- 1The Faculty of Engineering and the Institute of Nanotechnology and Advanced Materials, Bar Ilan University, Ramat Gan, Israel
- 2Center for Optical Technologies, Aalen University, Aalen, Germany
- 3Centre for Cellular Imaging, Sahlgrenska Academy, University of Gothenburg, Göteborg, Sweden
- 4Memorial Sloan Kettering Cancer Center, Molecular Pharmacology, New York, NY, United States
- 5Department of Dermatology Medical University of Vienna, Vienna, Austria
- 6Christian Doppler Laboratory for Skin Multimodal Imaging of Aging and Senescence - SKINMAGINE, Vienna, Austria
- 7Department of Informatics, University of Bergen, Bergen, Norway
- 8Department of Biomedical Imaging and Image-guided Therapy, Medical University of Vienna, Vienna, Austria
- 9Medical Imaging Cluster (MIC), Medical University of Vienna, Vienna, Austria
- 10Division of Anatomy, CMI, Medical University of Vienna, Vienna, Austria
- 11Centre for Image Analysis, Department of Information Technology, Uppsala University, Uppsala, Sweden
- 12Werner Siemens Imaging Center, Department of Preclinical Imaging and Radiopharmacy, Eberhard-Karls University Tuebingen, Tuebingen, Germany
- 13Cluster of Excellence iFIT (EXC 2180) “Image Guided and Functionally Instructed Tumor Therapies”, Tuebingen, Germany
- 14Institute of Chemical Technologies and Analytics, Wien, Austria
- 15Weizmann Institute of Science, Rehovot, Israel
- 16Nantes Université, CHU Nantes, CNRS, Inserm, BioCore, US16, SFR Bonamy Nantes, France
- 17i3S, Porto, Portugal
- 18NanoImaging Core facility, Institute Pasteur, Paris, France
- 19School of Biochemistry, University of Bristol, Bristol, United Kingdom
Correlated Multimodal Imaging (CMI) gathers information about the same specimen with two or more modalities that–combined–create a composite and complementary view of the sample (including insights into structure, function, dynamics and molecular composition). CMI allows one to reach beyond what is possible with a single modality and describe biomedical processes within their overall spatio-temporal context and gain a mechanistic understanding of cells, tissues, and organisms in health and disease by untangling their molecular mechanisms within their native environment. The field of CMI has grown substantially over the last decade and previously unanswerable biological questions have been solved by applying novel CMI workflows. To disseminate these workflows and comprehensively share the scattered knowledge present within the CMI community, an initiative was started to bring together imaging, image analysis, and biomedical scientists and work towards an open community that promotes and disseminates the field of CMI. This community project was funded for the last 4 years by an EU COST Action called COMULIS (COrrelated MUltimodal imaging in the LIfe Sciences). In this review we share some of the showcases and lessons learnt from the action. We also briefly look ahead at how we anticipate building on this initial initiative.
1 Introduction
The relentless pace of innovation in biomedical imaging has provided modern researchers with an unprecedented number of techniques and tools to choose from when analyzing their sample. New imaging methods have enabled the characterization of molecular mechanisms and biophysical properties, both in- and ex vivo, with a resolution of a few Angstroms (using cryogenic transmission electron microscopy) and penetration depths in the range of a centimeters even for light microscopy techniques (using light sheets or multiphoton excitations). However, no single imaging modality alone can decipher the inner working of a cell or even entire organisms. To gain such insights, imaging technologies need to be combined and, importantly, correlated: Correlated Multimodal Imaging (CMI) creates a composite and zoomable view of exactly the same specimen and region of interest by (sequentially) correlating two or more modalities to tackle biomedical research questions holistically and across multiple scales. CMI integrates the best features of the combined techniques and overcomes limitations faced when applying single modalities independently. However, it requires the design of complex pipelines, including sample preparation, probes, software and hardware relocation procedures, and visualization tools. CMI is specifically moving towards the integration of in vivo with subsequent ex vivo imaging modalities to bridge the gap between preclinical and biological imaging. Furthermore, access to CMI equipment is still limited in different parts of the world and user communities. To address these challenges and to build novel CMI pipelines which include cutting-edge technologies, a strong collaborative interdisciplinary network is essential. COMULIS’ main objective was to promote Correlated Multimodal Imaging (CMI) in biological and preclinical research. To achieve this inherently interdisciplinary goal, COMULIS successfully established a pan-European collaborative network of scientists across disciplines to foster and market CMI as a versatile tool in biomedical research. The work plan and implementation of COMULIS was divided in five Working Groups (WGs) that were organized accordingly:
• Establishing standards and optimizing CMI approaches in biological and preclinical settings with a focus on Correlative Light and Electron Microscopy (CLEM) and Preclinical Hybrid Imaging (PHI) as the best-established technologies in each area, and using these implementations as demonstrator technologies to showcase the wider benefits of CMI to the life science community (WG1, CLEM and WG 2, PHI);
• Triggering novel CMI implementations of modalities that have not been correlated before, thereby bridging the gap between biological and preclinical imaging (WG3);
• Involving all relevant scientific disciplines, and in the first-place software developers, for input on image data handling and analysis (WG4);
• Fostering recognition of CMI and promoting its benefits in life sciences via dissemination, training, and networking (WG5).
With this organization in place, COMULIS fueled urgently needed collaborations in the field of CMI and paved the way to become the premier transnational platform for knowledge and information exchange in CMI. Over the lifespan of COMULIS, the homepage has been visited more than 50 k times so far - and is indeed an important transnational platform to learn about multimodal imaging, related events and job posts:https://www.comulis.eu. In addition, COMULIS published a bioimaging compendium that highlights available imaging technologies, physical principles and biomedical applications with a focus on CMI and contributed 3 peer-reviewed reviews on the challenges, limitations, and standardization of CMI (Walter et al., 2020; Walter et al., 2021a; Walter et al., 2021b; Walter et al., 2021c; Keuenhof et al., 2021). COMULIS promoted and disseminated benefits of CMI through showcase pipelines and 3 conferences that were attended by over 500 people, and fostered its technological advancement and implementation as a versatile tool in biological and preclinical research through lab exchanges and 6 workshops (https://www.comulis.eu/schedule). The lab exchanges resulted in the publications of several showcase pipelines to tackle specific biomedical research questions, ranging an improved diagnostic of osteonecrosis or vascular lesions to novel dyes for correlative Super-Resolution microscopy or alignment of mass spectrometry and clinical imaging data (Váradi et al., 2019; Lucidi et al., 2020; Keuenhofer et al., 2021; Reier et al., 2021; Balluff et al., 2022). 2 additional examples of these novel pipeline showcases are highlighted below (tattoo removal and skin characterization). COMULIS has also triggered computational advancements and big data strategies to correlate and handle volumetric image data from different modalities (https://www.comulis.eu/correlation-software). Computer scientists included in the network have created a ontology for bioimage informatics (Ison et al., 2013; Kalaš et al., 2020; Lindblad et al., 2020) and novel approaches for automated image alignment (registration), segmentation of objects of interest, feature extraction, and visualization and exploration of complex, time-varying, multiscale, multimodal data volumes (Balluff et al., 2022; Cunha et al., 2023; Nešic et al., 2024). This was successfully achieved through an active dialogue between computer and life scientists and the full integration of computer scientists in the complete workflow (Walter et al., 2020).
COMULIS succeeded, through its activities and virtual alternatives during the COVID-19 pandemic, in raising awareness within the community of the relevance and possibilities, but also limitations of the available multimodal imaging approaches and software solutions (Walter et al., 2021a). In addition, it was able to highlight and set directions for further development within the dynamic and highly advanced imaging field. COMULIS revealed the so far not fully exploited potential of CMI towards a practical use in biomedical research and thereby combined biological and preclinical imaging–with synergetic benefits for medicine, including increased diagnostic accuracy (Reier et al., 2021). Holistic approaches help researchers to discover molecular interdependencies over a wide range of temporal and spatial scales and foster the study of cellular and molecular processes within their native context.
Apart from triggering the implementation of novel CMI approaches to tackle showcase projects, COMULIS worked towards optimizing and standardizing existing pipelines via the publication of reviews, databases and best practices. In particular, we aimed to facilitate data curation, as well as information seeking and identification of software useful for CMI approaches (Walter et al., 2021c). COMULIS hereby integrated all relevant stakeholders from academia and industry, instrument developers and users such as physicists, biologists, clinicians and computer scientists. Boosting communication across disciplines through dedicated communication channels and meeting formats has sped up advancements in CMI and triggered its recognition in biomedical research. Furthermore, COMULIS supported many PhD students and early career investigators (via Conference Grants) affiliated in Inclusiveness Targeted Countries (ITC) to attend high-level international conferences related to correlated multimodal imaging, giving them an opportunity to present and upgrade their scientific work, and also to establish new contacts for future collaborations. COMULIS specifically attracted and educated the next-generation of scientists in CMI approaches through training schools and short-term scientific missions (STSMs) and helped to guide the scientific mindset from isolated single technique approaches towards integrative approaches and mechanistic analysis of diseases and organisms. One of the examples from the STSMs was the development and integration of histology, still one of the cornerstones of clinical analysis, into a CLEM workflow (Sousa et al., 2021), whereas the other project aimed at understanding the sequence of molecular events that lead muscle precursor cells, called myoblasts, to fuse to form skeletal muscle development during myogenesis, and muscle regeneration in adults (Khan et al., 2023). This was also achieved by ‘democratizing’ CMI approaches through the organization of basic introductory courses that allowed attendees to transfer the skills learned during the course to their home setting and perform CMI even with limited instrument resources. Encouraging the use of CMI helped to expand the available spectrum of relevant methodologies in life sciences, transforming the study of biomedical processes by providing unprecedented information content. This was highlighted for WG-specific multimodal showcase projects, ranging from multi-center studies to tackling senescence.
2 Technological advances: Innovation, development, implementation, and dissemination
2.1 Correlated multimodal imaging
2.1.1 Correlative light and electron microscopy
There is still the conception within the larger research community that correlative microscopy is a technically very challenging technique that can only be carried out in specialized labs. Although this may be true for specific correlative workflows, there are a number of very powerful approaches that are within reach for almost any life science laboratory. This is especially true for the best established Correlative Multimodal Imaging approach, Correlative Light Electron Microscopy (CLEM). One of the major objectives of COMULIS was to democratize Correlative Multimodal Imaging. We believe this was achieved very successfully, for example, through the organization of a dedicated CLEM course at the Institut Gulbenkian in Portugal where the focus was on a “MacGyver” approach to CLEM (https://www.merriam-webster.com/words-at-play/what-does-macgyver-mean-slang-definition). Attendees were taught that any lab, which has access to a (fluorescence) light microscopy and a Transmission Electron Microscope (TEM), would be able to perform CLEM in their own setting. The course was built around one of the simplest but also most powerful CLEM approaches available, which was established in 2000. Roman Polishchuk and coworkers published a seminal paper (Polishchuk et al., 2000) using a special type of glass-bottom dishes. The coverslip contains an embossed finder pattern, which allows the exact same cell to be imaged under a light microscope and subsequently retraced in the electron microscope. Another part of the course focused on the data analysis of the sample. Whereas it is easy to say that the same cells match up in the two modalities, it requires dedicated software tools to map the exact same organelles on top of each other. This was a very good example in which working across different COMULIS working groups was essential, as it was the combined efforts of WG1 (CLEM) together with WG4, (Correlation software) that ensured the success of the course. After the course, the students were able to transfer the acquired skills learned during the course to their home setting and perform CLEM with limited instrument resources.
One of the major challenges and objectives of the COMULIS project was to bring together biomedical and (pre)clinical researchers. The best way to do this is by enabling such interactions through STSMs and other dedicated projects. Two showcase examples are highlighted below to present the benefits and power of CMI across scales, including and combining imaging techniques from microscopy to preclinical imaging.
2.1.2 Novel multimodal imaging pipelines
These novel CMI pipelines, that were set up to combine in and ex-vivo imaging approaches, aim at identifying, optimizing, triggering and evaluating (Walter et al., 2021a) sample preparation procedures that are compatible with various imaging modalities without compromising data quality, (Walter et al., 2021b) correlative probes and fiducial markers that can be visualized in different imaging technologies, (Keuenhof et al., 2021) hardware and software solutions to identify and correlate the same region after relocation between imaging platforms, (Walter et al., 2021c) software solutions to co-align data from different modalities, and (Walter et al., 2020) approaches for data handling and storage. Below, we highlight two showcase projects that succeeded in combining and correlating a variety of biomedical and (pre)clinical imaging techniques to gain complementary and holistic information about the specimen across scales. These two pipelines allow for identifying regions and volumes of interest (ROI/VOI) in vivo and subsequently zooming into these ROIs to visualize them at high (nanometer) resolution. The first showcase project studied biomarkers for tattoo removal, the second project studied the role of autophagy in the senescence of the skin.
