- 1Department of Biology, Georgetown University, Washington, DC, United States
- 2School of Environment and Natural Resources, The Ohio State University, Columbus, OH, United States
- 3Migratory Bird Center, Smithsonian’s National Zoo and Conservation Biology Institute, Washington, DC, United States
- 4McCourt School of Public Policy, Georgetown University, Washington, DC, United States
Understanding the consequences of global change for migratory birds is complex as individuals are exposed to diverse conditions and experiences that interact across their annual cycle. Species distribution models (SDMs) can serve as a powerful tool that help us understand how species distributions respond to global change. However, SDMs applied to migratory birds may fail to capture the effects of seasonal variability on species distributional changes, likely due to a lack of appropriate modeling frameworks and limited data availability that hamper the inclusion of events and conditions throughout the annual cycle. Here, we review patterns in the migratory bird SDM literature over the last two decades using a vote counting approach, and provide a framework for migratory bird SDMs moving forward. We found evidence that species distribution models applied to migratory birds (1) typically incorporate data from only one season of the full annual cycle and do not account for seasonal interactions, (2) are focused on terrestrial species in North America and Europe, (3) tend to model the distributions of obligate migratory species, especially songbirds and waterfowl, and (4) largely lack biologically relevant threat layers. To improve our ability to forecast how species cope with global change, we recommend a Bayesian modeling framework where existing knowledge about a species’ migratory connectivity, threats, and/or other biologically relevant factors can be specified via model priors. Full annual cycle species distribution models are important tools for improving forecasts of migratory bird distributions in response to global change.
Introduction
Accelerated global change threatens life on our planet and drives the sixth mass extinction (Lewis and Maslin, 2015). Migratory birds are particularly at risk given their exposure to multiple facets of global change as they move, often at hemispheric scales, between stages of their annual cycles (Marra et al., 2015; Culp et al., 2017; Rosenberg et al., 2019). For example, a migratory bird may encounter disorienting artificial light (McLaren et al., 2018) or collide with city buildings while migrating (Van Doren et al., 2021). Upon arrival on their stationary nonbreeding grounds (hereafter nonbreeding grounds) they may find their habitat has been destroyed, or must cope with intensifying drought conditions (La Sorte et al., 2017). Arrival back to the breeding grounds may involve adjusting to reduced habitat availability or shifting their phenologies (Socolar et al., 2017). Mounting environmental change or unpredictability during any period of the annual cycle can impact migratory populations via direct mortality and seasonal interactions - nonlethal or lethal effects from one stage of the annual cycle that carry over to affect individuals and populations during subsequent stages of the annual cycle (Marra et al., 1998; Norris and Marra, 2007). The magnitude of these effects on populations can depend on the strength of a species’ migratory connectivity – the degree to which individuals from a population remain together throughout the year (Webster et al., 2002). More directly, physical locations encountered on breeding and nonbreeeding areas and along migratory routes determine a bird’s exposure to the spatially heterogeneous threats and shifting phenologies associated with global change (Small-Lorenz et al., 2013). These processes select for individuals occupying regions where they survive and successfully reproduce and can ultimately shape distributional shifts over time.
Species distribution models (hereby referred to as SDMs), a quantitative approach for modeling and forecasting species responses to spatiotemporal variation in both abiotic and biotic factors, have been the primary tool used by ecologists over the past two decades to predict distributional changes for model organisms (Norberg et al., 2019). Due to increasing research interest in predicting species responses to global change, SDMs have been customized and implemented in an array of avian studies ranging from estimating current ranges of rare, range-restricted hummingbirds to predicting future changes to seabird distributions (Engler et al., 2017). Many typical SDMs make two critical assumptions: (1) that the species being modeled is at equilibrium with its environment, and (2) that individuals within a species respond identically and predictably to environmental change across their range (Elith et al., 2010). Because of these assumptions and other drawbacks of SDMs (e.g., overfitting with environmental variables that may not be relevant to a species’ distribution), critics have questioned their reliability for making accurate and robust predictions about future species distributions (Fourcade et al., 2018).
Despite these drawbacks, SDMs can provide important signals for distributional shifts when researchers integrate environmental variables relevant to their species’ biology, consider multiple avenues of validating and selecting candidate models, and carefully consider model assumptions when interpreting model outputs (Betts et al., 2019; Norberg et al., 2019; Zurell et al., 2020). However, for migratory species, a majority of SDM frameworks lack key information or oversimplify species’ biology, such as their migratory behavior, that is critical for accurately predicting the patterns and drivers of distributional change (Engler et al., 2017). For example, knowing whether a species may be resident and migratory within and among populations across parts of its range (e.g., partially migratory) is important not only for modeling where a species may be located regionally, but also whether that distribution might change seasonally, and/or year to year. We argue that including information into an SDM regarding when (timing of movements), where (locations and routes used), and the propensity (whether to migrate or not) for individuals to move throughout its annual cycle – all components constituting a species’ migratory connectivity – is critical for accurately linking environmental or anthropogenic drivers with where a species is distributed across space and time.
Full annual cycle integrated population models are gaining traction in migratory bird ecology (Hostetler et al., 2015), but the equivalent full annual cycle approach has not been applied to the majority of avian SDMs. To date, most SDMs used for avian species have overlooked the seasonal component intrinsic to the distributions of migratory birds, instead focusing on only one stage of the annual cycle (Engler et al., 2017). However, given the importance of incorporating seasonal interactions and events throughout the annual cycle more generally for migratory birds (Marra et al., 2015), these single-season SDMs likely fail to accurately forecast future distributions. Therefore, developing and promoting SDMs that incorporate drivers of global change across the annual cycle likely represents an important advance for our ability to forecast and manage how animals will respond to global change.
Here, we conducted a systematic review of peer-reviewed studies that implemented SDMs for migratory birds and asked the following questions: (1) How often do migratory bird SDMs account for full annual cycles?; (2) Are different major biogeographic regions represented equally?; (3) Are they applied appropriately to account for species that may differ in the degree of migratory behavior (e.g., a partial-migrant vs. a complete migrant)?; and (4) Do they incorporate major threats known to impact birds? By answering these questions, we aim to pinpoint current pitfalls of migratory bird SDMs, identify why gaps may exist, and provide a more holistic conceptual framework for modeling past, current, and future migratory bird distributions in the face of rapid environmental change.
Materials and methods
We conducted a search of English language peer-reviewed publications for studies using SDMs for migratory birds in Web of Science on 14 November 2022. Our definition of a migratory bird SDM included any instance of modeling spatially-explicit occurrence data of one or multiple migratory bird species as a function of some combination of environmental variables. We chose to use this more flexible definition of an SDM to capture the inherent variation in SDM frameworks that exists in the literature. We first developed a comprehensive list of search terms expanded from those used in Engler et al. (2017). We used a topic search (i.e., title, keywords, abstract) of derivatives of the word migrate (e.g., migra*) combined with synonyms of species distribution models (e.g., species distribution model, bioclimatic envelope, bioclimatic envelope model, ecological niche model, macroecological model, SDM, ENM, MEM, etc.) and derivatives of bird words (e.g., *bird*, avian*, duck*, raptor*, waterfowl*) in an attempt to capture all SDM-type papers that focus on any kind of migratory bird. We also added an abstract-only search of (migra* AND (bird* OR avian* OR duck* OR raptor* OR waterfowl*)) to eliminate papers with relevant keywords that did not focus on modeling the distributions of migratory birds (e.g., an SDM of a frog species). Our topic search was intentionally broad to ensure we did not exclude any relevant studies – our goal was to cast a wide net and systematically remove extraneous studies rather than beginning with narrow search terms and potentially missing important papers. We did not include a year filter initially; however, upon conducting the first search, we noticed that the number of relevant papers was near-zero up until 2000 when they began a notable yearly increase. Therefore, we decided to begin our search in 2000 and thus added a year filter of 2000 to 2022. This search resulted in over a thousand papers, so we focused only on even years beginning in January 2000 and ending in November 2022. Altogether, we used the following query in Web of Science to conduct our final search:
“TS=(migra* AND (species distribution model OR bioclimatic envelope OR bioclimatic envelope model OR ecological niche model OR macroecological model OR SDM OR ENM OR MEM) AND (*bird* OR avian* OR duck* OR raptor* OR waterfowl*)) AND AB=(migra* AND (bird* OR avian* OR duck* OR raptor* OR waterfowl*)) AND PY=(2000-2022)”
Next, we used a vote counting approach (i.e., tallying the number of papers that meet each criterion in our review protocol) to review and summarize relevant information from each paper. For each paper, we screened the abstract and methods to determine whether they were relevant to our study. Relevant studies (1) modeled the distribution of one or more migratory bird species during at least one stage of their annual cycle, and (2) adhered to our definition of a migratory bird SDM (i.e., modeled spatially-explicit occurrence data as a function of environmental variables). Our broad definition of a migratory bird SDM allowed for the inclusion of a variety of studies beyond those that used ‘traditional’ SDM frameworks (i.e., forecasting a species’ range in response to current or future conditions), including movement models based on tracking data or dynamic occupancy models, for example. Many papers that seemed relevant based on their keywords and abstract did not adhere to our definition of a migratory bird SDM; for example, studies modeling migratory bird occurrence data without a spatial reference, or studies using SDMs that solely focused on non-migratory bird species. A more detailed explanation of definitions and how we deemed relevancy is included in Supplemental Files. If a paper was deemed relevant, we extracted the data we sought to collect from each paper (e.g., number of periods of annual cycle considered, threat layers included, migratory system of modeled species, etc.) focusing on the objectives, methods, tables, and figures. We entered all data into a standardized spreadsheet. We took the objectives and methods of each study at face value. Our goal was not to assess the appropriateness of authors’ decisions but instead to determine how often studies took into consideration the questions from our four objectives. Finally, we performed all data collation, preparation, and summarizing in program R (R Core Team 2022).
