- 1Department of Biochemistry and Molecular Biology, Weill Bugando School of Medicine, Catholic University of Health and Allied Sciences, Mwanza, Tanzania
- 2Train-The-Trainers for Bioinformatics Group, Human Heredity and Health for Africa Bioinformatics Network (H3ABioNet), Cape Town, South Africa
- 3Department of Immunology and Molecular Biology, College of Health Sciences, School of Biomedical Sciences, Makerere University, Kampala, Uganda
- 4The African Center of Excellence in Bioinformatics and Data-Intensive Sciences, The Infectious Diseases Institute, College of Health Sciences, Makerere University, Kampala, Uganda
- 5Africa Centres for Disease Control and Prevention, African Union Commission, Addis Ababa, Ethiopia
Introduction
Precise prediction of the risk of acquiring complex human diseases using genomic data has gained a considerable traction among clinicians, medical geneticists and researchers, particularly in this era of next-generation sequencing. Multi-omics methods utilize various high-throughput screening technologies such as genomics (GWAS), DNA methylomics, metagenomics, transcriptomics, proteomics, metabolomics, and many others which play a crucial role in advancing the understanding of human diseases (Figure 1). These diverse multi-omics indicators create a comprehensive framework, yielding significant insights into future health status predictions. The polygenic risk scores (PRS) —a calculation of a person’s genetic predisposition to a trait or disease based on their genotype from pertinent genome-wide association study (GWAS) findings (Choi et al., 2020)— as well as methylation risk scores (MRS) —a linear combination of CpG (5′–C–phosphate–G–3′) methylation (covalent attachment of a methyl group onto the cytosine residue of DNA) states (Thompson et al., 2022)— have shown promise in predicting complex human diseases accurately (Liu et al., 2024). However, their translation into clinical care is yet to be realized. Several efforts have been made to improve their accuracy in predicting complex human diseases, such as increasing diversity in the genetic training databases, such as the All of Us Research Program, and including conventional risk factors in the PRS model (Liu et al., 2024). In the realm of predictive medicine, conventional risk factors span socio-demographic elements like age and sex, alongside anthropometric data such as body mass index (BMI) and crucial clinical measures, including blood pressure, lipid profiles, kidney and liver function tests, and other key biomarkers such as glycated hemoglobin (HbA1c). These conventional risk factors intertwine with lifestyle choices, behaviors, and environment.
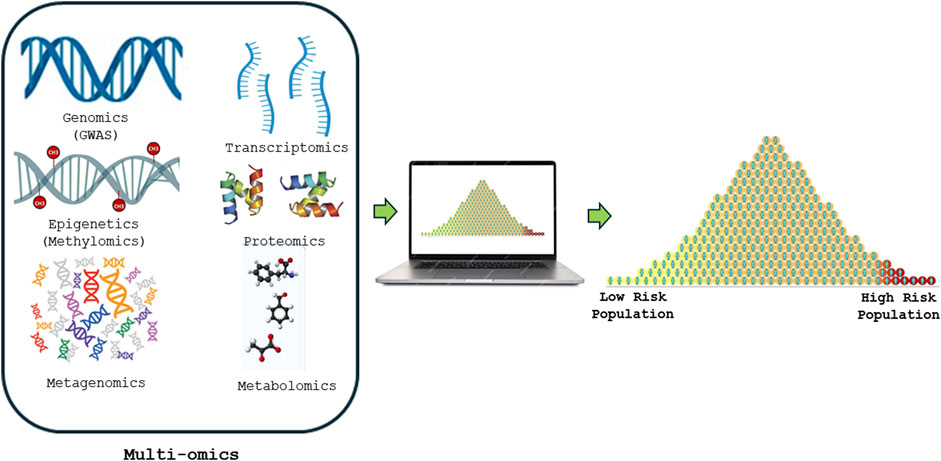
Figure 1. Shows how Multi-omics risk score is constructed from various high-throughput screening technologies such as genomics (GWAS), DNA methylomics, metagenomics, transcriptomics, proteomics and metabolomics to precisely predict and advancing the understanding of human diseases.
The advent of GWAS, methylome-wide association studies (MWAS), and transcriptome-wide association studies (TWAS) have propelled genetic research forward by leaps and bounds, enabling the genotyping, methylation typing, and transcriptome analysis of millions of human samples. Through this vast endeavor, researchers have extracted genetic variants (Wu et al., 2021) and methylation patterns intricately linked to disease susceptibility across the human genome. The genetic variants and methylation patterns serve as the building blocks for constructing PRS (Choi et al., 2020) and MRS tailored to predict complex diseases in individuals based on their unique architecture. The efficacy and clinical potential of these tools shine brightly, offering invaluable insights into risk prediction for a plethora of common complex human diseases including cardiovascular diseases, cancers, diabetes mellitus, Alzheimer’s disease, and ankylosing spondylitis (Cappozzo et al., 2022). They represent transformative applications in the arsenal of personalized medicine, promising to revolutionize healthcare by unlocking more secrets hidden within our genomes.