I. A Multimodal Parameter Space for Tattoo Removals
The rising popularity of tattoos has coincided with a notable demand for safe tattoo removal procedures. According to a 2019 study (Boardman, 2019), approximately 17% of individuals who have tattoos experience some degree of regret. Reasons for tattoo removal vary, ranging from getting inked at a young age to concerns about career prospects, social dynamics, religious beliefs, or personal circumstances (British Medical Laser Association, 2023). Laser-based removal methods, such as intense pulsed light (IPL), are particularly favored due to their precision and relatively fewer side effects compared to alternatives like dermabrasion, surgical removal, or creams. To achieve holistic information on tattoos in the tissue context, we applied CMI and aimed at showcasing its benefits. It allows for generating a multidimensional data landscape on tattoos to establish ways to remove them. In the tattoo removal procedure, the deciding parameters, such as laser wavelength and fluence, are based on the depth of the tattoo ink, concentration, and color of the ink in the tattoo (Rudraiah et al., 2022a). By combining different optical spectroscopic and imaging modalities, a realistic profile of tattoos from the macroscopic to microscopic level can be understood. CMI will provide the necessary quantitative information that is crucial in a safe tattoo removal procedure and will further enable the development of detection and monitoring modalities for quantifying, tracing, and tracking the ink in the tattoo in vivo. It can be translated to technologies that assess therapeutic success for tattoo removals. Presently, effective tattoo removal is possible using lasers without causing damage to the surrounding skin only if prior knowledge about tattoo size, colors of the ink, and depth of the tattoo inks in the skin is available (Evgenakis et al., 2023; Zima et al., 2023). In light-tissue interactions, the optical properties of the tissues, such as absorption and scattering, need to be considered. The skin consists of endogenous chromophores such as water, melanin, hemoglobin, oxyhemoglobin, lipids, collagen, etc., and each has a unique absorption characteristic (Steven, 2013). It is crucial to choose the exact wavelength that targets the tattoo pigments. In this project at first, the characteristics of the ink such as absorption, chemical composition, and pigment size were studied using spectroscopic techniques such as a spectrophotometer, Fourier-transform infrared spectroscopy (FTIR), Raman spectroscopy, dynamic light scattering (DLS), electron microscopy, and mass spectrometry technique (Bocca et al., 2017). The techniques are listed in Table 1.
The complication arises when working on real skin since the properties of tissue vary from tissue to tissue, person to person, and from time to time (Cheong et al., 1990; Bashkatov et al., 2011) For that, tissue-mimicking optical phantoms were used to study the tattoos. The phantoms can be made solid or liquid (Sousa et al., 2021). Solid phantoms have a long shelf life, can be modulated to a realistic organ shape, have stable optical properties (absorption and scattering coefficients), and are easy to handle (Rudraiah et al., 2021). In Figure 1, a solid phantom with different pigment types and location is presented.
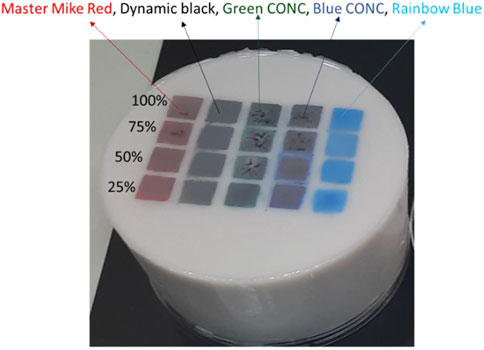
Figure 1. Tattooed skin tissue-mimicking optical silicone-Polydimethylsiloxane (PDMS) phantom. Thickness of the epidermis layer was 200 µm and optical properties: µs’ = 0.158 mm-1, and µa = 2.41 mm-1 at 650 nm. The tattoos were made at the junction between the dermis-epidermis layer using master mike red, dynamic black, green CONC, blue CONC, and rainbow blue with concentrations from 100%, 75%, 50%, and 25%.
A novel optical fiber-based diffusion reflection (DR) sensing technique (Rudraiah et al., 2022b) utilizes analytical models in the big clinical diagnosis picture (Rudraiah et al., 2022c). The DR intensity was received by scanning the detector fiber on the surface of the tattooed phantom. The data was plotted as a logarithm of the product between the collected intensity and distance vs distance at 650 nm (Figure 2) for the master mike red pigment with a concentration from 25%–100% (light to dark red lines). The tattooed 2L was compared with the non-tattooed 2L and 1L phantom (optical properties equal to the top layer of the 2L phantom). The tattooed 2L phantom with different ink concentrations exhibits two different slopes compared to the non-tattooed 2L phantom.
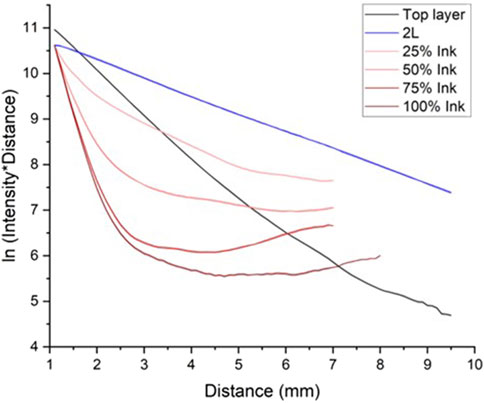
Figure 2. DR intensity profile from the tattooed phantoms with different pigment concentrations at 650 nm illumination. The top layer and 2L (black and blue lines) are without tattoo pigments. The red lines from lighter to darker are the increased pigment concentrations from 25% to 100%. The error bar indicates the standard deviations with respective shaded color thickness.
During the COMULIS showcase project the spectroscopic techniques were complemented by multimodal imaging techniques, such as diffuse reflectance technique, photoacoustic tomography (PAT) (Köksal et al., 2022), Raman spectroscopy (Moseman et al., 2024), FTIR spectroscopy (Bauer et al., 2019), Optical photothermal infrared (O-PTIR) spectroscopy (Darvin et al., 2018), multispectral Imaging (Kuzmina et al., 2022) electron microscopy (Lea and Pawlowski, 1987), and mass spectroscopy imaging (MALDI, LACIP, SIMS) (Holzlechner et al., 2018) to detect and confirm the tattoo ink location, pigment concentration, and type of tattoo ink in the skin mimicking tattooed phantoms. Table 2 provides an overview of the various techniques used to analyze tattoo ink properties, ranging from macroscopic imaging to high-resolution microscopic methods, each offering unique insights into ink location, concentration, and pigment types.
II. The Skin/Epidermal Equivalent Project
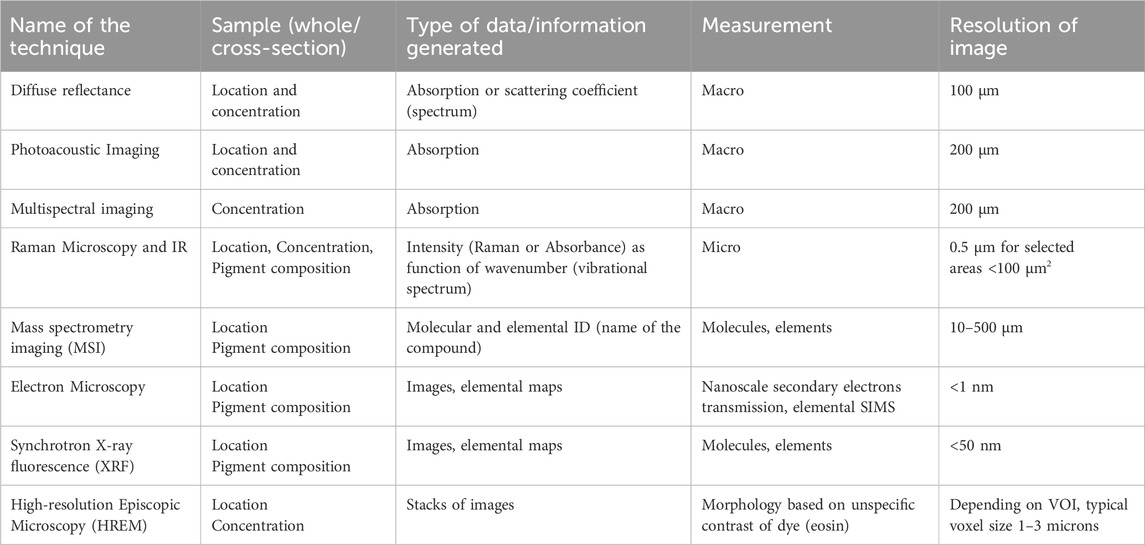
Table 2. A summary of the techniques that were applied to detect the tattoo ink location, concentration, and pigment types from the tattooed samples.
The 2nd project focused on a round-robin study to assess which modalities can be combined to study tissue of the size of a biopsy of approximately 6 mm in diameter. We provided the contributing investigators with the identical piece of tissue in sequential order (compare Figure 3). Figure 3 depicts one of the eight multi-station workflows, which was set up to combine light microscopy and mass spectrometry imaging to detect lipids within their cellular context after UVB irradiation. Alternatively, investigators were provided with parallel equivalent and highly comparable samples generated from the same donor material. We had not only the goal to gain experience on the technical and analytical feasibility of the experimental sequence order, but also on gaining insights into the logistics of shipping, storage, data management, data/image correlation and communication between the investigators. Equally important was to answer a basic science question that can be best addressed by multimodal correlated analysis: Can we identify, and correlate damages induced by ultraviolet radiation in single cells within the epidermis using CMI?“. Answering this question is fundamental, as damage exerted by ultraviolet B radiation (UVB) can cause cancers, precancerous skin conditions, and skin aging (Schuch et al., 2017). Humans are exposed to UV radiation as a result of work exposure, recreational habits or after therapeutic UV radiation. Furthermore, damage similar to the one exerted by UV can result from other environmental or developmental cues. Thus, there is substantial scientific, clinical, and translational need for technologies that can differentiate between damaged and undamaged cells within the tissue context. Correlating information from useful analytic modalities with the presence and location of damaged cells will allow a holistic understanding of the causes and consequences of damage and its prevention.
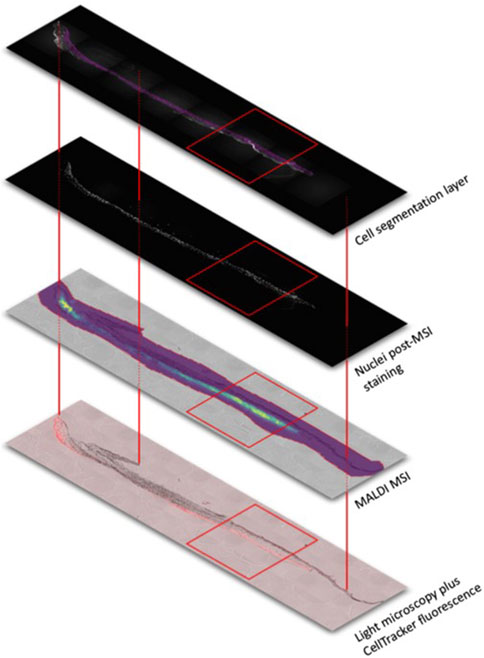
Figure 3. Schematic outline for image co-registration using three markers across all images (red lines) of a tissue section. The tissue section, approx. 6 × 3 mm2, was analysed by bright field (grey) and fluorescence microscopy (cell tracker red is highlighting UV exposed cells), MALDI mass spectrometry (lipid analysis showing the intensity heatmap of the 1-palmitoyl-2-arachidonoyl-sn-glycero-3-phosphocholine distribution with blue representing low and yellow high signal intensities) and nuclei distributions visualized by DAPI staining after mass spectrometric analysis. Advanced image analysis allow for cell segmentations based on the fluorescence data. The generated “cell mask” serves as guide for the in-depth interpretation of the molecular data within particular cells and areas (red rectangle).
Organotypic models containing epidermal keratinocytes cultured on a dermal equivalent composed of bovine collagen and dermal fibroblasts were prepared and mounted on a tissue culture support. This established model yields a stratified and functional epidermis (Mildner et al., 2010). We introduced 6% of ultraviolet B (UVB) irradiated and fluorescence-labeled keratinocytes into the epidermal compartment. This experimental treatment allowed the investigation of UV-induced changes to cells within their tissue environment and validating the results using the fluorescent label within the irradiated cells, which also serves as a fiducial marker, as demonstrated recently with a limited set of modalities (Kremslehner et al., 2020).
