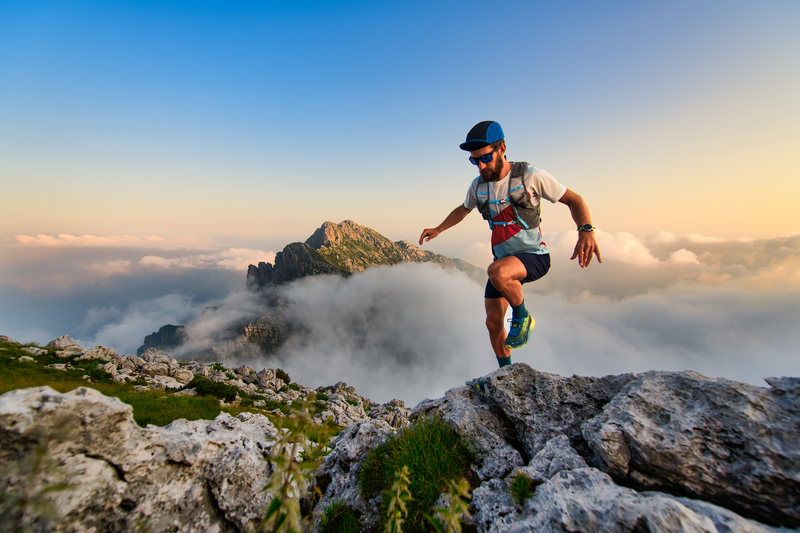
94% of researchers rate our articles as excellent or good
Learn more about the work of our research integrity team to safeguard the quality of each article we publish.
Find out more
BRIEF RESEARCH REPORT article
Front. Bioinform. , 29 January 2025
Sec. Computational BioImaging
Volume 4 - 2024 | https://doi.org/10.3389/fbinf.2024.1497539
In recent years, the exploitation of three-dimensional (3D) data in deep learning has gained momentum despite its inherent challenges. The necessity of 3D approaches arises from the limitations of two-dimensional (2D) techniques when applied to 3D data due to the lack of global context. A critical task in medical and microscopy 3D image analysis is instance segmentation, which is inherently complex due to the need for accurately identifying and segmenting multiple object instances in an image. Here, we introduce a 3D adaptation of the Mask R-CNN, a powerful end-to-end network designed for instance segmentation. Our implementation adapts a widely used 2D TensorFlow Mask R-CNN by developing custom TensorFlow operations for 3D Non-Max Suppression and 3D Crop And Resize, facilitating efficient training and inference on 3D data. We validate our 3D Mask R-CNN on two experiences. The first experience uses a controlled environment of synthetic data with instances exhibiting a wide range of anisotropy and noise. Our model achieves good results while illustrating the limit of the 3D Mask R-CNN for the noisiest objects. Second, applying it to real-world data involving cell instance segmentation during the morphogenesis of the ascidian embryo Phallusia mammillata, we show that our 3D Mask R-CNN outperforms the state-of-the-art method, achieving high recall and precision scores. The model preserves cell connectivity, which is crucial for applications in quantitative study. Our implementation is open source, ensuring reproducibility and facilitating further research in 3D deep learning.
In recent years, we have observed a growing interest in directly exploiting three-dimensional (3D) data in deep learning despite the challenges it presents (Yang et al., 2024). These challenges include the cost of data acquisition, annotation, and storage, as well as the need for substantial computing power for manipulating the volume of data. Many reasons concur with this development, such as the increasing availability of sufficiently voluminous 3D datasets for learning and the trivialization of adequate computing resources. However, the primary reason is that the real world evolves in three dimensions (Ahmed et al., 2018). Two-dimensional (2D) approaches applied to 3D data limit the learning performance because they lack the global context of the whole image. This is, in particular, the case for the voxel images that are used in medical or microscopy imaging, in which 3D deep learning has found numerous applications (Singh et al., 2020; Liu et al., 2021) to replace or complete 2D approaches (von Chamier et al., 2021).