The immense potential of genome-wide genotyping arrays lies in their ability to serve as a cost-effective approach capable of generating hundreds of PRSs. This groundbreaking technology is now undergoing rigorous evaluation in clinical studies across global healthcare systems. The allure of PRSs as predictive tools resonates profoundly, offering a glimpse into a future where personalized healthcare is not just a dream, but a tangible reality poised to transform medical practice. The full clinical potential of PRS and MRS remains largely untapped (Martin et al., 2019). This reality is especially pronounced in populations with high genetic diversity, diminished linkage blocks, and historical under-representation in genome databases, such as those of sub-Saharan African descent. The journey towards widespread clinical implementation of PRS is still in its infancy, with considerable challenges to overcome. Yet, with determination and concerted effort, bridging these gaps holds the key to unlocking the transformative power of PRS and MRS in diverse populations worldwide. Of note, there are concerted efforts such as the All of Us Research Program (Bick et al., 2024), Human Heredity and Health Africa (H3Africa), and others, to increase the representation of historically under-represented populations in the global genome databases to leverage this disparity. Ultimately the quantity and quality of data to compute PRS and MRS are escalating.
Discussion
The potential of multi-omics data in predicting precisely the human complex diseases
The advent of multi-omics technologies and accrued data thereof in recent era suggest the feasibility of measuring and combining various omics data and cellular factors. This enables the creation of multi-omics risk scores (MoRS) with enhanced predictability for complex diseases (Liu et al., 2024). PRS integrated with multi-omics data analyses, including metagenomics, epigenomics, and transcriptomics have revealed potential biomarkers and ultimately improved predictability for several prevalent age-related conditions like heart disease, diabetes, dementia, and various cancers (Liu et al., 2024).
The human gut microbiota, which refers to the collection of microorganisms residing in someone’s gastrointestinal tract, has been implicated in numerous common diseases (Chen et al., 2024; Huang et al., 2024). Specific microbial signatures in the gut have been linked to mortality and the development of diseases such as type 2 Diabetes (T2D), liver issues, and respiratory diseases among the general population (Liu et al., 2024). This suggests that the composition of the gut microbiome could potentially aid in predicting disease risk. It is worth noting that while GWAS has shed light on the genetic underpinnings of the gut microbiome, it is evident that the heritability of the gut microbiome is relatively low. Furthermore, similarities in the gut microbiome across generations are primarily associated with living in the same household rather than genetic factors. Recent studies have highlighted the association of various omics data such as gut metagenomics, DNA methylome data from epigenome-wide association studies and transcriptomics data with complex human diseases (Wu et al., 2021; Liu et al., 2024).
Recent research indicates that PRS models alone demonstrate superior predictive power compared to traditional risk factors (Liu et al., 2024). Furthermore, integrating MRS and transcriptomics data into the PRS showed a substantial improvement in prediction of prostate cancer (Wu et al., 2021). However, when conventional risk factors are incorporated into the PRS model, performance improves (Liu et al., 2024). Moreover, integrating both conventional risk factors and additional omics data such as from gut metagenomics into the PRS model significantly enhances its predictive performance for complex human diseases (Liu et al., 2024). Therefore, these studies demonstrated that the inclusion of other omics data from gut metagenomics, DNA methylomics and transcriptomics have shown a promise to improve the prediction of the incidence of age-related complex human diseases such as coronary artery disease, type 2 diabetes, Alzheimer’s disease, and prostate cancer (Wu et al., 2021; Liu et al., 2024).
Recent research suggests that studying blood DNA methylation at various CpG sites can serve as a valuable surrogate biomarker for exposure to risk factors, aiding in the prediction of complex human diseases such as cardiovascular diseases and in identifying high-risk populations (Cappozzo et al., 2022). Methylation risk scores (MRS) are typically constructed to model the relationship between methylation at CpG sites and specific traits or diseases through epigenome-wide association studies (EWAS). DNA methylation scores have been effectively utilized to assess an individual’s biological age (epigenetic clock) and have been strongly associated with several non-communicable diseases (NCDs) risk factors such as smoking, alcohol consumption, low physical activity, obesity, socioeconomic status, and occupational characteristics. This existing collinearity has made DNA methylation scores a powerful tool for predicting aging-related diseases as well as lifestyle-related diseases such as cancer and cardiovascular diseases (Cappozzo et al., 2022).
