- 1Department of Biotechnology and Biosciences, University of Milano-Bicocca, Milan, Italy
- 2Institute for Advanced Simulations, Forschungszentrum Juelich, Juelich, Germany
Proteins carry out their biological activity as dynamic structures and populate in solution or in biological membranes structural distributions with different degrees of heterogeneity. The central challenge in structural biology is to capture protein structural dynamics under equilibrium or kinetic conditions shifting from single, static pictures to movies of conformational ensembles. Ideally, this task should be pursued both in vitro and in vivo, under the influence of the native environment. The last decade has seen a tremendous development of biophysical methods for the investigation of protein structure and dynamics. However, each method has specific limitations and no single approach offers such a complex level of description. Nonetheless, the combination of experimental and computational, complementary methods is opening promising new avenues. Also the ambition of implementing structural studies on an “omic” scale is becoming more and more realistic. In spite of still major limitations, integrative structural biology is bringing dynamics into structural proteomics, with exciting perspectives for basic and applied sciences.
Introduction
Proteins are the main actors determining phenotype in the biological world. Their structural characterization is essential to understand, design and modify protein function, impacting on basic and applied research. Protein structures in solution or membranes are not static and interconvert among different conformations with different degrees of flexibility on a continuum from highly constrained to completely disordered states. A central challenge of structural biology is the dynamic characterization of conformational ensembles, rather than the determination of single structures or static ensembles that may represent only snapshots of biologically relevant transitions. Protein dynamics is relevant to virtually any kind of protein activity and manifests itself at multiple levels: conformational dynamics, conformational changes, protein folding and intermolecular interactions.
Molecular recognition is a general phenomenon that highlights the importance of conformational dynamics in shaping protein affinity and specificity (Chu et al., 2021; Carey, 2022). Consistently, the performance of drug-design methods is strongly affected by the extent of protein flexibility that is taken into account (Bekker and Kamiya, 2022). Enzymatic catalysis is intimately linked to protein structure dynamics (Fürst et al., 2019; Lycus et al., 2023) and catalytic properties can be illuminated by modeling transition-state ensembles (Bunzel et al., 2021). Conformation-based models for allostery are being displaced by a view invoking vibrations and fluctuations from the entire protein structure and associated entropy changes mediating allosteric communication (Madan et al., 2023). Chain flexibility has been adjusted by evolution to adapt to different environments, as shown by proteins from psychrophilic, mesophilic and thermophilic organisms (Arcus and Mulholland, 2020; Rabbani et al., 2023).
The mechanism of protein folding has been a “Holy Grail” of modern biology. Again, the key seems to be depicting conformational ensembles according to a rough energy landscape of protein folding with populated metastable states (Biasini and Faccioli, 2023). A related open question remains how to model the unfolded state, the goal being characterization of conformational ensembles along the folding coordinate from the unfolded to the native state. Furthermore, the intracellular environment affects conformational ensembles, stability and dynamic quaternary structure by crowding effects, phase transitions, post-translational modifications (PTMs) and transient intermolecular interactions (quinary structure) (Guin and Gruebele, 2019; Selenko, 2019; Marciano et al., 2022). The physiological folding process is in competition with aberrant pathways leading to misfolded conformations and amyloid aggregates. Innovative diagnostic and therapeutic strategies for amyloid diseases needs to take into account the dynamics of amyloidogenic proteins and supramolecular complexes (Sun et al., 2023).
Molecular evolution has posed varying degrees of selective pressure on protein dynamics (Patil, 2022). Some biological functions, mostly regulatory switches, involve proteins or protein regions that do not fold by themselves into an ordered three-dimensional structure. These “intrinsically disordered proteins” (IDPs) or “intrinsically disordered regions” (IDRs) may fold partially or completely upon binding a partner. This adaptability may enable combinatorial interactions with multiple partners (Chakrabarti and Chakravarty, 2022). More than half of the human proteome contains predicted IDRs of 30 residues or longer (Toth-Petroczy et al., 2016). The functional advantages of structural disorder coexist with the threat of misfolding and aggregation (Tsoi et al., 2023). IDPs and IDRs are key actors in physiology, as well as in many devastating diseases, and represent targets of central pharmacological interest (Choudhary et al., 2022).
Molecular chaperones (Margulies et al., 2022), molecular motors (Ariga et al., 2020), pores (Hendriks et al., 2021), transporters (Hou et al., 2022), and host proteins of membraneless organelles (Hardenberg et al., 2020) provide additional examples of the biological relevance of protein structural dynamics.
Methodological approaches
The shift from static to dynamic protein characterization poses major technical challenges. The ambition is to approach equilibrium and kinetics, therefore dealing with heterogeneity of conformational ensembles and motions describing conformational transitions. Advancements in biophysical methods are bringing these difficult tasks within reach, but still many bottlenecks must be overcome. While no single method can give us the answers, the combination of multiple techniques in an “integrative structural biology” approach is highly promising (Evans et al., 2023). Computational methods have an increasingly important role in guiding, interpreting and complementing experiments, and multiple experimental approaches exploiting orthogonal principles can be combined.
The experimental techniques yielding atomic-resolution models of protein structures are X-ray crystallography, cryogenic electron microscopy (cryo-EM) and nuclear magnetic resonance (NMR). By observing molecules in a crystal lattice, X-ray crystallography has limitations in studying protein dynamics. However, the development of time-resolved crystallography by X-ray free electron lasers (XFELs) has opened new avenues, yielding serial diffraction data-sets from protein microcrystals with femtosecond time resolution (serial femtosecond crystallography, SFX) and overcoming radiation damages by a “diffraction-before-destruction” approach (Liu and Lee, 2019). Combination with light pulses has revealed structural dynamics and transient states in light-sensitive proteins (Malla and Schmidt, 2022; Weik and Domratcheva, 2022). Other protein types can be approached, by using substrate or ligand diffusion, rather than irradiation, as an external stimulus (Malla and Schmidt, 2022).
Limitations of this approach are the dependence on crystallization, which can be difficult or impossible for some proteins and can affect conformational states, and data averaging, rather than yielding single-particle information. The latter view is of utmost importance in the investigation of heterogeneous systems. Thus, further development of XFEL technology towards single particle imaging (SPI) is highly attractive. An innovative perspective is to exploit the ion-sorting capabilities of mass spectrometry (MS) for sample injection in XFEL-SPI (Kadek et al., 2021), replacing the flow of microcrystals by the flow of single particles produced by electrospray ionization (ESI). These could then be presented to XFEL after mass selection and possibly also conformational selection by ion mobility (IM). Future improvements in XFEL power and resolution, and advances in hybrid technologies, could make this approach available for in-depth analysis of protein conformational ensembles.
