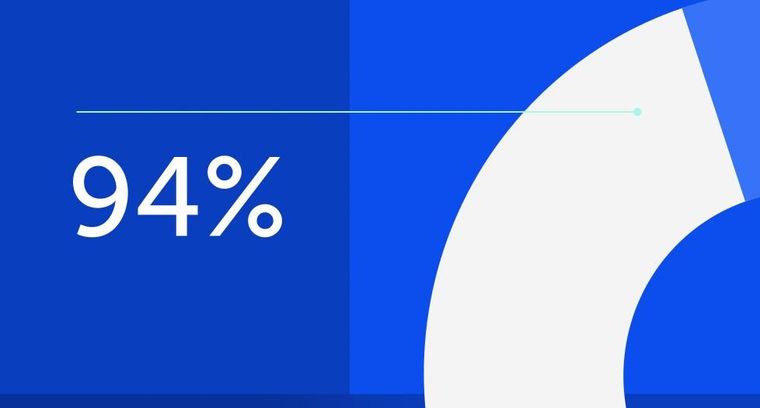
94% of researchers rate our articles as excellent or good
Learn more about the work of our research integrity team to safeguard the quality of each article we publish.
Find out more
ORIGINAL RESEARCH article
Front. Bird Sci., 27 October 2023
Sec. Bird Ecology and Behavior
Volume 2 - 2023 | https://doi.org/10.3389/fbirs.2023.1232737
This article is part of the Research TopicEditors' Showcase: Bird Ecology and BehaviorView all 6 articles
Long-distance avian migrants are threatened by a rapidly changing environment, which can induce a mismatch between favorable environmental conditions and the timing of breeding. Therefore, migratory birds must rely on phenotypic plasticity within migration timing to remain synchronized with earlier occurring resources over the shorter term. However, how this plasticity responds to en route environmental factors and whether or not it may allow migratory birds to remain synchronized with advancing springs is largely unknown. The objective of this study was to investigate how extrinsic factors, such as those related to the weather (e.g., temperature, precipitation, and wind speed) and daylength experienced along spring migration routes, and intrinsic factors, such as sex and age, migration departure date from wintering grounds, and migration destination (i.e., breeding site), influence the migration rate and spring arrival date at breeding grounds in purple martins (Progne subis). We investigated arrival timing and rate using 307 individual migration tracks sampled across the birds’ eastern breeding range. We found that the departure date and total stopover time explained most of the variation in migration rate, with birds departing later traveling faster but taking more stopover days, leading to a slower overall migration rate. To a lesser extent, facilitating southern winds and lower precipitation also induced a faster migration rate. We found that birds with a faster migration rate arrived earlier at their breeding sites. We also found that female birds traveling to more northern breeding sites arrived earlier than male birds, and that they migrated at a faster rate. Overall, our results suggest that the migration rate may be most impacted by intrinsic factors tied to spring departure dates that are aligned with the phenology of breeding sites rather than by conditions experienced en route. These findings support growing evidence that selection for spring departure date may be needed for the advancement of spring arrival date to match advancing springs with climate change. Future research investigating how environmental conditions at stopovers contribute to the overall migration rate would provide further insight into the factors influencing migration timing.
Changes in our climate are presenting new challenges to migratory bird species. Extreme and unpredictable weather conditions along migratory paths are predicted to increase the energetic costs of migration, and reduce individual survival during migration or at breeding sites (Newton, 2007; Bellard et al., 2012; Shipley et al., 2020). Moreover, earlier spring phenology driven by climate change can result in a mismatch with the spring arrival dates of migratory birds, which may lead to a decrease in overall fitness and, ultimately, population decline (Both and Visser, 2001; Both et al., 2006; Mayor et al., 2017). Arrival timing may also directly impact survival, that is, a precocious arrival relative to phenology may lead to direct mortality via heightened thermoregulatory costs and food scarcity (Lerche-Jørgensen et al., 2018). Long-distance migrants may be particularly vulnerable to rapid changes in spring phenology at their breeding sites as they may not experience environmental cues while at their tropical overwintering range (Knudsen et al., 2011). Phenotypic plasticity (i.e., the ability of an individual genotype to produce a variable phenotype in response to environmental variation; Fox et al., 2019) in migration timing can potentially ameliorate such a mismatch and optimize arrival timing (Bonamour et al., 2019; Fraser et al., 2019). Although our understanding of plasticity in migratory timing is developing, results to date suggest that the spring arrival date may be influenced by a combination of inherent timing (i.e., cueing departure date from overwintering grounds) and by variation in migration rate (Schmaljohann and Both, 2017). Selection for migration departure timing and rate can influence this plasticity in spring arrival dates, possibly through migration rate mediated by environmental conditions experienced while en route (Schmaljohann, 2019), yet technological limitations and sample size have generally limited the ability to investigate these interactions in songbirds across their full migrations.
Migration rate, which can be defined as the distance traveled per day (e.g., kilometers per day) during entire seasonal migrations (McKinnon et al., 2013), is most strongly correlated with stopover duration (Schmaljohann and Both, 2017), which is the sum of days a migrating bird remains relatively stationary for rest and refueling (i.e., a stopover) during migration. Variation in migration rate may be caused by intrinsic factors that vary by population (e.g., genetics, differing migratory paths, or length), from individual to individual (Nilsson et al., 2014), by sex and age, or by energetic condition (Alerstam, 2011; González et al., 2020). Geographic barrier crossing and associated refueling can induce longer or more frequent stopovers (Alerstam, 2011; Gómez et al., 2014), which may slow the migration rate.
Extrinsic factors within the environment may also impact migration as birds attempt to migrate with minimal energy losses (Wikelski et al., 2003; Alerstam, 2011). Weather can considerably impact the migration rate, as favorable weather associated with advanced spring phenology (e.g., warm temperatures and increased insect availability), tailwinds, and an absence of precipitation supports shorter stopovers or a faster migration rate (Wikelski et al., 2003; Haest et al., 2020; Åkesson and Bianco, 2021). Such environmental cues, through changes in migration rate, may drive phenotypic plasticity in spring arrival. Although most studies report little change in migration rate along routes (e.g., Nilsson et al., 2013), the rate is hypothesized to increase as birds approach their breeding site, owing to strong selection for earlier arrival via intraspecific competition (Alerstam, 2006), as demonstrated in a study of the long-distance migratory pied flycatcher (Ficedula hypoleuca) (Briedis et al., 2018). Seasonal change in daylength can be a critical migration cue and may also impact migration timing generally (Berthold, 1996). In diurnal migrants, longer photoperiods allow for longer duration of activity while migrating (Alerstam, 2009; Pokrovsky et al., 2021), which is predicted to lead to greater foraging ability during migration and fewer stopovers (or migration slowdowns) in a fly-and-forage migration strategy (Berthold et al., 2001; Alerstam, 2003; Alerstam, 2009). Numerous studies have examined migration timing at a specific point during migration, such as at arrival, departure, or specific stopovers (McKellar et al., 2013; Van Doren and Horton, 2018; Schmaljohann, 2019; Conklin et al., 2021; Lomas Vega et al., 2021; Tomotani et al., 2021). However, few studies have examined the entire migration process (Schmaljohann and Both, 2017; McKinnon and Love, 2018) and studies examining the interaction between timing, rate, and en route conditions in birds are also exceptionally rare (Haest et al., 2020).