One important but still challenging task that 3D deep learning offers to solve is instance segmentation in these types of images. Instance segmentation is already considered as the most complicated task in 2D deep learning (Hafiz and Bhat, 2020). Its difficulty is inherited from object detection (Girshick et al., 2014). Classical end-to-end supervised learning assumes there is a one-to-one mapping between the input and the ground truth output (Shalev-Shwartz and Ben-David, 2014). However, there exist
Here, we introduce this missing piece, which is the 3D Mask R-CNN network. Mask R-CNN is a powerful region-based end-to-end network that detects and segments many classes, up to 80, in its 2D application on the COCO dataset (He et al., 2017; Lin et al., 2014). It relies on the region-based paradigm (Girshick, 2015; Ren et al., 2017), which consists of first individualizing the instance regions of interest (RoIs) and then segmenting the object within, in contrast to a segment-first method.
We base our implementation on a well-known and widely used two-dimensional TensorFlow implementation (Abdulla, 2017), and we adapt all its operations to process 3D input tensors. In particular, to benefit from TensorFlow core execution speed, we propose the 3D Non Max Suppression and 3D Crop And Resize algorithms as TensorFlow custom operations because native algorithms only apply to 2D images or features. This adaptation allows the delivery of a truly end-to-end implementation with acceptable training and inference time.
We validate our 3D Mask R-CNN with two experiences. The first one involves training the network within a controlled environment that consists of noisy images of objects that can belong to three classes: cuboid, ellipsoid, and irregular pyramid. The Mask R-CNN then gives good results in the evaluation. Finally, we apply our network in the real case of cell instance segmentation during the morphogenesis of the ascidian Phallusia mammillata (PM) (Guignard et al., 2020a). We show that, although this second case constitutes an extreme regime for the Mask R-CNN, our implementation appears competitive against the state-of-the-art method for 3D instance segmentation in bio-imaging and illustrates itself in feature conservation. We provide the code of our 3D Mask R-CNN on GitHub (David, 2024).
The 3D Mask R-CNN is composed of two successive blocks: an initial Region Proposal Network (RPN) and a second part, often called the Head. The RPN takes the whole image as input, while the Head works on the RoI proposals extracted from the RPN feature maps.
The goal of the RPN is to detect objects in the input image without regard to their specific classes. To achieve this, it uses a system of predefined RoI proposals, also called anchors (Lin et al., 2017). From these anchors projected over the input image, the RPN aims (i) to determine which anchors are positive (overlapping with an object in the input) or negative (not overlapping with ground truth instances) and (ii) to predict the adjustments needed for each positive anchor to best match the object bounding boxes. The RPN is composed of a classifier backbone, usually a Residual Network (He et al., 2016) with pyramidal features (Lin et al., 2017), and a special layer that maps each anchor to a vector. These vectors are used by a classifier and a regressor that fulfill the RPN purpose. Due to the large number of anchors, the RPN samples the most relevant anchors, applies the predicted adjustments, and sorts them using a Non Max Suppression (NMS) algorithm to obtain the RoI proposals. These proposals are cropped from the appropriate RPN feature maps and resized to predetermined shapes for the Head modules. The cropping step relies on the RoIAlign approach (He et al., 2017), which samples the feature maps in the normalized space in order to avoid the importance of the quantification effect caused by the classical RoIPooling method.
The Mask R-CNN Head is composed of three branches: the regression, classification, and segmentation modules. The classification and regression modules refine the class and the bounding box predictions from the RPN. Although the RPN only determines if an anchor contains an object regardless of its precise class, the Head classifier identifies the object class within the submitted RoI, including the background class. The Head regressor shares the RPN regressor’s objective of inferring the adjustment to apply to RoI proposals to match the instance. The segmentation module finally decodes the masks of the detected objects from the RoI maps. During training, these three modules are trained simultaneously. Meanwhile, in prediction, the RoI proposals are first submitted to the Head classifier and regressor. The RoIs are then modified and sorted according to the regressor and classifier predictions with an NMS method by class and finally submitted to the segmentation module. An instance segmentation image is thus obtainable at this point.