Cryo-EM has entered into the realm of high-resolution techniques for protein structure determination. Rapid sample vitrification at cryogenic temperatures into a thin layer of vitreous ice, followed by EM imaging of a high number of single particles, allows reconstructing three-dimensional structures with atomic resolution, bypassing crystallization (Guaita et al., 2022). Rapid mixing and rapid freezing technologies are making time-resolved cryo-EM a reality on the millisecond time scale, although optimization will be needed for routine practice and improved time resolution (Klebl et al., 2021; Mäeots and Enchev, 2022). Cryo-EM can face the dual challenges of kinetic and equilibrium heterogeneity. Ensemble reconstruction into distinct conformers is already well established and progress is being made in methodologies to face continuous conformational heterogeneity (Toader et al., 2023). These tools will bring us closer to the fundamental goal of depicting conformational ensembles at atomic resolution.
NMR measures protein structural features in solution or solid state. It describes protein dynamics by an array of different protocols and observables (Camacho-Zarco et al., 2022; Nishiyama et al., 2023) on a wide time scale, from days to picoseconds in combination with molecular-dynamics (MD) simulations (Stenström et al., 2022). NMR has treated highly dynamic systems, such as IDPs (Dyson and Wright, 2021; Camacho-Zarco et al., 2022), and difficult-to-handle systems, such as membrane proteins (Xue et al., 2021; Günsel and Hagn, 2022) and amyloid aggregates (Bonaccorsi et al., 2021). NMR is inherently a bulk method with little potential for single-molecule implementations and is exposed to the issue of signal averaging, depending on the time scale of the transitions and the procedure employed. However, the combination with MD simulations and low-resolution methods describing species distributions is a powerful approach to tackle structural heterogeneity (Harish et al., 2017). Solid-state NMR at cryogenic temperatures could open new avenues to dissecting disordered ensembles (Kragelj et al., 2023). Combination with rapid-mixing and freeze-trapping technologies offers promising perspectives for kinetic studies, e.g., capturing intermediates of protein folding and assembly on the millisecond timescale (Jeon et al., 2019).
Ideally, we would also like to investigate proteins in vivo, under the influence of their native environment. The advancements of in-cell and in-situ NMR offer unique possibilities in this regard (Luchinat and Banci, 2022). In-cell NMR, in either solution- or solid-state, has already successfully characterized folded, soluble proteins disordered ensembles, PTMs, quinary structure, ligand binding, dynamics of membrane proteins and amyloid aggregation (Selenko, 2019; Bonaccorsi et al., 2021; Theillet and Luchinat, 2022). One challenge is sample maintenance during the long acquisition times. Dedicated bioreactors seem a promising perspective. Microorganisms and mammalian cells have proven amenable to in-cell NMR. Possibly, organoids or small animals will become tractable in the future, thanks to improved sensitivity. Again, the most promising perspective lies in hybrid strategies, particularly exploiting the complementarity of NMR and cryo-electron tomography (cryo-ET) (Theillet and Luchinat, 2022).
Cryo-ET takes projection images of appropriately thinned biological samples, such as cells and tissues, under cryogenic conditions from different angles, computationally reconstructing three-dimensional images (Doerr, 2017; Moebel and Kervrann, 2022). The presently limited resolution is offset by the amplitude of the inspected field, which could in principle embrace the entire proteome, motivating expectations for the emerging new field of “visual proteomics” (Baumeister, 2022). Averaging over multiple copies can lead to near-atomic resolution, although not yet for low-abundance or small structures. The so-called “resolution revolution” will likely continue, opening new avenues to in-situ structural biology (Kwon, 2021). Cryo-ET could address structural heterogeneity and fast-freezing technology could be adaptable to kinetic studies.
A very important synergism can be established between high-resolution techniques that struggle to dissect conformational ensembles and low-resolution techniques that describe, instead, structural heterogeneity. This synergism is well established for small-angle, X-ray or neutron scattering (SAXS, SANS), integrating measurements with advanced algorithms for spectra deconvolution and analysis of polydisperse systems (Da Vela and Svergun, 2020). Other methods, such as structural MS and atomic-force microscopy (AFM), go a step further, by physically sorting particles and assessing their individual properties, being intrinsically free from averaging shortcomings. Structural MS includes different strategies (Haubrich et al., 2023). Native MS provides species distributions of conformers and supramolecular complexes upon gentle desolvation and ionization, yielding values of stoichiometry and solvent accessible surface area (Tamara et al., 2022). Combination with IM introduces an additional dimension in ion separation and analysis, detecting structural heterogeneity even within each charge state and measuring rotationally-averaged, collisional cross-section (CCS) (Kaltashov et al., 2022; Santambrogio et al., 2022, Christofi and Barran, 2023; Reid et al., 2023).
Alternatively, conformation-sensitive labeling in solution is followed by denaturing MS analysis. Labeling can probe accessibility/flexibility, as isotope exchange (Masson et al., 2019), fast photochemical oxidation (FPOP) (Cornwell and Ault, 2022) and limited proteolysis (Malinovska et al., 2023), or generate distance restraints, as chemical cross-linking in vitro or in vivo (Piersimoni et al., 2022). This rich structural information can guide particle classification in cryo-EM/ET, ensemble deconvolution by NMR and computational modelling by experimental constraints. Structural heterogeneity deriving from PTMs, ligand binding and protein assemblies can also be described (Kaltashov et al., 2022; Reid et al., 2023). MS methods can in principle be implemented in a time-resolved mode on the millisecond scale (even microsecond for FPOP), although such instrumental setups are still not widespread (Lento and Wilson, 2022). An interesting hybrid approach (Martinez Molina et al., 2013) is thermal proteome profiling, which monitors proteome-wide changes in protein thermal stability in vitro or in vivo upon application of a stimulus (Mateus et al., 2020).
AFM measures height and force of a cantilever making interactions with proteins on a surface in aqueous media, a single molecule at a time (Müller et al., 2021; Yu and Yoshimura, 2021). The surface is scanned by a sharp tip, functionalized according to the experimental design, reaching speeds of ∼70 frames/second in high-speed AFM (HS-AFM) (Ando, 2022). AFM can be implemented for imaging (microscopy) of biological or nanostructured surfaces, or for unfolding and interaction analysis (force spectroscopy) on recombinant proteins and polyproteins. Images at micrometer-to-sub-nanometer resolution can be obtained on isolated proteins, membrane-mimetic systems, organelles, living cells and tissues (Müller et al., 2021; Yu and Yoshimura, 2021). HS-AFM provides movies of protein conformational dynamics, polymerization and depolymerization, folding and unfolding, binding and dissociation, and measurements of the involved forces (Jukic et al., 2023; Lostao et al., 2023).