In this study, we investigated the effects of en route extrinsic environmental variables (temperature, precipitation, wind speed, and daylength) and intrinsic factors (departure timing, geographic destination, age, and sex) on migration behavior in a long-distance migratory songbird, the purple martin (Progne subis). This swallow is a colonial breeder whose eastern subspecies (P. s. subis) is nearly entirely dependent on humanmade nest boxes (Brown et al., 2021). Martins exhibit high levels of variation in their year-to-year migration timing (Rohwer and Niles, 1977; Fraser et al., 2019), with an individual’s spring arrival dates occasionally differing by over 20 days (Fraser et al., 2019). Our specific objectives were to investigate the relative influence of intrinsic and extrinsic factors on (1) overall spring migration rate, (2) spring arrival date, and (3) variation in the spring migration rate between the southern (i.e., tropical) and northern (i.e., temperate) journey legs. As purple martins are nearly completely diurnal during their migration (Lavallée et al., 2021), longer daylengths as birds move further north may provide more time for migratory flights and foraging, thus increasing the birds’ migration rate. Previous research has found that migratory birds possibly accelerate as they approach their breeding sites (Alerstam, 2006; Briedis et al., 2018), which may be tied to changes in daylength (Pokrovsky et al., 2021), and may indicate a varying impact of extrinsic factors at different latitudes due to spring phenology.
We hypothesized that if long-distance migrants respond to both en route extrinsic and intrinsic factors, then favorable environmental factors would increase the migration rate of purple martins, especially when they were closer to the breeding grounds, and advance their arrival dates, and that intrinsic factors would have a varying influence on their migration rates and arrival dates. We also predicted that colder temperatures, opposing winds, and rainy conditions, would slow the spring migration rate and induce a later arrival timing (Wikelski et al., 2003; Van Doren and Horton, 2018; Haest et al., 2020). Moreover, compared with male birds and older birds, we expected that female birds and younger martins would migrate at a slower rate and arrive at a later date, due to protandry (i.e., male birds arrived earlier than female birds in spring; Coppack and Pulido, 2009) in songbirds and from differing levels of migratory experience between older and younger birds (Morton and Derrickson, 1990). We also predicted that later-departing birds would migrate faster, owing to selection to arrive more synchronously (competitively) at breeding sites with earlier-departing birds, and/or because environmental conditions in later spring facilitate faster flight (Fraser et al., 2019; González et al., 2020). For our third objective, we predicted that, in the northern leg of the migration journey, longer daylengths and conditions in proximity to the breeding grounds would lead to a faster migration rate than those associated with the journey leg at southern latitudes, in the regions of which there is little photoperiod variance.
Light-level geolocators (≤ 1.6 g; MK10s/12/12 s/14 s/20, British Antarctic Survey) were deployed between 2007 and 2019 across the purple martins’ breeding range (Fraser et al., 2012; Neufeld et al., 2021). Adult martins were captured using drop-door traps set up at the entrances of their nesting cavities during the nestling period. Tags were attached using leg-loop harnesses made of Teflon ribbon (Rappole and Tipton, 1991; Fraser et al., 2012) and weighed less than 5% of the body mass of the martins (Fair et al., 2010). Tags were retrieved in the year following deployment using the same capture method prior to incubation, including repeat-tracked individuals which carried different geolocators during each year that they were tracked. To maximize the likelihood of recapture and owing to a strong site fidelity, all birds tagged were breeding adults [(i.e., second-year and after-second-year birds, the age of which was determined using plumage characteristics (Pyle, 1997)], meaning that the spring migration tracks used in this study contained third-year (TY) and after-third-year (ATY) birds. The martins tagged showed high return rates that were comparable to the banding returns (Fraser et al., 2012; Neufeld et al., 2021), and resulted in 366 birds for analysis.
The birds tracked were sampled from 28 distinct breeding colonies distributed across latitudes (26°N to 53°N) in the US states and Canadian provinces of Alberta, Manitoba, Ontario, Minnesota, South Dakota, Pennsylvania, New Jersey, Virginia, South Carolina, Texas, and Florida (Figure 1, Supplemental Table 1). The purple martins were grouped into five latitudinal groups—based on the geographic destination of their spring migration—using the K-means clustering of their breeding colony latitude (Neufeld et al., 2021). We further divided the K-means cluster around 35°N of latitude into two distinct groups, resulting in the six following regions named by geographical location: Canadian prairies (~50°N), US prairies and Great Lakes (~45°N), Northeast coast (~40°N), semiarid Texas (~35°N), Southeast coast (~35°N), and the Gulf of Mexico coast (~30°N). The separation between the semiarid Texas and Southeast coast regions was carried out on the basis that they display large differences in migration timing (Table 1) and also show differences in climatic characteristics, in accordance with the Köppen–Geiger climate classification. The resulting semiarid Texas and Southeast coast regions were classified as BSk (arid, steppe, and cold) and Cfa (no dry season and warm summers), respectively (Beck et al., 2018).
Figure 1 Map of the breeding, migratory, and overwintering distributions of the eastern purple martin subspecies (Progne s. subis), showing the breeding colonies sampled with their respective latitudinal groups, five example spring migration paths, and the Tropic of Cancer (23.4°N), at which migration tracks were divided to provide southern and northern portions of migration. The figure using distribution maps adapted from BirdLife International and Handbook of the Birds of the World (2022) is available at http://datazone.birdlife.org/species/requestdis.
Of the 366 martins tracked, 59 were excluded from further analysis owing to poor-quality light data, resulting in 307 full-length spring migration tracks being available for further analysis (Supplemental Figure 2), including 25 individuals that had been tracked on more than one migration. Overall, our sample had a sex ratio of approximately four male birds to five female birds and an age ratio of approximately 1 TY bird to 5 ATY birds (Table 1). Within the regional groups, there were 74 birds in the Canadian prairies, 97 in the US prairies and Great Lakes, 55 in the Northeast coast, 18 in the semiarid Texas, 16 in the Southeast coast, and 47 in the Gulf of Mexico group. Sample size varied by regional groups and year owing to variation in tagging and recapture efforts.
The spring departure date from wintering grounds and spring arrival date at the breeding colony were determined using the GeoLight R package (version 2.0.0; Lisovski and Hahn, 2012) as described in Neufeld et al. (2021). Light data were manually verified for quality, and twilight (sunrise/sunset) outliers from shade or light pollution were adjusted based on adjacent twilights. A combination of the R packages GeoLight, BAStag (version 0.1.3; Wotherspoon et al., 2016), and FLightR (version 0.5.2; Rakhimberdiev et al., 2017) were used to create migration tracks. We used the template-fit method for the sun angle calibration (Rakhimberdiev et al., 2017). Migration tracks were composed of two location estimates per day, one made at 12:00 and one made at 00:00. The spatial error of these geolocators varies with the time of year and the latitude, but the spatial error is estimated to be up to approximately 200 km (Fraser et al., 2012).
Migration rates were calculated using the sp R package (version 1.4.6; Pebesma and Bivand, 2005), with the overall migration rate comprising every location along the migratory tracks from the departure date to the arrival date. We used the Tropic of Cancer (at a latitude of 23.4°N) as the midpoint to divide purple martins’ migration journeys into southern and northern legs; that is, one was a tropical region, in which temperature and daylength were fairly constant, and the other region was closer to breeding sites, in which temperatures and daylength varied much more (Figure 1). We calculated the migration rates for the southern and northern legs of each bird’s migration journey and we used a bird’s closest point to the Tropic of Cancer crossing to separate each bird’s southern and northern journey legs and in turn calculate their regional migration rate. Southern and northern migration legs exhibiting a distance traveled of <100 km and a duration of <1 day were excluded from further analysis, thus removing 14 sample tracks from the northern portion (1 from the Northeast coast, 2 from the Southeast coast, and 11 from the Gulf of Mexico coast). All migration track measurements and stopover analysis were conducted using R (version 3.6.1) in RStudio (version 1.2.1335), except for the migration rate during the southern and northern journey legs, which were calculated in different software versions (R version 4.1.2 and RStudio version 2021.09.1 + 372).