Our aim with this review was to demonstrate a general pattern in the SDM literature using qualitative information collected by vote counting. Importantly, our methodology does not meet the requirements of a systematic review, and therefore carries several associated caveats (Haddaway et al., 2020). Firstly, our review inherently represents only a subset of the SDM literature. We conducted our search in Web of Science, whereas a systematic review would have included searches in other databases, Google Scholar, and web searches. These additional searches are particularly important for dealing with publication bias and reporting bias, and for sampling gray literature, or manuscripts that are not published in academic journals (e.g., government reports, PhD dissertations; Foo et al., 2021). Secondly, our vote counting approach is inferior to meta-analytical methods for synthesizing qualitative evidence. Vote counting ignores study precision and validity (Haddaway et al., 2020). A systematic review of the SDM literature would deal with these issues via extracting effect sizes, variances, and model performance metrics from each paper. However, these issues likely do not present major errors in our study given our focus on author decisions from other manuscripts rather than their results. Nonetheless, given the simplicity of our review design, we caution readers from extracting detailed conclusions from our review. Our results should be used to assess where general differences exist (e.g., are obligate migrants modeled more than birds with other migration strategies)?, but not to estimate the magnitude of those differences (e.g., how many more studies include habitat threat layers versus climate change threat layers)?.
Results
Our query yielded 603 total papers. Of these 603 papers, only 163 were relevant. Most papers that we excluded were studies that modeled population dynamics, abundance, or phenological benchmarks (e.g., onset of singing or peak migration) of migratory species but lacked spatially-referenced occurrence data. The 163 remaining papers performed a total of 201 SDMs.
The majority of migratory bird SDMs in our review considered only one period of the annual cycle (57%), while only 14% considered all four (Figure 1A). Additionally, most SDMs incorporated data from the stationary periods of the annual cycle (i.e., breeding and nonbreeding), but few incorporated data from the non-stationary periods (i.e., spring and autumn). Specifically, data from breeding and stationary nonbreeding periods were incorporated into 64% and 61% of the SDMs, respectively, whereas spring and autumn periods were included in only 28% and 25% of SDMs, respectively (Figure 1B).
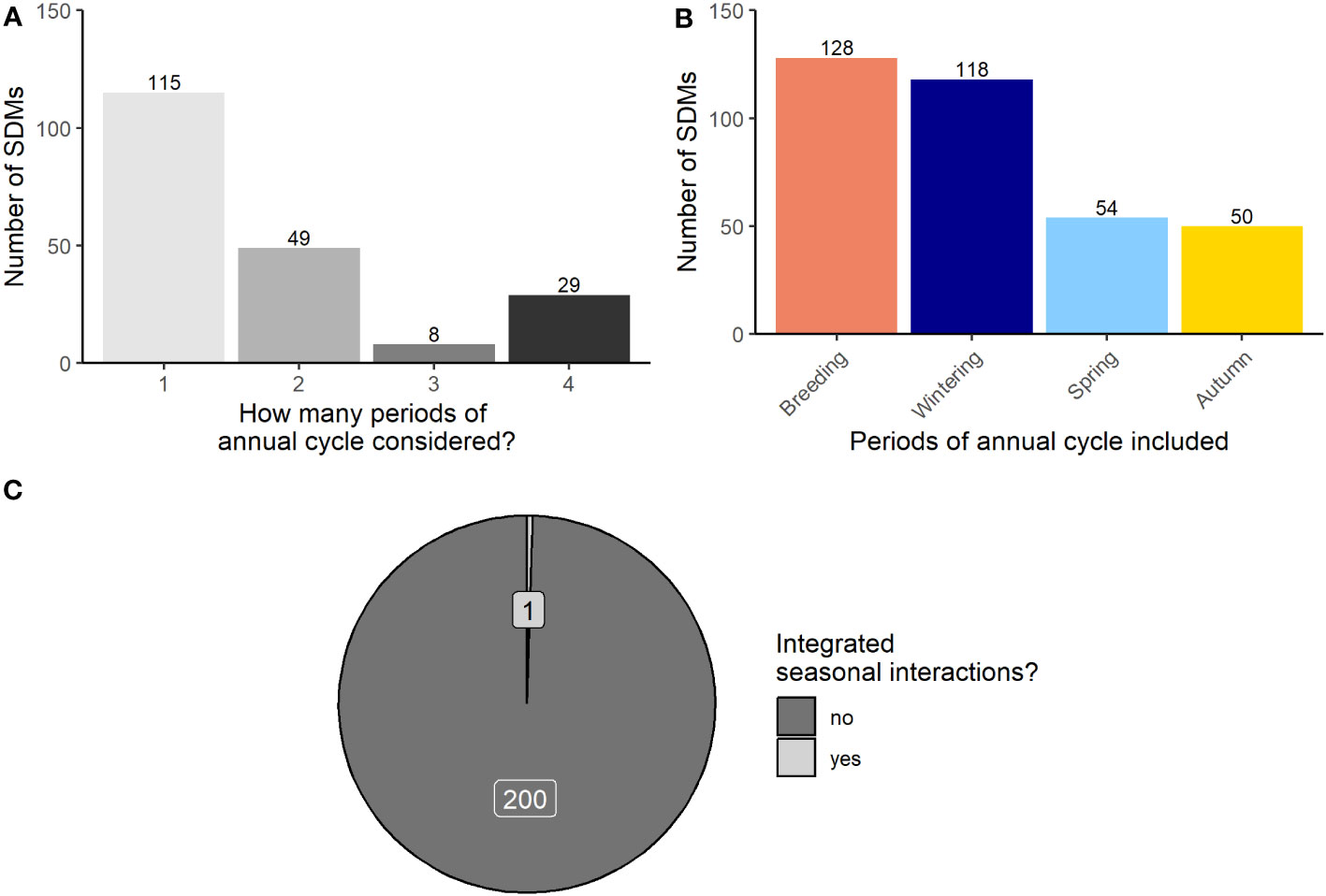
Figure 1 (A) shows the proportion of migratory bird SDMs that did (light gray) and did not (dark gray) account for seasonal interactions. (B) shows the periods of the annual cycle considered by each migratory bird SDM. (C) shows the representation of each major period of the annual cycle in the migratory bird SDMs from our literature review. Unlike in (A, B), one SDM can be represented more than once in (C) if it incorporates data from multiple seasons. Numbers at the top of each bar represent the number of observations for that category.
Of these 201 SDMs, only 1 (<1%) included a seasonal interaction by which species occurrence in one season was linked to conditions in a preceding season (Aagaard et al., 2022; Figure 1C). This study included environmental conditions in each of the primary periods of the nonbreeding season (e.g., autumn migration, stationary nonbreeding, spring migration) to evaluate the relative contributions of each phase on each subsequent phase of occurrence. Aagaard et al. (2022) found that environmental conditions experienced in the preceding season were important factors in influencing abundance and occurrence. Notably, they demonstrated that nonbreeding environmental conditions played an important role in influencing the distribution of mallards (Anas platyrhynchos) during spring migration (Aagaard et al., 2022).
Geographically, migratory bird SDMs occurred primarily for species in North America and Europe (Figure 2). Specifically, 118 of the 201 SDMs (59%) modeled species distributions in North America or Europe despite these two continents covering less than a quarter of global land mass. About 6% of SDMs modeled species distributions that spanned multiple continents. SDMs modeling migratory bird distributions in oceans accounted for less than 3% of those reviewed in our analysis. No reviewed SDMs modeled migratory bird distributions in Antarctica.
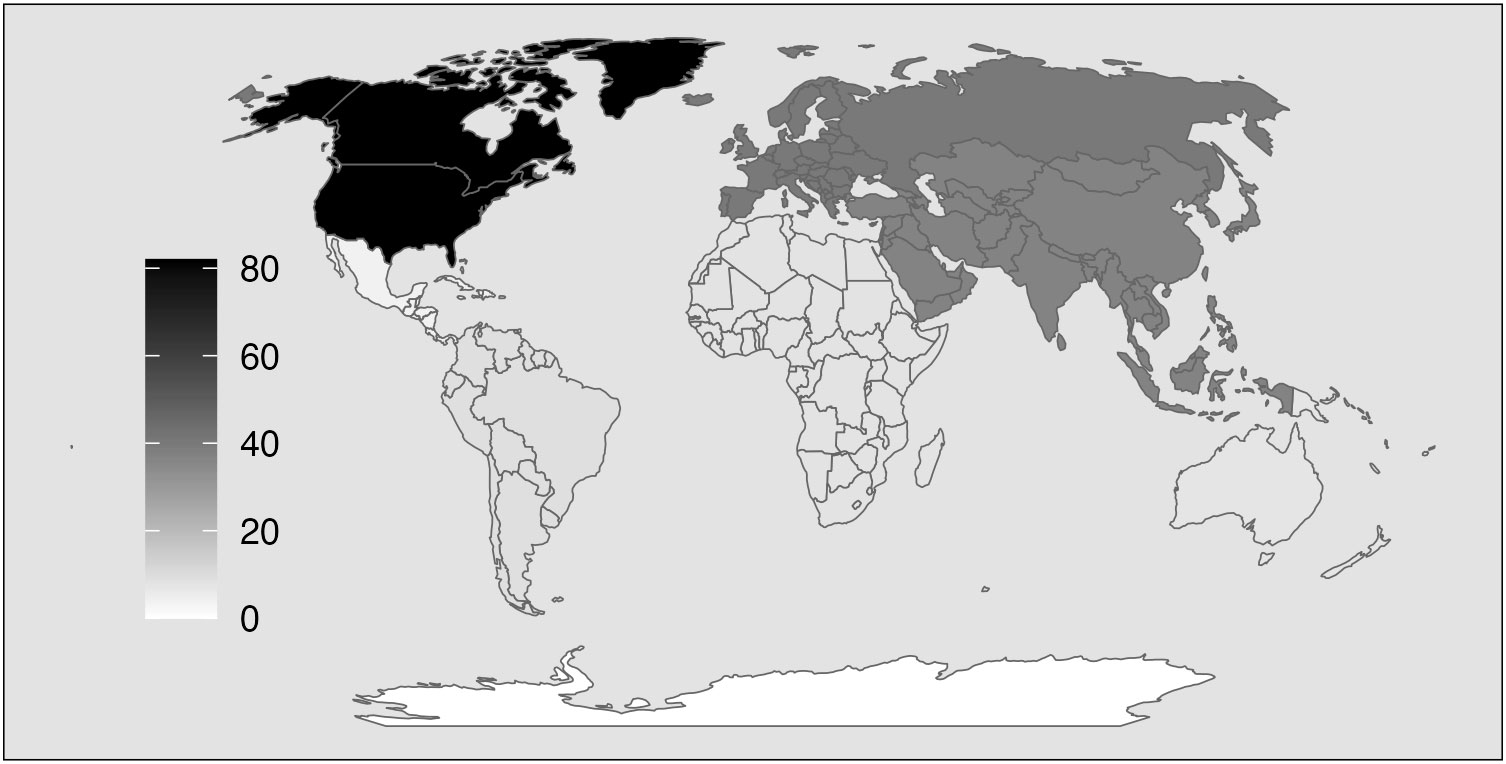
Figure 2 Migratory bird SDMs are concentrated in North America and Europe and fewer in marine areas and tropics. Major continents (7) and oceans (4) are colored based on the number of migratory bird SDMs that model species from that region, where darker shading indicates a higher number of migratory bird SDMs.