As the Mask R-CNN uses the TensorFlow NMS and Crop And Resize (CAR) methods, and because we want to benefit from the TensorFlow core execution speed, we implement the 3D NMS and CAR operations as TensorFlow custom operations. Custom operations are C-coded algorithms compiled within TensorFlow sources and callable with the Python API of TensorFlow. In order to build these algorithms, we start from the two-dimensional C implementations available in TensorFlow sources, and we rewrite all functions to manage tensors with one more dimension. In particular, the resizing part of the 2D CAR operation proposes two classical interpolation methods: the nearest neighbor and the bilinear modes. We thus convert the nearest neighbor approach to 3D, and we implement the equivalent of the bilinear interpolation method at 3D, which is the trilinear interpolation method. We deliver safety tests for the 3D NMS and CAR. In order to allow an end-to-end back-propagation during training, we finally link to these operations their exact analytical gradients. The code of the custom operation is open source. We facilitate the portability of our work by adopting a containerized approach.
We validate our Mask R-CNN implementation through two complementary experiences. The first one consists of training our network on a simple controlled environment, called the Toy dataset, whose purpose is to demonstrate that our 3D implementation is fully functional and to test the network limitations in the context of strong anisotropy and considerably weak signal-to-noise ratio. The use of such a synthetic dataset is essential due to the scarcity of 3D public datasets. The second validation illustrates the power of the 3D Mask R-CNN in a real use case, that is, the instance segmentation of PM embryo cells (Guignard et al., 2020b). Embryos show densely connected cells, with strong overlap between ground truth bounding boxes. For memory issues, we only use examples containing fewer than 300 cell instances. The COCO dataset, on which the 2D Mask R-CNN was benchmarked, exhibits roughly 4.5 instances per image on average, against 10 for the Toy dataset. With an average number of instances per image close to 125, the PM embryo dataset constitutes an extreme scenario compared to other datasets and allows us to test other limitations of this region-based method.
As a controlled environment, the Toy dataset is automatically generated owing to well-mastered parameters such as image size, maximum instance number, or object shape. No data preparation or augmentation is hence performed. This dataset contains three object classes, in addition to the background class, allowing validation of all the 3D Mask R-CNN modules. For this paper, we generate 10,000 noised images of
Figure 1. Ground truth training examples for the Toy dataset with cuboids, ellipsoids, and irregular pyramids and for the Phallusia mammillata dataset. The top pictures represent intensity maps of the input signal; strong intensity is displayed in orange. This signal corresponds to the objects in the case of the Toy dataset and the membrane signal in the SPIM fused images. Note that, in the case of the Toy dataset, we generate a special image with a signal-to-noise ratio much higher than the images used for training and validation for the sake of visualization (see implementation for creating original examples). Low intensity, mainly image noise, appears as purple. For display comfort, we eliminate low background values, and we crop the Phallusia mammillata images to exhibit the complexity of the internal structure of the membrane signal. The bottom pictures correspond to the respective instance segmentation of the top pictures: each color corresponds to the mask of one object instance in the input image. We show two developmental stages of the PM1 embryo: an early stage with a low number of cells and an intermediate stage with 250 cells.
The PM dataset consists of
The PM images and segmentations are cropped and resized to the shape
We observe one major bias in the PM dataset: the image containing voluminous cells, which appear at the early stages of the morphogenesis, are under-represented among the cell instances in comparison to the images exhibiting tinier cells, which are found in all development stages of the embryo and especially in the later ones. This imbalance would lead the RPN and the Head modules to under-perform on these images. Therefore, we balance our data according to the volume of the cell bounding boxes.