Spring stopover locations and durations were estimated using the FLightR stationary.migration.summary function with a probability of displacement cut-off of 0.1 (Rakhimberdiev et al., 2017). We defined stopovers as a time of rest in which a martin would take at least 1 day off from active migration, and used a minimum stay of three twilights, equivalent to roughly 36 hours. We then isolated tracks in which, when excluding the time spent at stopovers, the migration rate exceeded 1,000 km per day, which was the maximum daily travel speed found in purple martins using precise GPS tracking (Lavallée et al., 2021). Sustaining such a fast rate throughout migration, however, would be unrealistic biologically, and this result is probably due to the margins of error in the stopover analysis. For these tracks, we manually refined the stationary period estimates using the known spatial errors of geolocators (Fraser et al., 2012) and expected maximum daily travel distances for purple martins of 1,000 km per day (Lavallée et al., 2021). We considered points within a radius of 2° of the latitude and longitude (~220 km) as belonging to the same stopover site. Similarly, a part of the southern migration track (from the US prairies and Great Lakes region), in which martins exhibited a migration rate of over 1,000 km per day, was excluded from further analysis. To estimate the effect of stopovers on migration, while considering the wide variation in total migration duration among birds of the same breeding colony, we divided the stopover duration by the total migration duration, which enabled us to calculate the proportion of time a bird spent at stopover sites during their spring migration.
Individual migration tracks were annotated with environmental variables once at each location along the entire spring migration and at the time the location was collected (either at 12:00 or 00:00). Daylength was calculated using the geosphere R package (version 1.5.14; Forsythe et al., 1995), with daily tracking location information and the date used to estimate that day’s photoperiod (in R version 4.1.2 and RStudio 2021.09.1 + 372). However, this light measurement does not consider the absolute duration of daylight experienced by individual birds, which may fluctuate with cloud cover, light pollution, and habitat-dependent variations in daylight (caused by, for example, a tree canopy or mountain range). The temperature (°C) at 2 meters above ground, total hourly precipitation (mm), and wind speed (m/s) as meridional and zonal flows (i.e., the South to North and West to East wind vector components, respectively) at 10 meters above ground were annotated using the Env-DATA System on Movebank (movebank.org) (Dodge et al., 2013) from ERA5 Hourly Data on Single Levels provided by the European Centre for Medium-Range Weather Forecasts (ECMWF) Global Atmospheric Reanalysis (Hersbach et al., 2020). Meteorological values had a spatial granularity of 0.25° (~27.75 km) and temporal granularity of 1 hour. The total precipitation value was calculated as the 1-hour sum of rainfall for every location (at either 12:00 or 00:00). Although purple martins can occasionally forage at high altitudes, we expect environmental conditions at higher altitudes to greatly correlate with ground conditions, similar to those used for our weather measurements (within 10 meters above ground). Temperature and wind components had an inverse-distance-weighted interpolation and the total precipitation had a nearest-neighbor interpolation. Meridional and zonal wind components were used to calculate the average wind speed magnitude (); to these we manually added a sign of average wind favorability, which indicated if the direction of the average wind strength would facilitate spring migration. We denoted average south-facilitating winds as positive and average north headwinds as negative. Although the short temporal resolution for weather factors may not include all the variation in the weather of a particular day, those factors may be most accurate, especially for periods in which the tracked bird is actively migrating.
To investigate the relationship between en route extrinsic factors and intrinsic traits and (1) overall migration rate, (2) spring arrival date, and (3) southern and northern route migration rates, we used a series of generalized linear mixed models (hereafter GLMM) using glmmTMB (version 1.1.2.3; Brooks et al., 2017), in R (version 4.1.2) and RStudio (version 2021.09.1 + 372), with 95% confidence intervals (0.05 alpha value) to obtain estimates of significance. In every initial analysis, we used GLMMs with the individual and the year of deployment as random effects. Using the individual as a factor allowed us to consider the variation between individuals and also control for repeat tracks (n = 25) of the same bird. Using the year of deployment as a factor allowed us to account for unknown variation between sampling years. In all the models described below, both the individual and year of deployment random effects were considered and removed only for the purpose of model simplification due to convergence issues, or if the random effect was small. Marginal and conditional R2s were calculated using the R package modelsummary (version 0.9.6; Arel-Bundock, 2022) and were used to estimate the contribution of the explained variance of the model’s fixed and random effects.
The environmental factors of wind speed, temperature, precipitation, daylength, and migration timing (arrival date and departure date) all had some degree of collinearity among them (Supplemental Figure 1). To estimate and limit the contribution of collinearity in our models, fixed effects were tested with the variance inflation factor (VIF) using the performance r package (version 0.8.0; Lüdecke et al., 2021). When independent variables fell below a VIF threshold of 10 (Montgomery and Peck, 1992), the most collinear variables were excluded from the specific model using biological reasoning (Zuur et al., 2010).
The overall migration rate and spring arrival models used whole individual migration tracks to describe migration patterns; i.e., not localized responses to environmental factors but the total of these effects on the rate and timing of the whole journey. Therefore, we used the average temperature (°C), wind speed (m/s), and total precipitation (mm) measurements for all points along a route, including those at stopovers. We did not use daylength as a fixed effect for the overall migration rate and arrival date models because it correlated with both the spring departure date due to seasonality and the geographic destination of migration because of its relationship to latitude.
To investigate the effects of individual traits, timing of spring departure, and environmental factors on migration rate (1), we used a GLMM with the overall migration rate as our response variable. As fixed effects, we used the proportion of stopovers, age, sex, departure date, temperature, wind speed, precipitation, and latitudinal group of destination. As a random effect, we only included the year of deployment, removing the effect of the individual to allow the model to converge. In addition, we added a model omitting stopover duration as a covariate and using rate as a dependent variable to determine if its inclusion would control the variation in extrinsic variables (Supplemental Table 2), and we conducted a standardized model with the same intrinsic and extrinsic covariates, but with values that were scaled using the R package scales (version 1.2.1; Wickham and Seidel, 2022) to compare the magnitude of each estimate (Supplemental Table 3).
To investigate the effects of migration rate, individual traits, timing of spring departure, and environmental factors on the timing of spring arrival (2), we used a GLMM with the spring arrival date as our response variable. We used the individual and the year of deployment as random effects. The fixed effects were migration rate, age, sex, departure date, temperature, wind speed, precipitation, and latitudinal groups.
To investigate whether or not a bird’s response to intrinsic and extrinsic factors (including daylength) in migration rate varied between the southern and northern legs of their migration journey (3), we used two GLMMs with the regional (comprising those of the southern and northern journey legs) migration rate as our response variable. For the southern leg of the migration journey we used 306 samples and for the northern leg of the migration journey we used 293 samples; both sample groups included 25 repeat tracks (Figure 2). Initially, two-way interaction models including both the southern and northern journey were used, but these were replaced as they failed to converge. Since the regional migration rate had a skewed distribution, the response variable was logged using the natural log (ln) to meet our model assumptions. We fitted the two models for regional migration rate—one with the migration rate of the southern journey leg and one of the migration rate of the northern journey leg— with the age, sex, daylength, temperature, wind speed, precipitation, and latitudinal group of destination as the fixed effects. All environmental factors (i.e., daylength, temperature, wind speed, and total precipitation) were averaged within each region (in a manner similar to that described for the overall models above). In both regional models, we did not include the departure date because of its high collinearity with daylength. In terms of random effects, we used the year of deployment in both models and omitted the effect of the individual to allow the models to converge.
Figure 2 Raw variation of the migration rate by latitudinal region within the southern and northern legs of the migration journey. The statistical outliers that were included in the southern and northern legs of the migration journey models are shown.
Although all latitudinal groups had overlapping migration rates ranging from 121 km per day to 580 km per day, birds breeding in the Southeast coast region had slower average migration rates, which ranged from 109 km per day to 354 km per day (Table 1, Figure 3). Stopovers occurred between one and six times during spring migration, and stopover durations ranged from 36 hours to 47 days, with the stopover proportion (of total migration duration) ranging from 0.13 to 0.81. Spring migration timing varied with the latitude of breeding colony, with the wintering ground departure dates ranging from 10 January (Florida) to 12 May (Alberta), and arrival dates at the breeding site ranging from 28 January (Florida) to 9 June (Alberta) (Table 1, Supplemental Table 1). During their migration, migrating martins experienced average weather conditions that ranged from 18.5°C to 28.3°C for temperature (range of -2°C to +40°C), −5.0 m/s to 2.4 m/s for wind speed, and from 0.0 mm to 0.9 mm of rain for precipitation. The daylengths that birds experienced along their migration ranged from 10.7 hours for early-migrating birds breeding at low latitudes and 16.6 hours for late-migrating birds breeding at high latitudes.