The majority of migratory bird SDMs modeled distributions for suites of species (multiple families - mostly combinations of songbirds and waterfowl (34%), songbirds (22%), and waterfowl (16%); Figure 3A). Over half of all SDM studies focused on obligate migratory birds (53%) or did not specify the migratory behavior of modeled species (38%). Scant studies recognized multiple migratory behaviors within their SDM models (4%; Figure 3B).
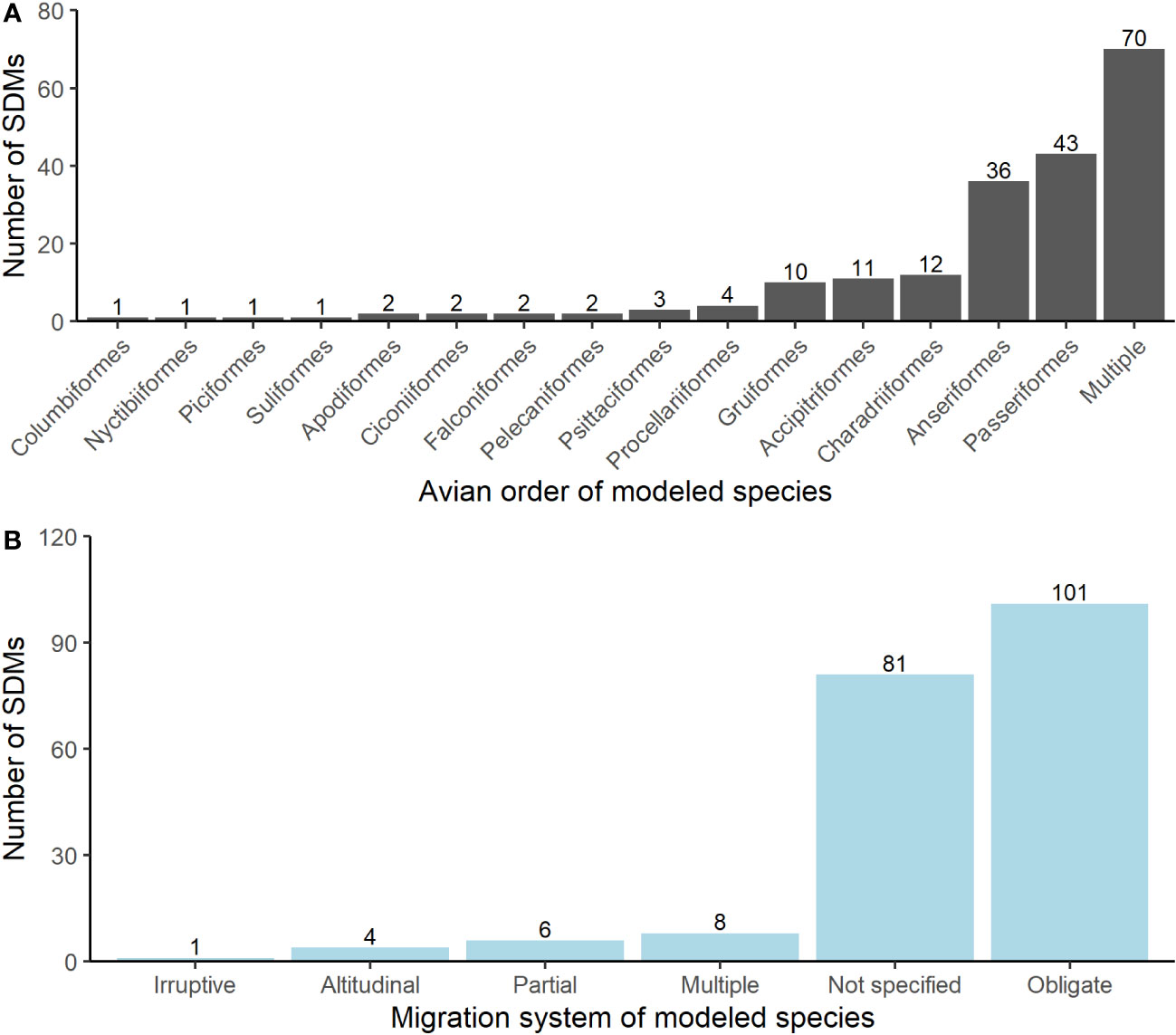
Figure 3 (A) demonstrates the different avian orders among migratory bird SDMs from our literature review. (B) shows the breakdown of the major migration behaviors of species modeled in the SDMs from our review. The ‘Multiple’ category accounts for multi-species SDMs where modeled species exhibit different primary migration systems. The ‘Not specified’ category includes instances where researchers did not include any information about the migratory system of the species they modeled. Numbers at the top of each bar represent the number of observations for that category.
Across all studies, just 64 out of 201 (32%) included threat factors within their SDMs (Figure 4A). Of the studies that included threat factors, the majority (71%) included habitat change as a predictor variable, and less than one third (31%) included climate change (Figure 4B). Other threat factors used in SDMs as predictor variables included vehicle collisions (<1%), energy infrastructure collisions (<1%), window collisions (<1%), and other threat factors (3%) (Figure 4B). No SDMs included threat factors related to predation by cats, pesticides, or pollution and other contaminants. Over one third of the SDMs (40%) included multiple threat factors in their SDMs (Figure 4B). The majority of SDMs (77%) used a direct measure of an anthropogenic threat, while just over one third relied on proxy measures of the threat factor (39%).
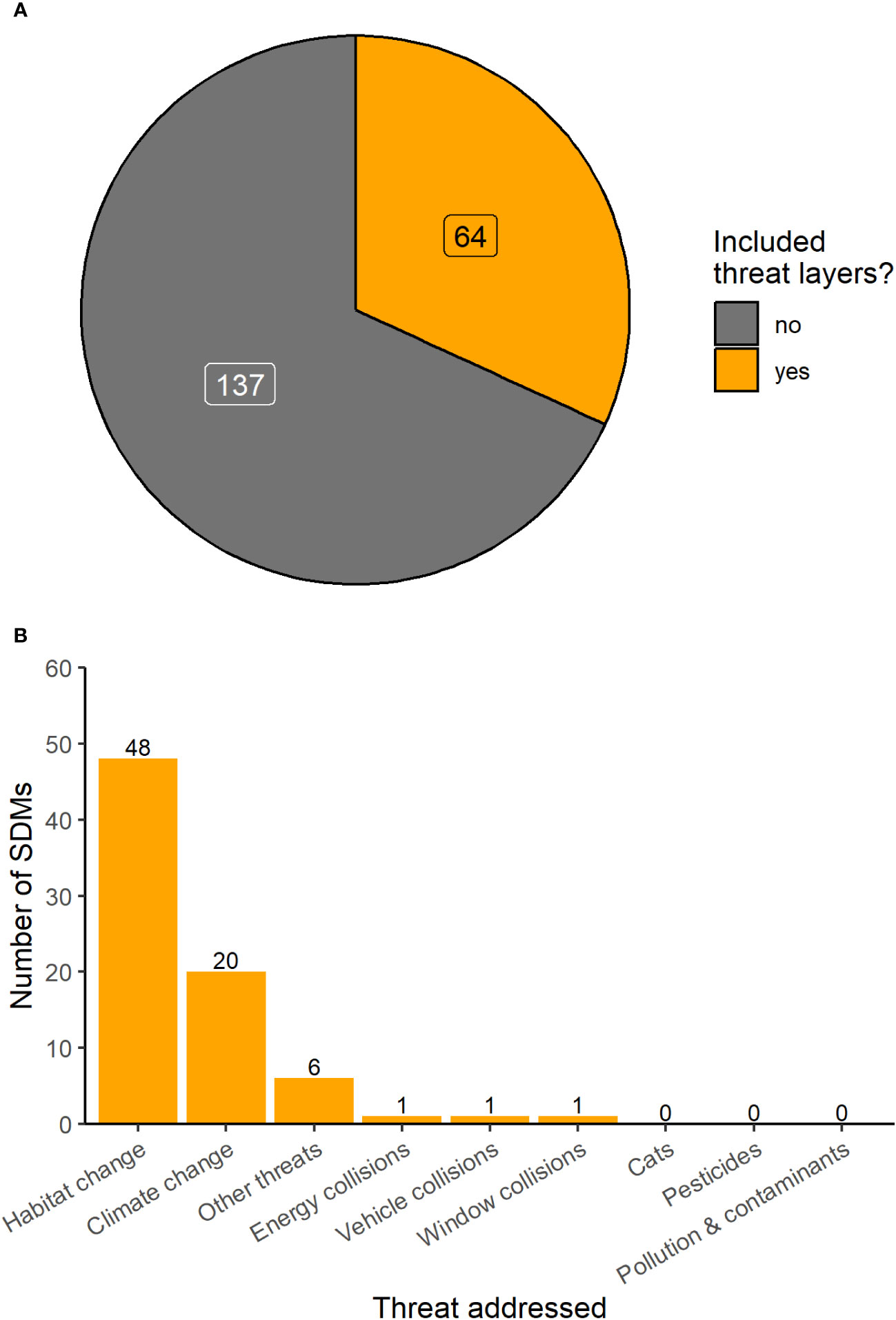
Figure 4 (A) shows the proportion of migratory bird SDMs that either did or did not include relevant threat layers. (B) shows the threats represented in the subset of migratory bird SDMs that did include relevant threat factors. Numbers at the top of each bar represent the number of observations for that category. Note that some studies included multiple threats.