We select
Our objective is to develop a controlled training procedure that achieves satisfactory results as quickly as possible for both datasets. The fine-tuning of the 3D Mask R-CNN requires weeks or months. To significantly reduce the training time, we employ a two-step training strategy. We initially train the RPN for up to 20 epochs. Subsequently, we freeze the RPN and train the Head modules for 20 epochs. We generate the Head targets using
Among the numerous hyperparameters of the 3D Mask R-CNN, we identify key parameters that significantly affect the network size, training duration, and performance. The two primary hyperparameters impacting memory usage are the number of channels of the RPN and the maximal number of RoIs handled by the Head. Our work is conducted on an IBM Power System AC922 computer equipped with an NVIDIA V100 graphic card featuring 32 GB of memory. This hardware configuration supports up to 256 RPN feature channels and 350 instances per input image. Concerning the network performance, alongside the detection of minimal confidence, the NMS threshold of the RPN influences the sorting of overlapping bounding boxes and the detection of connected objects within the input image. In addition, we observe that the ratio of positive to negative RoIs generated by the RPN during the Head training greatly affects the Head classifier performance and should be chosen carefully.
We validate both experiments using precision, recall, and mean average precision (mAP) scores for an intersection over union (IoU) threshold defined as
The 3D Mask R-CNN trained over the Toy dataset shows fine results on the test subset with a median mAP of
The results of the Mask R-CNN trained over the PM dataset (see Figure 2) show a good median recall score of
Figure 2. The plots on the left gather the 3D Mask R-CNN results, and the images on the right are the 3D Cellpose ones. The top plots represent the precision score against recall, while the bottom ones display the mean instance IoUs against recall. The color scale indicates the number of instances in each image in the test dataset. The Y-axis is shared between the horizontal pairs, and the X-axis is shared between vertical ones. The 3D Mask R-CNN shows better results than 3D Cellpose for precision and mean instance IoUs. The 3D Cellpose outperforms the 3D Mask R-CNN in the recall score case.
Figure 3. Middle-section plots of 3D PM1 signal images and segmentation predictions at various developmental stages. Each row concerns one signal image, whose stage is given by the ground truth cell number in the row title. The first column offers a representation of the SPIM images that we use as inputs for both networks, and the second column corresponds to ground truth ASTEC segmentations of the signal images. The third and fourth columns are the segmentations predicted by the 3D Mask R-CNN and the 3D Cellpose, respectively. The last column represents the cell IoUs for each prediction method in comparison with the ground truth masks. The mean of the distribution is given by a red cross. It consistently falls below the
The IoU score exhibits regular behavior close to its median value
We compare the performance of the 3D Mask R-CNN with the state-of-the-art deep learning method for cell instance segmentation in microscopy images, 3D Cellpose, which is known to perform very well on a variety of microscopy styles as well as species (Stringer et al., 2020; Pachitariu and Stringer, 2022). In contrast with the 3D Mask R-CNN, 3D Cellpose does not rely on the region-based paradigm but on vector fields to delineate cells. 3D Cellpose consists of the application of the 2D Cellpose on all image slices, according to the three-space direction. The predicted 3D instance segmentation results from a fusion step of the three stacks. In the next paragraphs, we compare our predictions to those obtained using the 3D Cellpose pre-trained model called cyto3 because it offers the best results not only in comparison to the other pre-trained model but also to a version of 3D Cellpose retrained over our data (see Supplementary Table S1).
3D Cellpose shows a better overall success than the 3D Mask R-CNN for cell detection (see Figure 2) with a median mAP value of
In Figure 3, we expose the typical segmentation errors of both methods by highlighting them with colored bounding boxes. 3D Cellpose also produces some poor segmentations (green boxes). 3D Cellpose mistakes are mainly false positives (yellow boxes). These errors, almost nonexistent in Mask R-CNN predictions, come from the vector field approach of 3D Cellpose, which can lead to confusion between signal noise and instances.