AFM can be combined with microscopy and spectroscopy probes, achieving higher levels of morphological and biochemical characterization (Müller et al., 2021). Coupling with Fourier-transform infrared spectroscopy enables chemical imaging and quantitative secondary-structure determination of single protein molecules (Ruggeri et al., 2020). Complementation by optical and magnetic tweezers (Alegre-Cebollada, 2021) offers versatile approaches to study protein folding and protein machineries (Banerjee et al., 2021; Buzón et al., 2021; Dai et al., 2021). Förster Resonance Energy Transfer (FRET) brings single-molecule investigation inside living cells, with nanoscale spatiotemporal resolution (Puthenveetil et al., 2022). Although it requires protein labeling, it is a versatile method for in-vivo investigation of protein dynamics (Bhat and Blunck, 2022; Majumdar and Mukhopadhyay, 2022). It has also been combined with AFM for simultaneous recording of pulling force and FRET trajectories of individual unfolding events (He et al., 2012).
MD simulations describe vibrations and motions at atomic resolution, offering a unique view into mechanistic aspects of protein dynamics. Advancements in computational biophysics are making accessible progressively larger systems on progressively larger timescales (Borkotoky et al., 2022). Multiscale quantum mechanics/molecular mechanics (QM/MM) simulations bring quantum-mechanical accuracy into simulations of large protein structures and supramolecular complexes towards the exascale (Bolnykh et al., 2021; Vennelakanti et al., 2022; Kar, 2023). Supercomputers with exascale calculation power afford simulation times in the submillisecond (or even millisecond) timescale, covering biochemically relevant processes, from enzyme catalysis to conformational transitions and can simulate large supramolecular structures (millions of atoms) on the microsecond timescale (Bolnykh et al., 2021; Melo and Bernardi, 2023). Force fields are being optimized to face the specific complexity of folded, soluble proteins membrane proteins and IDPs (Piana et al., 2020; Coskuner-Weber and Caglayan, 2021; Robertson and Skiniotis, 2022). Enhanced sampling algorithms improve simulation performance on rough energy landscapes (Capelli et al., 2019; Hénin et al., 2022). The combination with machine-learning methods further improve investigation of transitions between metastable states and simulations of large ensembles (Ansari et al., 2021; Bhatia et al., 2023).
MD simulations are suitable for equilibrium and kinetic investigation of macromolecular systems. For the relatively small IDP α-synuclein (140 aa), simulations of the entire conformational ensemble, validated against NMR chemical shifts, describe the conformational landscape in the presence or absence of ligands (Robustelli et al., 2022). Large-scale, all-atom, explicit-solvent simulations have reached impressive sample sizes, such as the chromatophore organelle (Singharoy et al., 2019) and bacterial cytoplasm (Yu et al., 2016; Oliveira Bortot et al., 2020). Kinetic studies can capture transition states, calculate dissociation rates of protein-drug complexes (Ahmad et al., 2022; Sohraby and Nunes-Alves, 2022), simulate irreversible conformational changes (Ansari et al., 2021) and amyloid aggregation (Rizzuti, 2022). The role of the computational approach is continually expanding towards multidisciplinary investigation guided by reciprocal input and mutual validation with experiments (Paissoni and Camilloni, 2021; Sali, 2021) and the computational challenges become larger rather than smaller, as the experimental methods become more sophisticated and integrated. Exciting perspectives take shape, translating structural into functional models, designing new proteins, making disordered targets druggable and simulating protein dynamics in vivo.
A revolution we are witnessing is de-novo structural prediction of folded proteins at atomic resolution and near-experimental accuracy by deep-learning methods (AlQuraishi, 2021; Varadi et al., 2023). Notably, AlphaFold2 and RoseTTAFold decipher co-evolution of residue pairs from multiple sequence alignments and compute inter-residue distance probabilities, then guiding three-dimensional modeling (Baek et al., 2021; Jumper et al., 2021). Language models, such as ESMFold, learn to speak the protein language from known structures and predict structures from single sequences, relieving the requirement for multiple-sequence alignments and enabling exploration of regions of the protein universe that are not represented in current databases, including products of metagenomic sequences (Callaway, 2022; Lin et al., 2023). The number of parameters used to train the language model impacts on model resolution and fidelity. By increasing the ESMFold parameters up to 15 billion, model accuracy from single sequences compares with those by RoseTTAFold and AlphaFold2 from multiple sequence alignments (Lin et al., 2023). These impressive achievements make predicted structures of primary importance in structural proteomics, potentially closing the “sequence-structure gap” (Varadi et al., 2023), boosting in-silico drug screening and drug design, interactomics, functional investigation, evolutionary studies and more. Deep-learning methods will also be applicable to the reverse issue of predicting sequences from structures for protein design (Dauparas et al., 2022).
However, a still missing ingredient in these predictors is protein dynamics, since they output single structures rather than structural distributions and fail to predict folding pathways and conformational changes (Outeiral et al., 2022; Lane, 2023). Efforts are being devoted to predict alternative conformations (Stein and Mchaourab, 2022; Hatos et al., 2023; Vani et al., 2023). Expectations are legitimate, since protein dynamics and folding pathways, just like structures, are encoded by the protein sequence and subjected to selective pressure (Carvalho et al., 2015; Tang et al., 2022; Zhao et al., 2023). Increasing experimental characterization of protein dynamics will also help training more sophisticated deep-learning algorithms. AlphaFold2 achieves a ∼60% structural coverage of the human proteome, on a per-residue basis, with good confidence (Tunyasuvunakool et al., 2021). The portion that still lacks structural models largely overlaps with the predicted disordered proteome, to the point that the AlphaFold2 confidence score performs as an excellent disorder predictor, compared with state-of-the-art tools (Tunyasuvunakool et al., 2021; Guo et al., 2022; Ma et al., 2023). The challenge is shifting from predicting disorder to modeling disordered ensembles. The combination with MD simulations and NMR is promising and has been undertaken (Laurents, 2022; Nussinov et al., 2022; Agajanian et al., 2023; Ma et al., 2023). Deep-learning structural proteomics is a new important actor in integrative structural biology and mutual exchange with complementary approaches will be extremely fruitful (Figure 1).
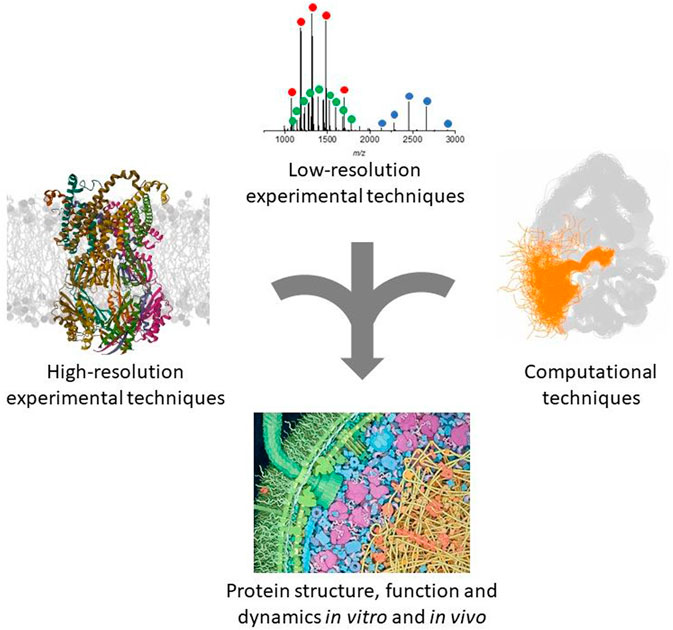
FIGURE 1. Schematic representation of the complementary approaches discussed in this review. The ribbon protein structure was generated from the PDB entry 8DDJ. The native MS spectrum was obtained in our laboratory as described (Halabelian et al., 2014). The other panels were adapted with permission from (Ahuja et al., 2019) and (Goodsell, 2012).