Discussion
We demonstrate support for multiple potential biases in how SDMs were constructed, the types of data they included, which species were modeled, and geographic areas where they were implemented. Such biases can introduce error and uncertainty into projections of how the distributions of migratory birds are shifting in the face of global change. Moving forward, we recommend that researchers carefully consider the biology of their study species when designing SDMs. Our recommendations include identifying the full annual cycle range of the population of interest to better estimate climate and disturbance exposure rates, the role of seasonal interactions in influencing distributional shifts, and incorporating relevant anthropogenic factors in addition to environmental layers. Below, we discuss each of the potential biases supported by our literature review and the next steps forward in improving the utility of SDMs to understand past, current, and future changes to migratory bird distributions.
Seasonal bias
Migratory species, by definition, occupy different ranges across the annual cycle. Focusing on only one stage of the annual cycle unintentionally limits our ability to obtain accurate predictions regarding the change of future species’ distributions. We found that SDMs for migratory birds do exactly that – the majority of SDMs from our review considered data from only one period of the annual cycle. Migratory species often have different habitat requirements and nutritional needs and are exposed to different environmental factors and threats during different seasons of their annual cycles (Small-Lorenz et al., 2013; Fink et al., 2020; Lamb et al., 2020). Therefore, an SDM focusing exclusively on modeling a species’ distribution during the breeding period, for example, without data from other periods of the annual cycle provides likely inaccurate information about the species’ range-wide distributional shifts in response to global change.
Surprisingly, we did not find a seasonal bias towards SDMs focused on the breeding period compared to the stationary non-breeding period. However, we did find support for a bias towards stationary periods of the annual cycle (breeding and nonbreeding) compared to non-stationary periods (spring and autumn migration). Mortality is often highest during non-stationary periods (Sillett and Holmes, 2002; Klaassen et al., 2014; Rushing et al., 2017; but see Conklin et al., 2017; Senner et al., 2019) and certain threats (e.g., collisions with buildings and power lines, cats) can impact migratory birds heavily during migration, whereas these risks pose less of a danger during stationary periods (Loss et al., 2015). Future research with tracking technologies should focus on uncovering patterns of range-wide migratory connectivity for migratory birds so that we can better identify population linkages and exposures to climate and environmental threats for connected populations.
A host of competing abiotic and biotic effects throughout the annual cycle differentially affect population dynamics and operate over large spatiotemporal scales to determine patterns of distribution and abundance (Gaston, 2009; Sexton et al., 2009). Seasonal interactions (lethal or nonlethal events in one season that carry-over and influence individuals or populations in a subsequent season) are inherent properties of these systems and are vital for understanding how events and selective pressures operate throughout the annual cycle to ultimately drive population dynamics and species distributions (Norris and Marra, 2007). Yet, most studies focus solely on one stage of the annual cycle, which remains the dominant framework for investigating species distributions. In large part, this is likely driven by a heavy breeding-centric seasonal bias in where research is conducted, and also when occurrence/monitoring data is collected (Marra et al., 2015). Although these biases are improving, we still found that of the 201 SDMs examined, nearly 55% only included factors from one stage of the annual cycle and of those, most were conducted during the breeding season only. Yet, the one study that did incorporate seasonal interactions (Aagaard et al., 2022) found that seasonal interactions were critical - environmental conditions operating outside of the season of study played an important role in influencing the abundance and distribution of their study species.
By ignoring seasonal interactions, studies that examine and attempt to predict future species distributions are missing critical drivers, hindering accurate prediction (Illán et al., 2014; Lee-Yaw et al., 2022). More to the point, they potentially provide flawed guidance for climate adaptation and conservation planning (Small-Lorenz et al., 2013). Environmental conditions experienced across the annual cycle vary drastically from season to season and are often undergoing different rates of climatic change. For example, in the Neartic-Neotropical migratory flyway, conditions on the temperate breeding grounds have warmed significantly leading to advances in the timing of breeding for many species (Brown et al., 1999; Dunn and Winkler, 1999; Townsend et al., 2013; Shipley et al., 2020) and have been suspected of driving species ranges north (Langham et al., 2015). Yet on the tropical nonbreeding grounds, continued and progressive drought (Neelin et al., 2006; Herrera and Ault, 2017) is delaying the start of migration (Studds and Marra, 2011; Rockwell et al., 2012) and strongly influencing survival patterns (Rockwell et al., 2017; Dossman et al., 2023a). These differences in climate change and thus climate exposure across different periods of the annual cycle almost certainly differentially impact changes in abundance and distribution of a species on the breeding grounds (Carey, 2009). The overall direction and magnitude in these distributional changes are likely to represent the cumulative effect of full annual cycle events on the demography of a species across its range (Dossman et al., 2023b). Accurately predicting complex future patterns of range shifts and distributional changes will require integration of full annual cycle events at the level of single species. Complex life history events drive the natural history of these species and ignoring these features dangerously oversimplifies future predictions. To date, no species distribution models have formally integrated seasonal interactions into their design and as such remain a key limitation towards advancing our understanding of how species distributions will respond in the face of climate change.
Geographic bias
We found evidence for a geographic bias in current SDMs towards migratory birds in North America and Europe. Our findings are consistent with results from other studies spanning various disciplines within ecology and conservation research (Feldman et al., 2021). This geographic bias likely stems from a global imbalance in access to research and funding. Due to a multitude of social, political, and economical factors (Culumber et al., 2019), conservation and climate change research activity and funding occurs primarily in the United States, Canada, and European nations (Pasgaard et al., 2015; Di Marco et al., 2017). This is not to imply that research is not occurring in other regions of the world (Soares et al., 2023). In fact, there have been recent calls to acknowledge work from underrepresented regions where the ability to conduct and disseminate research is frequently inhibited due to language hegemony, journal access, and disproportionately lower access to research funding (Soares et al., 2023). Indeed, we conducted our literature review in English, so we may have missed studies on species distribution modeling for migratory birds published in other languages.
As a result of this geographic bias, much of what we understand about the distributional responses of migratory birds to global change comes from North American and European models (Stephens et al., 2016). These models typically conclude that the breeding ranges of migratory birds are shifting poleward (i.e., north) or to higher elevations via tracking of their preferred niche (Hitch and Leberg, 2007; Chen et al., 2011; Brommer et al., 2012). Although this likely holds true for some migratory species, recent evidence suggests the opposite pattern (i.e., southward range shifts) could be occurring for others due to climate change on the nonbreeding grounds (Dossman et al., 2023b), or that the southern range limit could be contracting with no concurrent shifts in the northern range limit (Rushing et al., 2020). Instead of moving, migratory birds may also track their preferred niche by altering the phenological onset of key life history events (e.g., breeding; Socolar et al., 2017).
A corollary of the geographic bias towards North America and Europe is that current SDMs also show a bias towards modeling terrestrial migratory birds versus marine species. Historically, building SDMs for seabirds in general – and particularly migratory seabirds – proved challenging due to sparse distributional data (toward breeding season and nesting sites), limited environmental information, and a comparatively poorer understanding of movement patterns compared to terrestrial species (Engler et al., 2017). Fortunately, these limitations have become increasingly less problematic thanks to the collection and dissemination of publicly-available citizen science and tracking data (e.g., Fauchald et al., 2019). Moreover, commonly used environmental data layers like sea surface temperature can now be combined with more advanced environmental data derived from remote sensing and satellite imagery (Engler et al., 2017). Combining these datasets should facilitate the development of better informed SDMs for migratory seabirds.
Taxonomic and migration system biases
Most SDMs modeled songbirds, waterfowl, and shorebirds, which reflect the groups of birds most abundant globally (Callaghan et al., 2021) and the avian orders with the highest species richness - 60% of all avian species are in the Order Passeriformes (Avonet database; Tobias et al., 2022). However, both waterfowl and shorebirds make up 2% and 3%, respectively, of all avian species globally (Avonet database; Tobias et al., 2022). As a result, while we have a relatively better understanding of the drivers influencing species distributions for songbirds, waterfowl, and shorebirds, this taxonomic bias underscores the gap left for more speciose orders.
In addition to showing a taxonomic bias, the majority of SDM studies were conducted for obligate migratory species. The bias towards obligate migratory species combined with the geographic bias towards North American and European breeding species is not surprising; long-distance migration is common in strongly seasonal environments characteristic of temperate zones (Winger et al., 2014; Winger et al., 2019). This bias may also be partially explained by the dearth of studies on species that may engage in less dramatic (e.g., within rather than across continents) or predictable migrations (Jahn et al., 2012). While the majority of studies on migration focus on obligate, long-distance migrations and geographic regions (reviewed by Jahn et al., 2004), species that are partially migratory, irruptive, or migrate over shorter distances represent most species globally (Levey, 1994; Chapman et al., 2011; Hsiung et al., 2018). Thus, current SDMs are overlooking the vast majority of migratory bird species.