The initial steps upon completion of stratification and differentiation of the skin equivalents were performed either with multiphoton microscopy as in (Jain et al., 2023) before further treatment or immediately after 1) embedding in OCT (optimal cutting temperature) medium, 2) fixing the epidermal equivalents in 4% paraformaldehyde, or 3) fixing the epidermal equivalents in 4% formaldehyde and 0.5% glutaraldehyde. Part of the fixed samples were embedded in paraffin prior to distribution to the contributing investigators.
Eight sequential multi-station protocols were initiated to cover the various aspects of this project:
(1) The initial sample underwent chemical fixation without embedding and was examined using Correlative Array Tomography (CAT) in Sweden before proceeding to micro-Computed Tomography (CT) analysis in Southampton. Further elaboration on CAT is provided later in this manuscript (Mannheim et al., 2019). Through this integrated workflow, we anticipate obtaining insights into the interdependencies of density, morphology, subcellular phenotypes, cell types, and organelles.
(2) Another sample was first studied by CAT (Göteborg), before Large Scale immune EM (Groningen) and Mass Spectrometry Imaging (MSI) (Vienna) were performed. Besides the 3D information from CAT, large-scale EM provides ultrastructural information. Although the tissue is fixed and treated for EM, there is nevertheless the chance to assess information of post-translational modifications of proteins, like glycosylations, using MSI. The whole tissue block is cut into sections and every section is treated enzymatically for glycan release. MALDI mass spectrometry is then used to visualize the distribution of such glycans to add untargeted molecular information.
(3) We established a workflow for a chemically fixed sample, sequentially employing Multiphoton Microscopy (Athens), followed by CAT (combination of LM and EM, Göteborg), and µCT (Southampton) analysis. This methodology facilitates the acquisition of structural and insights ranging from the subcellular and single-cell level to the entire tissue, all from a single sample. While Multiphoton Microscopy excelled in identifying various cell types within precisely delineated tissue regions at high resolution, µCT provided a comprehensive morphological overview and general cell localization. Meanwhile, CAT afforded us detailed views of single-cell and subcellular ultrastructures.
(4) The power of Multiphoton Microscopy (Florence) was also combined with High Resolution Episcopic Microscopy (Vienna) and MSI (Vienna). Single cell localization gathered from autofluorescence, and the cell tracker red label was combined with the 3D information after volume rendering (data processing) of single tissue sections prepared during High-resolution episcopic microscopy (HREM) analysis (Keuenhof et al., 2021). 3D images of single cells in the skin equivalents were generated. Molecular information based on MSI analysis is hampered due to the embedding medium used for HREM.
(5) A 3D scan of chemically fixed, non-embedded tissue by Multiphoton Microscopy (Florence) was also combined with immunofluorescence (IF), immunohistochemistry (IHC), Stimulated Emission Depletion microscopy (STED) (Vienna) and molecular barcoding based on Immunofluorescence (Nantes) or MSI. Data analyses visualized the localization of cell-tracker red-labelled cells, the autofluorescence of collagen and the 2nd and 3rd harmonic images distinguished the different layers of the skin equivalents. Implementing immunology (IHC, and molecular barcoding) introduced a targeted molecular analysis to localize protein markers of the different cell types. The parallel workflow of MALDI MSI allowed for untargeted molecular analysis of lipids. This workflow was especially designed for high resolution information in combination with general tissue structure analysis.
(6) In this imaging setup, µCT analysis (Southampton) provided histologically relevant videos of the tissue structure in x, y and z-direction. After conventional formalin fixing, and paraffin embedding confocal fluorescence imaging and STED (Kosice), the Golgi apparatus and basal mitochondria localization was co-localized with cell tracker red and Hoechst staining of the nuclei. Detailed cell information can be gathered.
(7) Optical Coherence Tomography (Paris) of snap-frozen, non-embedded samples provided 3D information on keratinocyte localization after careful data interpretation. An average volume of nuclei was determined together with their average compactness and density. Subsequent Fluorescence Lifetime Imaging Microscopy (FLIM) (Genova) of 4 different color channels showed targeted nuclear and cell tracker red information to correlate UV exposed cells with nuclei and OCT information. Image segmentation after FLIM can be correlated with OCT info for more comprehensive information of cell shapes. Implementing molecular barcoding (Nantes) allows for targeted analysis, which is then combined with the cellular features to identify cell structures characteristic for UV exposed cells.
(8) Finally, a multiphoton microscopy approach (Vienna) was combined with superresolution microscopy (Genova), MSI (Vienna) and IF (Kosice) to showcase the power of combining targeted and untargeted imaging technologies. This workflow allowed for the correlation of cell morphology and localization with the distributions of small molecules like lipids as measured by MSI and proteins characteristic for certain cell types and cellular structures (compare Figure 3). This dataset was partially used to test the joint effort to further develop a collaborative software platform to share and analyze multimodal datasets (for details see Section 2.5 on data handling).
In the following, we focus on Correlative Array Tomography (CAT) and highlight this technique as an example of how the technique can be used in a multimodal workflow in terms of technical and computational aspects:
2.1.2.1 Method
CAT encompasses a range of techniques where multiple ribbons of serial ultrathin sections of tissues or cells are collected on a solid substrate such as glass, silicon, or tapes, from which images can be acquired using both light and electrons. This technique offers unparalleled opportunities to explore three-dimensional cellular architectures of large samples in extremely fine structural and molecular detail; it is hence particularly suited to tackle the intricate questions arising in biological and biomedical research as the skin/epidermal equivalent project. From a practical point of view, CAT combines scanning electron microscopy methods of sample preparation with fluorescence, and then applies image registration and volume rendering software tools to visualize the results. The sequential combination and application of techniques to the same specimen and region of interest also allow to validate and evaluate single-modality conclusions since each technique can provide unique information based on fundamentally different contrast mechanisms. The CAT approach also offers other important advantages for correlative microscopy: 1) it can provide very-high-content information at the light microscopy level, including markers as the fluorescence-labeled keratinocytes used in this project; 2) it enables robust registration between the light and electron microscopy channels, because the same array of ultrathin sections is imaged with the two modalities; 3) CAT, as a nondestructive technique, subsequently enables that, upon completion of the electron microscopy imaging, the samples can be revisited again at a later time and reimaged at the scanning electron microscope at a different magnification, or in a different region.
2.1.2.2 Sample Preparation
Before the initial treatments, at end of the tissue culture period, samples 1 and 5 were imaged using multiphoton microscopy, sample 3 using arrayscan microscopy.
• Initial treatment/fixation:Samples 1,2 and 3 were fixed in a special fixative (4%FA and 0.5%GA in 0.1M PB pH 7.4). Samples 4,5,6 and 7b were fixed in formalin (7,5%) samples 7a and 8 were snap frozen.
• Secondary treatment/post fixationSamples 2 and 3 were post-fixed in 1% GA + 1% Tannic Acid (TA) in 0.1M PIPES for 4 h at 4°C, followed by 5x washing in 0.1M PIPES. These samples were post-incubated in 1% TA in PBS for 15 min. Fluorescence was checked in the widefield microscope and was still present.
• Embedding
Samples 1b, 2b, 5b and 6 were embedded in paraffin for sectioning. Samples 1a, 2a and 3 were further dissected and incubated for additional 10 min in TA, and then transferred to a free-substitution machine for dehydration with ethanol and for embedding with HM20 and R221 acrylate resins that preserve the fluorescence signals (curing with UV: 40 h at −30°C, 5 h at 2 °C/h, 5 h at −20°C, 4 h at 10 °C/h, 5 h at 20°C). Samples 7a and 8 were embedded in OCT resin and stored at −20°C Samples 4 and 5a were not embedded.
• Sectioning
Samples 1b, 2b, 5b and 6 were sectioned with microtomes at room temperature. Samples 7a and 8 were sectioned with cryomicrotomes. Samples 1a, 2a and 3 were sectioned with the ultramicrotome RMC PowerTomePC and placed on gelatine-Chromium slide +0.5 nm Platinum (sampel 1); on a silicon-wafer glow-discharged for 20 s at 10 mA just before use (samples 2 & 3). For CAT, short ribbons of 300 nm thickness were lifted with an eye lash and placed on a drop of water on the wafer. Sections were then dried out on a plate heater for several minutes at a heat of around 50°C. Note that neither Osmium, nor any other heavy metal, was usedmto preserve the fluorescence. Samples 4 and 5b were not sectioned.
2.1.2.3 Microscopy
Light microscopy images from the sections were acquired with a widefield microscope, upright Zeiss AxioImager.Z2. Transmission or reflection microscopy were used for general morphological characterizations, depending on the transparency of the substrate used. While several transparent substrates were tested, the best performance was obtained with sections on Si wafers, using reflection microscopy for morphological characterization, and fluorescence for locating irradiated cells (positive cells Figure 4). The microscope is equipped with an EC Epiplan 50x/0.75 H air objective, reflection was acquired using 653 nm light source and exposure times of 10 ms, while fluorescence was measured by 548 nm Excitation, 570–640 nm Emission and exposure times of 2–4 s depending on the sample. The imaging pixel size was 182 nm/pixel. After acquiring the images, the sections underwent a baking process on a hotplate for 1 h at approximately 70°C to enhance adhesion before proceeding with heavy metal contrasting. Then the sections were subjected to a contrasting procedure, involving a 10 min treatment with 4% OsO4, followed by a subsequent 10 min immersion in 2% UA, and then a further 10 min exposure to Raynold’s Pb-citrate before they were positioned into the chamber of the field emission scanning electron microscope.
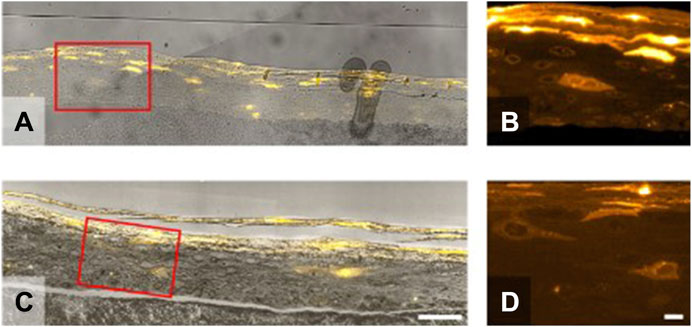
Figure 4. Sample preparation–Samples were prepared with the aim of preserving fluorescence during the generation of the arrays on a solid substrate. Two substrates were tested (A,B) gelatine-Chromium slide +0.5 nm Platinum and (C,D) silicon-wafer. The latter displayed excellent morphological landmarks in the light microscope using reflection of a 653 nm light source, and better contrast in the SEM due to increased conductivity. The improved preparation protocol of samples 2 and 3 (C,D) post fixation with Tannic Acid incubation removed unspecific fluorescent signals present in Sample 1 (A,B) located, for example, around the nucleus compare panels (B,D). Scale bars are 50 (A,C) and 10 (B,D) microns.
Scanning Electron Microscopy images were acquired with a Zeiss Gemini 450 equipped with a Backscatter Detector (BSD). The electron beam was set to 2.0 kV and 100 pA and a working distance of 4 mm. As a compromise between charging effects, resolution and speed, the sample was scanned with a 6.4 µs dwell time, line average of 5, and an imaging pixel size of 10 nm. The Atlas 5 software was used for automated acquisition of large areas via tiles. Previously acquired light microscopy images were superimposed on low resolution SEM overviews to facilitate the targeted acquisition of the areas of interest.
2.1.2.4 Image data properties
Light microscopy–Lateral resolution 300–400 nm, axial resolution depends on section thickness. Data size of about 80 MB per section with 10M pixels covering a FOV of 300 × 1200 microns, 2 color channels and 12 bit encoding. Electron microscopy–Lateral resolution down to 10 nm. Data size up to 12 Gb per section covering a FOV of 100 × 1000 microns. A full data set can consist of hundreds of sections but for this proof of concept we acquired around 10 sections per ROI. SEM raw data is 16 bit, however, in the majority of cases exports after stitching and 3D alignment (see next section for processing) are done at 8 bit.
2.1.2.5 Image processing
Light microscopy images were processed for shading correction and stitching via ZEN blue (ZEN 3.2 - Carl Zeiss GmbH, Figure 5A). Axial alignment of the data for 3D reconstruction was performed in FIJI (Schindelin et al., 2012) by using BigWarp (Bogovic et al., 2016). For this purpose the Reflected Light (RL) modality was used to define off-target landmarks (Figure 5B). By matching landmarks from consecutive sections a transformation map (Thin Plate Spline) is defined. Registration was done in a semi-automated way, with one step of computer assisted segmentation of nucleolus, followed by manual curation and definition of other landmarks in areas of poor registration. Registration accuracy was judged by a human using the RL modality. No information from the Fluorescent (FL) channel was used to avoid bias (Figure 5C).
