2h0QG5tc3UuZWR1