These epigenetic clocks demonstrate strong predictive capabilities for aging-related diseases and overall mortality. Research indicates that the risk of developing complex human diseases depends on the interaction between host genetic factors, environmental influences, and human behaviors or lifestyles. Incorporating conventional risk factors such as age, sex, smoking, and alcohol consumption into models accounts for human behavior (Levine et al., 2018). Studies, including the one conducted by Liu et al., have demonstrated that including these conventional risk factors improves the predictive ability for complex human diseases (Liu et al., 2024). Moreover, environmental factors, gene-environment interaction, and host lifestyle can be surrogated by epigenetic methylation analysis. Therefore, it is of the essence for the DNA methylomics data to be integrated into PRS models to enhance predictability for complex human diseases. There is a hypothesis suggesting that epigenomics data from DNA methylation might offer better predictive ability than many of the current PRS utilized today (Thompson et al., 2022). Consequently, integrating these multi-omics data into the PRS model could potentially yield the most effective predictive model for complex human diseases. To the best of our knowledge, there are very limited studies reported to integrate the DNA methylomics data into the PRS. Therefore, further investigations are warranted to explore the impact of integrating DNA methylomics data into PRS models for enhancing and predicting the development of complex human diseases.
Epigenetic modifications are widely recognized as influential factors in the biological pathways of both communicable diseases and non-communicable complex human diseases like hypertension and cancer with DNA methylation being the most extensively studied. Epigenetics involves the alteration of gene expression without changing the genetic code through processes such as DNA methylation and histone modification. This procedure entails attaching covalently a methyl group to the cytosine base found within sections containing repeated cytosine-guanine bonds, also referred to as CpG islands. When a gene undergoes heavy methylation, it typically remains transcriptionally silent (Irizarry et al., 2009). Environmental factors can trigger significant changes in methylation levels. The methylation patterns found in promoter CpG islands, which are clusters of CpG sites located in gene promoters, hold significant promise as potential biomarkers. They could play crucial roles in disease detection, disease classification, prognosis, and forecasting treatment responses (Ehrlich, 2019).
Recent research has revealed compelling links between DNA methylation and fluctuations in blood pressure, cardiovascular ailments, and various other non-communicable diseases. Han et al highlighted the pivotal role of gene-specific DNA methylation in elevating blood pressure, notably concerning factors like angiotensin-converting enzyme, lipid and amino acid metabolism, and impaired glucose metabolism (Han et al., 2016). Richard et al. pinpointed 13 replicated CpG sites, explaining 1.4% and 2.0% of individual differences in systolic and diastolic blood pressure, respectively (Richard et al., 2017). Intriguingly, new findings propose a strong link between DNA methylation and lifestyle choices (like smoking, alcohol intake, and diet), aging, obesity, and gender—all vital risk factors for hypertension. Kim et al. identified an association between DNA methylation in peripheral blood leukocytes and hypertension prevalence hints at the potential of DNA methylation as a high blood pressure biomarker (Kim et al., 2010). This highlights the promise of integrating DNA methylomics data into models to significantly enhance PRS performance.
The most common bioinformatics and computational tools for multi-omics risk prediction of complex human diseased
Constructing multi-omics risk scores for complex human diseases typically involves integrating multiple layers of biological data (genomics, methylomics, metagenomics, transcriptomics, proteomics and metabolomics) into a single predictive score that quantifies disease risk. Bioinformatics and computational tools for construction multi-omics risk scores use various statistical, machine learning, and feature selection techniques to identify predictive markers across omics layers and combine them into a single aggregated risk score. Table 1 summarizes the most commonly used analytical tools for multi-omics data integration. By combining these bioinformatics and computational tools and frameworks, researchers can construct multi-omics risk scores that provide comprehensive, predictive insights into complex human disease susceptibility and progression. Ultimately enhancing our understanding of the molecular basis of these complex diseases by leveraging complementary information across multi-omics data.