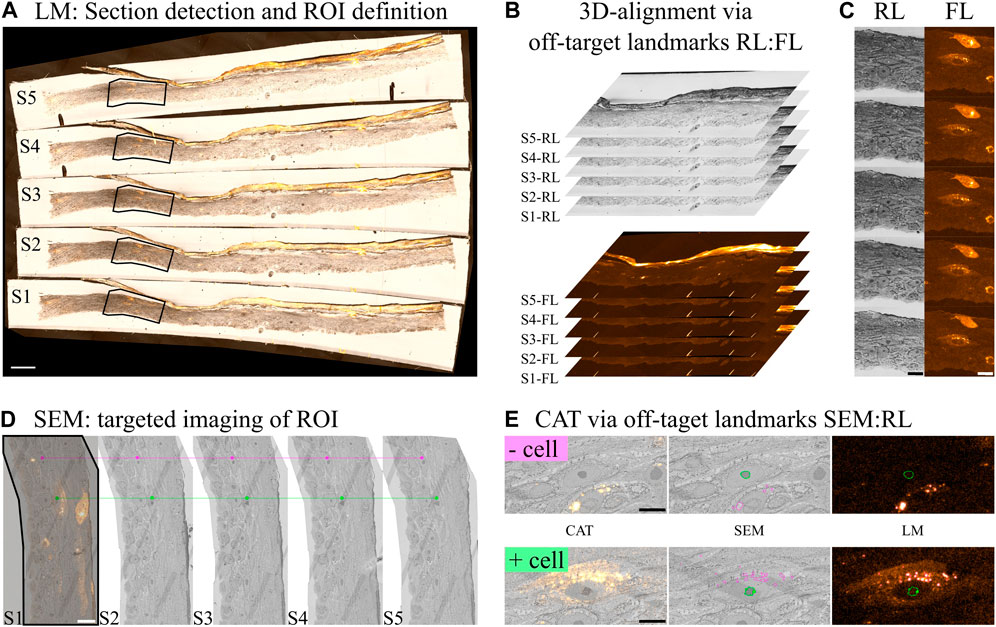
Figure 5. Image processing–(A) Light Microscopy (LM) images of the sections were processed for stitching and shading correction. From these images sections are detected and (B) the process of z-alignment carried out by using off-target landmarks (mostly the nucleolus) between the reflected (RL: general morphology) and fluorescence (FL: cells of interest) channels. (D) After post staining, the sample was imaged in the SEM and the acquisition targeted towards the regions of interest based on the LM results. Off-target landmarks were used between the SEM and RL for CAT registration. (E) CAT results for a fluorescently positive (+) and negative (−) cell. (E) Magenta segmentation shows areas of high fluorescence intensity while green segmentation shows the nucleolus. Scale bars are: (A) 100, (C,D) 20 and (E) 10 microns.
Electron microscopy images were processed for shading correction, stitching and 3D alignment using Zeiss ATLAS 5 (Figure 5D).
CAT Registration of the LM and EM datasets was performed in FIJI, using BigWarp. In a similar fashion to the LM dataset, off-target landmarks were defined in the SEM image and matched to the RL channel. No information from FL was used to avoid bias. The Thin Plate Spline transformation was then performed to register the FL channel onto the SEM image. Figure 5D shows an example of an irradiated cell (fluorescently stained) versus a non-irradiated cell (non-fluorescent).
2.1.2.6 Image visualization
Aligned datasets were exported in different file formats to test the performance of Cytomine (Marée et al., 2016). This evaluation enabled the identification of several technical issues in the software, which were reported to the Cytomine team. In addition, several new features in the user interface were requested to facilitate the exploration of, and collaboration on, multimodal datasets. Cytomine was identified as a promising platform for correlation and correlative analysis of multimodal image data, however further development is needed before it reaches its full potential of this type of data and tasks.
Aligned datasets were also imported into MoBIE (Pape et al., 2023), a FIJI plugin for sharing, exploration, and visualization of large multi-modal datasets. During the project we have been in communication with MoBIE’s developers and provided feedback to contribute to its further improvement, in particular tools for project generation using both FIJI’s macro language and Python.
2.2 Standardization
Due to the complexity of multimodal imaging approaches involving various (in vivo) imaging techniques, there is a considerable demand for standardization/harmonization of (pre-)clinical imaging methods and analysis software to assess and determine correlation precision. Specifically, reproducibility, reliability, and also transferability of preclinical imaging data towards clinical research/diagnosis (from bench to bedside) has been intensively discussed over the last decade(s) (Peers et al., 2012; Lieu et al., 2013; Begley and Ioannidis, 2015; Mannheim et al., 2019). Multiple studies have demonstrated that reproducibility and standardization might be well advanced in single preclinical institutions but is limited and challenging on a multicenter level, thereby pointing out that multiple factors play a crucial role in preclinical in vivo imaging (see Figure 6 as an example for preclinical positron emission tomography (PET) imaging), potentially significantly affecting the comparability of (multicenter) preclinical studies, such as animal handling and scanner quality control/performance (Fueger et al., 2006; Osborne et al., 2017; McDougald et al., 2019; Herfert et al., 2020). Performing preclinical studies in a multi–center approach might, however, mimic the situation in the clinics more closely, potentially leading to fewer biases and increased reliability of results (Bath et al., 2009).
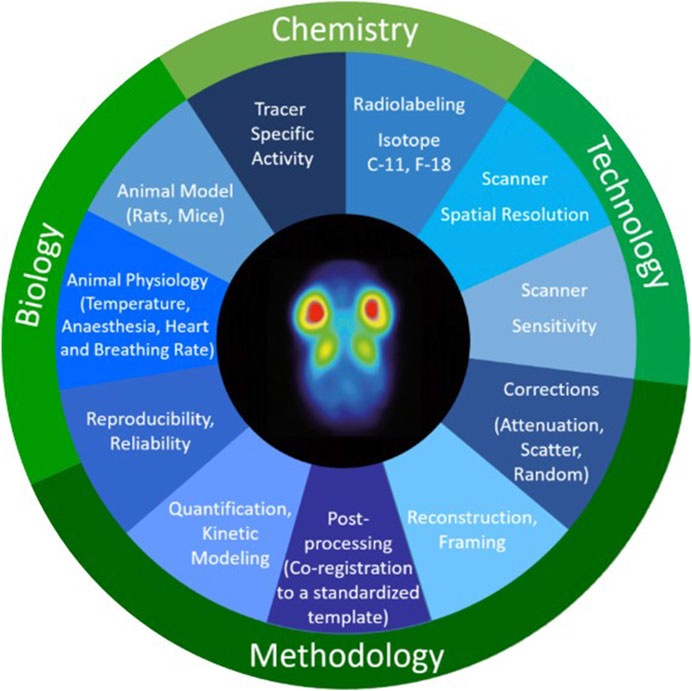
Figure 6. Chemical, technical, methodological, and biological factors that can play a crucial role in preclinical in vivo imaging potentially significantly affecting the comparability of preclinical PET data. Figure adapted from [7] with reprint permission from Springer according to their creative common license http://creativecommons.org/licenses/by/4.0/.
One factor that up-to-now has not been investigated in depth is the comparability of image analysis techniques on a multicenter level. Especially in preclinical in vivo imaging, the analysis of imaging data is strongly user-dependent, and no standard guidelines are available or agreed on at this point. This is also reflected by the fact that many preclinical researchers develop their own software packages to accommodate specific research/analyzing tasks that are not yet available in preclinical or clinical image analysis software toolboxes, hence potentially introducing biases in the reproducibility of image analysis techniques if not evaluated in depth. Furthermore, fully automatic and standardized segmentation methods are not yet available.
To investigate the interobserver variability of preclinical quantitative image analysis, WG2 of COMULIS has initiated a multicenter study in which 12 observers (4 beginners, 8 experts) from 8 different European institutions were asked to analyze/segment multiple organs (brain, tumor, heart, liver, kidneys, muscle and bladder) on the same set of 2-deoxy-2-[18F]fluoro-D-glucose ([18F]FDG) PET(/CT)-images. The study was divided in 3 separate work packages:
1. Analyze [18F]FDG PET-only data with default image analysis protocol of each institution
2. Analyze [18F]FDG PET/CT data with default image analysis protocol of each institution with the option of performing the segmentation on the CT-image
3. Analyze [18F]FDG PET/CT data following a standardized analysis protocol
For work package 1 and 2, the observers were asked to perform the PET/(CT) analysis according to their institutional standard procedure (standard used image analysis software, delineation of regions or volumes of interest (ROI, VOI) using e.g., fixed objects, manual delineation, or thresholding). On the other hand, the standardized analysis protocol for work package 3 comprised detailed segmentation instructions for each investigated organ, including whether the segmentation must be performed on the CT or PET images, shapes and volumes of individual VOIs (e.g., box or ellipsoid), and contrast thresholds if analyses were performed on the PET images.
Preliminary analysis of the 12 observer analyses revealed significant interobserver variability for work packages 1 and 2, both in the quantitative PET results and in the VOI sizes in general. Especially the muscle region showed significant differences between observers. As this region typically serves as a reference tissue region in [18F]FDG tumor imaging due to unspecific binding, significant changes in uptake for this region hamper comparability on a multicenter level significantly. Using a CT image for co-registration and segmentation of VOIs decreased the variability for some organs (e.g., tumor and brain) only. For work package 3, in which a standardized segmentation was performed as described above, the reproducibility in image analysis was further enhanced for some organs (e.g., liver and kidneys). In contrast, there was no reproducibility improvement for other organs (e.g., urinary bladder and muscle). As expected, VOI sizes were comparable in work package 3, as these were pre-defined.
These findings clearly support the fact that there is a high demand for the harmonization of preclinical image analysis on a multicenter level to increase the reliability of preclinical imaging results. Performing this multicenter image analysis study, COMULIS aimed at improving the reproducibility and reliability of preclinical imaging data and worked towards higher throughput of preclinical findings into clinical research and practice (from bench to bedside translation).
2.3 Correlation software
To enable correlated analysis and fusion of the heterogeneous information captured by different devices, the first requirement is to establish precise geometric correspondence between the acquired images–to find a spatial transformation that brings, and best aligns the data in the same coordinate system. Depending on the application scenario, different types of transformations (rigid, affine, deformable) are used. This process, known as image registration, is still often performed semi-automatically today, where a user identifies and marks salient features visible in the images to align. A successful tool for this task is eC-CLEM (Paul-Gilloteaux et al., 2017) relying on user-interaction, it has demonstrated applicability to registration tasks involving a wide range of modality combinations. However, reliance on manual interaction makes alignment a tedious and difficult process. As acquired data grows in size and dimensionality, manual alignment is less and less a feasible option. Although many software tools for automated image registration are available, the vast majority are only able to handle registration of monomodal images. The large variation in information content, dimensionality and scale of images acquired by different techniques used in CMI make automated multimodal image registration a very challenging problem.
Most registration methods for life science data rely on iterative optimization to find the transformation which maximizes a similarity measure between the images to align. The optimization is typically highly non-convex, which makes the process complicated and time consuming, while still often only delivering suboptimal solutions (e.g., a local maximum of the similarity measure). A dialog between software developers and end-users (life scientists) has been stimulated at COMULIS conferences and special sessions (e.g., at the special session “Holistic approach to correlative microscopies: From sample preparation to data integration” organized at the IEEE 2021 International symposium on Biomedical Imaging (IEEE ISBI 2021)), where the properties of novel proposed approaches were evaluated against the expressed needs. Throughout the COMULIS Action, several state-of-the-art methods for multimodal image registration have been presented and discussed within the COMULIS community. They have included both model-based and the most recent deep learning-based approaches proposed to solve, or circumvent, the problems observed with the iterative methods (Pielawski et al., 2020; Fourcade et al., 2022; Lu et al., 2022).
While data-driven deep-learning approaches typically outperform traditional model-based methods on image analysis tasks such as segmentation and classification, it has been observed that non-learning methods may still provide state-of-the-art performance for image registration (O’Mahony et al., 2019; Öfverstedt et al., 2022). At the same time, deep learning may deliver means to speed up the process, which is of a high relevance for contemporary imaging and image analysis contexts. Recent advancements, however, have led to highly accurate and time efficient non-learning approaches (Lu et al., 2022), as well as successful combinations of representation learning and monomodal iterative methods as described in following publications (O’Mahony et al., 2019; Pielawski et al., 2020; Paul-Gilloteaux et al., 2021; Öfverstedt et al., 2022; Nordling et al., 2023).
2.4 Findability and performance evaluation of software and other resources
Now that a wide variety of software for correlated imaging data analysis is available, how do the researchers who need it actually find it (Table 3)?