Collectively, most SDMs in this review either prioritized obligate migratory birds or, by the lack of explicitly defining what type of species they modeled (whether migrant, partial, resident, etc.), do not acknowledge the importance of specifying migratory behavior in modeling where species may be across space and time. This is exacerbated by the fact that many of these studies oversimplify the variation in migratory behavior present in species across their range or annual cycle (Berthold, 2001). Different populations of the same species may differ in when, how far, and if they even migrate at all in a given year (e.g.,Taylor and Norris, 2007; Jahn et al., 2019). This variability in potential migratory behaviors has major ramifications for accurately predicting where and when a species is exposed to a particular climate or threat (Nilsson et al., 2006). Furthermore, over a third of all studies we reviewed modeled multiple species simultaneously, many of which exhibit interspecific variation in migratory behavior, which was not accounted for in models. Interspecific variation in migration influences species’ exposure and resilience to global change by nature of differences in species-level ecological or life-history traits (Jiguet et al., 2007; Stevens et al., 2023), migratory connectivity (Patchett et al., 2018), and whether a species may be facultative or obligate across its range (Gilroy et al., 2016). Understanding how individuals and populations of species may be spatially and temporally connected throughout the year is critical to determining a species’ risk to threats, which ultimately shape population dynamics (Bauer et al., 2016). Thus, including variation in migratory behavior and connectivity within and among individuals and populations of the same and different species will greatly improve SDMs, and therefore also our ability to predict, target threats, and effectively manage and conserve species in the face of climate change and other drivers of global change.
Threat bias
Human-driven changes such as habitat loss, climate change and other direct sources of mortality are major drivers of decline in migratory bird populations (Robbins et al., 1989; Loss et al., 2015; Rigal et al., 2023) and are increasingly expected to play an important role in influencing the distribution of bird species (Jetz et al., 2007). Despite the potential impact of anthropogenic activities on migratory bird distributions, there were no examples of SDMs that incorporated threats from connected, spatially-explicit populations throughout the annual cycle, and only one third of the SDMs incorporated anthropogenic factors at all. Furthermore, of those SDMs that incorporated anthropogenic factors, the vast majority only incorporated habitat loss and/or climate change as potential predictor variables. By contrast, direct sources of mortality were only represented in 5% of SDMs that included anthropogenic factors. Interestingly, a number of studies assessing the exposure of bird populations to direct sources of anthropogenic threats did not incorporate anthropogenic factors directly into their SDMs, but instead, overlaid their predicted species distributions (i.e., SDM output) and threat layers in separate spatial analyses (Loring et al., 2014; Pereira et al., 2018). In spite of growing recognition of the impact of human influence on the distribution of species, most models do not appear to directly incorporate anthropogenic factors, which could undermine forecasts of future distributions (Yates et al., 2018).
Challenges exist for incorporating anthropogenic factors into SDMs. For many species, the relationship between anthropogenic activities and species distributions are not well understood and likely varies heterogeneously across spatiotemporal scales (Guisan and Thuiller, 2005; Zuckerberg et al., 2016). For example, researchers have found that artificial light at night can drive migratory bird distributions but the effect varies seasonally and across spatial scales (La Sorte et al., 2017; McLaren et al., 2018). Therefore, care must be taken to ensure that the resolution of data sources match the scale of human impact on species distribution. Additionally, standardized datasets on anthropogenic factors, particularly direct sources of mortality, are not widely available, limiting their potential incorporation as predictors into SDM projects (Loss et al., 2015). Datasets for habitat attributes and climate variables exist across multiple spatiotemporal scales and resolutions, which could explain why we found the majority of SDMs incorporating anthropogenic factors addressed these two categories of threats (Wüest et al., 2020; Zurell et al., 2023). Despite the paucity of standardized datasets for anthropogenic factors, over one third of studies (39%) were able to include anthropogenic factors by incorporating proxies of these threats. For example, Araujo et al. (2022) used distance to port as a proxy for disturbance from fishing boats and Adams and Root (2022) used building density as a proxy for disturbance from window collisions. Given the rapidly increasing pressure from a growing human population, the omission of anthropogenic processes from SDMs represents a major limitation to our ability to understand and forecast the distribution of species across multiple scales.
Working towards full annual cycle SDMs
Full annual cycle SDMs are not a novel concept. Researchers have long acknowledged the importance of accounting for the annual cycle in work involving migratory species (Temple, 1988; Faaborg et al., 2010). Recently, the Cornell Lab of Ornithology unveiled a lineup of full annual cycle SDMs for hundreds of bird species in North America with sufficient observation data from eBird (Fink et al., 2020; Fink et al., 2022). These eBird full annual cycle SDMs address many of the limitations we describe above. Namely, they include relevant threat layers affecting all bird species (e.g., nighttime lights, Cao et al., 2014; land cover and land use classifications, Friedl and Sulla-Menashe, 2019) in addition to other standard predictor variables, can be applied to any species with sufficient observation data, and model the relative abundance of the species throughout the annual cycle using ensemble modeling (i.e., multiple, overlapping models based on specific time frames). While this novel approach represents a monumental achievement for migratory bird SDMs, it still lacks two critical aspects affecting the distribution of migratory birds around the world – migratory connectivity and seasonal interactions.
Only one study in our review integrated the effects of seasonal interactions into SDMs (Aagaard et al., 2022), making an important advance to developing full annual cycle SDMs for migratory birds. Notably, this study did not use any novel modeling designs to integrate seasonal interactions – they simply modified an existing mechanistic SDM framework (i.e., energetics-based individual based model; Aagaard et al., 2022). Moreover, Aagaard et al. (2022) reported that seasonal interactions played an important role in influencing distributional change for their study species, North American Mallards (Anas platyrhynchos). The fact that Aagaard et al. (2022) modeled mallards, a well-studied migratory bird species, using an existing SDM framework suggests that the key limitation inhibiting the development of full annual cycle SDMs is not necessarily a lack of modeling tools but instead may be missing abundance data across the annual cycle, information on patterns of migratory connectivity, and what controls distributional change for migratory birds (Howard et al., 2014). Including a seasonal interaction in an SDM is as simple as, for example, linking a winter covariate to each breeding observation in a SDM modeling the breeding distribution of a migratory species. Once we have these relevant data on the full annual cycle biology for a given migratory species, developing full annual cycle SDMs could be as straightforward as modifying existing SDM frameworks. We describe one of these potential modifications below.
Bayesian hierarchical modeling frameworks seem particularly promising for building the next generation of full annual cycle SDMs. Bayesian hierarchical models allow for the specification and inclusion of multiple simultaneous processes and stochasticity intrinsic to ecological systems – e.g., the probability that an American Redstart (Setophaga ruticilla) occurs in a given environment within their breeding range depends on breeding season environmental variables like forest and insect availability, but also on climate on their nonbreeding grounds (Dossman et al., 2023b). Studies focused on integrated population models use Bayesian hierarchical modeling frameworks routinely (Hostetler et al., 2015), but instead of modeling distributional change, they model population dynamics. In fact, we excluded several papers from our review that did incorporate seasonal interactions using a Bayesian hierarchical framework but did so with population models rather than SDMs (Almaraz et al., 2012; Rushing et al., 2016). The main difference between a population model and a full annual cycle SDM is that the former focuses on modeling population change, while an SDM models the probability of occurrence during a specified time period and relies exclusively on spatially-referenced occurrence and environmental data. Given this subtle difference, it should be straightforward to tweak these population modeling frameworks and apply them as full annual cycle SDMs in cases where spatially-referenced occurrence and environmental data as well as data on migratory connectivity are available.
Bayesian hierarchical models crucially allow for the specification of informative priors. The capability to specify priors in a modeling framework serves as an ideal system to incorporate previous knowledge not only about what influences range dynamics for a species (e.g., nonbreeding rainfall; Dossman et al., 2023b), but also information about their migratory connectivity. Including information about migratory connectivity helps reconcile one of the key assumptions of all SDMs – that all individuals respond equally and predictably to environmental change throughout their range – by allowing for different populations to respond to different drivers of global change as they would naturally according to their patterns of migratory connectivity. Using priors, it could be possible to model a species distribution using joint SDMs where you (1) divide a species into regional populations which the model considers separate ‘species’ and (2) limit different populations to respond only to the environment in regions where they occur throughout the annual cycle (Figure 5).