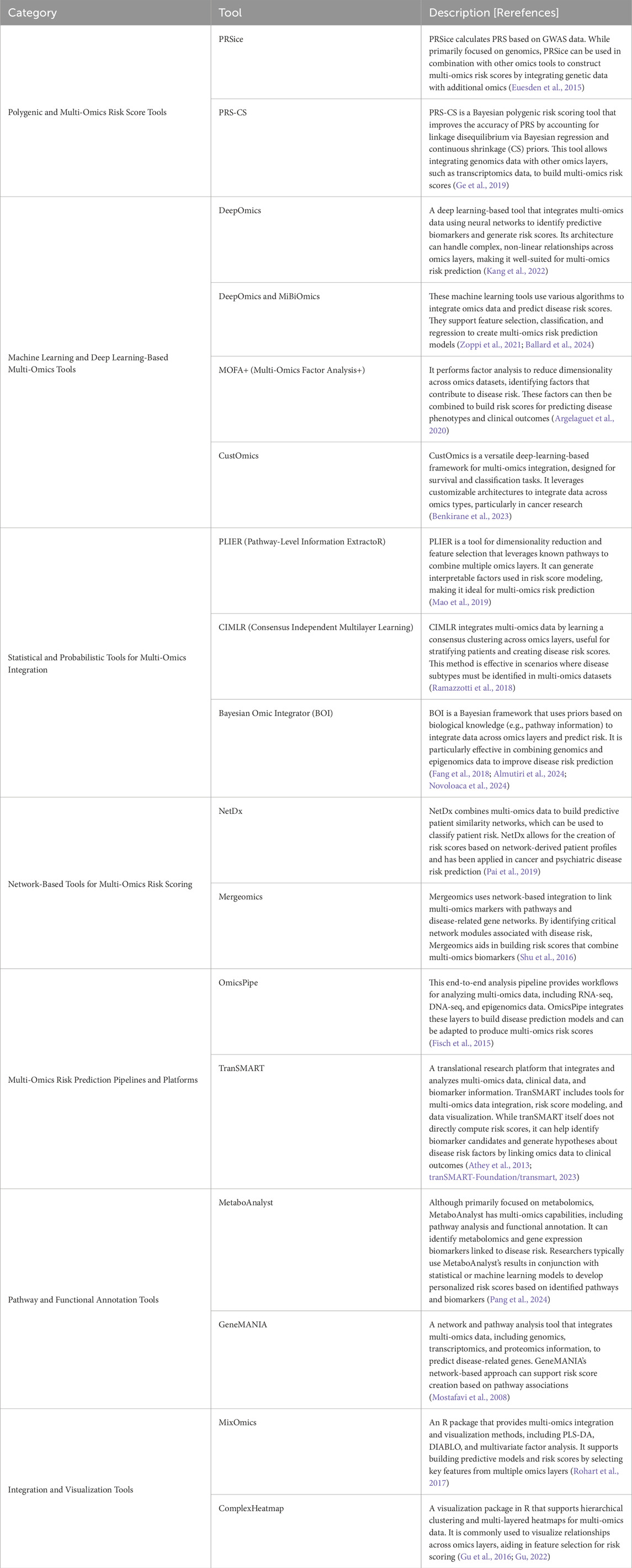
Table 1. The most common bioinformatics and computational tools for multi-omics risk prediction of human complex diseased.
Challenges, limitations and future research direction
The main challenges and limitations for multi-omics utility for improving prediction of complex human diseases are underrepresentation of diverse population in the genome databases, due to the fact that collecting multi-omics data is challenging due to the time consuming and costly process, particularly from large scale human genetic studies. As a result, multi-omics data may only be available for a subset of participants in a study limiting its statistical power, generalizability and hence transferability (Hasin et al., 2017; Chen and Han, 2023). Furthermore, lack of expertise among clinicians and biological scientists particularly from low income countries slows down the process to realize its clinical utility of multi-omics for all. Therefore, the concerted efforts are needed for establishment of multi-omics databases, as well as fostering training among biological scientists in health and life sciences in underserved areas.
Furthermore, several key limitations affect the reliability and effectiveness of multi-omics technologies. Batch effects are a significant challenge in omics data analysis, particularly in large-scale studies where samples are processed in batches or over extended periods. These technical variations between experimental runs can greatly impact data quality. Batch effects in omics studies refer to systematic differences in data caused by variations in experimental conditions across different batches of samples. These differences are unrelated to the biological variables or phenomena being studied and, if not properly addressed, can lead to misleading conclusions. Batch effects can arise from issues related to study design, such as flawed or confounded experimental setups or treatment effects. Variations in sample preparation and storage, including differences in protocols, reagents, equipment, storage conditions, operators, or laboratories, can also contribute to batch effects. Technical variations, such as inconsistencies in laboratory equipment, reagents, operators, or protocols across batches, further exacerbate the problem. Temporal variations, which occur when samples are processed at different times, and environmental factors, such as changes in temperature, humidity, or other conditions during sample preparation or analysis, are additional sources of variability (Yu et al., 2024).