We found a strong positive correlation between spring migration rate and the proportion of stopover days within a track (Table 2; Figure 4); for each 10% of the overall migration duration invested in stopover, the migration rate was 26.5 km per day slower when the other fixed effects were constant. For spring departure timing, on average, for every 10 days later that purple martins departed from their wintering grounds, the migration rate increased by 40 km per day (Figure 5A). Favorable winds led to a faster migration rate, that is, each increase of 1 m/s in southern wind speed increased the migration rate by 10.7 km per day (Figure 6A). For each 0.1-mm increase in total average precipitation, the migration rate slowed by −6.3 km per day (Figure 6B). Among migration destinations, we found that the migration rate in the Southeast coast region (South Carolina) was 53 km per day slower than that in the Canadian prairies region (Figure 3). The random effect of year had a variance of 449 km2 per day2, whereas the residual had a variance of 4,077 km2 per day2. For this model, the marginal R2 value was 0.41, and the conditional R2 value was 0.46 (Table 2).
Table 2 Result summary of the generalized linear mixed model (GLMM) of the migration strategy and en route weather experienced over spring migration rate of purple martins.
Figure 4 Raw linear correlation between the proportion of stopover duration during migration and resulting migration rate in purple martins, with all circles representing the migration of a different purple martin.
Figure 5 Raw graph of departure date (A) and the latitudinal destination (B) that drives most of the variation in spring migration rate in purple martins. The birds arriving earlier at their breeding site (B) or departing later (A) exhibit a faster rate during migration. The colored circles represent the different individuals tracked.
Figure 6 Raw correlation of spring migration rate with average en route (A) migration supporting southern winds and (B) total precipitation in purple martins.
In addition, in the model with standardized variables, we found that the proportion of stopovers and departure date have a greater impact on migration rate than average wind speed and precipitation (Supplemental Table 3). Indeed, the confidence intervals of the estimates for each of these variables were −0.47 to −0.29 for the proportion of stopovers, 0.05 to 0.38 for the departure date, 0.04 to 0.30 for wind speed, and −0.2 to −0.03 for precipitation.
All of the intrinsic factors considered, except age, had a statistically significant effect (p< 0.05) on the spring arrival date, but en route extrinsic factors did not significantly impact the arrival date (Table 3). We found that birds departing their overwintering grounds 1 day later would arrive 1 day later at their breeding site (Figure 5B). Faster migration rates resulted in earlier arrival dates, that is, a 15 km-per-day faster migration rate led to a spring arrival that was earlier by 1 day. We found an unexpected sex difference in migration timing, as male birds arrived at their breeding site 1.5 days later than female birds (i.e. protogyny) (Mills, 2005). Unsurprisingly, we also found that martins in all destinations had significantly different arrival dates compared to birds breeding in the Canadian prairies region. The variance of the random effects were 5.7 days2 for the individual, 0.1 days2 for the year of deployment, and 6.1 days2 for the residual. For this model, the marginal R2 value was 0.988, and the conditional R2 value was 0.994 (Table 3).
Table 3 Summary of results of the generalized linear mixed model (GLMM) of the migration strategy and en route weather experienced over the timing of spring arrival of purple martins to their breeding colonies.
In the southern migration leg model, we found a negative correlation between the migration rate of the southern journey leg and the average temperature (Table 4). The migration rate of the southern region slowed by −0.054 log km per day for each 1°C increase in the average temperature. The migration rate of the southern journey leg for birds of the Canadian prairies region was significantly different from that for birds in the Northeast coast, Southeast coast, and the Gulf of Mexico coast regions, by −0.14 log km per day, −0.62 log km per day, and −0.38 log km per day, respectively. In contrast, daylength did not significantly impact the birds’ migration rate over the southern routes. The random effect of year had a variance of 0.0007 [log(km/day)]2, whereas the residual had a variance of 0.0979 [log(km/day)]2. The marginal R2 value was 0.18, and the conditional R2 value was 0.19 (Table 4).
Table 4 Result summary of generalized linear mixed models (GLMM) for the log of spring migration rate in the southern leg of purple martins’ migration journeys.
In the northern migration leg model, we found a positive correlation between the migration rate of the northern journey leg and both the average daylength and average wind speed (Table 5). The migration rate in the northern region increased by 0.149 log km per day for each additional hour of daylength and increased by 0.031 log km per day on average for each increase in favorable wind speed of 1 m/s. Surprisingly, in the northern region, we found a difference in migration rates between sexes, with male birds migrating 0.130 log km per day less than female birds. We also found that in the northern region there were no significant differences between the latitudinal groups. Similar to what was observed in the other models, the year had a small variance of 0.0071 [log(km/day)]2 and the residual had a variance of 0.1580 [log(km/day)]2. The model resulted in a marginal R2 value of 0.20, with a conditional R2 value of 0.23 (Table 5).
Table 5 Result summary of generalized linear mixed models (GLMM) for the log of spring migration rate in the northern leg of purple martins’ migration journeys.
We investigated the influence of extrinsic and intrinsic factors on the spring migration rate, spring arrival date, and migration rates of southern vs. northern routes in purple martins using 307 individual migration tracks. First, we found that a later spring departure date from the wintering grounds and less time spent at stopovers were associated with a faster migration rate. To a lesser extent, favorable southern winds and lower precipitation also resulted in a faster migration rate, whereas there was no effect observed by our proxies for spring phenology and temperature, suggesting that there is little plasticity in these birds’ response to the en route environment. Second, an earlier spring arrival date at the breeding ground was driven by earlier departure date, faster migration rate, breeding location, and sex. Surprisingly, despite impacting migration rate to some degree, en route environmental factors did not have significant effects on arrival timing at the breeding grounds. Last, we found that migration rate within the southern leg of the migration varied only with temperature, whereas in the northern journey leg the migration rate increased with facilitating winds, longer daylength, and lower levels of precipitation. Although we did not directly measure phenotypic plasticity in this study, our results suggest that en route weather plays only a minor role in advancing spring migration timing overall or in explaining the within-individual variation reported in the study by Fraser et al. (2019). Overall, our findings suggest that the spring migration rate and spring arrival timing are driven mostly by intrinsic factors rather than extrinsic factors encountered en route. Our results support the notion that long-term advances in spring arrival timing as a result of climate change will be constrained by selection for the spring departure date (Ouwehand and Both, 2017; Schmaljohann and Both, 2017; Schmaljohann, 2019). Further research is required to determine the role of phenotypic plasticity and phenological microevolution in the long-term advances on spring timing noted for purple martins (Arab and Courter, 2015).
Although climate change advances phenology on average (in such a case, selection would be the best response mechanism), extreme weather during the spring may also drive greater variation in arrival dates (either by selection for inherent scheduling or by phenotypic plasticity, the levels of which may also be driven by selection). In cliff swallows (Petrochelidon pyrrhonota), Brown and Brown (1999) found a large shift in arrival dates in the year after an extreme selection event (early cold snap), providing evidence for short-term shifts in optimal timing. Moreover, timing phenotypes in purple martins are associated with genomic variation, suggesting phenological microevolution (de Greef et al., 2022). However, the repeat tracking of individual purple martins shows individual variability in timing (Fraser et al., 2019). The minor effect of weather on migration rate that was reported here may contribute to some of the individual variation reported in this species (Fraser et al., 2019), but additional factors should also be investigated, such as the role of parental effort and timing of nesting and overwintering conditions.