Future grand challenges
Integrative structural biology began approximately a decade ago (Ward et al., 2013) and impressive progress is continually made, interpreting the role of conformational entropy and structural dynamics in biological activity. The major challenge is modeling conformational ensembles and their changes during biological processes, as well as making such investigation accessible in vivo. Another major challenge is high-throughput application of biophysical methods to reach the omic scale and integrated omic sciences. Structural and functional proteomics will then be mature to support systems biology and precision medicine.
Author contributions
The author confirms being the sole contributor of this work and has approved it for publication.
Conflict of interest
The author RG declared that she was an editorial board member of Frontiers, at the time of submission. This had no impact on the peer review process and the final decision.
Publisher’s note
All claims expressed in this article are solely those of the authors and do not necessarily represent those of their affiliated organizations, or those of the publisher, the editors and the reviewers. Any product that may be evaluated in this article, or claim that may be made by its manufacturer, is not guaranteed or endorsed by the publisher.
References
Agajanian, S., Alshahrani, M., Bai, F., Tao, P., and Verkhivker, G. M. (2023). Exploring and learning the universe of protein allostery using artificial intelligence augmented biophysical and computational approaches. J. Chem. Inf. Model. 63 (5), 1413–1428. doi:10.1021/acs.jcim.2c01634
Ahmad, K., Rizzi, A., Capelli, R., Mandelli, D., Lyu, W., and Carloni, P. (2022). Enhanced-sampling simulations for the estimation of ligand binding kinetics: Current status and perspective. Front. Mol. Biosci. 9, 899805. doi:10.3389/fmolb.2022.899805
Ahuja, L. G., Aoto, P. C., Kornev, A. P., Veglia, G., and Taylor, S. S. (2019). Dynamic allostery-based molecular workings of kinase:peptide complexes. Proc. Natl. Acad. Sci. 116 (30), 15052–15061. doi:10.1073/pnas.1900163116
Alegre-Cebollada, J. (2021). Protein nanomechanics in biological context. Biophys. Rev. 13 (4), 435–454. doi:10.1007/s12551-021-00822-9
AlQuraishi, M. (2021). Machine learning in protein structure prediction. Curr. Opin. Chem. Biol. 65, 1–8. doi:10.1016/j.cbpa.2021.04.005
Ando, T. (2022). Functional implications of dynamic structures of intrinsically disordered proteins revealed by high-speed AFM imaging. Biomolecules 12 (12), 1876. doi:10.3390/biom12121876
Ansari, N., Rizzi, V., Carloni, P., and Parrinello, M. (2021). Water-triggered, irreversible conformational change of SARS-CoV-2 main protease on passing from the solid state to aqueous solution. J. Am. Chem. Soc. 143 (33), 12930–12934. doi:10.1021/jacs.1c05301
Arcus, V. L., and Mulholland, A. J. (2020). Temperature, dynamics, and enzyme-catalyzed reaction rates. Annu. Rev. Biophysics 49 (1), 163–180. doi:10.1146/annurev-biophys-121219-081520
Ariga, T., Tomishige, M., and Mizuno, D. (2020). Experimental and theoretical energetics of walking molecular motors under fluctuating environments. Biophys. Rev. 12 (2), 503–510. doi:10.1007/s12551-020-00684-7
Baek, M., DiMaio, F., Anishchenko, I., Dauparas, J., Ovchinnikov, S., Lee, G. R., et al. (2021). Accurate prediction of protein structures and interactions using a three-track neural network. Science 373 (6557), 871–876. doi:10.1126/science.abj8754
Banerjee, S., Chakraborty, S., Sreepada, A., Banerji, D., Goyal, S., Khurana, Y., et al. (2021). Cutting-edge single-molecule technologies unveil new mechanics in cellular biochemistry. Annu. Rev. Biophysics 50 (1), 419–445. doi:10.1146/annurev-biophys-090420-083836
Baumeister, W. (2022). Cryo-electron tomography: The power of seeing the whole picture. Biochem. Biophysical Res. Commun. 633, 26–28. doi:10.1016/j.bbrc.2022.08.078
Bekker, G.-J., and Kamiya, N. (2022). Advancing the field of computational drug design using multicanonical molecular dynamics-based dynamic docking. Biophys. Rev. 14 (6), 1349–1358. doi:10.1007/s12551-022-01010-z
Bhat, S., and Blunck, R. (2022). Characterising ion channel structure and dynamics using fluorescence spectroscopy techniques. Biochem. Soc. Trans. 50 (5), 1427–1445. doi:10.1042/BST20220605
Bhatia, H., Aydin, F., Carpenter, T. S., Lightstone, F. C., Bremer, P. T., Ingólfsson, H. I., et al. (2023). The confluence of machine learning and multiscale simulations. Curr. Opin. Struct. Biol. 80, 102569. doi:10.1016/j.sbi.2023.102569
Biasini, E., and Faccioli, P. (2023). Functional, pathogenic, and pharmacological roles of protein folding intermediates. Proteins [Preprint]. doi:10.1002/prot.26479
Bolnykh, V., Rossetti, G., Rothlisberger, U., and Carloni, P. (2021). Expanding the boundaries of ligand–target modeling by exascale calculations. WIREs Comput. Mol. Sci. 11 (4), e1535. doi:10.1002/wcms.1535
Bonaccorsi, M., Le Marchand, T., and Pintacuda, G. (2021). Protein structural dynamics by magic-angle spinning NMR. Curr. Opin. Struct. Biol. 70, 34–43.doi:10.1016/j.sbi.2021.02.008
Borkotoky, S., Dey, D., Hazarika, Z., Joshi, A., and Tripathi, K. (2022). Unravelling viral dynamics through molecular dynamics simulations - a brief overview. Biophys. Chem. 291, 106908. doi:10.1016/j.bpc.2022.106908
Bunzel, H. A., Anderson, J. L. R., Hilvert, D., Arcus, V. L., van der Kamp, M. W., and Mulholland, A. J. (2021). Evolution of dynamical networks enhances catalysis in a designer enzyme. Nat. Chem. 13 (10), 1017–1022. doi:10.1038/s41557-021-00763-6