The high performance of the Mask R-CNN is directly linked to the region-based paradigm on which it relies, which ensures that the false positive detections remain low. The Mask R-CNN also shows a general under-segmentation tendency at instance boundaries, which comes from the mask processing at the RoI level. In consequence, the cell connectivity, while well conserved between correctly segmented cells, does not reach the esthetic of a watershed approach, at least at this point of training. In contrast, the 3D Cellpose approach offers a cell connectivity quality close to ASTEC. However, it predicts many false positive cells. The 3D Cellpose bias is thus to over-segment cells.
Cell instance segmentation of a PM embryo is performed to measure cell instance volume or cell–cell contact surface. This information is important because it directly translates the coupling between genetic expression and morphogenesis regulation (Guignard et al., 2020b). Membrane conservation is thus a key criterion to validate the success of an instance segmentation deep learning method intended to ensure reliable experimental measurements in laboratory use. In order to evaluate the membrane conservation, we first generate the masks of the cell segmentation boundaries predicted by the 3D Mask R-CNN and 3D Cellpose, as well as the ground truth membrane masks given by ASTEC for the test subset examples. Afterward, we count the true positive voxels that correspond to the conserved part of the cell interface between ground truth and prediction (see Figure 4). We find that the average true positive voxel rate for the 3D Mask R-CNN is approximately
Figure 4. Distribution of true positive (blue), false positive (orange), and false negative (red) voxel maps after comparison between 3D Mask R-CNN (top pictures) and 3D Cellpose (bottom pictures) predictions and ground truth cell interface maps of a PM1 example. The left images show a 3D representation of each map to illustrate the global differences between the two tested methods, while an intermediate slice plot of the 3D interface maps is given in the right pictures. Each interface map is generated from the predicted instance segmentation by finding the boundaries of each instance label. The 3D Mask R-CNN conserves more cell interfaces than Cellpose.
The 3D Mask R-CNN demonstrates its reliability and limitations on the Toy dataset and shows promising results for the instance segmentation of cell embryos and for morphogenetic quantitative studies. Its competitiveness against the state-of-the-art method is particularly evident in preserving important features such as instance interfaces. The analysis of segmentation errors reveals certain challenges, including fragmented cell segmentations and boundary divergence between instances due to under-training. Despite these issues, the 3D Mask R-CNN outperforms its competitor, 3D Cellpose, in terms of reproducing gold-standard cell interfaces. This finding highlights the Mask R-CNN as the most promising network for practical applications that require great precision.
The inference time of the 3D Mask R-CNN is relatively long, taking approximately 13 h for 100 time steps, whereas 3D Cellpose offers quicker predictions with a rough inference time of 3.5 h. This difference in speed illustrates the decision that bio-image analysts must make between a method like 3D Cellpose and a region-based approach like the 3D Mask R-CNN. Although 3D Cellpose may provide faster predictions, it may exhibit limited performance on specific usage. On the other hand, despite being slower in both training and inference, the 3D Mask R-CNN possesses the ability to reproduce the ground truth features. This trade-off between speed and accuracy should be carefully considered when choosing an appropriate method for specific bio-imaging analysis tasks.
The datasets presented in this study can be found in online repositories. The names of the repository/repositories and accession number(s) can be found at: 3D Mask R-CNN data: https://figshare.com/articles/dataset/3D_Mask_R-CNN_data/26973085.
GD: writing–original draft, writing–review and editing, investigation, methodology, software, validation, and visualization. EF: writing–review and editing, conceptualization, data curation, funding acquisition, investigation, methodology, project administration, resources, and supervision.
The author(s) declare that financial support was received for the research, authorship, and/or publication of this article. This work was funded by core support from CNRS to EF and by the Cell Whisper project (ANR-19-CE13) to GD.
The authors thank Patrick Lemaire and Killian Biasuz for their discussions and advice throughout this project.
The authors declare that the research was conducted in the absence of any commercial or financial relationships that could be construed as a potential conflict of interest.