Our results suggest that the spring migration rate is dependent on intrinsic factors such as departure date, migration destination (latitude of the breeding location), some extrinsic factors, and stopover duration. Since the spring departure date from the wintering grounds and stopover duration had greater effects on the migration rate than did extrinsic variables, our results suggest that there is an intrinsically cued difference in rate between earlier- and later-departing birds, rather than that later birds encounter improved migratory conditions that increase their rate. The impact of the number of stopover days on overall migration rate was unsurprising, as longer stopovers have been associated with slower migration rates in other studies (Nilsson et al., 2013; Schmaljohann, 2019). When considering latitudinal groups, stopover duration, and environmental factors, we did not find increases in migration rate that were associated with breeding latitude. However, we found that the Southeast coast region displayed a slower migration rate than the other regions, which may reflect smaller, regional impacts on rate that could be further investigated in future studies.
We found overall that martins that departed earlier and those that migrated at a faster rate arrived earlier at their breeding colonies. However, we also found that birds that departed their overwintering grounds at later dates migrated at a faster rate than earlier-departing birds, which may indicate that later-departing martins are under stronger selection to travel at faster rates to arrive at similar times to earlier-departing birds, as arrival date may be critical to fitness (Kokko, 1999). This has also been described for Swainson’s thrush (Catharus ustulatus); it was observed that earlier-departing birds migrated at a slower rate than later-departing birds, meaning that both groups arrived at their breeding sites at a similar date (González et al., 2020). Although these changes in migration rate based on departure timing may be caused by favorable weather along the migration route, our models accounted for such extrinsic factors (e.g., temperature and wind speed), and these were kept constant, supporting the idea that departure date and possible accounted intrinsic factors intrinsic influences migration rate. These patterns may also be related to condition-dependent strategies, where birds that depart later are better prepared for migration with higher fuel loads (i.e., better conditions) (González et al., 2020). Our finding that martins that migrated at a slower rate spent a larger proportion of their time at stopovers, supports the idea that migration rate is directly influenced by the number of days spent resting during migration (Nilsson et al., 2014; Schmaljohann and Both, 2017). This aligns with previous work with varied groups of birds showing that stopover duration, rather than the pace maintained during flight, has the greatest impact on overall migration rate and duration (Schmaljohann and Both, 2017). It would be valuable to explore how en route environmental factors influence either stopover duration or the proportion of stopover to flight days in long-distance migrants (Beauchamp et al., 2020). For instance, aerial insectivores such as purple martins are sensitive to cold snaps (Brown, 1976), which can induce stopovers (Alerstam, 2011).
As predicted, we found that impeding weather, such as headwinds and precipitation, slowed migration rate. Optimal migration strategies for energy selection would result in the avoidance of contrary weather conditions for active migration (Alerstam, 2011). Facilitating winds, in contrast, would allow purple martins to cover greater grounds over the same duration of time and cross geographical barriers while saving energy (Abdulle and Fraser, 2018). Using GPS tracks, migrating common swifts (Apus apus), another aerial insectivore, were shown to take advantage of tailwinds to greatly increase their flight speed (Åkesson and Bianco, 2021) and thus increase their migration rate. Similarly, avoiding migrating during precipitation may avoid thermoregulatory losses and the additional weight from rain (Alerstam, 2011).
Despite the influence of environmental factors on en route rate, the spring arrival date itself was little influenced by environmental factors while birds were en route. We found that faster and earlier-departing birds arrived earlier at their breeding colony. Due to the variability of en route weather along migration routes and its effect on migration efficiency and stopover duration (Nilsson et al., 2013; Haest et al., 2020), it is usually assumed that migration timing varies depending on these en route conditions (Both, 2010; Conklin et al., 2021; Neufeld et al., 2021). For instance, in pied flycatchers (Ficedula hypoleuca), population-wide advancements in spring departure dates from wintering grounds did not result in earlier arrival dates, and it was inferred that this was caused by en route environmental factors limiting the migration rate (Both, 2010). Further contrasting our finding of environmental factors having little effect on migration timing, a study on six trans-Saharan long-distance songbirds found that environmental conditions during stopovers contributed to most of the variation observed in migration timing (Haest et al., 2020). The lack of impact of extrinsic factors on arrival date and their minimal impact on migration rate in purple martins supports the idea that migration rate cannot be increased, and that rate and timing are mainly impacted by the departure date and destination (Schmaljohann and Both, 2017). Thus, this long-distance migrant has limited plastic response to the environment. As such, in earlier springs, migratory birds may not be able to increase their migration rate in response to favorable en route environmental cues. Indeed, in response to a record-setting early and warm spring in 2012, purple martins did not advance their migration timing (Fraser et al., 2013). Alternatively, the lack of impact of en route environmental factors on arrival date at the breeding colony could be owing to en route plasticity to arrive at a time dictated by an inherent individual schedule. Some songbird species have shown remarkable individual consistency in individual timing behaviors across seasons (e.g., Stanley et al., 2012). Although purple martin timing was less repeatable individually (Fraser et al., 2019), future research could investigate the effect of stopover duration adjustments to local conditions which may influence migration rate. Although our results suggest that plastic responses play a minor role in advancing spring migration schedules in response to warmer temperatures, selection may act more directly on timing phenotypes if it is not masked by phenotypic plasticity (Fox et al., 2019). Therefore, changes to maintain an optimal arrival date brought about by climate change may induce selection pressure for martins to depart for spring migration earlier.
Environmental effects had differing influences on migration rate depending on the leg of the migration journey. In the southern leg of the migration journey, contrary to our expectations, we found that warmer temperatures correlated with a slower migration rate. Birds migrating through the tropics may avoid the costs of flying during high ambient temperatures (Bryant, 2008), which could slow migration rate through these regions. However, the impact of temperature on migration has mostly been studied for higher latitudes, at which bird thermoregulatory needs increase with colder temperatures (Alerstam, 2011), so further studies are required to understand how temperature affects migration at southern latitudes. In the northern leg of the migration journey, the migration rate of birds increased with supporting wind, reduced precipitation, and longer daylength. Given that we found that en route temperature had no effect on migration rate in the more northern leg of migration, our results suggest that sensitivity to warming temperatures with climate change is not a mechanism by which birds can advance their migration timing.
It is noteworthy that, as is found in all migration tracking studies where units must be retrieved, this study is biased for individuals that survived their migration and returned to their breeding colony. As such, birds that encountered extreme weather or migrated too early are underrepresented in the sample. Although a formal survival analysis has yet to be performed, purple martins have high site fidelity and return rates between tagged and untagged individuals (banded only) were similar (Fraser et al., 2012; Neufeld et al., 2021). Combined with a meta-analysis that demonstrated little impact of tagging on the survival of purple martins and other small songbirds (Brlík et al., 2020), likely reflecting a general pattern, we expect that our data represent the migration behaviors of individuals from our study colonies. Future work with purple martins and other small songbirds using miniaturized transmitting tracking tags will enable the inclusion of non-survivors to determine how their routes and timing may contribute to population-level variation.
Our finding that longer average daylength increased the migration rate was expected for a diurnal migrant that must allocate time to both fly and forage within daylight hours (Alerstam, 2009; Lavallée et al., 2021). The lack of effect of daylength on migration rate in the southern region, in which there is little variation in the photoperiod, further supports this. The effect of daylength on migrating passerines has been mostly theoretical, but direct tracking studies have found evidence of foraging time being combined with active migration in two large diurnal migratory bird species (Berthold et al., 2001; Klaassen et al., 2008). In our models, as daylength was correlated with temperature and date (as changing seasons are reflected in changes in daylength), we are unable to conclude that daylength has an independent effect on migration rate. To determine the effect of daylength on migration rate, future studies are needed to estimate the influence of daylength on daily flight distances while considering intrinsic and extrinsic factors such as wind speed, precipitation, temperature, and geographic barriers.