Buzón, P., Maity, S., Christodoulis, P., Wiertsema, M. J., Dunkelbarger, S., Kim, C., et al. (2021). Virus self-assembly proceeds through contact-rich energy minima. Sci. Adv. 7 (45), eabg0811. doi:10.1126/sciadv.abg0811
Callaway, E. (2022). AlphaFold’s new rival? Meta AI predicts shape of 600 million proteins. Nature 611 (7935), 211–212. doi:10.1038/d41586-022-03539-1
Camacho-Zarco, A. R., Schnapka, V., Guseva, S., Abyzov, A., Adamski, W., Milles, S., et al. (2022). NMR provides unique insight into the functional dynamics and interactions of intrinsically disordered proteins. Chem. Rev. 122 (10), 9331–9356. doi:10.1021/acs.chemrev.1c01023
Capelli, R., Bochicchio, A., Piccini, G., Casasnovas, R., Carloni, P., and Parrinello, M. (2019). Chasing the full free energy landscape of neuroreceptor/ligand unbinding by metadynamics simulations. J. Chem. Theory Comput. 15 (5), 3354–3361. doi:10.1021/acs.jctc.9b00118
Carey, J. (2022). Affinity, specificity, and cooperativity of DNA binding by bacterial gene regulatory proteins. Int. J. Mol. Sci. 23 (1), 562. doi:10.3390/ijms23010562
Carvalho, H. F., Roque, A. C. A., Iranzo, O., and Branco, R. J. F. (2015). Comparison of the internal dynamics of metalloproteases provides new insights on their function and evolution. PloS One 10 (9), e0138118. doi:10.1371/journal.pone.0138118
Chakrabarti, P., and Chakravarty, D. (2022). Intrinsically disordered proteins/regions and insight into their biomolecular interactions. Biophys. Chem. 283, 106769. doi:10.1016/j.bpc.2022.106769
Choudhary, S., Lopus, M., and Hosur, R. V. (2022). Targeting disorders in unstructured and structured proteins in various diseases. Biophys. Chem. 281, 106742. doi:10.1016/j.bpc.2021.106742
Christofi, E., and Barran, P. (2023). Ion mobility mass spectrometry (IM-MS) for structural biology: Insights gained by measuring mass, charge, and collision cross section. Chem. Rev. 123 (6), 2902–2949. doi:10.1021/acs.chemrev.2c00600
Chu, W.-T., Yan, Z., Chu, X., Zheng, X., Liu, Z., Xu, L., et al. (2021). Physics of biomolecular recognition and conformational dynamics. Rep. Prog. Phys. 84 (12), 126601. doi:10.1088/1361-6633/ac3800
Cornwell, O., and Ault, J. R. (2022). Fast photochemical oxidation of proteins coupled with mass spectrometry. Biochimica Biophysica Acta (BBA) - Proteins Proteomics 1870 (9), 140829. doi:10.1016/j.bbapap.2022.140829
Coskuner-Weber, O., and Caglayan, S. I. (2021). Secondary structure dependence on simulation techniques and force field parameters: From disordered to ordered proteins. Biophys. Rev. 13 (6), 1173–1178. doi:10.1007/s12551-021-00850-5
Da Vela, S., and Svergun, D. I. (2020). Methods, development and applications of small-angle X-ray scattering to characterize biological macromolecules in solution. Curr. Res. Struct. Biol. 2, 164–170. doi:10.1016/j.crstbi.2020.08.004
Dai, X., Fu, W., Chi, H., Mesias, V. S. D., Zhu, H., Leung, C. W., et al. (2021). ‘Optical tweezers-controlled hotspot for sensitive and reproducible surface-enhanced Raman spectroscopy characterization of native protein structures’, Nat. Commun., 12 (1), p. 1292.doi:10.1038/s41467-021-21543-3
Dauparas, J., Anishchenko, I., Bennett, N., Bai, H., Ragotte, R. J., Milles, L. F., et al. (2022). Robust deep learning-based protein sequence design using ProteinMPNN. Science 378 (6615), 49–56. doi:10.1126/science.add2187
Dyson, H. J., and Wright, P. E. (2021). NMR illuminates intrinsic disorder. Curr. Opin. Struct. Biol. 70, 44–52. doi:10.1016/j.sbi.2021.03.015
Evans, R., Ramisetty, S., Kulkarni, P., and Weninger, K. (2023). Illuminating intrinsically disordered proteins with integrative structural biology. Biomolecules 13 (1), 124. doi:10.3390/biom13010124
Fürst, M. J., Fiorentini, F., and Fraaije, M. W. (2019). Beyond active site residues: Overall structural dynamics control catalysis in flavin-containing and heme-containing monooxygenases. Curr. Opin. Struct. Biol. 59, 29–37. doi:10.1016/j.sbi.2019.01.019
Goodsell, D. S. (2012). Putting proteins in context. BioEssays 34 (9), 718–720. doi:10.1002/bies.201200072
Guaita, M., Watters, S. C., and Loerch, S. (2022). Recent advances and current trends in cryo-electron microscopy. Curr. Opin. Struct. Biol. 77, 102484. doi:10.1016/j.sbi.2022.102484
Guin, D., and Gruebele, M. (2019). Weak chemical interactions that drive protein evolution: Crowding, sticking, and quinary structure in folding and function. Chem. Rev. 119 (18), 10691–10717. doi:10.1021/acs.chemrev.8b00753
Günsel, U., and Hagn, F. (2022). Lipid nanodiscs for high-resolution NMR studies of membrane proteins. Chem. Rev. 122 (10), 9395–9421. doi:10.1021/acs.chemrev.1c00702
Guo, H.-B., Perminov, A., Bekele, S., Kedziora, G., Farajollahi, S., Varaljay, V., et al. (2022). AlphaFold2 models indicate that protein sequence determines both structure and dynamics. Sci. Rep. 12 (1), 10696. doi:10.1038/s41598-022-14382-9
Halabelian, L., Ricagno, S., Giorgetti, S., Santambrogio, C., Barbiroli, A., Pellegrino, S., et al. (2014). Class I major histocompatibility complex, the trojan horse for secretion of amyloidogenic β2-microglobulin. J. Biol. Chem. 289 (6), 3318–3327. doi:10.1074/jbc.M113.524157
Hardenberg, M., Horvath, A., Ambrus, V., Fuxreiter, M., and Vendruscolo, M. (2020). Widespread occurrence of the droplet state of proteins in the human proteome. Proc. Natl. Acad. Sci. 117 (52), 33254–33262. doi:10.1073/pnas.2007670117
Harish, B., Swapna, G., Kornhaber, G. J., Montelione, G. T., and Carey, J. (2017). Multiple helical conformations of the helix-turn-helix region revealed by NOE-restrained MD simulations of tryptophan aporepressor, TrpR. Proteins 85 (4), 731–740. doi:10.1002/prot.25252