High-throughput experiments are particularly prone to batch effects due to variability in data generated by different sequencing machines or mass spectrometry instruments. For example, DNA sequencing platforms may differ in kits, sequencing depth, quality, or laboratory practices. Bulk and single-cell RNA sequencing protocols may vary in terms of laboratory methods, RNA quality, or library size. Similarly, LC-MS-based proteomics and metabolomics can be influenced by differences in instruments, processing order, or laboratory-specific procedures (Yu et al., 2024). Finally, data analysis introduces its own challenges, with variability arising from differences in analysis platforms or pipelines, software tools, reference databases, and the treatment of low-detected or missing values. Addressing these sources of batch effects is critical to ensuring the reliability and reproducibility of multi-omics studies (Yu et al., 2024). Therefore, addressing batch effects is crucial to ensuring the reliability of results in multi-omics studies and minimizing the risk of drawing inaccurate conclusions.
Batch effects are notoriously common technical variations in multi-omics data and can lead to misleading outcomes if not properly addressed or if over-corrected. These effects can obscure true biological signals, create spurious associations, reduce the reproducibility and reliability of studies, and compromise the accuracy of downstream analyses, such as clustering, classification, or biomarker discovery. To address this challenge, several batch-effect correction algorithms have been developed to facilitate multi-omics data integration. However, their respective advantages and limitations must be thoroughly evaluated based on the type of omics data, performance metrics, and specific application scenarios before selecting an appropriate method for use (Ugidos et al., 2022; Yu et al., 2023; 2024). Assessing and mitigating batch effects is crucial to ensuring the reliability and reproducibility of omics data while minimizing the impact of technical variation on biological interpretation. As multi-omics data continue to expand, the importance of robust experimental design, optimized pipelines, and effective batch-effect correction algorithms is expected to grow, becoming central to large-scale research and clinical applications. The review by Yu et al. provides detailed insights into the sources, diagnostics, visualization techniques, and potential solutions for addressing batch effects in large-scale omics studies, including an overview of the currently available batch-effect correction algorithms (Yu et al., 2024). Reproducibility between individual studies is another significant concern, as experimental conditions can vary across studies, potentially leading to inconsistent results (Yu et al., 2023; Yu et al., 2024).
Additionally, certain omics types, such as transcriptomics, are particularly sensitive to sample extraction and preservation methods, which can introduce variability. Moreover, differences in platform technologies for the same omics type can further contribute to variability. For instance, DNA methylation analysis can be performed using a variety of BeadChip microarrays for detecting human DNA methylation. These include the HumanMethylation27 BeadChip (27K, which covers approximately 27,000 CpGs), the HumanMethylation450 BeadChip (450K, measuring over 485,000 CpGs), the HumanMethylationEPIC BeadChip (EPICv1 or 850K, measuring over 850,000 CpGs), and the HumanMethylationEPIC v2.0 BeadChip (EPICv2 or 900K, measuring over 900,000 CpGs (Lussier et al., 2024) as well as the gold standard, whole-genome bisulfite sequencing (WGBS), each offering different levels of resolution and coverage (Graw et al., 2021). Similarly, metabolomics can vary significantly depending on whether it is targeted or untargeted, presenting challenges in comparability. Lastly, there is the issue of interpreting absolute versus relative measures of risk, which complicates the translation of findings into practical applications (Canzler et al., 2020).
Furthermore, additional challenges include data complexity as integrating diverse data types with distinct scales, noise and missing values is challenging and requires advanced statistical and computational techniques, sophisticated normalization and imputation methods. Computational requirements are also a challenge as multi-omics data integration is computationally intensive, requiring high performance computing resources and efficient algorithms. Lastly, interpretability due to the complexity of multi-omics models that makes them challenging to interpret, and limiting their utility in clinical settings. The opportunity and future research direction of multi-omics risk prediction hinge at the fact that integrating multi-omics data within a systems biology framework fosters researchers to model pathways and networks that drive diseases, leading to insight into causative mechanisms and potential therapeutic target. Multi-omics risk prediction has the potential to tailor interventions based on an individual’s molecular profile, personalized medicine, optimizing prevention strategies and improving outcomes. This can be used in population preventive screening to identify high-risk individual for early intervention, particularly for diseases which early detection is critical such as cancer, cardiovascular diseases, diabetes mellitus, etc. Furthermore, the multi-omics has the potential to be used in understanding disease mechanisms by identifying the key molecular drivers of disease, it can highlight new therapeutic targets and inform drug development. Multi-omics risk prediction holds the promise for enhancing precision in complex human diseases risk assessment, with the potential to move to healthcare towards more individualized, proactive care. As methodologies and computational tools continue to advance, multi-omics integration will likely play an increasingly pivotal role in predicting, preventing and managing complex human diseases.