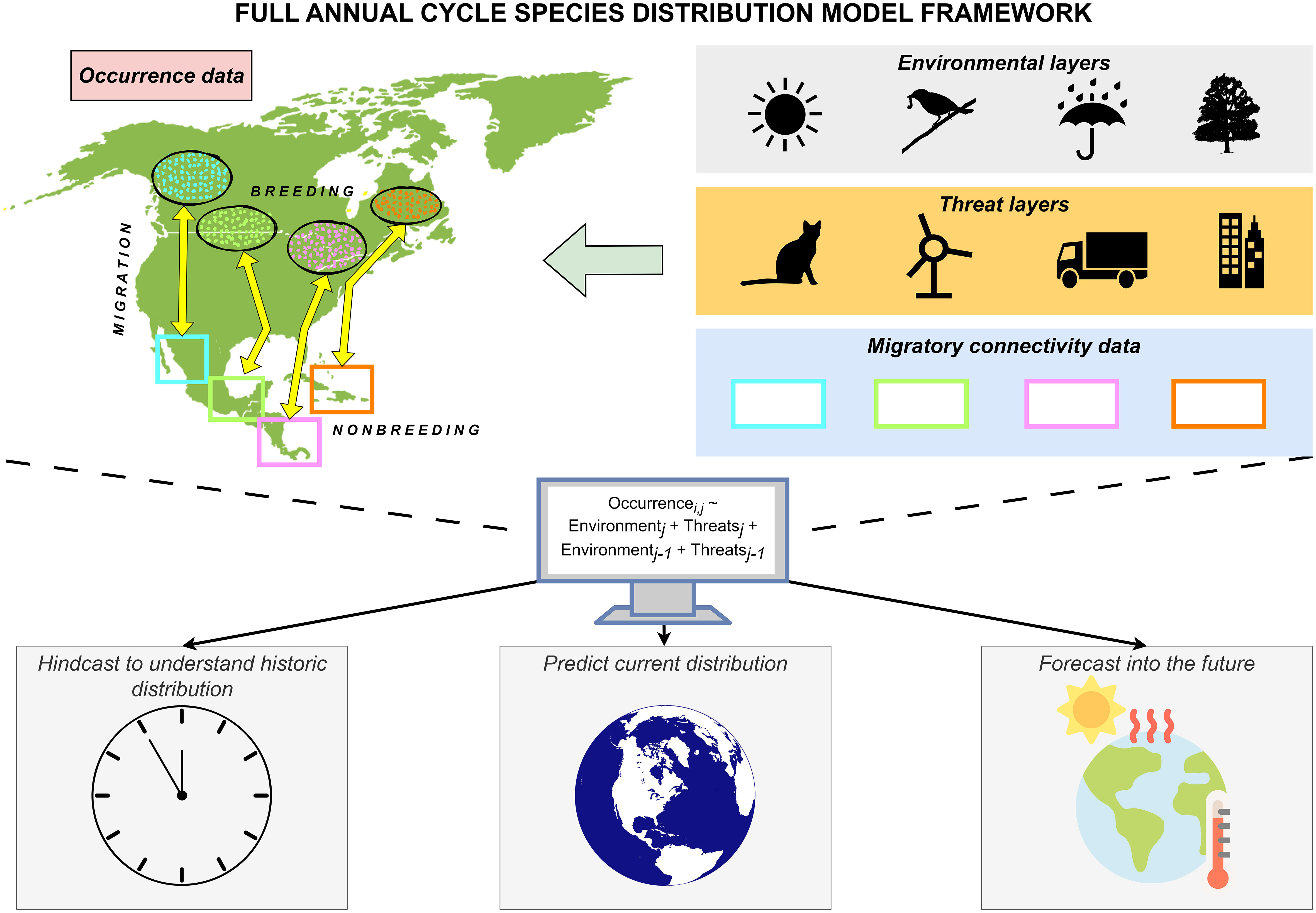
Figure 5 Conceptual framework demonstrating our proposed full annual cycle species distribution model. First, choose a season of the annual cycle during which to model a species’ distribution (e.g., breeding). Then, divide spatially-referenced occurrence data into discrete populations based on the species’ migratory connectivity (e.g., cyan, light green, pink, and orange populations). Model each population as a function of (1) relevant environmental (e.g., temperature, food and competition, precipitation, and habitat) and threat layers (e.g., cats, renewable energy structures, roads, and land-use change) from the species’ range during that season (i.e., breeding, or j), and (2) relevant environmental and threat layers from the season before (i.e., nonbreeding, or j-1) to account for seasonal interactions known to affect the focal species. To do this, set priors so that each discrete population only responds to environmental and threat layers from the geographic areas where they occur during relevant periods of the annual cycle (e.g., for the cyan population, set priors to 0 for environmental and threat layers that occur anywhere besides British Columbia and the Pacific slope of Mexico). Together, the full annual cycle SDM models occurrence data for population i from season j as a function of environmental and threat layers from season j and environmental and threat layers from season j-1. Finally, use the derived relationships between each discrete population and relevant environmental & threat layers to hindcast past distributional change, estimate current distributions, or forecast how distributions might change into the future.
Developing full annual cycle SDMs that connect populations within a species’ range throughout the annual cycle and incorporate the effects of seasonal interactions remains an important frontier for macroecology. Challenges include first defining patterns of migratory connectivity, then determining appropriate ways for incorporating the effects of seasonal interactions, customizing and tailoring full annual cycle SDMs to the diversity of migratory behaviors exhibited by birds around the globe, and gathering relevant, global environmental data on threats. Meanwhile, we face an increasing time constraint as the drivers of global change continue to quicken, strengthen, and become more volatile (IPCC 2023). How current environmental and anthropogenic processes impact future species distributions and abundance is one of the major questions and challenges of our time. Building realistic modeling frameworks based on accurate species biology is imperative to us making advances toward these challenges.
Author contributions
HS, EW, CS, and PM conceived the idea for the paper. All authors contributed to data collection. HS analyzed the data and HS and BD produced the figures in the paper. HS, EW, CS, BD, and IC wrote the paper. All authors contributed to the article and approved the submitted version.
Funding
Funding and support was provided by the Department of Biology and the Earth Commons at Georgetown University.
Acknowledgments
We thank Dr. Benjamin Zuckerberg, Dr. Lane Scher, and two anonymous reviewers for their helpful comments that improved the manuscript.
Conflict of interest
The authors declare that the research was conducted in the absence of any commercial or financial relationships that could be construed as a potential conflict of interest.
Publisher’s note
All claims expressed in this article are solely those of the authors and do not necessarily represent those of their affiliated organizations, or those of the publisher, the editors and the reviewers. Any product that may be evaluated in this article, or claim that may be made by its manufacturer, is not guaranteed or endorsed by the publisher.
Supplementary material
The Supplementary Material for this article can be found online at: https://www.frontiersin.org/articles/10.3389/fbirs.2023.1230978/full#supplementary-material
References
Aagaard K. J., Lonsdorf E. V., Thogmartin W. E. (2022). Effects of weather variation on waterfowl migration: Lessons from a continental-scale generalizable avian movement and energetics model. Ecol. Evol. 12, 2. doi: 10.1002/ece3.8617
Adams T. B., Root V. K. (2022). Multi-scale responses of bird species to tree cover and development in an urbanizing landscape. Urban For. Urban Green. 73, 127601. doi: 10.1016/j.ufug.2022.127601
Almaraz P., Green A. J., Aguilera E., Rendon M. A., Bustamante J. (2012). Estimating partial observability and nonlinear climate effects on stochastic community dynamics of migratory waterfowl. J. Anim. Ecol. 81, 5. doi: 10.1111/j.1365-2656.2012.01972.x
Araujo H., Rodrigues P. C., Bastos-Santos J., Ferreira M., Pereira A., Martinez-Cedeira J., et al. (2022). Monitoring abundance and distribution of northern gannets Morus bassanus in Western Iberian waters in autumn by aerial surveys. Ardeola 69, 2. doi: 10.13157/arla.69.2.2022.ra1
Bauer S., Lisovski S., Hahn S. (2016). Timing is crucial for consequences of migratory connectivity. Oikos 125, 5. doi: 10.1111/oik.02706
Berthold P. (2001). Bird Migration: A General Survey (Oxford, England, UK: Oxford University Press).
Betts M. G., Gutiérrez Illán J., Yang Z., Shirley S. M., Thomas C. D. (2019). Synergistic effects of climate and land-cover change on long-term bird population trends of the Western USA: A test of modeled predictions. Front. Ecol. Evol. 7. doi: 10.3389/fevo.2019.00186
Brommer J. E., Lehikoinen A., Valkama J. (2012). The breeding ranges of Central European and Arctic bird species move poleward. PloS One 7, 9. doi: 10.1371/journal.pone.0043648
Brown J. L., Li S.-H., Bhagabati N. (1999). Long-term trend toward earlier breeding in an American bird: A response to global warming? Proc. Natl. Acad. Sci. U.S.A. 96, 10. doi: 10.1073/pnas.96.10.5565
Callaghan C. T., Nakagawa S., Cornwell W. K. (2021). Global abundance estimates for 9,700 bird species. Proc. Natl. Acad. Sci. U.S.A. 118, 21. doi: 10.1073/pnas.2023170118
Cao C., De Luccia F. J., Xiong X., Wolfe R., Weng F. (2014). Early on-orbit performance of the visible infrared imaging radiometer suite onboard the suomi national polar-orbiting partnership (S-NPP) satellite. IEEE Trans. Geosci. Remote. Sens. 52, 1142–1156. doi: 10.1109/TGRS.2013.2247768
Carey C. (2009). The impacts of climate change on the annual cycles of birds. Phil. Trans. R. Soc B. 364, 1534. doi: 10.1098/rstb.2009.0182
Chapman B. B., Brönmark C., Nilsson J.-Å., Hansson L.-A. (2011). The ecology and evolution of partial migration. Oikos 120, 12. doi: 10.1111/j.1600-0706.2011.20131.x
Chen I.-C., Hill J. K., Ohlemüller R., Roy D. B., Thomas C. D. (2011). Rapid range shifts of species associated with high levels of climate warming. Science 333, 6045. doi: 10.1126/science.1206432
Conklin J. R., Senner N. R., Battley P. F., Piersma T. (2017). Extreme migration and the individual quality spectrum. J. Avian Biol. 48, 1. doi: 10.1111/jav.01316
Culp L. A., Cohen E. B., Scarpignato A. L., Thogmartin W. E., Marra P. P. (2017). Full annual cycle climate change vulnerability assessment for migratory birds. Ecosphere 8, 3. doi: 10.1002/ecs2.1565
Culumber Z. W., Anaya-Rojas J. M., Booker W. W., Hooks A. P., Lange E. C., Pluer B., et al. (2019). Widespread biases in ecological and evolutionary studies. BioScience 69, 8. doi: 10.1093/biosci/biz063
Di Marco M., Chapman S., Althor G., Kearney S., Besancon C., Butt N., et al. (2017). Changing trends and persisting biases in three decades of conservation science. Glob. Ecol. Conserv. 10, 32–42 doi: 10.1016/j.gecco.2017.01.008
Dossman B. C., Rodewald A. D., Studds C. E., Marra P. P. (2023a). Migratory birds with delayed spring departure migrate faster but pay the costs. Ecology 104, 2. doi: 10.1002/ecy.3938
Dossman B. C., Studds C. E., LaDeau S. L., Sillett T. S., Marra P. P. (2023b). "The role of tropical rainfall in driving range dynamics for a long-distance migratory bird" in Proceedings of the National Academy of Sciences. In Review.
Dunn P. O., Winkler D. W. (1999). Climate change has affected the breeding date of tree swallows throughout North America. Proc. R. Soc Lond. B. 266, 1437. doi: 10.1098/rspb.1999.0950
Elith J., Kearney M., Phillips S. (2010). The art of modelling range-shifting species. Methods Ecol. Evol. 1, 4. doi: 10.1111/j.2041-210X.2010.00036.x
Engler J. O., Stiels D., Schidelko K., Strubbe D., Quillfeldt P., Brambilla M. (2017). Avian SDMs: current state, challenges, and opportunities. J. Avian Biol. 48, 12. doi: 10.1111/jav.01248
Faaborg J. R. T., Holmes A. D., Anders K. L., Bildstein K. M., Dugger S. A., Gauthreaux P., et al. (2010). Recent advances in understanding migration systems of New World land birds. Ecol. Monogr. 80, 3–48.