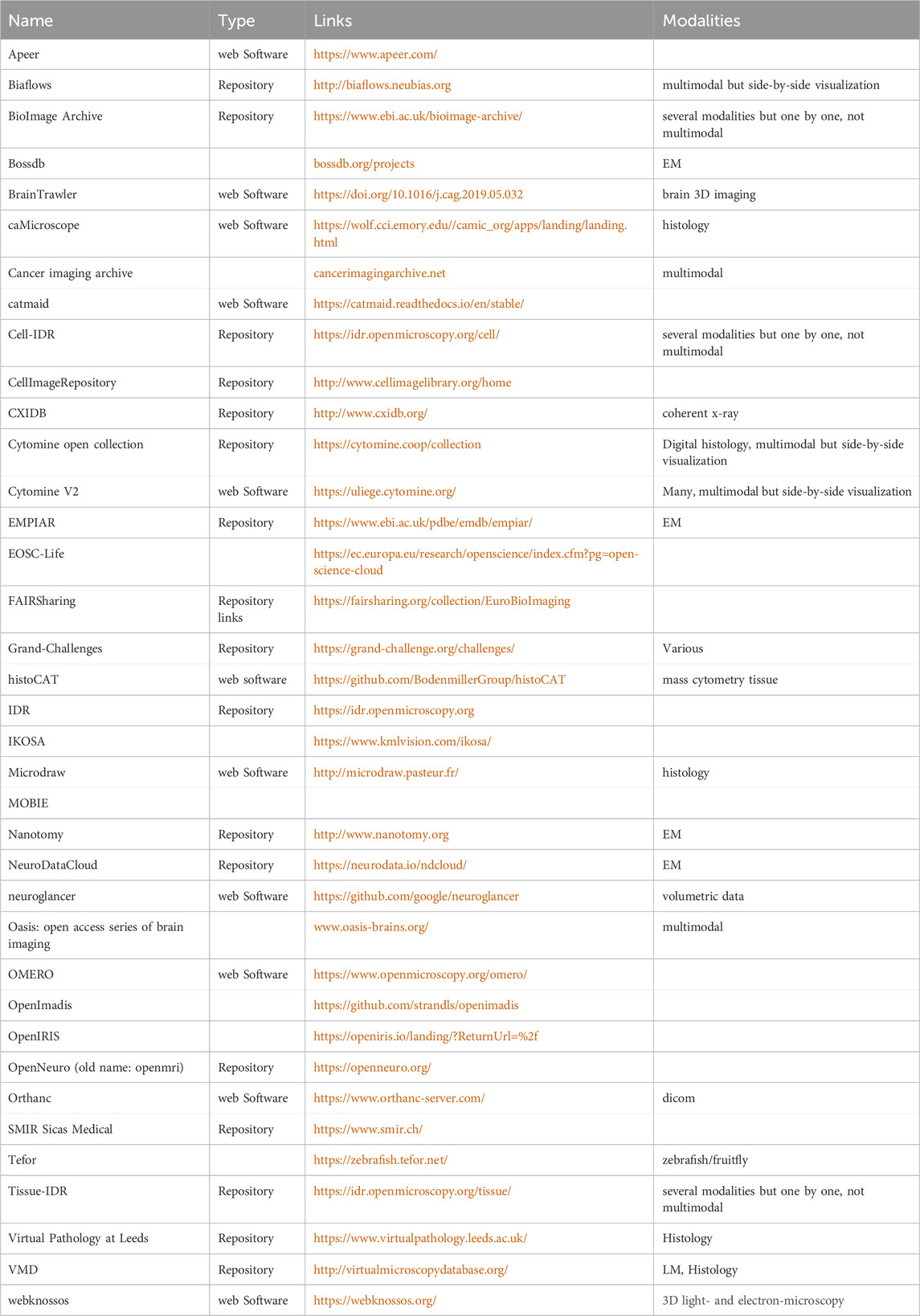
Table 3. Available software tools (visualization, exploration, annotation, and sharing) and repositories for CMI.
To ensure the reusable research objects - such as software tools, workflows, and learning materials - are findable, two layers of infrastructure are required:
1. An ontology for defining the appropriate search terms and concepts (categories)
2. A registry where the tools’ and other research objects’ functionality, application domains, and other details are stored, curated, and searchable.
2.4.1 An ontology - EDAM Bioimaging
The COMULIS project has ensured that all the concepts and search terms for finding software and other resources for analyzing correlative and multimodal imaging data, are added to the EDAM Bioimaging ontology (Kalaš et al., 2020). EDAM-bioimaging is an extension of the EDAM ontology (Ison et al., 2013; Lindblad et al., 2020) dedicated to bioimage analysis, bioimage informatics, and bioimaging. EDAM-bioimaging contains an inter-related hierarchy of concepts including bioimage analysis and related operations, bioimaging topics and technologies, and bioimage data and their formats. The modeled concepts enable interoperable descriptions of software, publications, data, and workflows, fostering reliable, transparent and “reproducible” bioimage analysis. EDAM-bioimaging is under active development, with a couple of alpha releases publicly available. It is developed by a large crowd-sourced effort in a welcoming collaboration between bioimaging experts from COMULIS and ontology developers. It is used in BIII, (BioImage Informatics Index, http://biii.eu/, more details follow below), to describe the applications of these tools, by describing the operations performed (such as segmentation, visualization, or lower level operation) and the field of applications of these tools such as the imaging modalities to which it can be applied, http://bioportal.bioontoly.org/ontologies/EDAM-BIOIMAGING.
In addition to findability, COMULIS contributed to information seeking, identification, collection and/or data curation.
2.4.2 A registry - BIII
Throughout the Action, we have collected and shared information about available software tools for CMI, enhanced with the information about their properties and relevance acquired directly through communication with the users. This has been possible due to intensive collaboration in the multidisciplinary context. Within WG4, we have created a web interface (https://www.comulis.eu/correlation-software) connecting the COMULIS community and the BIII (BioImage Informatics Index) database (https://www.biii.eu) originally developed by bioimage analyst from NEUBIAS (https://www.neubias.org), to further facilitate compilation of this valuable information and to make it accessible to any interested user. COMULIS WG4 has initiated discussions with COMULIS members across working groups to identify popular software in use for three categories of tasks: segmentation, registration and visualization. The entries of these tools were then edited, or created if not existing, in BIII by adding the multimodal imaging tag, in order to be indexed on the COMULIS webpage. This is now an automatic process such that any software tools existing in BIII and tagged with one of the three tasks identified and with “multimodal imaging” will appear in the curated list on the COMULIS website. WG4 also identified publicly available datasets as well as data sharing platforms, relevant for both the biological and medical communities, that were collected in BIII. As an example, datasets are listed in BIII at https://biii.eu/dataset. The dataset gathering is a starting point to select and further develop fully automatic correlation software as it was described in the previous section.
2.4.3 Performance assessment
Indeed, to enable selection of the most suitable software in any concrete context, it is of high importance to provide not only access to the available methods, but also a possibility to compare and evaluate them qualitatively and quantitatively. Furthermore, evaluation is essential for further development of these tools. Evaluation of novel methods, as well as training of deep learning models, require high quality annotated (ground truth) data. To facilitate the critically important step of assembling ground truth data, we have initiated collection of annotated multimodal datasets–the diverse expertise and access to a broad variety of imaging techniques, including those supporting integrated (simultaneous) imaging, available within COMULIS community provided a unique opportunity to ensure quality control and reproducibility of the involved processes. The collected curated datasets will be made publicly available, as a precious resource for a broad and diverse community of users. Another important aspect that we have discussed is selection of the most suitable set of evaluation metrics, with awareness of the diverse interests of users., COMULIS community is currently preparing a registration challenge–a public evaluation and benchmarking of available software–which will further improve our understanding of the needs and directions for advancement.
All these initiatives fit into coordination efforts which are ongoing in the direction of the findability, accessibility, interoperability, and reusability (FAIR principles) of workflows, but also the ease to access high performance computing (HPC) resources to run them (Walter et al., 2021b). They are led by European Research Infrastructures and consortiums, such as ELIXIR and EOSC-Life.
2.5 Data handling
As a result of these technological evolutions, it is becoming routine to collect raw images of hundreds of gigabytes per imaging session. Recent advances in cryo-EM, including those in detector technology with faster frame rates, have substantially increased data generation, with a typical single particle imaging session acquiring data in the gigabyte range. Light-sheet microscopy allows integrating super-resolution modules with Lattice Light-Sheet Microscopy acquiring data in the range of terabytes for a single 4D data set, and the volume of data of complex features and broad dimensions acquired in biomedical imaging, including µMagnetic Resonance Imaging (MRI) and µCT, is growing exponentially. In combination with dynamic CMI approaches, the sheer size of the bioimaging data truly enters the big data regime. Indeed, the biggest bottleneck of CMI is currently data handling and storage due to the plethora of complex, multimodal, time-varying, and diverse volumetric imaging data. Further increases in throughput and automation will generate even more data and raise the pivotal question of how to handle these huge amounts of data. Below we highlight three showcases that drafted solutions to i) share, ii) segment, and iii) reduce these big multimodal image datasets.
III. A collaborative software platform to share and analyze multimodal datasets - Cytomine:
User requirements and feedback for a multimodal imaging sharing platform were collected through workgroup meetings (at COMULIS conferences, or on-line through virtual workshops). Important features include efficient remote visualization of large images, collaborative semantic annotation of regions of interest within images, and various analysis tools (from image registration to training of deep learning models). To meet these requirements, the Cytomine open-source tool (originally developed for digital pathology (Marée et al., 2016) was further extended during the COMULIS project to support multiple imaging modalities (including x + y + c + z + t fluorescent images, mass spectrometry imaging, … ) through the complete rewrite of PIMS, its image management system (https://github.com/cytomine/pims). A Cytomine server was setup to host multimodal imaging data from the skin/epidermal equivalent project and 25 members from the COMULIS project were granted access to remotely visualize and collaboratively annotate their datasets. The tool includes the organization of datasets into “projects” with user access controls, a visualization module to split the screen to jointly visualize multiple images, new annotation concepts to link annotations across imaging modalities (as illustrated by Figure 7 and documented here: https://doc.uliege.cytomine.org/user-guide/image-groups-annotation-links), and preliminary data analysis packages that exploit multimodal sources. Cytomine’s architecture allows further extension through PIMS plug-ins (to support additional image formats) and “Apps” using Cytomine REST API and packaged as containers (e.g., to add image registration or deep learning algorithms and visualize their results in the web user interface).
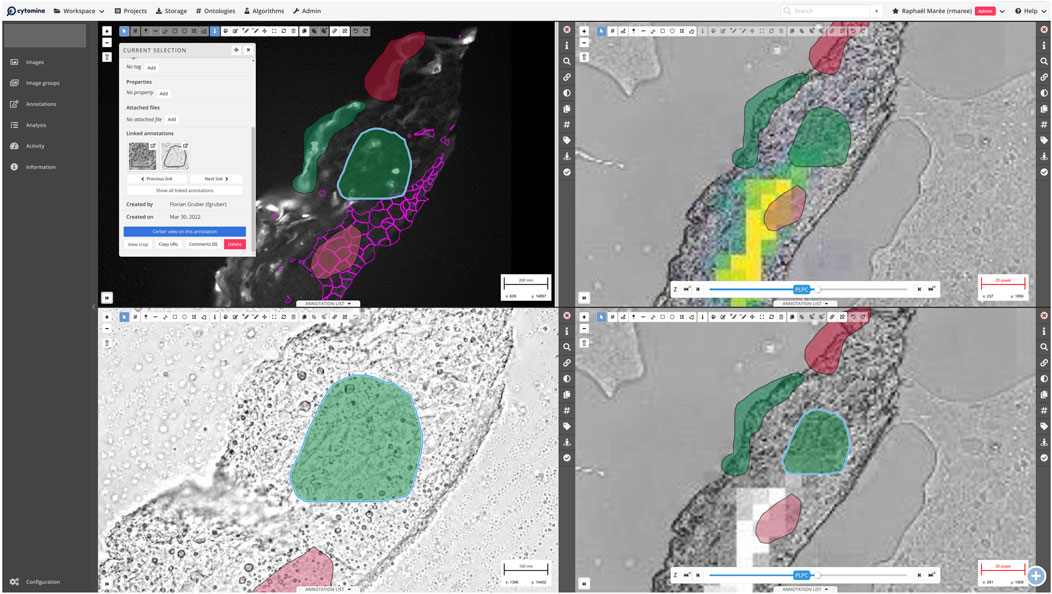
Figure 7. Screenshot of the Cytomine web viewer with multimodal imaging data (A): fluorescent imaging, (B): bright-field microscopy, and right: imaging mass spectrometry data) from the Skin/epidermal equivalent project. The viewer is split into four views where annotations are displayed to link similar regions across modalities. In each view, it is possible to use various drawing tools (top horizontal bar) to delineate regions of interest, copy-paste annotations from one view to another (with automatic scaling based on each image resolution), and link annotations. In the top-left view, a specific annotation is selected and the annotation panel shows basic annotation information (e.g., area, ontology term associated to the annotation) and to which other annotations the selected annotation is linked (“Linked annotations”). In each view, the right vertical panel allows to (un)select user annotation layers, filter annotations based on ontology terms, and to apply image processing to adapt channel contrast/brightness. The pink mesh-like structure overlaying the microscopy image outlines cell areas imported from advanced image analysis (TissueFAXS, TissueGnostics). The (B) shows an enlarged area of the sample with annotations. The top-right view displays MALDI imaging data on top of the bright field image, again annotated with areas of interest. In the presented example, MALDI data of one specific lipid, PLPC, were imported as heatmaps from MALDI MSI software (SCiLS, Bruker), representing the intensity distribution in the sample.