Conclusion
In conclusion, human complex diseases arise from a complex interplay between the host genetics, host behaviors or lifestyle, and environmental factors. Integrating multi-omics data—such as metagenomics, epigenomics, particularly DNA methylomics, and transcriptomics data—and conventional risk factors into risk score models holds the potential for achieving the highest predictive performance for age-related complex human diseases compared to models based solely on PRS. Further research investigating the integration of multi-omics data is warranted to enhance PRS performance in predicting complex human diseases.
Author contributions
BK: Conceptualization, Writing–original draft, Writing–review and editing. GM: Conceptualization, Writing–original draft, Writing–review and editing.
Funding
The author(s) declare that no financial support was received for the research, authorship, and/or publication of this article.
Acknowledgments
The authors would like to acknowledge the Human Heredity and Health Africa Bioinformatics Network (H3ABioNet) for the training that significantly helped them to conceptualize this work.
Conflict of interest
The authors declare that the research was conducted in the absence of any commercial or financial relationships that could be construed as a potential conflict of interest.
Generative AI statement
The author(s) declare that no Generative AI was used in the creation of this manuscript.
Publisher’s note
All claims expressed in this article are solely those of the authors and do not necessarily represent those of their affiliated organizations, or those of the publisher, the editors and the reviewers. Any product that may be evaluated in this article, or claim that may be made by its manufacturer, is not guaranteed or endorsed by the publisher.
References
Almutiri, T. M., Alomar, K. H., and Alganmi, N. A. (2024). Integrating multi-omics using bayesian ridge regression with iterative similarity bagging. Appl. Sci. 14, 5660. doi:10.3390/app14135660
Argelaguet, R., Arnol, D., Bredikhin, D., Deloro, Y., Velten, B., Marioni, J. C., et al. (2020). MOFA+: a statistical framework for comprehensive integration of multi-modal single-cell data. Genome Biol. 21, 111. doi:10.1186/s13059-020-02015-1
Athey, B. D., Braxenthaler, M., Haas, M., and Guo, Y. (2013). tranSMART: an open source and community-driven informatics and data sharing platform for clinical and translational research. AMIA Jt. Summits Transl. Sci. Proc. 2013, 6–8.
Ballard, J. L., Wang, Z., Li, W., Shen, L., and Long, Q. (2024). Deep learning-based approaches for multi-omics data integration and analysis. BioData Min. 17, 38. doi:10.1186/s13040-024-00391-z
Benkirane, H., Pradat, Y., Michiels, S., and Cournède, P.-H. (2023). CustOmics: a versatile deep-learning based strategy for multi-omics integration. PLoS Comput. Biol. 19, e1010921. doi:10.1371/journal.pcbi.1010921
Bick, A. G., Metcalf, G. A., Mayo, K. R., Lichtenstein, L., Rura, S., Carroll, R. J., et al. (2024). Genomic data in the all of us research Program. Nature 627, 340–346. doi:10.1038/s41586-023-06957-x
Cappozzo, A., McCrory, C., Robinson, O., Freni Sterrantino, A., Sacerdote, C., Krogh, V., et al. (2022). A blood DNA methylation biomarker for predicting short-term risk of cardiovascular events. Clin. Epigenetics 14, 121. doi:10.1186/s13148-022-01341-4
Canzler, S., Schor, J., Busch, W., Schubert, K., Rolle-Kampczyk, U. E., Seitz, H., et al. (2020). Prospects and challenges of multi-omics data integration in toxicology. Arch. Toxicol. 94 (2), 371–388. doi:10.1007/s00204-020-02656-y
Chen, C., and Han, L. (2023). Multi-omic genetic scores advance disease research. Trends Genet. 39, 600–601. doi:10.1016/j.tig.2023.05.002
Chen, H., Ma, Y., Xu, J., Wang, W., Lu, H., Quan, C., et al. (2024). Circulating microbiome DNA as biomarkers for early diagnosis and recurrence of lung cancer. Cell Rep. Med. 5, 101499. doi:10.1016/j.xcrm.2024.101499
Choi, S. W., Mak, T. S.-H., and O’Reilly, P. F. (2020). Tutorial: a guide to performing polygenic risk score analyses. Nat. Protoc. 15, 2759–2772. doi:10.1038/s41596-020-0353-1