Fauchald P., Tarroux A., Bråthen V. S., Descamps S., Ekker M., Helgason H. H., et al. (2019). Arctic-breeding seabirds’ hotspots in space and time - A methodological framework for year-round modelling of environmental niche and abundance using light-logger data. NINA Report 1657 (Trondheim, Norway: Norwegian Institute for Nature Research).
Feldman M. J., Imbeau L., Marchand P., Mazerolle M. J., Darveau M., Fenton N. J. (2021). Trends and gaps in the use of citizen science derived data as input for species distribution models: A quantitative review. PloS One 16, 3. doi: 10.1371/journal.pone.0234587
Fink D., Auer T., Johnston A., Ruiz-Gutierrez V., Hochachka W. M., Kelling S. (2020). Modeling avian full annual cycle distribution and population trends with citizen science data. Ecol. Appl. 30, 3. doi: 10.1002/eap.2056
Fink D., Auer T., Johnston A., Strimas-Mackey M., Ligocki S., Robinson O., et al. (2022). eBird Status and Trends, Data Version: 2021; Released: 2022 (Ithaca, New York: Cornell Lab of Ornithology). doi: 10.2173/ebirdst.2021
Foo Y. Z., O’Dea R. E., Koricheva J., Nakagawa S., Lagisz M. (2021). A practical guide to question formation, systematic searching and study screening for literature reviews in ecology and evolution. Methods Ecol. Evol. 12, 1705–1720. doi: 10.1111/2041-210X.13654
Fourcade Y., Besnard A. G., Secondi J. (2018). Paintings predict the distribution of species, or the challenge of selecting environmental predictors and evaluation statistics. Global Ecol. Biogeogr. 27, 2. doi: 10.1111/geb.12684
Friedl M., Sulla-Menashe D. (2019). MCD12Q1 MODIS/Terra+Aqua Land Cover Type Yearly L3 Global 500m SIN Grid V006 [Data set] (USA: NASA EOSDIS Land Processes DAAC).
Gaston K. J. (2009). Geographic range limits: achieving synthesis. Proc. R. Soc B. 276, 1661. doi: 10.1098/rspb.2008.1480
Gilroy J. J., Gill J. A., Butchart S. H. M., Jones V. R., Franco A. M. A. (2016). Migratory diversity predicts population declines in birds. Ecol. Lett. 19, 3. doi: 10.1111/ele.12569
Guisan A., Thuiller W. (2005). Predicting species distribution: Offering more than simple habitat models. Ecol. Lett. 8, 993–1009. doi: 10.1111/J.1461-0248.2005.00792.X
Haddaway N. R., Bethel A., Dicks L. V., Koricheva J., Macura B., Petrokofsky G., et al. (2020). Eight problems with literature reviews and how to fix them. Nat. Ecol. Evol. 4, 1582–1589. doi: 10.1038/s41559-020-01295-x
Herrera D., Ault T. (2017). Insights from a new high-resolution drought atlas for the Caribbean spanning 1950–2016. J. Clim. 30, 19. doi: 10.1175/JCLI-D-16-0838.1
Hitch A. T., Leberg P. L. (2007). Breeding distributions of North American bird species moving north as a result of climate change. Conserv. Biol. 21, 2. doi: 10.1111/j.1523-1739.2006.00609.x
Hostetler J. A., Sillett T. S., Marra P. P. (2015). Full-annual-cycle population models for migratory birds. Auk. 132, 2. doi: 10.1642/AUK-14-211.1
Howard C., Stephens P. A., Pearce-Higgins J. W., Gregory R. D., Willis S. G. (2014). Improving species distribution models: the value of data on abundance. Methods Ecol. Evol. 5, 6. doi: 10.1111/2041-210X.12184
Hsiung A. C., Boyle W. A., Cooper R. J., Chandler R. B. (2018). Altitudinal migration: ecological drivers, knowledge gaps, and conservation implications: Animal altitudinal migration review. Biol. Rev. 93, 4. doi: 10.1111/brv.12435
Illán J. G., Thomas C. D., Jones J. A., Wong W.-K., Shirley S. M., Betts M. G. (2014). Precipitation and winter temperature predict long-term range-scale abundance changes in Western North American birds. Glob. Change Biol. 20, 11. doi: 10.1111/gcb.12642
IPCC (2023). "Climate change 2023: synthesis report," in A report of the intergovernmental panel on climate change. contribution of working groups i, ii and iii to the sixth assessment report of the intergovernmental panel on climate change. Eds. Core Writing Team, Lee H., Romero J.. (Geneva, Switzerland: IPCC).
Jahn A. E., Bravo S. P., Cueto V. R., Levey D. J., Morales M. V. (2012). Patterns of partial avian migration in northern and southern temperate latitudes of the New World. Emu 112, 1. doi: 10.1071/MU10091
Jahn A. E., Lerman S. B., Phillips L. M., Ryder T. B., Williams E. J. (2019). First tracking of individual American Robins (Turdus migratorius) across seasons. Wilson J. Ornithol. 131, 2. doi: 10.1676/18-124
Jahn A. E., Levey D. J., Smith K. G. (2004). Reflections across hemispheres: A system-wide approach to New World bird migration. Auk. 121, 4. doi: 10.1093/auk/121.4.1005
Jetz W., Wilcove D. S., Dobson A. P. (2007). Projected impacts of climate and land-use change on the global diversity of birds. PloS Biol. 5, 6. doi: 10.1371/journal.pbio.0050157
Jiguet F., Gadot A.-S., Julliard R., Newson S. E., Couvet D. (2007). Climate envelope, life history traits and the resilience of birds facing global change. Glob. Change Biol. 13, 8. doi: 10.1111/j.1365-2486.2007.01386.x
Klaassen R. H. G., Hake M., Strandberg R., Koks B. J., Trierweiler C., Exo K.-M., et al. (2014). When and where does mortality occur in migratory birds? Direct evidence from long-term satellite tracking of raptors. J. Anim. Ecol. 83, 1. doi: 10.1111/1365-2656.12135
Lamb J. S., Paton P. W. C., Osenkowski J. E., Badzinski S. S., Berlin A. M., Bowman T., et al. (2020). Assessing year-round habitat use by migratory sea ducks in a multi-species context reveals seasonal variation in habitat selection and partitioning. Ecography 43, 12. doi: 10.1111/ecog.05003
Langham G. M., Schuetz J. G., Distler T., Soykan C. U., Wilsey C. (2015). Conservation status of North American birds in the face of future climate change. PloS One 10, 9. e0135350. doi: 10.1371/journal.pone.0135350
La Sorte F. A., Fink D., Blancher P. J., Rodewald A. D., Ruiz-Gutierrez V., Rosenberg K. V., et al. (2017). Global change and the distributional dynamics of migratory bird populations wintering in Central America. Glob. Change Biol. 23, 12. doi: 10.1111/gcb.13794
Lee-Yaw J. A., McCune J. L., Pironon S., Sheth S. N. (2022). Species distribution models rarely predict the biology of real populations. Ecography 2022, 6. doi: 10.1111/ecog.05877
Levey D. J. (1994). Why we should adopt a broader view of Neotropical migrants. Auk. 111, 1. doi: 10.2307/4088533
Lewis S. L., Maslin M. A. (2015). Defining the Anthropocene. Nature 519, 7542. doi: 10.1038/nature14258
Loring P. H., Paton P. W., Osenkowski J. E., Gilliland S. G., Savard J. P. L., Mcwilliams S. R. (2014). Habitat use and selection of black scoters in southern New England and siting of offshore wind energy facilities. J. Wildl. Manage. 78, 4. doi: 10.1152/japplphysiol.00552.2014
Loss S. R., Will T., Marra P. P. (2015). Direct mortality of birds from anthropogenic causes. Annu. Rev. Ecol. Evol. Syst. 46, 1. doi: 10.1146/annurev-ecolsys-112414-054133
Marra P. P., Cohen E. B., Loss S. R., Rutter J. E., Tonra C. M. (2015). A call for full annual cycle research in animal ecology. Biol. Lett. 11, 8. doi: 10.1098/rsbl.2015.0552
Marra P. P., Hobson K. A., Holmes R. T. (1998). Linking winter and summer events in a migratory bird by using stable-carbon isotopes. Science 282, 5395. doi: 10.1126/science.282.5395.1884
McLaren J. D., Buler J. J., Schreckengost T., Smolinsky J. A., Boone M., van Loon E. E., et al. (2018). Artificial light at night confounds broad-scale habitat use by migrating birds. Ecol. Lett. 21, 3. doi: 10.1111/ele.12902
Neelin J. D., Münnich M., Su H., Meyerson J. E., Holloway C. E. (2006). Tropical drying trends in global warming models and observations. Proc. Natl. Acad. Sci. U.S.A. 103, 16. doi: 10.1073/pnas.0601798103
Nilsson A. L. K., Lindström Å., Jonzén N., Nilsson S. G., Karlsson L. (2006). The effect of climate change on partial migration – the blue tit paradox. Glob. Change Biol. 12, 10. doi: 10.1111/j.1365-2486.2006.01237.x
Norberg A., Abrego N., Blanchet F. G., Adler F. R., Anderson B. J., Anttila J., et al. (2019). A comprehensive evaluation of predictive performance of 33 species distribution models at species and community levels. Ecol. Monogr. 89, 3. doi: 10.1002/ecm.1370
Norris D. R., Marra P. P. (2007). Seasonal interactions, habitat quality, and population dynamics in migratory birds. Condor. 109, 3. doi: 10.1093/condor/109.3.535
Pasgaard M., Dalsgaard B., Maruyama P. K., Sandel B., Strange N. (2015). Geographical imbalances and divides in the scientific production of climate change knowledge. Glob. Environ. Change 35, 279–288. doi: 10.1016/j.gloenvcha.2015.09.018
Patchett R., Finch T., Cresswell W. (2018). Population consequences of migratory variability differ between flyways. Curr. Biol. 28, 8. doi: 10.1016/j.cub.2018.03.018
Pereira J. M., Kruger L., Oliveira N., Meirinho A., Silva A., Ramos J. A., et al. (2018). Using a multi-model ensemble forecasting approach to identify key marine protected areas for seabirds in the Portuguese coast. Ocean Coast. Manag. 153, 98–107. doi: 10.1016/j.ocecoaman.2017.12.014
Rigal S., Dakos V., Alonso H., Auniņš A., Benkő Z., Brotons L., et al. (2023). Farmland practices are driving bird population decline across Europe. Proc. Natl. Acad. Sci. U.S.A. 120, 21. doi: 10.1073/pnas.2216573120
R Core Team. (2022). R: a language and environment for statistical computing. (Vienna, Austria: R Foundation For Statistical Computing). Available at: http://www.R-project.org/.