2.6 Data curation and accessibility
While performing CMI experiments a wealth of data is acquired whereas only a fraction is generally used and properly analyzed. This “other” data should not go to waste and could be very useful for other research questions. This has led to a push to deposit data in repositories so they can be verified, further analyzed (refined), or re-analyzed for a complete new research question. This has been common practice in the structural biology field. For example, high resolution protein structures derived from cryo electron microscopy (largely single particle analysis, SPA, but also cryo Electron Tomography, cryo ET) have been deposited in the EMDB for over 20 years.
3 Discussion & outlook
In COMULIS, the main challenge was to bring together communities with different expertise, skill sets, and interests so that they find benefits in combining them and understand and appreciate each other’s strengths. We used different tools to reach this goal. The process was first to improve understanding of each single modality and its possibilities. As a starting point to enhance the combination of modalities, in addition to presentation of different techniques at the COMULIS conferences, a compendium of imaging modalities was prepared and published (Walter et al., 2021a; Walter et al., 2021b).
The second step was to identify existing multimodal workflows, by gathering the knowledge of the different experts in the network, and to identify the tools and techniques used. From these two steps, showcase project were defined based on initial proposals by members of the network, in order to conceive new correlative workflows mixing modalities never used before, thereby enhancing the methodological difficulties and pushing discussions between expert of every modality about, e.g., the input and output state of the sample, or to arrange ideal multimodal workflows. Image processing experts were also in the loop during these processes, allowing for them to better understand the challenges, but also to define some requirements to help the image analysis (as, e.g., requirement to add nuclei staining or other overlapping to help the registration). Another way to push new workflows was via exchange between labs that was promoted on the strict criteria of multimodal acquisition, and was encouraged by a joint participation in the construction of these workflows. In the context of the showcase on skin damage, the CMI approach offered several advantages: For example, by employing a fluorescent cell mask, light microscopy was used to assess overall skin morphology, locate cells of interest, and evaluate spatial relationships between cells at the scale of microns to millimeters. Subsequently, the same sample was imaged in a targeted manner using SEM, which provided a high spatial resolution (10–5 nm) for investigating ultrastructure, imperceptible at the light microscopy level. Furthermore, by implementing 3D-EM techniques like serial-section AT, this high-resolution volume was correlated with the broader mesoscopic view obtained from light microscopy or other techniques such as X-ray volumetric imaging. The CMI workflow enabled researchers to segment and compare organelles and structures among different scales and cell types, including but not limited to single cells, nuclei, nucleoli, interstitial spaces, mitochondria, desmosomes, and keratohyalin granules. In addition, each modality contributed complementary and quantitative measurements associated with UVB damage, as for example, the shape and integrity of cell nuclei using immunofluorescence microscopy, EM, or CLEM–since sun burnt cells have pyknotic, condensed nuclei. Direct or indirect photoproducts resulting from UV damage were quantitatively screened using immunofluorescence microscopy. DNA photoproducts can be assessed using mass spectrometry (O’Neil and Deane, 2021). Cellular responses to UV damage can also be quantified using CLEM, including adaptive stress responses, inflammation, autophagosomal/lysosomal responses, mitochondrial swelling and cell death induction. Changes in metabolism resulting from UV damage can be quantitatively assessed with metabolic activity imaging (Kremslehner et al., 2020) in brightfield or fluorescent microscopy, whereas the corresponding metabolites can be quantified with MSI. One immediate goal will be to determine whether these quantitative measurements of damage can be correlated with non-invasive techniques like multiphoton imaging or OCT to assess cellular UV damage.
One of the objectives of the COMULIS project was also to engage and strengthen the link with industrial partners. To reach this goal dedicated discussion sessions (round table discussions) with industry representatives were initiated to explore how we can benefit from each other and together build a stronger community. Although the objectives of academia and industry do not always match completely, it was clear there is a lot of common interest from both sides to explore opportunities together. This was taken to the practical level through the award of a dedicated follow-on grant for COMULIS - a COST Innovators Grant (CIG). The CIG aims to develop an early-stage idea into a practical solution with the potential for commercialisation. The COMULIS CIG will focus on the development of new tools to facilitate the acquisition of cryo Electron Tomography data for in situ structural cell biology, the next level of cryo EM, critically dependent on correlative approaches.
At the other end of the EM spectrum, the interest in volumeEM (vEM, (Peddie et al., 2022; Collinson et al., 2023), has been sparked substantially. vEM was highlighted as one of the technologies to watch for 2023 (Eisenstein, 2023). Importantly, a large number of the vEM workflows are critically dependent on the incorporation of a correlative element to aid with retracing of the proverbial “needle in the haystack” in such a large volume (Lees et al., 2017; Hoffman et al., 2020; Yoshida et al., 2020). Strong interactions between the CMI and vEM communities are essential to drive the field further. Not by coincidence, many researchers in the vEM community are also heavily involved in the CMI community.
The COMULIS project was funded for 4 years (October 2018 - October 2022) through an EU COST action (https://www.cost.eu) which is specifically designed to build up communities. The action allowed us to organize conferences, focused WG meetings, and courses. Through Short Term Scientific Missions (STSM), it also funded lab exchanges to train scientists to use CMI technology and apply this knowledge to address their biological question. With the end of the grant looming, and thus the loss of the resources and opportunities provided by the action, a strategy was required to make this community initiative sustainable through further funding mechanisms and the establishment of the COMULIS community as a recognised legal entity. We are proud to announce that we have been successful in securing continued funding for another 2 years through a Chan Zuckerberg Initiative (CZI) grant dedicated to community building. Our ambitions have grown over these last 4 years and whereas the COST action was limited to European countries, we are now, via the CZI initiative, reaching out to the whole world: COMULISglobe community will be relying on, and further developing, the same tools, conferences, training, STSMs, etc., that we have used so successfully before.
Future challenges for CMI will include integrating preclinical in vivo imaging and biological (ex vivo) microscopy to zoom in from a living sample to individual close-to-native ultrastructure and adding localized spectroscopic or chemical information to the acquired structural and functional parameters. Clinical or diagnostic imaging today is very much already both a multiparametric, as well as multimodal approach–but for the most part it is all not done simultaneously. In a typical example a cancer patient undergoes an annual surveillance CT scan and a small lesion in the liver is found, which is too small to characterize. Therefore, typically another modality with different features is added next and the patient undergoes either an ultrasound or MRI scan. MRI provides enhanced soft tissue contrast and in particular makes detecting hemangiomas, fat and cysts quite easy. It can also be used to characterize the blood flow. Ultrasound can be used to evaluate any possible associated vascularity (and also fluid content), but is very user dependent. Let us assume in our example that the lesion was not well seen on ultrasound and the MRI remained still equivocal, whether it is a benign lesion or also potentially a metastasis. Next, the patient could undergo a PET scan for metabolic imaging to explore if that lesion has high glucose metabolism, indicative of a tumor. However, few scanners are geared towards multimodal imaging. PET scanners are usually combined with a CT or even MR scanner to combine anatomical (CT and MR) and/or functional imaging (MRI) with molecular information from a PET scanner (or a SPECT scanner). While most expensive, a PET/MRI scanner has found use in mostly oncologic and neurologic imaging over the last 10 years, particularly for locoregional staging of diseases for which MR is the anatomical imaging modality of choice, e.g., brain tumors, head and neck, gynecologic and prostate cancers. PET can e.g., detect presence of prostate-specific membrane antigen, combined with anatomic and parametric MR imaging such as diffusion and perfusion imaging, providing an overall multiparametric information on the tumor and therefore a much better insight in the primary disease than any standard scan could do (Weber, 2020) It is important to note though that most of these studies are still experimental and require larger studies to determine the real clinical impact (Riola-Parada et al., 2016).
To achieve meaningful statistics, throughput and quantitative imaging are crucial to biomedical research. Considering that the amount of imaging data acquired has increased massively, automation of microscopy will be crucial for mainstream biomedical research and quantitative conclusions. Automation will be accompanied by an additional boost in imaging data. Big data in bioimaging will hence be a substantial future challenge for the community. It is already becoming routine to collect raw images of hundreds of gigabytes per imaging session. Recent advances in cryo-EM, including the advances in detector technology with faster frame rates, have substantially increased data generation with a typical single particle imaging session acquiring data in the gigabyte range, and lattice light-sheet microscopy, for example, acquires data in the range of terabytes for a single 4D data set. Additionally, the biggest bottleneck of CMI is currently data handling and storage due to the plethora of complex, multimodal, time-varying and diverse volumetric imaging data (Paul-Gilloteaux et al., 2017). As throughput and automation increase, the challenge of managing vast amounts of data intensifies. Compounding this issue, there are currently no established guidelines for data handling or plans for data retention and management. Even submission to public archives like EMPIAR or Cell-IDR can only accommodate a fraction of the acquired data. Furthermore, the inclusion of diverse image modalities in multimodal workflows complicates data management and analysis. One strategy to address this challenge is data compression. Since many quantitative analyses of image datasets focus on specific macro-structures unaffected by compression artifacts, even lossy compression methods may offer a versatile approach to managing the extensive data volumes in bioimaging. Deposition in public repositories will also drive increased standardization of acquiring, processing and storing complex, multidimensional, time-varying, multiscale and complementary data from various disciplines, and foster their correlation and discovery of interdependencies. There exist high-quality dedicated repositories for microscopy data produced in the context of large research projects such as the Allen Brain Map (https://portal.brain-map.org). More “general-purpose” data emanating from CLEM or Correlative Light and X-Ray Microscopy (CLXM) experiments can be deposited in two archives with a global scope and operated by EMBL-EBI: EMPIAR (Iudin, Korir, Salavert-Torres, Kleywegt and Patwardhan, 2016; https://empiar.org) for the electron or X-ray microscopy (XM) data and BioImage Archive (https://www.ebi.ac.uk/bioimage-archive) for the light-microscopy data. New formats such as those based on ZARR (Moore et al., 2021) are also exploring the representation of data in file formats which allow to optimize the handling of data, mainly using lazy processing, i.e., load only the part of the data (at a suitable scale) only when one needs it. This also comes with strategies of superimposition of data for visualisation, avoiding duplication of transformed data, with on-the-fly display of the relative positioning of two raw datasets.
The increasing diversity of imaging modalities and the growing scale and dimensionality of acquired data urgently call for efficient approaches for their automated correlated analysis. The rapid increase of both computing power and the amount of available data have brought into focus data-driven, learning-based image analysis methods. Their successful application requires addressing several challenges. Deep learning excels when trained on large and reasonably homogeneous data; CMI in life sciences, on the other hand, typically results in few heterogeneous images, due to complexity of the acquisition, combined with exploratory aims of the research. In combination with the great difficulty to collect reliable annotations of biomedical data, this becomes a major hurdle for the adoption of learning-based methods. Few-shot and un/self-supervised methods may reduce the need for training data, but this often comes at a cost reduced performance. It should be emphasized that annotated data is required not only for model training, but also for evaluation of novel methods. It is therefore of critical importance to continue efforts to assemble and publish high quality datasets, enabling method development, quality control and reproducibility.
Most popular methods used in practice for multimodal image analysis still rely on semi-automatic approaches; this is imposed by a high heterogeneity, complexity, and high dimensionality of the data that needs to be analyzed. At the same time, modern imaging scenarios easily result in terabytes of image data, which prohibits manual analysis, but also puts high demands on performance and efficiency of automated approaches. The COMULIS community has made great progress in establishing collaborative multidisciplinary efforts towards increased awareness and assessment of the available software solutions. However, novel CMI pipelines require further development effort, since generality of the existing automatic tools, and their applicability to new, or additional modalities, is still very limited. Our aim towards modality-agnostic, generally applicable, but still powerful, fast, and easy to use techniques and tools, to advance CMI, and life sciences, remains a long-term challenge for the community.