Ehrlich, M. (2019). DNA hypermethylation in disease: mechanisms and clinical relevance. Epigenetics 14, 1141–1163. doi:10.1080/15592294.2019.1638701
Euesden, J., Lewis, C. M., and O’Reilly, P. F. (2015). PRSice: polygenic risk score software. Bioinformatics 31, 1466–1468. doi:10.1093/bioinformatics/btu848
Fang, Z., Ma, T., Tang, G., Zhu, L., Yan, Q., Wang, T., et al. (2018). Bayesian integrative model for multi-omics data with missingness. Bioinformatics 34, 3801–3808. doi:10.1093/bioinformatics/bty775
Fisch, K. M., Meißner, T., Gioia, L., Ducom, J.-C., Carland, T. M., Loguercio, S., et al. (2015). Omics Pipe: a community-based framework for reproducible multi-omics data analysis. Bioinformatics 31, 1724–1728. doi:10.1093/bioinformatics/btv061
Ge, T., Chen, C.-Y., Ni, Y., Feng, Y.-C. A., and Smoller, J. W. (2019). Polygenic prediction via Bayesian regression and continuous shrinkage priors. Nat. Commun. 10, 1776. doi:10.1038/s41467-019-09718-5
Graw, S., Chappell, K., Washam, C. L., Gies, A., Bird, J., Robeson, M. S., et al. (2021). Multi-omics data integration considerations and study design for biological systems and disease. Mol. Omics 17 (2), 170–185. doi:10.1039/d0mo00041h
Gu, Z., Eils, R., and Schlesner, M. (2016). Complex heatmaps reveal patterns and correlations in multidimensional genomic data. Bioinformatics 32, 2847–2849. doi:10.1093/bioinformatics/btw313
Han, L., Liu, Y., Duan, S., Perry, B., Li, W., and He, Y. (2016). DNA methylation and hypertension: emerging evidence and challenges. Briefings Funct. Genomics 15, 460–469. doi:10.1093/bfgp/elw014
Hasin, Y., Seldin, M., and Lusis, A. (2017). Multi-omics approaches to disease. Genome Biol. 18, 83. doi:10.1186/s13059-017-1215-1
Huang, Z., Huang, X., Huang, Y., Liang, K., Chen, L., Zhong, C., et al. (2024). Identification of KRAS mutation-associated gut microbiota in colorectal cancer and construction of predictive machine learning model. Microbiol. Spectr. 12, e0272023. doi:10.1128/spectrum.02720-23
Irizarry, R. A., Ladd-Acosta, C., Wen, B., Wu, Z., Montano, C., Onyango, P., et al. (2009). The human colon cancer methylome shows similar hypo- and hypermethylation at conserved tissue-specific CpG island shores. Nat. Genet. 41, 178–186. doi:10.1038/ng.298
Kang, M., Ko, E., and Mersha, T. B. (2022). A roadmap for multi-omics data integration using deep learning. Brief. Bioinform 23, bbab454. doi:10.1093/bib/bbab454
Kim, M., Long, T. I., Arakawa, K., Wang, R., Yu, M. C., and Laird, P. W. (2010). DNA methylation as a biomarker for cardiovascular disease risk. PLoS One 5, e9692. doi:10.1371/journal.pone.0009692
Levine, M. E., Lu, A. T., Quach, A., Chen, B. H., Assimes, T. L., Bandinelli, S., et al. (2018). An epigenetic biomarker of aging for lifespan and healthspan. Aging (Albany NY) 10, 573–591. doi:10.18632/aging.101414
Liu, Y., Ritchie, S. C., Teo, S. M., Ruuskanen, M. O., Kambur, O., Zhu, Q., et al. (2024). Integration of polygenic and gut metagenomic risk prediction for common diseases. Nat. Aging 4, 584–594. doi:10.1038/s43587-024-00590-7
Lussier, A. A., Schuurmans, I. K., Großbach, A., Maclsaac, J., Dever, K., Koen, N., et al. (2024). Technical variability across the 450K, EPICv1, and EPICv2 DNA methylation arrays: lessons learned for clinical and longitudinal studies. Clin. Epigenetics 16, 166. doi:10.1186/s13148-024-01761-4
Mao, W., Zaslavsky, E., Hartmann, B. M., Sealfon, S. C., and Chikina, M. (2019). Pathway-level information extractor (PLIER) for gene expression data. Nat. Methods 16, 607–610. doi:10.1038/s41592-019-0456-1
Martin, A. R., Kanai, M., Kamatani, Y., Okada, Y., Neale, B. M., and Daly, M. J. (2019). Clinical use of current polygenic risk scores may exacerbate health disparities. Nat. Genet. 51, 584–591. doi:10.1038/s41588-019-0379-x
Mostafavi, S., Ray, D., Warde-Farley, D., Grouios, C., and Morris, Q. (2008). GeneMANIA: a real-time multiple association network integration algorithm for predicting gene function. Genome Biol. 9, S4. doi:10.1186/gb-2008-9-s1-s4