Unexpectedly, we found that female purple martins migrated faster than male purple martins in the northern leg of the spring migration journey and arrived earlier at their breeding sites than male birds when all other factors were kept constant. In songbirds, male birds typically arrived earlier to their breeding grounds than female birds (i.e. protandry), due to the strong selection for arrival of male birds for territorial selection (Morton and Derrickson, 1990; Kokko, 1999). However, Morton and Derrickson (1990) found that, although this trend was observed in both TY and older purple martins, second-year (SY) female birds showed an earlier arrival on average than SY male birds (i.e. protogyny). As our spring arrival date model keeps age (TY and ATY) constant (together with environmental factors and migration rate), our results do not include the interactions of sex with age. As male martins depart from their overwintering grounds earlier than female birds (Fraser et al., 2019), our finding that female birds migrated at a faster rate and arriving earlier may also relate to our finding suggesting that later-departing migrants migrate at faster rate (see above). It is possible that highly variable spring phenology early in the season at higher latitudes may prevent male martins from arriving earlier at the breeding grounds than female birds despite their head start, whereas later phenology may facilitate a faster migration rate in female birds and allow them to arrive at a similar or earlier date. The arrival dates of female birds may also be under strong selection because, upon arrival, female birds fight aggressively for nest cavity ownership. Interestingly, such fights over cavities are only observed among individuals of the same sex (Allen and Nice, 1952). We are unaware of past studies that indicate signs of protogyny in travel speeds and spring arrival in a songbird. Such relationships and assertions require more in-depth study in the future.
As for other aerial insectivores, population declines have been observed in purple martins in recent years (Sauer et al., 2017; Rosenberg et al., 2019). For its conservation, it is imperative to better understand how environmental factors influence this migrating species in a rapidly changing environment. Overall, our results show that departure date and destination have the greatest influence on migration rate and arrival dates, with en route environmental factors exerting only a minor influence. This suggests that these migratory birds may be under selective pressure to depart earlier from their wintering grounds to remain synchronized with advancing springs due to climate change, and that changes in migration rate are unlikely to have a strong influence on arrival timing, as has been suggested in recent studies (Ouwehand and Both, 2017; Schmaljohann and Both, 2017). The reliance on the slower process of natural selection for migration timing may render such long-distance migratory species increasingly vulnerable to advancing springs, as phenotypic mismatches may be accentuated over time if the selective change is unable to keep up with rapidly advancing springs (Radchuk et al., 2019). Since most of the variation in rate and spring arrival date is influenced by departure date and stopover duration, and not plastic responses to en route environmental variability, we suggest that the selection for earlier departure dates would be required to advance spring arrival timing at breeding sites in response to climate change.
The datasets presented in this study can be found in online repositories. The names of the repository/repositories and accession number(s) can be found below: https://www.movebank.org/cms/webapp?gwt_fragment=page=studies,path=study1765768285. Data available on Movebank.org (Movebank ID 1765768285).
The animal study was approved by the Animal Care Committee of the University of Manitoba (F18-031). The study was conducted in accordance with the local legislation and institutional requirements.
Hypotheses and study aims conceived by CT-vR and KF; CT-vR, SM, and KF designed the analyses; KF and collaborators collected the data; CT-vR performed the analysis with guidance from SM and KF; CT-vR wrote the first draft of the paper, with contributions and editing provided by KF and SM. All authors contributed to the article and approved the submitted version.
We thank the following funding agencies, which supported this research through grants and scholarships: the University of Manitoba, Natural Sciences and Engineering Research Council of Canada (NSERC) Discovery Grant Program (NSERC RGPIN-2017-04940), and the NSERC Canada Graduate Scholarships—Master’s program (CGS M) and Fonds de recherche du Québec—Nature et technologie (FRQNT) Master’s research scholarship (BX1).
All the data was gathered by current and past members of the Avian Behavioural Lab, partners, contributors, and volunteers, without whom this research would not have been possible. We also wish to thank the landlords, private and public organizations, and other contacts who granted us access to their grounds to monitor their purple martin colonies. We wish to thank Lakesha H. N. Smith for her help with reviewing the manuscript, with the geolocator analysis, and for making Figure 1; Supplemental Figure 2. We also wish to thank Saeedeh Bani Assadi, Leanne R. Neufeld, and Evelien de Greef for their work and help with the geolocator analysis. We also thank Debbie M. Kelly and Jim D. Roth, who provided fair criticism of and ideas for this research.
The authors declare that the research was conducted in the absence of any commercial or financial relationships that could be construed as a potential conflict of interest.
Author(s) KF declared that they were an editorial board member of Frontiers, at the time of submission. This had no impact on the peer review process and the final decision.
All claims expressed in this article are solely those of the authors and do not necessarily represent those of their affiliated organizations, or those of the publisher, the editors and the reviewers. Any product that may be evaluated in this article, or claim that may be made by its manufacturer, is not guaranteed or endorsed by the publisher.
The Supplementary Material for this article can be found online at: https://www.frontiersin.org/articles/10.3389/fbirs.2023.1232737/full#supplementary-material
Abdulle S. A., Fraser K. C. (2018). Does wind speed and direction influence timing and route of a trans-hemispheric migratory songbird (purple martin) at a migration barrier? Anim. Migration 5 (1), 49–58. doi: 10.1515/ami-2018-0005
Åkesson S., Bianco G. (2021). Wind-assisted sprint migration in northern swifts. iScience 24 (6). doi: 10.1016/j.isci.2021.102474
Alerstam T. (2003). “Bird Migration Speed,” in Avian Migration. Eds. Berthold P., Gwinner E., Sonnenschein E. (Berlin, Heidelberg: Springer Berlin Heidelberg), 253–267. doi: 10.1007/978-3-662-05957-9_17
Alerstam T. (2006). Strategies for the transition to breeding in time-selected bird migration. Ardea 94 (3), 347–357.
Alerstam T. (2009). Flight by night or day? Optimal daily timing of bird migration. J. Theor. Biol. 258 (4), 530–536. doi: 10.1016/j.jtbi.2009.01.020
Alerstam T. (2011). Optimal bird migration revisited. J. Ornithol. 152 (S1), 5–23. doi: 10.1007/s10336-011-0694-1
Allen R. W., Nice M. M. (1952). A study of the breeding biology of the purple martin (Progne subis). Am. Midland Nat. 47 (3), 606. doi: 10.2307/2422034
Arab A., Courter J. R. (2015). Spatio-temporal trend analysis of spring arrival data for migratory birds. Commun. Stat - Simul. Comput. 44 (10), 2535–2547. doi: 10.1080/03610918.2013.809100
Arel-Bundock V. (2022). modelsummary: data and model summaries in R. J. Stat. Softw. 103 (1), 1–23. doi: 10.18637/JSS.V103.I01
Beauchamp A. T., Guglielmo C. G., Morbey Y. E. (2020). Stopover refuelling, movement and departure decisions in the white-throated sparrow: The influence of intrinsic and extrinsic factors during spring migration. J. Anim. Ecol. 89, 2553–2566. doi: 10.1111/1365-2656.13315
Beck H. E., Zimmermann N. E., McVicar T. R., Vergopolan N., Berg A., Wood E. F. (2018). Present and future köppen-geiger climate classification maps at 1-km resolution. Sci. Data 5. doi: 10.1038/sdata.2018.214
Bellard C., Bertelsmeier C., Leadley P., Thuiller W., Courchamp F. (2012). Impacts of climate change on the future of biodiversity. Ecol. Lett. 15, 365–377. doi: 10.1111/j.1461-0248.2011.01736.x
Berthold P. (1996). “Circadian and Circannual Rhythms,” in Control of Bird Migration., 1st edn (Dordrecht, Netherlands: Springer Netherlands).