Hatos, A., Teixeira, J. M., Barrera-Vilarmau, S., Horvath, A., Tosatto, S. C. E., Vendruscolo, M., et al. (2023). FuzPred: a web server for the sequence-based prediction of the context-dependent binding modes of proteins. Nucleic Acids Res. [Preprint]. doi:10.1093/nar/gkad214
Haubrich, K., Spiteri, V. A., Farnaby, W., Sobott, F., and Ciulli, A. (2023). Breaking free from the crystal lattice: Structural biology in solution to study protein degraders. Curr. Opin. Struct. Biol. 79, 102534. doi:10.1016/j.sbi.2023.102534
He, Y., Lu, M., Cao, J., and Lu, H. P. (2012). Manipulating protein conformations by single-molecule AFM-FRET nanoscopy. ACS Nano 6 (2), 1221–1229. doi:10.1021/nn2038669
Hendriks, K., Öster, C., and Lange, A. (2021). Structural plasticity of the selectivity filter in cation channels. Front. Physiology 12, 792958. doi:10.3389/fphys.2021.792958
Hénin, J. (2022). Enhanced sampling methods for molecular dynamics simulations. Living J. Comput. Mol. Sci. 4 (1), 1583. doi:10.33011/livecoms.4.1.1583
Hou, W., Xu, D., Wang, L., Chen, Y., Chen, Z., Zhou, C., et al. (2022). Plastic structures for diverse substrates: A revisit of human abc transporters. Proteins Struct. Funct. Bioinforma. 90 (10), 1749–1765. doi:10.1002/prot.26406
Jeon, J., Thurber, K. R., Ghirlando, R., Yau, W. M., and Tycko, R. (2019). Application of millisecond time-resolved solid state NMR to the kinetics and mechanism of melittin self-assembly. Proc. Natl. Acad. Sci. 116 (34), 16717–16722. doi:10.1073/pnas.1908006116
Jukic, N., Perrino, A. P., Redondo-Morata, L., and Scheuring, S. (2023). Structure and dynamics of ESCRT-III membrane remodeling proteins by high-speed atomic force microscopy. J. Biol. Chem. 299 (4), 104575. doi:10.1016/j.jbc.2023.104575
Jumper, J., Evans, R., Pritzel, A., Green, T., Figurnov, M., Ronneberger, O., et al. (2021). Highly accurate protein structure prediction with AlphaFold. Nature 596 (7873), 583–589. doi:10.1038/s41586-021-03819-2
Kadek, A., Lorenzen, K., and Uetrecht, C. (2021). In a flash of light: X-Ray free electron lasers meet native mass spectrometry. Drug Discov. Today Technol. 39, 89–99. doi:10.1016/j.ddtec.2021.07.001
Kaltashov, I. A., Ivanov, D. G., and Yang, Y. (2022). Mass spectrometry-based methods to characterize highly heterogeneous biopharmaceuticals, vaccines, and nonbiological complex drugs at the intact-mass level. Mass Spectrom. Rev., e21829. doi:10.1002/mas.21829
Kar, R. K. (2023). Benefits of hybrid QM/MM over traditional classical mechanics in pharmaceutical systems. Drug Discov. Today 28 (1), 103374. doi:10.1016/j.drudis.2022.103374
Klebl, D. P., White, H. D., Sobott, F., and Muench, S. P. (2021). On-grid and in-flow mixing for time-resolved cryo-EM. Acta Crystallogr. Sect. D. Struct. Biol. 77 (10), 1233–1240. doi:10.1107/S2059798321008810
Kragelj, J., Dumarieh, R., Xiao, Y., and Frederick, K. K. (2023). Conformational ensembles explain NMR spectra of frozen intrinsically disordered proteins. Protein Sci. , 32 (5), e4628.Conformational ensembles explain NMR
Kwon, D. (2021). The secret lives of cells — As never seen before. Nature 598 (7882), 558–560. doi:10.1038/d41586-021-02904-w
Lane, T. J. (2023). Protein structure prediction has reached the single-structure frontier. Nat. Methods 20 (2), 170–173. doi:10.1038/s41592-022-01760-4
Laurents, D. V. (2022). AlphaFold 2 and NMR spectroscopy: Partners to understand protein structure, dynamics and function. Front. Mol. Biosci. 9, 906437. doi:10.3389/fmolb.2022.906437
Lento, C., and Wilson, D. J. (2022). Subsecond time-resolved mass spectrometry in dynamic structural biology. Chem. Rev. 122 (8), 7624–7646. doi:10.1021/acs.chemrev.1c00222
Lin, Z., Akin, H., Rao, R., Hie, B., Zhu, Z., Lu, W., et al. (2023). Evolutionary-scale prediction of atomic-level protein structure with a language model. Science 379 (6637), 1123–1130. doi:10.1126/science.ade2574
Liu, H., and Lee, W. (2019). The XFEL protein crystallography: Developments and perspectives. Int. J. Mol. Sci. 20 (14), 3421. doi:10.3390/ijms20143421
Lostao, A., Lim, K., Pallarés, M. C., Ptak, A., and Marcuello, C. (2023). Recent advances in sensing the inter-biomolecular interactions at the nanoscale – a comprehensive review of AFM-based force spectroscopy. Int. J. Biol. Macromol. 238, 124089. doi:10.1016/j.ijbiomac.2023.124089
Luchinat, E., and Banci, L. (2022). In-cell NMR: From target structure and dynamics to drug screening. Curr. Opin. Struct. Biol. 74, 102374. doi:10.1016/j.sbi.2022.102374
Lycus, P., Einsle, O., and Zhang, L. (2023). Structural biology of proteins involved in nitrogen cycling. Curr. Opin. Chem. Biol. 74, 102278. doi:10.1016/j.cbpa.2023.102278
Ma, P., Li, D., and Brüschweiler, R. (2023). Predicting protein flexibility with AlphaFold. Proteins Struct. Funct. Bioinforma. 91, 847–855. doi:10.1002/prot.26471
Madan, L. K., Welsh, C. L., Kornev, A. P., and Taylor, S. S. (2023). The “violin model”: Looking at community networks for dynamic allostery. J. Chem. Phys. 158 (8), 081001. doi:10.1063/5.0138175
Mäeots, M.-E., and Enchev, R. I. (2022). Structural dynamics: Review of time-resolved cryo-EM. Acta Crystallogr. Sect. D. Struct. Biol. 78 (8), 927–935. doi:10.1107/S2059798322006155
Majumdar, A., and Mukhopadhyay, S. (2022). Excitation energy migration to study protein oligomerization and amyloid formation. Biophys. Chem. 281, 106719. doi:10.1016/j.bpc.2021.106719
Malinovska, L., Cappelletti, V., Kohler, D., Piazza, I., Tsai, T. H., Pepelnjak, M., et al. (2023). Proteome-wide structural changes measured with limited proteolysis-mass spectrometry: An advanced protocol for high-throughput applications. Nat. Protoc. 18 (3), 659–682. doi:10.1038/s41596-022-00771-x
