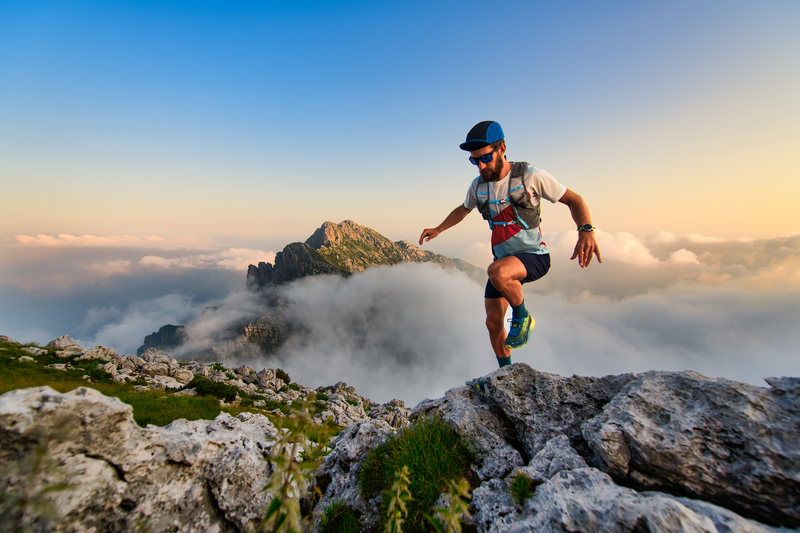
95% of researchers rate our articles as excellent or good
Learn more about the work of our research integrity team to safeguard the quality of each article we publish.
Find out more
MINI REVIEW article
Front. Bioinform.
Sec. Single Cell Bioinformatics
Volume 5 - 2025 | doi: 10.3389/fbinf.2025.1554010
This article is part of the Research Topic Multiomics Approaches in the Central Nervous System and Neuropathies View all articles
The final, formatted version of the article will be published soon.
You have multiple emails registered with Frontiers:
Please enter your email address:
If you already have an account, please login
You don't have a Frontiers account ? You can register here
Single-cell and spatial technologies have transformed our understanding of brain immunology, providing unprecedented insights into immune cell heterogeneity and spatial organisation within the central nervous system. These methods have uncovered complex cellular interactions, rare cell populations, and the dynamic immune landscape in neurological disorders.This review highlights recent advances in single-cell "omics" data analysis and discusses their applicability for brain immunology. Traditional statistical techniques, adapted for single-cell omics, have been crucial in categorizing cell types and identifying gene signatures, overcoming challenges posed by increasingly complex datasets. We explore how machine learning, particularly deep learning methods like autoencoders and graph neural networks, is addressing these challenges by enhancing dimensionality reduction, data integration, and feature extraction. Newly developed foundation models present exciting opportunities for uncovering gene expression programs and predicting genetic perturbations.Focusing on brain development, we demonstrate how single-cell analyses have resolved immune cell heterogeneity, identified temporal maturation trajectories, and uncovered potential therapeutic links to various pathologies, including brain malignancies and neurodegeneration.
Keywords: single-cell genomics, data integration, deep learning, multi-omcis, development, trajectory analysis, Gene Co-expression Network, perturbation prediction
Received: 07 Jan 2025; Accepted: 24 Mar 2025.
Copyright: © 2025 Binder, Khavaran and Sankowski. This is an open-access article distributed under the terms of the Creative Commons Attribution License (CC BY). The use, distribution or reproduction in other forums is permitted, provided the original author(s) or licensor are credited and that the original publication in this journal is cited, in accordance with accepted academic practice. No use, distribution or reproduction is permitted which does not comply with these terms.
* Correspondence:
Roman Sankowski, University of Freiburg Medical Center, Freiburg, Germany
Disclaimer: All claims expressed in this article are solely those of the authors and do not necessarily represent those of their affiliated organizations, or those of the publisher, the editors and the reviewers. Any product that may be evaluated in this article or claim that may be made by its manufacturer is not guaranteed or endorsed by the publisher.
Research integrity at Frontiers
Learn more about the work of our research integrity team to safeguard the quality of each article we publish.