Author contributions
PR: Writing–original draft, Formal Analysis, Investigation, Methodology. RC: Data curation, Methodology, Writing–original draft, Investigation, Formal Analysis. JF-R: Data curation, Methodology, Writing–original draft, Investigation. DF: Conceptualization, Methodology, Supervision, Writing–original draft, Data curation, Investigation, Resources. JG: Writing–original draft, Conceptualization. Florian Gruber: Formal Analysis, Investigation, Methodology, Project administration, Writing–original draft. MK: Formal Analysis, Methodology, Validation, Writing–original draft, Investigation. CK: Data curation, Methodology, Writing–original draft, Investigation. CK: Conceptualization, Supervision, Visualization, Writing–original draft, Investigation, Data curation, Formal Analysis, Methodology. DK-W: Resources, Writing–review and editing, Project administration. JL: Formal Analysis, Methodology, Project administration, Writing–review and editing, Investigation, Data curation, Software. JM: Investigation, Writing–original draft, Conceptualization, Project administration, Supervision, Visualization. MM-D: Writing–original draft, Conceptualization, Investigation, Methodology, Resources, Supervision. PP-G: Writing–review and editing, Writing–original draft, Investigation, Methodology, Software, Supervision. PaS: Conceptualization, Writing–original draft, Validation. PeS: Methodology, Investigation, Writing–original draft, AS-R: Conceptualization, Writing–review and editing. NS: Investigation, Methodology, Writing–original draft, Data curation, Software, Validation, Writing–review and editing. PV: Funding acquisition, Writing–review and editing, Conceptualization, Methodology, Supervision, Validation, Writing–original draft. AW: Conceptualization, Funding acquisition, Investigation, Resources, Supervision, Writing–original draft, Writing–review and editing, Data curation. SZ: Conceptualization, Formal Analysis, Investigation, Visualization, Writing–original draft.
Funding
The author(s) declare that financial support was received for the research, authorship, and/or publication of this article. The COMULIS project was funded for 4 years (October 2018 - October 2022) through an EU COST action CA17121 (https://www.cost.eu). This publication was funded by Aalen University.
Acknowledgments
This publication is based upon work from COST Action COMULIS (CA17121, Correlated Multimodal Imaging in Life Sciences), supported by COST (European Cooperation in Science and Technology).
Conflict of interest
The authors declare that the research was conducted in the absence of any commercial or financial relationships that could be construed as a potential conflict of interest.
The author(s) declared that they were an editorial board member of Frontiers, at the time of submission. This had no impact on the peer review process and the final decision
Publisher’s note
All claims expressed in this article are solely those of the authors and do not necessarily represent those of their affiliated organizations, or those of the publisher, the editors and the reviewers. Any product that may be evaluated in this article, or claim that may be made by its manufacturer, is not guaranteed or endorsed by the publisher.
References
Balluff, B., Heeren, R. M. A., and Race, A. M. (2022). An overview of image registration for aligning mass spectrometry imaging with clinically relevant imaging modalities. J. Mass Spectrom. Adv. Clin. Lab 23, 26–38. doi:10.1016/j.jmsacl.2021.12.006
Bashkatov, A. N., Genina, E. A., and Tuchin, V. V. (2011). Optical properties of skin, subcutaneous and muscle tissues: a review. J. Innov. Opt. Health Sci. 4 (1), 9–38. doi:10.1142/S1793545811001319
Bath, P. M., Macleod, M. R., and Green, A. R. (2009). Emulating multicentre clinical stroke trials: a new paradigm for studying novel interventions in experimental models of stroke. Int. J. Stroke 4 (6), 471–479. doi:10.1111/j.1747-4949.2009.00386.x
Bauer, E. M., De Caro, T., Tagliatesta, P., and Carbone, M. (2019). Unraveling the real pigment composition of tattoo inks: the case of bi-components phthalocyanine based greens. Dyes Pigments 167, 225–235. doi:10.1016/j.dyepig.2019.04.018
Begley, C. G., and Ioannidis, J. P. (2015). Reproducibility in science: improving the standard for basic and preclinical research. Circ. Res. 116 (1), 116–126. doi:10.1161/CIRCRESAHA.114.303819
Boardman, C. (2019). An insight into laser tattoo removal: reasoning, methods and limitations. J Aesthetic Nurs. 8 (8), 377–381. doi:10.12968/joan.2019.8.8.377
Bocca, B., Sabbioni, E., Micetic, I., Alimonti, A., and Petrucci, F. (2017). Size and metal composition characterization of nano-and microparticles in tattoo inks by a combination of analytical techniques. J. Anal. A. T. Spectrom. 32, 616–628. doi:10.1039/C6JA00210B
Bogovic, J. A., Hanslovsky, P., Wong, A., and Saalfeld, S. (2016). Robust registration of calcium images by learned contrast synthesis. Biomed. Imaging (ISBI), 1123–1126. doi:10.1109/ISBI.2016.7493463
British Medical Laser Association (2023). Selected abstracts from the 40th annual conference of the British medical laser association: BMLA 2023 annual conference. Lasers Med. Sci. 38, 197. doi:10.1007/s10103-023-03842-x
Cheong, W. F., Prahl, S. A., and Welch, A. J. (1990). A review of the optical properties of biological tissues. IEEE J. Quant. Electr. 26 (12), 2166–2185. doi:10.1109/3.64354
Collinson, L. M., Bosch, C., Bullen, A., Burden, J. J., Carzaniga, R., Cheng, C., et al. (2023). Volume EM: a quiet revolution takes shape. Nat. Methods 20 (6), 777–782. doi:10.1038/s41592-023-01861-8
Cunha, F. F., Blüml, V., Zopf, L. M., Walter, A., Wagner, M., Weninger, W. J., et al. (2023). Correction to: lossy image compression in a preclinical multimodal imaging study. J. Digit. Imaging 36, 2322. doi:10.1007/s10278-023-00800-5
Darvin, M. W., Schleusener, J., Parenz, F., Seidel, O., Krafft, C., Popp, J., et al. (2018). Confocal Raman microscopy combined with optical clearing for identification of inks in multicolored tattooed skin in vivo. Analyst 143 (29), 4990–4999. doi:10.1039/c8an01213j
Eisenstein, M. (2023). Seven technologies to watch in 2023. Nature 613 (7945), 794–797. doi:10.1038/d41586-023-00178-y
Evgenakis, E., Giouri, K., Bourliva, A., Christophoridis, C., Zacharis, C. K., and Fytianos, K. (2023). Determination of selected elements in commercially available tattoo inks of the Greek market using atomic absorption spectrometry. At. Spectrosc. 57, 1038–1053. doi:10.1080/00032719.2023.2237141
Fourcade, C., Ferrer, L., Moreau, N., Santini, G., Brennan, A., Rousseau, C., et al. (2022). Deformable image registration with deep network priors: a study on longitudinal PET images. Phys. Med. Biol. 67 (15), 155011. doi:10.1088/1361-6560/ac7e17
Fueger, B. J., Czernin, J., Hildebrandt, I., Tran, C., Halpern, B. S., Stout, D., et al. (2006). Impact of animal handling on the results of 18F-FDG PET studies in mice. J. Nucl. Med. 47 (6), 999–1006.
Herfert, K., Mannheim, J. G., Kuebler, L., Marciano, S., Amend, M., Parl, C., et al. (2020). Quantitative rodent brain receptor imaging. Mol. Imaging Biol. 22 (2), 223–244. doi:10.1007/s11307-019-01368-9
Hoffman, D. P., Shtengel, G., Xu, C. S., Campbell, K. R., Freeman, M., Wang, L., et al. (2020). Correlative three-dimensional super-resolution and block-face electron microscopy of whole vitreously frozen cells. Science 367 (6475), eaaz5357. doi:10.1126/science.aaz5357
Holzlechner, M., Bonta, M., Lohninger, H., Limbeck, A., and Marchetti-Deschmann, M. (2018). Multisensor imaging—from sample preparation to integrated multimodal interpretation of LA-ICPMS and MALDI MS imaging data. Anal. Chem. 90 (15), 8831–8837. doi:10.1021/acs.analchem.8b00816
Ison, J., Kalas, M., Jonassen, I., Bolser, D., Uludag, M., McWilliam, H., et al. (2013). EDAM: an ontology of bioinformatics operations, types of data and identifiers, topics and formats. Bioinformatics 29 (10), 1325–1332. doi:10.1093/bioinformatics/btt113
Jain, R., Tikoo, S., On, K., Martinez, B., Dervish, S., Cavanagh, L. L., et al. (2023). Visualizing murine breast and melanoma tumor microenvironment using intravital multiphoton microscopy. Star. Protoc. 2 (3), 100722. doi:10.1016/j.xpro.2021.100722
Kalaš, M., Plantard, L., Lindblad, J., Jones, M., Sladoje, N., Kirschmann, M. A., et al. (2020). EDAM-bioimaging: the ontology of bioimage informatics operations, topics, data, and formats (update 2020) [version 1; not peer reviewed]. F1000Research 9 (ELIXIR), 162. (Poster). doi:10.7490/f1000research.1117826.1
Keuenhof, K. S., Kavirayani, A., Reier, S., Geyer, S. H., Weninger, W. J., and Walter, A. (2021). High-resolution episcopic microscopy (HREM) in multimodal imaging approaches. Biomedicines 9, 1918. doi:10.3390/biomedicines9121918
Keuenhofer, K., Macfelda, K., Streli, C., Schoefer, C., Weninger, W., Geyer, S., et al. (2021). Multimodality imaging beyond CLEM: showcases of combined in-vivo preclinical imaging and ex-vivo microscopy to detect murine mural vascular lesions. Meth Cell Biol. 162, 389–415. doi:10.1016/bs.mcb.2020.10.002
Khan, S., Scher, N., and Avinoam, O. (2023). “Synchronization to visualization: dissecting myogenesis and regeneration using correlative light and electron microscopy (CLEM),” in Bioimaging in tissue engineering and regeneration. Reference series in biomedical engineering. Editors A. Walter, P. Slezak, R. Mueller, G. Kerckhofs, and B. Bajoghli (Cham: Springer). doi:10.1007/978-3-030-85569-7_5-1
Köksal, S., Ergüven, B. Z., Güzel, A., and Özdemir, Ö. (2022). “Pigment boundary and depth determination technique for photoacoustic microscopy image of tissue,” in 2022 30th signal processing and communications applications conference (SIU), 1–4. doi:10.1109/SIU55565.2022.9864739
Kremslehner, C., Miller, A., Nica, R., Nagelreiter, I.-M., Narzt, M.-S., Golabi, B., et al. (2020). Imaging of metabolic activity adaptations to UV stress, drugs and differentiation at cellular resolution in skin and skin equivalents - implications for oxidative UV damage. Redox Biol. 37, 101583. doi:10.1016/j.redox.2020.101583
Kuzmina, I., Oshina, I., Dambite, L., Lukisone, V., Maslobojeva, A., Berzina, A., et al. (2022). Skin chromophore mapping by smartphone RGB camera under spectral band and spectral line illumination. J. Biomed. Opt. 27 (2), 026004. doi:10.1117/1.JBO.27.2.026004
Lea, P. J., and Pawlowski, A. (1987). Human tattoo electron microscopic assessment of epidermis, epidermal-dermal junction, and dermis. Int. J. Dermatol 26 (7), 453–458. doi:10.1111/j.1365-4362.1987.tb00590.x
Lees, R. M., Peddie, C. J., Collinson, L. M., Ashby, M. C., and Verkade, P. (2017). Correlative two-photon and serial block face scanning electron microscopy in neuronal tissue using 3D near-infrared branding maps. Methods Cell Biol. 140, 245–276. doi:10.1016/bs.mcb.2017.03.007
Lieu, C. H., Tan, A. C., Leong, S., Diamond, J. R., and Eckhardt, S. G. (2013). From bench to bedside: lessons learned in translating preclinical studies in cancer drug development. J. Natl. Cancer Inst. 105 (19), 1441–1456. doi:10.1093/jnci/djt209
Lindblad, J., Plantard, L., Jones, M., Sladoje, N., Dormant, M., Karreman, M., et al. (2020). EDAM Bioimaging: the ontology of (bio-)image informatics and machine learning. Zenodo Versions alpha 06. doi:10.7490/f1000research.1117826.1
Lu, J., Öfverstedt, J., Lindblad, J., and Sladoje, N. (2022). Is image-to-image translation the panacea for multimodal image registration? A comparative study. PLoS One 17 (11), e0276196. doi:10.1371/journal.pone.0276196
Lucidi, M., Hristu, R., Nichele, L., Stanciu, G. A., Tranca, D. E., Holban, A. M., et al. (2020). STED nanoscopy of KK114-stained pathogenic bacteria. J. Biophot. 13, e202000097. doi:10.1002/jbio.202000097
Mannheim, J. G., Mamach, M., Reder, S., Traxl, A., Mucha, N., Disselhorst, J. A., et al. (2019). Reproducibility and comparability of preclinical PET imaging data: a multicenter small-animal PET study. J. Nucl. Med. 60 (10), 1483–1491. doi:10.2967/jnumed.118.221994
Marée, R., Rollus, L., Stévens, B., Hoyoux, R., Louppe, G., Vandaele, R., et al. (2016). Collaborative analysis of multi-gigapixel imaging data using Cytomine. Bioinformatics 32 (9), p1395–p1401. doi:10.1093/bioinformatics/btw013
McDougald, W., Vanhove, C., Lehnert, A., Lewellen, B., Wright, J., Mingarelli, M., et al. (2019). Standardization of preclinical PET/CT imaging to improve quantitative accuracy, precision and reproducibility: a multi-center study. J. Nucl. Med., jnumed.119.231308. doi:10.1007/s11307-018-01305-2
Mildner, M., Jin, J., Eckhart, L., Sterniczky, B., Födinger, D., Tschachler, E., et al. (2010). Knockdown of filaggrin impairs diffusion barrier function and increases UV sensitivity in a human skin model. J. Invest. Dermatol 130 (9), 2286–2294. doi:10.1038/jid.2010.115
Moore, J., Allan, C., Besson, S., Burel, J.-M., Diel, E., Gault, D., et al. (2021). OME-NGFF: a next-generation file format for expanding bioimaging data-access strategies. Nat. Methods 18, 1496–1498. doi:10.1038/s41592-021-01326-w
Moseman, K., Ahmed, A., Ruhren, A., and Swierk, J. R. (2024). What’s in my ink: an analysis of commercial tattoo ink on the US market. Anal. Chem. 96 (9), 3906–3913. doi:10.1021/acs.analchem.3c05687
Nešic, N., Heiligenstein, X., Zopf, L., Blüml, V., Keuenhof, K. S., Wagner, M., et al. (2024). Automated segmentation of cell organelles in volume electron microscopy using deep learning. Microsc. Res. Tech., 1–15. doi:10.1002/jemt.24548
Nordling, L., Öfverstedt, J., Lindblad, J., and Sladoje, N. (2023). “Contrastive learning of equivariant image representations for multimodal deformable registration,” in IEEE 20th international symposium on biomedical imaging, 1–5. ISBI2023.