Novoloaca, A., Broc, C., Beloeil, L., Yu, W.-H., and Becker, J. (2024). Comparative analysis of integrative classification methods for multi-omics data. Briefings Bioinforma. 25, bbae331. doi:10.1093/bib/bbae331
Pai, S., Hui, S., Isserlin, R., Shah, M. A., Kaka, H., and Bader, G. D. (2019). netDx: interpretable patient classification using integrated patient similarity networks. Mol. Syst. Biol. 15, e8497. doi:10.15252/msb.20188497
Pang, Z., Lu, Y., Zhou, G., Hui, F., Xu, L., Viau, C., et al. (2024). MetaboAnalyst 6.0: towards a unified platform for metabolomics data processing, analysis and interpretation. Nucleic Acids Res. 52, W398–W406. doi:10.1093/nar/gkae253
Ramazzotti, D., Lal, A., Wang, B., Batzoglou, S., and Sidow, A. (2018). Multi-omic tumor data reveal diversity of molecular mechanisms that correlate with survival. Nat. Commun. 9, 4453. doi:10.1038/s41467-018-06921-8
Richard, M. A., Huan, T., Ligthart, S., Gondalia, R., Jhun, M. A., Brody, J. A., et al. (2017). DNA methylation analysis identifies loci for blood pressure regulation. Am. J. Hum. Genet. 101, 888–902. doi:10.1016/j.ajhg.2017.09.028
Rohart, F., Gautier, B., Singh, A., and Cao, K.-A. L. (2017). mixOmics: an R package for ‘omics feature selection and multiple data integration. PLoS Comput. Biol. 13, e1005752. doi:10.1371/journal.pcbi.1005752
Shu, L., Zhao, Y., Kurt, Z., Byars, S. G., Tukiainen, T., Kettunen, J., et al. (2016). Mergeomics: multidimensional data integration to identify pathogenic perturbations to biological systems. BMC Genomics 17, 874. doi:10.1186/s12864-016-3198-9
Thompson, M., Hill, B. L., Rakocz, N., Chiang, J. N., Geschwind, D., Sankararaman, S., et al. (2022). Methylation risk scores are associated with a collection of phenotypes within electronic health record systems. npj Genom. Med. 7, 50–11. Available at: https://github.com/tranSMART-Foundation/transmart (Accessed November 17, 2024). doi:10.1038/s41525-022-00320-1
tranSMART-Foundation/transmart (2023). Dataset. Available at: https://github.com/tranSMART-Foundation/transmart.
Ugidos, M., Nueda, M. J., Prats-Montalbán, J. M., Ferrer, A., Conesa, A., and Tarazona, S. (2022). MultiBaC: an R package to remove batch effects in multi-omic experiments. Bioinformatics 38, 2657–2658. doi:10.1093/bioinformatics/btac132
Wu, C., Zhu, J., King, A., Tong, X., Lu, Q., Park, J. Y., et al. (2021). Novel strategy for disease risk prediction incorporating predicted gene expression and DNA methylation data: a multi-phased study of prostate cancer. Cancer Commun. 41, 1387–1397. doi:10.1002/cac2.12205
Yu, Y., Mai, Y., Zheng, Y., and Shi, L. (2024). Assessing and mitigating batch effects in large-scale omics studies. Genome Biol. 25, 254. doi:10.1186/s13059-024-03401-9
Yu, Y., Zhang, N., Mai, Y., Ren, L., Chen, Q., Cao, Z., et al. (2023). Correcting batch effects in large-scale multiomics studies using a reference-material-based ratio method. Genome Biol. 24, 201. doi:10.1186/s13059-023-03047-z
Keywords: complex human disease prediction, multi-omics risk score, polygenic risk score (PRS), multi-omics, trait prediction
Citation: Kidenya BR and Mboowa G (2024) Unlocking the future of complex human diseases prediction: multi-omics risk score breakthrough. Front. Bioinform. 4:1510352. doi: 10.3389/fbinf.2024.1510352
Received: 12 October 2024; Accepted: 29 November 2024;
Published: 16 December 2024.
Edited by:
Matthew Bashton, Northumbria University, United KingdomReviewed by:
Chen Yao, National Institutes of Health (NIH), United StatesGiovanni Fiorito, IRCCS Giannina Gaslini Institute, Italy
Copyright © 2024 Kidenya and Mboowa. This is an open-access article distributed under the terms of the Creative Commons Attribution License (CC BY). The use, distribution or reproduction in other forums is permitted, provided the original author(s) and the copyright owner(s) are credited and that the original publication in this journal is cited, in accordance with accepted academic practice. No use, distribution or reproduction is permitted which does not comply with these terms.
*Correspondence: Benson R. Kidenya, YmVua2lkZW55YUBnbWFpbC5jb20=
†These authors have contributed equally to this work