Malla, T. N., and Schmidt, M. (2022). Transient state measurements on proteins by time-resolved crystallography. Curr. Opin. Struct. Biol. 74, 102376. doi:10.1016/j.sbi.2022.102376
Marciano, S., Dey, D., Listov, D., Fleishman, S. J., Sonn-Segev, A., Mertens, H., et al. (2022). Protein quaternary structures in solution are a mixture of multiple forms. Chem. Sci. 13 (39), 11680–11695. doi:10.1039/D2SC02794A
Margulies, D. H., Taylor, D. K., Jiang, J., Boyd, L. F., Ahmad, J., Mage, M. G., et al. (2022). Chaperones and catalysts: How antigen presentation pathways cope with biological necessity. Front. Immunol. 13, 859782. doi:10.3389/fimmu.2022.859782
Masson, G. R., Burke, J. E., Ahn, N. G., Anand, G. S., Borchers, C., Brier, S., et al. (2019). Recommendations for performing, interpreting and reporting hydrogen deuterium exchange mass spectrometry (HDX-MS) experiments. Nat. Methods 16 (7), 595–602. doi:10.1038/s41592-019-0459-y
Mateus, A., Kurzawa, N., Becher, I., Sridharan, S., Helm, D., Stein, F., et al. (2020). Thermal proteome profiling for interrogating protein interactions. Mol. Syst. Biol. 16 (3), e9232. doi:10.15252/msb.20199232
Melo, M. C. R., and Bernardi, R. C. (2023). Fostering discoveries in the era of exascale computing: How the next generation of supercomputers empowers computational and experimental biophysics alike. Biophysical J. doi:10.1016/j.bpj.2023.01.042
Moebel, E., and Kervrann, C. (2022). Towards unsupervised classification of macromolecular complexes in cryo electron tomography: Challenges and opportunities. Comput. Methods Programs Biomed. 225, 107017. doi:10.1016/j.cmpb.2022.107017
Molina, D. M., Jafari, R., Ignatushchenko, M., Seki, T., Larsson, E. A., Dan, C., et al. (2013). Monitoring drug target engagement in cells and tissues using the cellular thermal shift assay. Science 341 (6141), 84–87. doi:10.1126/science.1233606
Müller, D. J., Dumitru, A. C., Lo Giudice, C., Gaub, H. E., Hinterdorfer, P., Hummer, G., et al. (2021). Atomic force microscopy-based force spectroscopy and multiparametric imaging of biomolecular and cellular systems. Chem. Rev. 121 (19), 11701–11725. doi:10.1021/acs.chemrev.0c00617
Nishiyama, Y., Hou, G., Agarwal, V., Su, Y., and Ramamoorthy, A. (2023). Ultrafast magic angle spinning solid-state NMR spectroscopy: Advances in methodology and applications. Chem. Rev. 123 (3), 918–988. doi:10.1021/acs.chemrev.2c00197
Nussinov, R., Zhang, M., Liu, Y., and Jang, H. (2022). AlphaFold, artificial intelligence (AI), and allostery. J. Phys. Chem. B 126 (34), 6372–6383. doi:10.1021/acs.jpcb.2c04346
Oliveira Bortot, L., Bashardanesh, Z., and van der Spoel, D. (2020). Making soup: Preparing and validating models of the bacterial cytoplasm for molecular simulation. J. Chem. Inf. Model. 60 (1), 322–331. doi:10.1021/acs.jcim.9b00971
Outeiral, C., Nissley, D. A., and Deane, C. M. (2022). Current structure predictors are not learning the physics of protein folding. Bioinformatics 38 (7), 1881–1887. doi:10.1093/bioinformatics/btab881
Paissoni, C., and Camilloni, C. (2021). How to determine accurate conformational ensembles by metadynamics metainference: A chignolin study case. Front. Mol. Biosci. 8, 694130. doi:10.3389/fmolb.2021.694130
Patil, A. (2022). Enrichment patterns of intrinsic disorder in proteins. Biophys. Rev. 14 (6), 1487–1493. doi:10.1007/s12551-022-01016-7
Piana, S., Robustelli, P., Tan, D., Chen, S., and Shaw, D. E. (2020). Development of a force field for the simulation of single-chain proteins and protein–protein complexes. J. Chem. Theory Comput. 16 (4), 2494–2507. doi:10.1021/acs.jctc.9b00251
Piersimoni, L., Kastritis, P. L., Arlt, C., and Sinz, A. (2022). Cross-linking mass spectrometry for investigating protein conformations and protein–protein Interactions─A method for all seasons. Chem. Rev. 122 (8), 7500–7531. doi:10.1021/acs.chemrev.1c00786
Puthenveetil, R., Christenson, E. T., and Vinogradova, O. (2022). New horizons in structural biology of membrane proteins: Experimental evaluation of the role of conformational dynamics and intrinsic flexibility. Membranes 12 (2), 227. doi:10.3390/membranes12020227
Rabbani, G., Ahmad, E., Ahmad, A., and Khan, R. H. (2023). Structural features, temperature adaptation and industrial applications of microbial lipases from psychrophilic, mesophilic and thermophilic origins. Int. J. Biol. Macromol. 225, 822–839. doi:10.1016/j.ijbiomac.2022.11.146
Reid, D. J., Thibert, S., and Zhou, M. (2023). Dissecting the structural heterogeneity of proteins by native mass spectrometry. Protein Sci. 32 (4), e4612. doi:10.1002/pro.4612
Rizzuti, B. (2022). Molecular simulations of proteins: From simplified physical interactions to complex biological phenomena. Biochim Biophys Acta Proteins Proteom 1870 (3), 140757. doi:10.1016/j.bbapap.2022.140757
Robertson, M. J., and Skiniotis, G. (2022). Development of OPLS-AA/M parameters for simulations of G protein-coupled receptors and other membrane proteins. J. Chem. Theory Comput. 18 (7), 4482–4489. doi:10.1021/acs.jctc.2c00015
Robustelli, P., Ibanez-de-Opakua, A., Campbell-Bezat, C., Giordanetto, F., Becker, S., Zweckstetter, M., et al. (2022). Molecular basis of small-molecule binding to α-synuclein. J. Am. Chem. Soc. 144 (6), 2501–2510. doi:10.1021/jacs.1c07591
Ruggeri, F. S., Mannini, B., Schmid, R., Vendruscolo, M., and Knowles, T. P. J. (2020). Single molecule secondary structure determination of proteins through infrared absorption nanospectroscopy. Nat. Commun. 11 (1), 2945. doi:10.1038/s41467-020-16728-1
Sali, A. (2021). From integrative structural biology to cell biology. J. Biol. Chem. 296, 100743. doi:10.1016/j.jbc.2021.100743