Öfverstedt, J., Lindblad, J., and Sladoje, N. (2022). Fast computation of mutual information in the frequency domain with applications to global multimodal image alignment. Pattern Recognit. Lett. 159, 196–203. doi:10.1016/j.patrec.2022.05.022
O’Mahony, N., Campbell, S., Carvalho, A., Harapanahalli, S., Hernandes, G. V., Krpalkova, L., et al. (2019). “Deep learning vs. Traditional computer vision,” in Advances in computer vision. CVC. Advances in intelligent systems and computing. Editors K. Arai, and S. Kapoor (Cham: Springer), 943, 128–144. doi:10.1007/978-3-030-17795-9_10
O’Neil, L. J., and Deane, K. D. (2021). Striking a balance in rheumatoid arthritis prevention trials. Nat. Rev. Rheumat 17, 385–386. doi:10.1038/s41584-021-00627-w
Osborne, D. R., Kuntner, C., Berr, S., and Stout, D. (2017). Guidance for efficient small animal imaging quality control. Mol. Imaging Biol. 19 (4), 485–498. doi:10.1007/s11307-016-1012-3
Pape, C., Meechan, K., Moreva, E., Schorb, M., Chiaruttini, N., Zinchenko, V., et al. (2023). MoBIE: a Fiji plugin for sharing and exploration of multi-modal cloud-hosted big image data. Nat. Methods 20, 475–476. doi:10.1038/s41592-023-01776-4
Paul-Gilloteaux, P., Heiligenstein, X., Belle, M., Domart, M.-C., Larijani, B., Collinson, L., et al. (2017). eC-CLEM: flexible multidimensional registration software for correlative microscopies. Nat. Methods 14 (2), 102–103. doi:10.1038/nmeth.4170
Paul-Gilloteaux, P., Tosi, S., Hériché, J.-K., Gaignard, A., Ménager, H., Marée, R., et al. (2021). Bioimage analysis workflows: community resources to navigate through a complex ecosystem. F1000Res 10, 320. doi:10.12688/f1000research.52569.1
Peddie, C. J., Genoud, C., Kreshuk, A., Meechan, K., Micheva, K. D., Narayan, K., et al. (2022). Volume electron microscopy. Nat. Rev. Methods Prim. 2, 51. doi:10.1038/s43586-022-00131-9
Peers, I. S., Ceuppens, P. R., and Harbron, C. (2012). In search of preclinical robustness. Nat. Rev. Drug Discov. 11 (10), 733–734. doi:10.1038/nrd3849
Pielawski, N., Wetzer, E., Öfverstedt, J., Lu, J., Wählby, C., Lindblad, J., et al. (2020). CoMIR: contrastive multimodal image representation for registration. Adv. Neural Inf. Process. Syst. 33 (33), 18433–18444. doi:10.48550/arXiv.2006.06325
Polishchuk, R. S., Polishchuk, E. V., Marra, P., Alberti, S., Buccione, R., Luini, A., et al. (2000). Correlative light-electron microscopy reveals the tubular-saccular ultrastructure of carriers operating between Golgi apparatus and plasma membrane. J. Cell Biol. 148 (1), 45–58. doi:10.1083/jcb.148.1.45
Reier, S., Turyanskaya, A., Heimel, P., Frischauf, N., Meusburger, D., Heuser, T., et al. (2021). Cross-modality imaging of bisphosphonate-treated murine jawbones. Analyst 14, 4683–4699. doi:10.1039/D0AN02373F
Riola-Parada, C., García-Cañamaque, L., Pérez-Dueñas, V., Garcerant-Tafur, M., and Carreras-Delgado, J. L. (2016). Simultaneous PET/MRI vs PET/CT in oncology. A systematic review. Rev. Esp. Med. Nucl. Imagen Mol. 35 (5), 306–312. doi:10.1016/j.remn.2016.06.001
Rudraiah, P. S., Duadi, H., and Fixler, D. (2021). Bottom layer absorption coefficients extraction from two-layer phantoms based on crossover point in diffuse reflectance. J. Biomed. Opt. 26 (11), 117001. doi:10.1117/1.JBO.26.11.117001
Rudraiah, P. S., Duadi, H., and Fixler, D. (2022b). “Extraction of absorption coefficients from two-layer phantoms based on crossover point in diffuse reflectance,” in Proceedings volume 11976, nanoscale imaging, sensing and actuation for biomedical applications XIX. doi:10.1117/12.2608530
Rudraiah, P. S., Duadi, H., and Fixler, D. (2022c). Diffused reflectance measurements to detect tattoo ink location in skin using the crossover point. J. Biophot. 15 (4), e202200003. doi:10.1002/jbio.202200003
Rudraiah, P. S., Nandi, S., Duadi, H., and Fixler, D. (2022a). Deep tattoo ink depth profiling in ex vivo porcine skin using diffuse reflectance spectroscopy. IEEE Xplore 29, 1–6. doi:10.1109/JSTQE.2022.3208570
Schindelin, J., Arganda-Carreras, I., Frise, E., Kaynig, V., Longair, M., Pietzsch, T., et al. (2012). Fiji: an open-source platform for biological-image analysis. Nat. Methods 9, 676–682. doi:10.1038/nmeth.2019
Schuch, A. P., Moreno, N. C., Schuch, N. J., Menck, C. F. M., and Garcia, C. C. M. (2017). Sunlight damage to cellular DNA: focus on oxidatively generated lesions. Free Radic. Biol. Med. 107, 110–124. doi:10.1016/j.freeradbiomed.2017.01.029
Sousa, A. L., Rodrigues Lóios, J., Faísca, P., and Tranfield, E. M. (2021). The Histo-CLEM Workflow for tissues of model organisms. Methods Cell Biol. 162, 13–37. doi:10.1016/bs.mcb.2020.12.001
Steven, L. (2013). Corrigendum: optical properties of biological tissues: a review. Phys. Med. Biol. 58, 5007–5008. doi:10.1088/0031-9155/58/14/5007
Váradi, T., Schneider, M., Sevcsik, E., Kiesenhofer, D., Baumgart, F., Batta, G., et al. (2019). Homo- and heteroassociations drive activation of ErbB3. Biophysical J. 117 (10), 1935–1947. doi:10.1016/j.bpj.2019.10.001
Walter, A., Kleywegt, G. J., and Verkade, P. (2021c). Correlated multimodal imaging: building a community. Methods Cell Biol. 162, 417–430. doi:10.1016/bs.mcb.2020.12.010
Walter, A., Mannheim, J. G., and Caruana, C. J. (2021a). Imaging modalities for biological and preclinical research: a compendium, volume 1, Part I: ex vivo biological imaging. IOP Sci., 541p. doi:10.1088/978-0-7503-3059-6
Walter, A., Munoz-Baruttia, A., Jones, M., Mannheim, J. G., Collinson, L., Andreana, M., et al. (2021b). “Emerging technologies and outlook,” in Imaging modalities for biological and preclinical research: a compendium, volume 2, parts II-IV in vivo preclinical imaging, multimodality imaging and outlook. Chapter 31. doi:10.1088/978-0-7503-3747-2ch31
Walter, A., Paul-Gilloteaux, P., Plochberger, B., Sefc, L., Verkade, P., Mannheim, J. G., et al. (2020). Correlated multimodal imaging in life sciences: expanding the biomedical horizon. Front. Phys. 8. doi:10.3389/fphy.2020.00047
Weber, W. (2020). Clinical PET/MR. Recent Results Cancer Res. 216, 747–764. doi:10.1007/978-3-030-42618-7_22
Yoshida, N., Domart, M. C., Peddie, C. J., Yakimovich, A., Mazon-Moya, M. J., Hawkins, T. A., et al. (2020). The zebrafish as a novel model for the in vivo study of Toxoplasma gondii replication and interaction with macrophages. Dis. Model Mech. 20 (7), dmm043091. doi:10.1242/dmm.043091
Zima, N. G., Verdel, N., Lukac, M., and Majaron, B. (2023). Objective monitoring of laser tattoo removal in human volunteers using an innovative optical technique: a proof of principle. Lasers Surg. Med. 55 (8), 724–733. doi:10.1002/lsm.23720
Glossary
Keywords: correlated multimodal imaging, preclinical hybrid imaging, correlated light and electron microscopy, image registration, correlation software, image databases and repositories
Citation: Rudraiah PS, Camacho R, Fernandez-Rodriguez J, Fixler D, Grimm J, Gruber F, Kalaš M, Kremslehner C, Kuntner C, Kuzdas-Wood D, Lindblad J, Mannheim JG, Marchetti-Deschmann M, Paul-Gilloteaux P, Sampaio P, Sandbichler P, Sartori-Rupp A, Sladoje N, Verkade P, Walter A and Zoratto S (2024) Correlated multimodal imaging in life sciences: lessons learnt. Front. Biomater. Sci. 3:1338115. doi: 10.3389/fbiom.2024.1338115
Received: 14 November 2023; Accepted: 11 June 2024;
Published: 30 July 2024.
Edited by:
Paul Campagnola, University of Wisconsin-Madison, United StatesReviewed by:
Shuvashis Das Gupta, University of Oulu, FinlandKirby Campbell, St. Jude Children’s Research Hospital, United States
Copyright © 2024 Rudraiah, Camacho, Fernandez-Rodriguez, Fixler, Grimm, Gruber, Kalaš, Kremslehner, Kuntner, Kuzdas-Wood, Lindblad, Mannheim, Marchetti-Deschmann, Paul-Gilloteaux, Sampaio, Sandbichler, Sartori-Rupp, Sladoje, Verkade, Walter and Zoratto. This is an open-access article distributed under the terms of the Creative Commons Attribution License (CC BY). The use, distribution or reproduction in other forums is permitted, provided the original author(s) and the copyright owner(s) are credited and that the original publication in this journal is cited, in accordance with accepted academic practice. No use, distribution or reproduction is permitted which does not comply with these terms.
*Correspondence: Andreas Walter, YW5kcmVhcy53YWx0ZXJAaHMtYWFsZW4uZGU=