Berthold P., Bossche W. V. D., Fiedler W., Kaatz C., Kaatz M., Leshem Y., et al. (2001). Detection of a new important staging and wintering area of the White Stork Ciconia ciconia by satellite tracking. Ibis 143 (4), 450–455. doi: 10.1111/j.1474-919X.2001.tb04946.x
Bonamour S., Chevin L. M., Charmantier A., Teplitsky C. (2019). Phenotypic plasticity in response to climate change: The importance of cue variation. Philos. Trans. R. Soc. B: Biol. Sci. 374 (1768). doi: 10.1098/rstb.2018.0178
Both C. (2010). Flexibility of timing of avian migration to climate change masked by environmental constraints en route. Curr. Biol. 20 (3), 243–248. doi: 10.1016/j.cub.2009.11.074
Both C., Bouwhuis S., Lessells C. M., Visser M. E. (2006). Climate change and population declines in a long-distance migratory bird. Nature 441 (7089), 81–83. doi: 10.1038/nature04539
Both C., Visser M. E. (2001). Adjustment to climate change is constrained by arrival date in a long-distance migrant bird. Nature 411 (6835), 296–298. doi: 10.1038/35077063
Briedis M., Hahn S., Krist M., Adamík P. (2018). Finish with a sprint: Evidence for time-selected last leg of migration in a long-distance migratory songbird. Ecol. Evol. 8 (14), 6899–6908. doi: 10.1002/ece3.4206
Brlík V., Koleček J., Burgess M., Hahn S., Humple D., Krist M., et al. (2020). Weak effects of geolocators on small birds: a meta-analysis controlled for phylogeny and publication bias. J Anim Ecol 89 (1), 207–220. doi: 10.1111/1365-2656.12962
Brooks M. E., Kristensen K., Van Benthem K. J., Magnusson A., Berg C. W., Nielsen A., et al. (2017). glmmTMB Balances Speed and Flexibility Among Packages for Zero-inflated Generalized Linear Mixed Modeling. The R Journal 9 (2), 378–400. doi: 10.32614/RJ-2017-066
Brown C. R. (1976). Minimum temperature for feeding by Purple Martins. Wilson Bull. 88 (4), 672–673.
Brown C. R., Airola D. A., Tarof S. (2021). “Purple Martin (Progne subis),” in Birds of the World. Ed. Rodewald P. G. (Ithaca, NY, USA: Cornell Lab of Ornithology). doi: 10.2173/bow.purmar.02
Brown C. R., Brown M. B. (1999). Fitness components associated with laying date in the cliff swallow. Condor 101 (2), 230–245. doi: 10.2307/1369986
Bryant D. M. (2008). Heat stress in tropical birds: behavioural thermoregulation during flight. Ibis 125 (3), 313–323. doi: 10.1111/j.1474-919X.1983.tb03117.x
Conklin J. R., Lisovski S., Battley P. F. (2021). Advancement in long-distance bird migration through individual plasticity in departure. Nat. Commun. 12 (1). doi: 10.1038/s41467-021-25022-7
Coppack T., Pulido F. (2009). Proximate control and adaptive potential of protandrous migration in birds. Integr. Comp. Biol. 49 (5), 493–506. doi: 10.1093/icb/icp029
de Greef E., Brashear W., Delmore K. E., Fraser K. C. (2022). Population structure, patterns of natal dispersal and demographic history in a declining aerial insectivore, the purple martin Progne subis. J. Avian Biol. 2022 (6), 1–12. doi: 10.1111/jav.02929
Dodge S., Bohrer G., Weinzierl R., Davidson S. C., Kays R., Douglas D., et al. (2013). The environmental-data automated track annotation (Env-DATA) system: Linking animal tracks with environmental data. Move. Ecol. 1 (1). doi: 10.1186/2051-3933-1-3
Fair J., Paul E., Jones J. (2010). Guidelines to the Use of Wild Birds in Research. (Washington, D.C: The Ornithological Council).
Forsythe W. C., Rykiel E. J., Stahl R. S., Wu H. i., Schoolfield R. M. (1995). A model comparison for daylength as a function of latitude and day of year. Ecol. Model. 80 (1), 87–95. doi: 10.1016/0304-3800(94)00034-F
Fox R. J., Donelson J. M., Schunter C., Ravasi T., Gaitán-Espitia J. D. (2019). Beyond buying time: the role of plasticity in phenotypic adaptation to rapid environmental change. Philos. Trans. R. Soc. B: Biol. Sci. 374 (1768), 20180174. doi: 10.1098/rstb.2018.0174
Fraser K. C., Stutchbury B. J. M., Silverio C., Kramer P. M., Barrow J., Newstead D., et al. (2012). Continent-wide tracking to determine migratory connectivity and tropical habitat associations of a declining aerial insectivore. Proc. R. Soc. B: Biol. Sci. 279 (1749), 4901–4906. doi: 10.1098/rspb.2012.2207
Fraser K. C., Silverio C., Kramer P., Mickle N., Aeppli R., Stutchbury B. J. M. (2013). A trans-hemispheric migratory songbird does not advance spring schedules or increase migration rate in response to record-setting temperatures at breeding sites. PloS One 8 (5), 64587. doi: 10.1371/journal.pone.0064587
Fraser K. C., Shave A., de Greef E., Siegrist J., Garroway C. J. (2019). Individual variability in migration timing can explain long-term, population-level advances in a songbird. Front. Ecol. Evol. 7. doi: 10.3389/fevo.2019.00324
Gómez C., Bayly N. J., Rosenberg K. V. (2014). Fall stopover strategies of three species of thrush (Catharus) in northern South America. Auk 131 (4), 702–717. doi: 10.1642/AUK-14-56.1
González A. M., Bayly N. J., Hobson K. A. (2020). Earlier and slower or later and faster: Spring migration pace linked to departure time in a Neotropical migrant songbird. J. Anim. Ecol. 89 (12), 2840–2851. doi: 10.1111/1365-2656.13359
Haest B., Hüppop O., Bairlein F. (2020). Weather at the winter and stopover areas determines spring migration onset, progress, and advancements in Afro-Palearctic migrant birds. Proc. Natl. Acad. Sci. U.S.A. 117 (29), 17056–17062. doi: 10.1073/pnas.1920448117
Hersbach H., Bell B., Berrisford P., Hirahara S., Horányi A., Muñoz-Sabater J., et al. (2020). The ERA5 global reanalysis. Q. J. R. Meteorol. Soc. 146 (730), 1999–2049. doi: 10.1002/qj.3803
Klaassen R. H. G., Strandberg R., Hake M., Alerstam T. (2008). Flexibility in daily travel routines causes regional variation in bird migration speed. Behav. Ecol. Sociobiol. 62 (9), 1427–1432. doi: 10.1007/s00265-008-0572-x
Knudsen E., Lindén A., Both C., Jonzén N., Pulido F., Saino N., et al. (2011). Challenging claims in the study of migratory birds and climate change. Biol. Rev. 86 (4), 928–946. doi: 10.1111/J.1469-185X.2011.00179.X
Kokko H. (1999). Competition for early arrival in migratory birds. J. Anim. Ecol. 68 (5), 940–950. doi: 10.1046/j.1365-2656.1999.00343.x
Lavallée C. D., Assadi S. B., Korpach A. M., Ray J. D., Fischer J. D., Siegrist J., et al. (2021). The use of nocturnal flights for barrier crossing in a diurnally migrating songbird. Move. Ecol. 9 (1). doi: 10.1186/s40462-021-00257-7
Lerche-Jørgensen M., Korner-Nievergelt F., Tøttrup A. P., Willemoes M., Thorup K. (2018). Early returning long-distance migrant males do pay a survival cost. Ecol. Evol. 8 (23), 11434–11449. doi: 10.1002/ece3.4569
Lisovski S., Hahn S. (2012). GeoLight-processing and analysing light-based geolocator data in R. Methods Ecol Evol 3, 1055–105. doi: 10.1111/j.2041-210X.2012.00248.x
Lomas Vega M., Fransson T., Kullberg C. (2021). The effects of four decades of climate change on the breeding ecology of an avian sentinel species across a 1,500-km latitudinal gradient are stronger at high latitudes. Ecol. Evol. 11, 6233. doi: 10.1002/ece3.7459
Lüdecke D., Ben-Shachar M., Patil I., Waggoner P., Makowski D. (2021). performance: an R package for assessment, comparison and testing of statistical models. J. Open Source Softw. 6 (60), 3139. doi: 10.21105/joss.03139
Mayor S. J., Guralnick R. P., Tingley M. W., Otegui J., Withey J.C., Elmendorf S. C., et al. (2017). Increasing phenological asynchrony between spring green-up and arrival of migratory birds. Sci. Rep. 7 (1). doi: 10.1038/s41598-017-02045-z
McKellar A. E., Marra P. P., Hannon S. J., Studds C. E., Ratcliffe L. M. (2013). Winter rainfall predicts phenology in widely separated populations of a migrant songbird. Oecologia 172 (2), 595–605. doi: 10.1007/s00442-012-2520-8
McKinnon E. A., Fraser K. C., Stutchbury B. J. M. (2013). New discoveries in landbird migration using geolocators, and a flight plan for the future. Auk 130 (2), 211–222. doi: 10.1525/auk.2013.12226
McKinnon E. A., Love O. P. (2018). Ten years tracking the migrations of small landbirds: Lessons learned in the golden age of bio-logging. Auk 135, 834–856. doi: 10.1642/AUK-17-202.1
Mills A. M. (2005). Protogyny in autumn migration: do male birds "play chicken"? The Auk 122 (1), 71–81. doi: 10.1093/auk.122.1.71
Morton E. S., Derrickson K. C. (1990). The biological significance of age-specific return schedules in breeding purple martins. Condor 92 (4), 1040–1050.