All claims expressed in this article are solely those of the authors and do not necessarily represent those of their affiliated organizations, or those of the publisher, the editors and the reviewers. Any product that may be evaluated in this article, or claim that may be made by its manufacturer, is not guaranteed or endorsed by the publisher.
The Supplementary Material for this article can be found online at: https://www.frontiersin.org/articles/10.3389/fbinf.2024.1497539/full#supplementary-material
Abdulla, W. (2017). Mask r-cnn for object detection and instance segmentation on keras and tensorflow. GitHub.
Ahmed, E. M., Saint, A., Shabayek, A. E. R., Cherenkova, K., Das, R., Gusev, G., et al. (2018). A survey on deep learning advances on different 3d data representations. arxiv. doi:10.48550/arxiv.1808.01462
Eschweiler, D., Spina, T. V., Choudhury, R., Meyerowitz, E. M., Cunha, A., and Stegmaier, J. (2019). “Cnn-based preprocessing to optimize watershed-based cell segmentation in 3d confocal microscopy images,” in 2019 IEEE 16th International Symposium on Biomedical Imaging (ISBI 2019), Venice, Italy, 08-11 April 2019 (IEEE). doi:10.1109/isbi.2019.8759242
Girshick, R. (2015). “Fast r-cnn,” in 2015 IEEE International Conference on Computer Vision (ICCV), Santiago, Chile, 07-13 December 2015 (IEEE). doi:10.1109/iccv.2015.169
Girshick, R., Donahue, J., Darrell, T., and Malik, J. (2014). “Rich feature hierarchies for accurate object detection and semantic segmentation,” in 2014 IEEE conference on computer vision and pattern recognition. Columbus, USA, 23-28 June 2014 (IEEE), doi:10.1109/cvpr.2014.81
Guignard, L., Fiúza, U.-M., Leggio, B., Laussu, J., Faure, E., Michelin, G., et al. (2020a). Contact-area dependent cell communications and the morphological invariance of ascidian embryogenesis. doi:10.6084/m9.figshare.c.4529990.v2
Guignard, L., Fiúza, U.-M., Leggio, B., Laussu, J., Faure, E., Michelin, G., et al. (2020b). Contact area–dependent cell communication and the morphological invariance of ascidian embryogenesis. Science 369, eaar5663. doi:10.1126/science.aar5663
Hafiz, A. M., and Bhat, G. M. (2020). A survey on instance segmentation: state of the art. Int. J. Multimedia Inf. Retr. 9, 171–189. doi:10.1007/s13735-020-00195-x
He, K., Gkioxari, G., Dollár, P., and Girshick, R. (2017). “Mask r-cnn,” in 2017 IEEE International Conference on Computer Vision (ICCV), Venice, Italy, 22-29 October 2017 (IEEE). doi:10.1109/iccv.2017.322
He, K., Zhang, X., Ren, S., and Sun, J. (2016). “Deep residual learning for image recognition,” in 2016 IEEE Conference on Computer Vision and Pattern Recognition (CVPR), Las Vegas, USA, 27-30 June 2016 (IEEE). doi:10.1109/cvpr.2016.90
Hirsch, P., Mais, L., and Kainmueller, D. (2020). Patchperpix for instance segmentation. Corr. abs/2001, 07626. doi:10.48550/arXiv.2001.07626
Leggio, B., Laussu, J., Carlier, A., Godin, C., Lemaire, P., and Faure, E. (2019). Morphonet: an interactive online morphological browser to explore complex multi-scale data. Nat. Commun. 10, 2812. doi:10.1038/s41467-019-10668-1
Lin, T.-Y., Dollár, P., Girshick, R., He, K., Hariharan, B., and Belongie, S. (2017). “Feature pyramid networks for object detection,” in 2017 IEEE Conference on Computer Vision and Pattern Recognition (CVPR), Honolulu, USA, 21-26 July 2017 (IEEE). doi:10.1109/cvpr.2017.106
