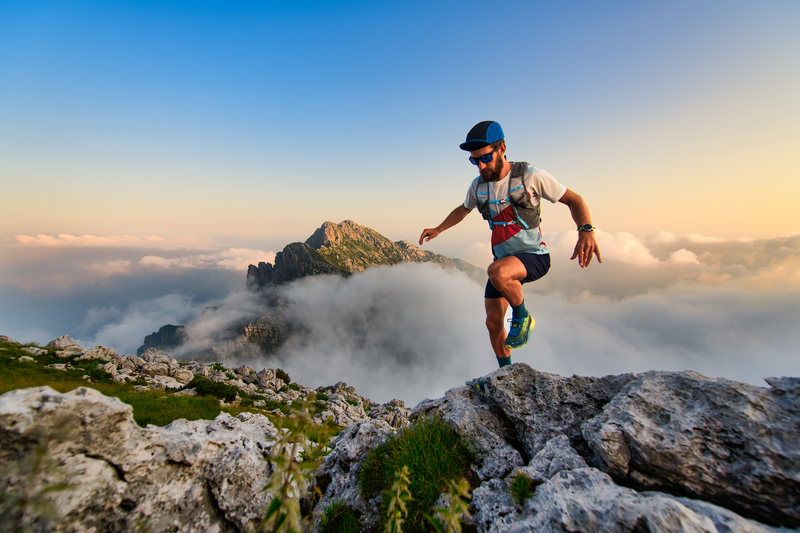
95% of researchers rate our articles as excellent or good
Learn more about the work of our research integrity team to safeguard the quality of each article we publish.
Find out more
ORIGINAL RESEARCH article
Front. Bioinform.
Sec. Drug Discovery in Bioinformatics
Volume 5 - 2025 | doi: 10.3389/fbinf.2025.1522401
The final, formatted version of the article will be published soon.
You have multiple emails registered with Frontiers:
Please enter your email address:
If you already have an account, please login
You don't have a Frontiers account ? You can register here
The incidence of non-alcoholic fatty liver disease (NAFLD), encompassing the more severe non-alcoholic steatohepatitis (NASH), is rising alongside the surges in diabetes and obesity.Increasing evidence indicates that NASH is responsible for a significant share of idiopathic hepatocellular carcinoma (HCC) cases, a fatal cancer with a five-year survival rate below 22%. Biomarkers can facilitate early screening and monitoring of at-risk NAFLD/NASH patients and assist in identifying potential drug candidates for treatment. This study utilized an ensemble feature selection framework to analyze transcriptomic data, identifying biomarker genes associated with the stage-wise progression of NAFLD-related HCC. Seven machine learning algorithms were assessed for disease stage classification. Twelve feature selection methods including correlation-based techniques, mutual information-based methods, and embedded techniques were utilized to rank the top genes as features, through this approach, multiple feature selection methods were combined to yield more robust features important in this disease progression. Cox regression-based survival analysis was carried out to evaluate the biomarker potentiality of these genes. Furthermore, multiphase drug repurposing strategy and molecular docking were employed to identify potential drug candidates against these biomarkers. Among the seven machine learning models initially evaluated, DISCR resulted as the most accurate disease stage classifier. Ensemble feature selection identified ten top genes, among which eight were recognized as potential biomarkers based on survival analysis. These include genes ABAT, ABCB11, MBTPS1, and ZFP1 mostly involved in alanine and glutamate metabolism, butanoate metabolism, and ER protein processing. Through drug repurposing, 81 candidate drugs were found to be effective against these markers genes, with Diosmin, Esculin, Lapatinib, and Phenelzine as the best candidates screened through molecular docking and MMGBSA. The consensus derived from multiple methods enhances the accuracy of identifying relevant robust biomarkers for NAFLD-associated HCC. The use of these biomarkers in a multiphase drug repurposing strategy highlights potential therapeutic options for early intervention, which is essential to stop disease progression and improve outcomes.
Keywords: NAFLD, HCC, machine learning, Ensemble feature selection, drug repurposing, molecular docking
Received: 28 Nov 2024; Accepted: 04 Apr 2025.
Copyright: © 2025 Ghosh, Mandal and Thakur. This is an open-access article distributed under the terms of the Creative Commons Attribution License (CC BY). The use, distribution or reproduction in other forums is permitted, provided the original author(s) or licensor are credited and that the original publication in this journal is cited, in accordance with accepted academic practice. No use, distribution or reproduction is permitted which does not comply with these terms.
* Correspondence:
Sukhen Das Mandal, Ghani Khan Choudhury Institute of Engineering & Technology (GKCIT), West Bengal, 732102, India
Subarna Thakur, Department of Bioinformatics, University of North Bengal, Darjeeling, 734013, West Bengal, India
Disclaimer: All claims expressed in this article are solely those of the authors and do not necessarily represent those of their affiliated organizations, or those of the publisher, the editors and the reviewers. Any product that may be evaluated in this article or claim that may be made by its manufacturer is not guaranteed or endorsed by the publisher.
Research integrity at Frontiers
Learn more about the work of our research integrity team to safeguard the quality of each article we publish.