Neufeld L. R., Muthukumarana S., Fischer J. D., Ray J. D., Siegrist J., Fraser K. C. (2021). Breeding latitude is associated with the timing of nesting and migration around the annual calendar among Purple Martin (Progne subis) populations. J. Ornithol. 162 (4), 1009–1024. doi: 10.1007/s10336-021-01894-w
Newton I. (2007). Weather-related mass-mortality events in migrants. Ibis 149 (3), 453–467. doi: 10.1111/j.1474-919X.2007.00704.x
Nilsson J.-Å., Brönmark C., Hansson L.-A., Chapman B. B. (2014). “Individuality in movement: the role of animal personality,” in Animal Movement Across Scales. Eds. Hansson L.-A., Akesson S. (Oxford, England: OUP Oxford). doi: 10.10910.1093/acprof:oso/9780199677184.003.0006
Nilsson C., Klaassen R. H. G., Alerstam T. (2013). Differences in speed and duration of bird migration between spring and autumn. Am. Nat. 181 (6), 837–845. doi: 10.5061/dryad.82d4q
Ouwehand J., Both C. (2017). African departure rather than migration speed determines variation in spring arrival in pied flycatchers. J. Anim. Ecol. 86 (1), 88–97. doi: 10.1111/1365-2656.12599
Pokrovsky I., Kölzsch A., Sherub S., Fiedler W., Glazov P., Kulikova O., et al. (2021). Longer days enable higher diurnal activity for migratory birds. J. Anim. Ecol. 90 (9), 2161–2171. doi: 10.1111/1365-2656.13484
Pyle P. (1997). Identification Guide to North American Birds: Columbidae to Ploceidae. (Bolinas, Calif: Slate Creek Press).
Radchuk V., Reed T., Teplitsky C., van de Pol M., Charmantier A., Hassall C., et al. (2019). Adaptive responses of animals to climate change are most likely insufficient. Nat. Commun. 10 (1), 3109. doi: 10.1038/s41467-019-10924-4
Rakhimberdiev E., Saveliev A., Piersma T., Karagicheva J. (2017). FLightR: an r package for reconstructing animal paths from solar geolocation loggers. Methods Ecol. Evol. 8, 1482–1487. doi: 10.1111/2041-210X.12765. British Ecological Society.
Rappole J. H., Tipton A. R. (1991). New harness design for attachment of radio transmitters to small passerines. J. Field ornithol. 62 (3), 335–337.
Rohwer S., Niles D. M. (1977). An historical analysis of spring arrival times in purple martins: A test of two hypotheses. Bird-Banding 48 (2), 162–167.
Rosenberg K. V., Dokter A. M., Blancher P. J., Sauer J. R., Smith A. C., Smith P. A., et al. (2019). Decline of the North American avifauna. Science 366 (6461), 120–124. doi: 10.1126/science.aaw1313
Sauer J. R., Pardieck K. L., Ziolkowski D. J., Smith A. C., Hudson M. A. R., Rodriguez V., et al. (2017). The first 50 years of the North American Breeding Bird Survey. Condor 119, 576–593. doi: 10.1650/CONDOR-17-83.1
Schmaljohann H. (2019). The start of migration correlates with arrival timing, and the total speed of migration increases with migration distance in migratory songbirds: A cross-continental analysis. Move. Ecol. 7 (1). doi: 10.1186/s40462-019-0169-1
Schmaljohann H., Both C. (2017). The limits of modifying migration speed to adjust to climate change. Nat. Climate Change 7 (8), 573–576. doi: 10.1038/NCLIMATE3336
Shipley J. R., Twining C. W., Taff C. C., Vitousek M. N., Flack A., Winkler D. W. (2020). Birds advancing lay dates with warming springs face greater risk of chick mortality. Proc. Natl. Acad. Sci. 117 (41), 25590–25594. doi: 10.1073/pnas.2009864117
Stanley C. Q., MacPherson M., Fraser K. C., McKinnon E. A., Stutchbury B. J. M. (2012). Repeat tracking of individual songbirds reveals consistent migration timing but flexibility in route. PloS One 7 (7), e40688. doi: 10.1371/journal.pone.0040688
Tomotani B. M., Gienapp P., de la Hera I., Terpstra M., Pulido F., Visser M. E. (2021). Integrating causal and evolutionary analysis of life-history evolution: arrival date in a long-distant migrant. Front. Ecol. Evol. 9. doi: 10.3389/fevo.2021.630823
Van Doren B. M., Horton K. G. (2018). A continental system for forecasting bird migration. Science 361 (6407), 1115–1118. doi: 10.1126/science.aat7526
Wickham H., Seidel D. (2022). scales: Scale Functions for Visualization. Available at: https://github.com/r-lib/scales.
Wikelski M., Tarlow E. M., Raim A., Diehl R. H., Larkin R. P., Visser G. H. (2003). Costs of migration in free-flying songbirds. Nature 423 (6941), 704. doi: 10.1038/423704a
Wotherspoon S., Sumner M., Lisovski S. (2016). BAStag: Basic data processing for light based geolocation archival tags. R Package version 0.1.3.
Keywords: phenotypic plasticity, phenology, migration rate, migration timing, passerine, climate change, neotropical migrant
Citation: Turcotte-van de Rydt C, Muthukumarana S and Fraser KC (2023) Spring departure date, not en route conditions, drive migration rate and arrival timing in a long-distance migratory songbird. Front. Bird Sci. 2:1232737. doi: 10.3389/fbirs.2023.1232737
Received: 01 June 2023; Accepted: 22 September 2023;
Published: 27 October 2023.
Edited by:
Noah G. Perlut, University of New England, United StatesReviewed by:
Nathaniel E. Seavy, National Audubon Society, United StatesCopyright © 2023 Turcotte-van de Rydt, Muthukumarana and Fraser. This is an open-access article distributed under the terms of the Creative Commons Attribution License (CC BY). The use, distribution or reproduction in other forums is permitted, provided the original author(s) and the copyright owner(s) are credited and that the original publication in this journal is cited, in accordance with accepted academic practice. No use, distribution or reproduction is permitted which does not comply with these terms.
*Correspondence: Christophe Turcotte-van de Rydt, Y3B0dmFuZGVyeWR0QGhvdG1haWwuY29t
Disclaimer: All claims expressed in this article are solely those of the authors and do not necessarily represent those of their affiliated organizations, or those of the publisher, the editors and the reviewers. Any product that may be evaluated in this article or claim that may be made by its manufacturer is not guaranteed or endorsed by the publisher.
Research integrity at Frontiers
Learn more about the work of our research integrity team to safeguard the quality of each article we publish.