Robbins C. S., Sauer J. R., Greenberg R. S., Droege S. (1989). Population declines in North American birds that migrate to the Neotropics. Proc. Natl. Acad. Sci. U.S.A. 86, 19. doi: 10.1073/pnas.86.19.7658
Rockwell S. M., Bocetti C. I., Marra P. P. (2012). Carry-over effects of winter climate on spring arrival date and reproductive success in an endangered migratory bird, Kirtland’s Warbler (Setophaga kirtlandii). Auk. 129, 4. doi: 10.1525/auk.2012.12003
Rockwell S. M., Wunderle J. M., Sillett T. S., Bocetti C. I., Ewert D. N., Currie D., et al. (2017). Seasonal survival estimation for a long-distance migratory bird and the influence of winter precipitation. Oecologia 183, 715–726. doi: 10.1007/s00442-016-3788-x
Rosenberg K. V., Dokter A. M., Blancher P. J., Sauer J. R., Smith A. C., Smith P. A., et al. (2019). Decline of the north American avifauna. Science 366, 6461. doi: 10.1126/science.aaw1313
Rushing C. S., Hostetler J. A., Sillett T. S., Marra P. P., Rotenberg J. A., Ryder T. B. (2017). Spatial and temporal drivers of avian population dynamics across the annual cycle. Ecology 98, 11. doi: 10.1002/ecy.1967
Rushing C. S., Royle J. A., Ziolkowski D. J., Pardieck K. L. (2020). Migratory behavior and winter geography drive differential range shifts of eastern birds in response to recent climate change. Proc. Natl. Acad. Sci. U.S.A. 117, 23. doi: 10.1073/pnas.2000299117
Rushing C. S., Ryder T. B., Marra P. P. (2016). Quantifying drivers of population dynamics for a migratory bird throughout the annual cycle. Proc. Biol. Sci. 283, 1823. doi: 10.1098/rspb.2015.2846
Senner N. R., Verhoeven M. A., Abad-Gómez J. M., Alves J. A., Hooijmeijer J. C., Howison R. A., et al. (2019). High migratory survival and highly variable migratory behavior in black-tailed godwits. Front. Ecol. Evol. 7. doi: 10.3389/fevo.2019.00096
Sexton J. P., McIntyre P. J., Angert A. L., Rice K. J. (2009). Evolution and ecology of species range limits. Annu. Rev. Ecol. Evol. Syst. 40, 415–436. doi: 10.1146/annurev.ecolsys.110308.120317
Shipley J. R., Twining C. W., Taff C. C., Vitousek M. N., Flack A., Winkler D. W. (2020). Birds advancing lay dates with warming springs face greater risk of chick mortality. Proc. Natl. Acad. Sci. U.S.A. 117, 41. doi: 10.1073/pnas.2009864117
Sillett T. S., Holmes R. T. (2002). Variation in survivorship of a migratory songbird throughout its annual cycle. J. Anim. Ecol. 71, 2. doi: 10.1046/j.1365-2656.2002.00599.x
Small-Lorenz S. L., Culp L. A., Ryder T. B., Will T. C., Marra P. P. (2013). A blind spot in climate change vulnerability assessments. Nat. Clim. Change. 3, 2. doi: 10.1038/nclimate1810
Soares L., Cockle K. L., Ruelas Inzunza E., Ibarra J. T., Miño C. I., Zuluaga S., et al. (2023). Neotropical ornithology: Reckoning with historical assumptions, removing systemic barriers, and reimagining the future. Condor 125, 1. doi: 10.1093/ornithapp/duac046
Socolar J. B., Epanchin P. N., Beissinger S. R., Tingley M. W. (2017). Phenological shifts conserve thermal niches in North American birds and reshape expectations for climate-driven range shifts. Proc. Natl. Acad. Sci. U.S.A. 114, 49. doi: 10.1073/pnas.1705897114
Stephens P. A., Mason L. R., Green R. E., Gregory R. D., Sauer J. R., Alison J., et al. (2016). Consistent response of bird populations to climate change on two continents. Science 352, 6281. doi: 10.1126/science.aac4858
Stevens H. C., Smith A. C., Buechley E. R., Şekercioğlu Ç. H., Shirey V. M., Rosenberg K. V., et al. (2023). Species-specific ecological traits, phylogeny, and geography underpin vulnerability to population declines for North American birds. Ornitholog. Appl. doi: 10.1093/ornithapp/duad046
Studds C. E., Marra P. P. (2011). Rainfall-induced changes in food availability modify the spring departure programme of a migratory bird. Proc. R. Soc B. 278, 1723. doi: 10.1098/rspb.2011.0332
Taylor C. M., Norris D. R. (2007). Predicting conditions for migration: effects of density dependence and habitat quality. Biol. Lett. 3, 3. doi: 10.1098/rsbl.2007.0053
Temple S. A. (1988). What’s behind long-term declines in some breeding bird populations? Passenger Pigeon. 50, 133–138.
Tobias J. A., Sheard C., Pigot A. L., Devenish A. J. M., Yang J., Sayol F., et al. (2022). AVONET: morphological, ecological and geographical data for all birds. Ecol. Lett. 25, 3. doi: 10.1111/ele.13898
Townsend A. K., Sillett T. S., Lany N. K., Kaiser S. A., Rodenhouse N. L., Webster M. S., et al. (2013). Warm springs, early lay dates, and double brooding in a North American migratory songbird, the black-throated blue warbler. PloS One 8, 4. doi: 10.1371/journal.pone.0059467
Van Doren B. M., Willard D. E., Hennen M., Horton K. G., Stuber E. F., Sheldon D., et al. (2021). Drivers of fatal bird collisions in an urban center. Proc. Natl. Acad. Sci. U.S.A. 118, 24. doi: 10.1073/pnas.2101666118
Webster M. S., Marra P. P., Haig S. M., Bensch S., Holmes R. T. (2002). Links between worlds: unraveling migratory connectivity. Trends Ecol. Evol. 17, 2. doi: 10.1016/S0169-5347(01)02380-1
Winger B. M., Auteri G. G., Pegan T. M., Weeks B. C. (2019). A long winter for the Red Queen: rethinking the evolution of seasonal migration. Biol. Rev. 94, 3. doi: 10.1111/brv.12476
Winger B. M., Barker F. K., Ree R. H. (2014). Temperate origins of long-distance seasonal migration in New World songbirds. Proc. Natl. Acad. Sci. U.S.A. 111, 33. doi: 10.1073/pnas.1405000111
Wüest R. O., Zimmermann N. E., Zurell D., Alexander J. M., Fritz S. A., Hof C., et al. (2020). Macroecology in the age of big data – Where to go from here? J. Biogeogr. 47, 1. doi: 10.1111/jbi.13633
Yates K. L., Bouchet P. J., Caley M. J., Mengersen K., Randin C. F., Parnell S., et al. (2018). Outstanding challenges in the transferability of ecological models. Trends Ecol. Evol. 33, 10. doi: 10.1016/J.TREE.2018.08.001
Zuckerberg B., Fink D., La Sorte F. A., Hochachka W. M., Kelling S. (2016). Novel seasonal land cover associations for eastern North American forest birds identified through dynamic species distribution modelling. Divers. Distrib. 22, 6. doi: 10.1111/ddi.12428
Zurell D., Franklin J., König C., Bouchet P. J., Dormann C. F., Elith J., et al. (2020). A standard protocol for reporting species distribution models. Ecography 43, 9. doi: 10.1111/ecog.04960
Keywords: ecological niche modeling, macroecological model, bioclimatic model, migration, migratory birds, full annual cycle ecology, seasonal interactions
Citation: Stevens HC, Williams EJ, Stanley CQ, Dossman BC, Ciaburri I, Cooper NW, Bowden LS, Dees CM, Huang J, McCabe J, Wyman B and Marra PP (2023) Incorporating drivers of global change throughout the annual cycle in species distribution models for migratory birds: a gap in ecological forecasting. Front. Bird Sci. 2:1230978. doi: 10.3389/fbirs.2023.1230978
Received: 29 May 2023; Accepted: 22 August 2023;
Published: 25 September 2023.
Edited by:
Benjamin Zuckerberg, University of Wisconsin-Madison, United StatesReviewed by:
Jesús Domínguez, University of Santiago de Compostela, SpainFranz Bairlein, Heligoland Bird Observatory, Germany
Copyright © 2023 Stevens, Williams, Stanley, Dossman, Ciaburri, Cooper, Bowden, Dees, Huang, McCabe, Wyman and Marra. This is an open-access article distributed under the terms of the Creative Commons Attribution License (CC BY). The use, distribution or reproduction in other forums is permitted, provided the original author(s) and the copyright owner(s) are credited and that the original publication in this journal is cited, in accordance with accepted academic practice. No use, distribution or reproduction is permitted which does not comply with these terms.
*Correspondence: Henry C. Stevens, aGVucnljc3RldmVuc0BnbWFpbC5jb20=; Emily J. Williams, ZmZ5bmdhdUBnbWFpbC5jb20=