Lin, T.-Y., Maire, M., Belongie, S., Hays, J., Perona, P., Ramanan, D., et al. (2014). Microsoft coco: common objects in context. Comput. Vis. – ECCV 2014, 740–755. doi:10.1007/978-3-319-10602-1_48
Liu, Z., Jin, L., Chen, J., Fang, Q., Ablameyko, S., Yin, Z., et al. (2021). A survey on applications of deep learning in microscopy image analysis. Comput. Biol. Med. 134, 104523. doi:10.1016/j.compbiomed.2021.104523
Pachitariu, M., and Stringer, C. (2022). Cellpose 2.0: how to train your own model. Nat. Methods 19, 1634–1641. doi:10.1038/s41592-022-01663-4
Ren, S., He, K., Girshick, R., and Sun, J. (2017). Faster r-cnn: towards real-time object detection with region proposal networks. IEEE Trans. Pattern Analysis Mach. Intell. 39, 1137–1149. doi:10.1109/tpami.2016.2577031
Shalev-Shwartz, S., and Ben-David, S. (2014). Understanding machine learning: from theory to algorithms. Cambridge University Press.
Singh, S. P., Wang, L., Gupta, S., Goli, H., Padmanabhan, P., and Gulyás, B. (2020). 3d deep learning on medical images: a review. Sensors 20, 5097. doi:10.3390/s20185097
Stringer, C., and Pachitariu, M. (2024). Cellpose3: one-click image restoration for improved cellular segmentation. bioRxiv. doi:10.1101/2024.02.10.579780
Stringer, C., Wang, Y., Michaelos, M., and Pachitariu, M. (2020). Cellpose: a generalist algorithm for cellular segmentation. Nat. Chem. Biol. 18, 100–106. doi:10.1038/s41592-020-01018-x
von Chamier, L., Laine, R. F., Jukkala, J., Spahn, C., Krentzel, D., Nehme, E., et al. (2021). Democratising deep learning for microscopy with zerocostdl4mic. Nat. Commun. 12, 2276. doi:10.1038/s41467-021-22518-0
Wolny, A., Cerrone, L., Vijayan, A., Tofanelli, R., Barro, A. V., Louveaux, M., et al. (2020). Accurate and versatile 3d segmentation of plant tissues at cellular resolution. eLife 9, e57613. doi:10.7554/elife.57613
Keywords: 3D deep learning, instance segmentation, Mask R-CNN, microscopy, Phallusia mammillata, embryos, synthetic dataset, tensorflow
Citation: David G and Faure E (2025) End-to-end 3D instance segmentation of synthetic data and embryo microscopy images with a 3D Mask R-CNN. Front. Bioinform. 4:1497539. doi: 10.3389/fbinf.2024.1497539
Received: 17 September 2024; Accepted: 20 December 2024;
Published: 29 January 2025.
Edited by:
Thomas Pengo, University of Minnesota Twin Cities, United StatesReviewed by:
Christoph Sommer, Institute of Science and Technology Austria (IST Austria), AustriaCopyright © 2025 David and Faure. This is an open-access article distributed under the terms of the Creative Commons Attribution License (CC BY). The use, distribution or reproduction in other forums is permitted, provided the original author(s) and the copyright owner(s) are credited and that the original publication in this journal is cited, in accordance with accepted academic practice. No use, distribution or reproduction is permitted which does not comply with these terms.
*Correspondence: Emmanuel Faure, ZW1tYW51ZWwuZmF1cmVAbGlybW0uZnI=
Disclaimer: All claims expressed in this article are solely those of the authors and do not necessarily represent those of their affiliated organizations, or those of the publisher, the editors and the reviewers. Any product that may be evaluated in this article or claim that may be made by its manufacturer is not guaranteed or endorsed by the publisher.
Research integrity at Frontiers
Learn more about the work of our research integrity team to safeguard the quality of each article we publish.