Santambrogio, C., Ponzini, E., and Grandori, R. (2022). Native mass spectrometry for the investigation of protein structural (dis)order. Biochim Biophys Acta Proteins Proteom 1870 (10), 140828. doi:10.1016/j.bbapap.2022.140828
Selenko, P. (2019). Quo vadis biomolecular NMR spectroscopy? Int. J. Mol. Sci. 20 (6), 1278. doi:10.3390/ijms20061278
Singharoy, A., Maffeo, C., Delgado-Magnero, K. H., Swainsbury, D. J., Sener, M., Kleinekathöfer, U., et al. (2019). Atoms to phenotypes: Molecular design principles of cellular energy metabolism. Cell 179 (5), 1098–1111.e23. doi:10.1016/j.cell.2019.10.021
Sohraby, F., and Nunes-Alves, A. (2023). Advances in computational methods for ligand binding kinetics. Trends Biochem. Sci. 48, 437–449. doi:10.1016/j.tibs.2022.11.003
Stein, R. A., and Mchaourab, H. S. (2022). SPEACH_AF: Sampling protein ensembles and conformational heterogeneity with Alphafold2. PLoS Comput. Biol. 18 (8), e1010483. doi:10.1371/journal.pcbi.1010483
Stenström, O., Champion, C., Lehner, M., Bouvignies, G., Riniker, S., and Ferrage, F. (2022). How does it really move? Recent progress in the investigation of protein nanosecond dynamics by NMR and simulation. Curr. Opin. Struct. Biol. 77, 102459. doi:10.1016/j.sbi.2022.102459
Sun, X., Dyson, H. J., and Wright, P. E. (2023). Role of conformational dynamics in pathogenic protein aggregation. Curr. Opin. Chem. Biol. 73, 102280. doi:10.1016/j.cbpa.2023.102280
Tamara, S., den Boer, M. A., and Heck, A. J. R. (2022). High-Resolution Native Mass Spectrometry. Chem. Rev. 122 (8), 7269–7326. doi:10.1021/acs.chemrev.1c00212
Tang, Q.-Y., Ren, W., Wang, J., and Kaneko, K. (2022). The statistical trends of protein evolution: A lesson from AlphaFold database. Mol. Biol. Evol. 39 (10), msac197. doi:10.1093/molbev/msac197
Theillet, F.-X., and Luchinat, E. (2022). In-cell NMR: Why and how? Prog. Nucl. Magnetic Reson. Spectrosc. 133, 1–112. doi:10.1016/j.pnmrs.2022.04.002
Toader, B., Sigworth, F. J., and Lederman, R. R. (2023). Methods for cryo-EM single particle reconstruction of macromolecules having continuous heterogeneity. J. Mol. Biol. 435, 168020. doi:10.1016/j.jmb.2023.168020
Toth-Petroczy, A., Palmedo, P., Ingraham, J., Hopf, T. A., Berger, B., Sander, C., et al. (2016). Structured states of disordered proteins from genomic sequences. Cell 167 (1), 158–170.e12. doi:10.1016/j.cell.2016.09.010
Tsoi, P. S., Quan, M. D., Ferreon, J. C., and Ferreon, A. C. M. (2023). Aggregation of disordered proteins associated with neurodegeneration. Int. J. Mol. Sci. 24 (4), 3380. doi:10.3390/ijms24043380
Tunyasuvunakool, K., Adler, J., Wu, Z., Green, T., Zielinski, M., Žídek, A., et al. (2021). Highly accurate protein structure prediction for the human proteome. Nature 596 (7873), 590–596. doi:10.1038/s41586-021-03828-1
Vani, B. P., et al. (2023). AlphaFold2-RAVE: From sequence to Boltzmann ranking. J. Chem. Theory Comput.
Varadi, M., Bordin, N., Orengo, C., and Velankar, S. (2023). The opportunities and challenges posed by the new generation of deep learning-based protein structure predictors. Curr. Opin. Struct. Biol. 79, 102543. [Preprint]. doi:10.1016/j.sbi.2023.102543
Vennelakanti, V., Nazemi, A., Mehmood, R., Steeves, A. H., and Kulik, H. J. (2022). Harder, better, faster, stronger: Large-scale QM and QM/MM for predictive modeling in enzymes and proteins. Curr. Opin. Struct. Biol. 72, 9–17. doi:10.1016/j.sbi.2021.07.004
Ward, A. B., Sali, A., and Wilson, I. A. (2013). Integrative structural biology. Science 339 (6122), 913–915. doi:10.1126/science.1228565
Weik, M., and Domratcheva, T. (2022). Insight into the structural dynamics of light sensitive proteins from time-resolved crystallography and quantum chemical calculations. Curr. Opin. Struct. Biol. 77, 102496. doi:10.1016/j.sbi.2022.102496
Xue, K., Movellan, K. T., Zhang, X. C., Najbauer, E. E., Forster, M. C., Becker, S., et al. (2021). Towards a native environment: Structure and function of membrane proteins in lipid bilayers by NMR. Chem. Sci. 12 (43), 14332–14342. doi:10.1039/D1SC02813H
Yu, I., Mori, T., Ando, T., Harada, R., Jung, J., Sugita, Y., et al. (2016). Biomolecular interactions modulate macromolecular structure and dynamics in atomistic model of a bacterial cytoplasm. eLife 5, e19274. doi:10.7554/eLife.19274
Yu, Y., and Yoshimura, S. H. (2021). Investigating the morphological dynamics of the plasma membrane by high-speed atomic force microscopy. J. Cell Sci. 134 (17), jcs243584. doi:10.1242/jcs.243584
Keywords: crystallography, nuclear magnetic resonance, cryo-electron microscopy, cryo-electron tomography, mass spectrometry, atomic force microscopy, molecular dynamics simulations, deep-learning technology
Citation: Grandori R (2023) Protein structure and dynamics in the era of integrative structural biology. Front. Biophys. 1:1219843. doi: 10.3389/frbis.2023.1219843
Received: 09 May 2023; Accepted: 19 June 2023;
Published: 17 July 2023.
Edited by:
David Alsteens, Université Catholique de Louvain, BelgiumReviewed by:
Yuichi Togashi, Ritsumeikan University, JapanJannette Carey, Princeton University, United States
Albert Guskov, University of Groningen, Netherlands
Copyright © 2023 Grandori. This is an open-access article distributed under the terms of the Creative Commons Attribution License (CC BY). The use, distribution or reproduction in other forums is permitted, provided the original author(s) and the copyright owner(s) are credited and that the original publication in this journal is cited, in accordance with accepted academic practice. No use, distribution or reproduction is permitted which does not comply with these terms.
*Correspondence: Rita Grandori, rita.grandori@unimib.it