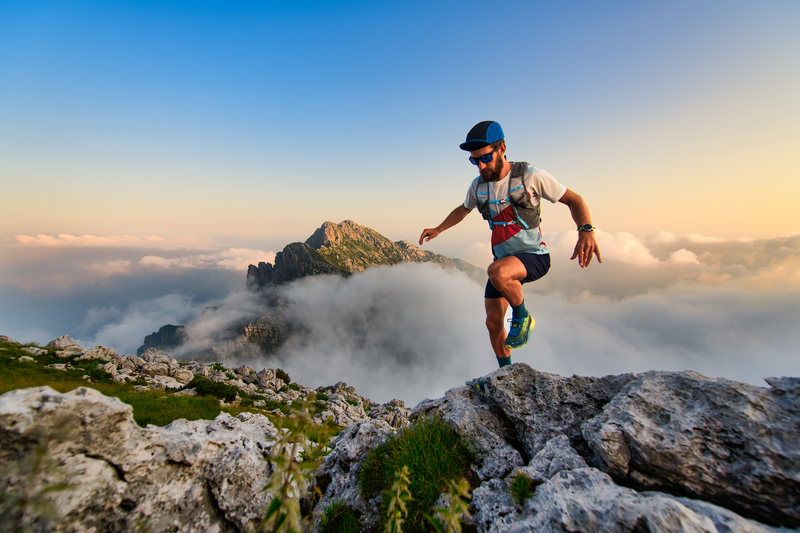
94% of researchers rate our articles as excellent or good
Learn more about the work of our research integrity team to safeguard the quality of each article we publish.
Find out more
ORIGINAL RESEARCH article
Front. Bioinform. , 06 January 2025
Sec. RNA Bioinformatics
Volume 4 - 2024 | https://doi.org/10.3389/fbinf.2024.1493712
Phytoplasma, a potentially hazardous pathogen associated with witches’ broom, is an economically harmful disease-producing bacteria that damages chilli cultivation. Phytoplasma-infected plants display various symptoms that indicate significant disruptions in normal plant physiology and behaviour. Diseases caused by phytoplasma are widespread and have a major economic impact on crop quality and yield. This work focuses on identifying and examining chilli microRNAs (miRNAs) as potential targets against the 16S rRNA and secA gene of “Candidatus Phytoplasma trifolii” (“Ca. P. trifolii”) through plant miRNA prediction algorithms. Mature chilli miRNAs (CA-miRNAs) were collected and used to hybridise the 16S rRNA and secA genes. A total of four common CA-miRNAs were picked according to genetic consensus. Three algorithms applied in the present study suggested that the physiologically relevant, top-ranked miR169b_2 has a possibly specific site at nucleotide position 1,006 for targeting the ‘Ca. P. trifolii’ 16S rRNA gene. The circos algorithm was then utilised to create the miRNA-mRNA regulatory network. The free energy between the miRNA:mRNA duplex was also computed, and the best value of −17.46 kcal/mol was obtained for CA-miR166c_2. Currently, there are no suitable commercial ‘Ca. P. trifolii’-resistant chilli crops. As a result, the expected biological data provide useful evidence for developing ‘Ca. P. trifolii’-resistant chilli plants.
Chilli (Capsicum annuum L.) is a staple vegetable and spice crop, valued for its young green and red ripe fruits. As a medicinal plant, it is known to possess various pharmacologically and biochemically active compounds (Bosland, 1996; Powis et al., 2013). Chilli fruits are attributed to the richness and diversity of bioactive components, including capsaicinoids, carotenoids, and vitamins (Bal et al., 2019b; Bal et al., 2020a; Bal et al., 2020b). Consuming capsaicin in chilli has antioxidant properties and can bind and destroy cancer cells (Oh et al., 2010). Agriculture crops face numerous biotic and abiotic challenges, with phytoplasma-associated diseases being a major concern in many parts of the world. These diseases significantly reduce both production yield and quality. (Bertaccini et al., 2014). Phytoplasma, which causes little leaf disease, is one of the major constraints for chilli production and can result in significant economic losses (Singh and Singh, 2000). Phytoplasmas, which are prokaryotic wall-less bacteria that flourish in isotonic habitats in insect hemolymph and phloem tissues of plants. They possess a small genome, approximately 680–1,600 kb in size. Phytoplasmas are associated with over 600 diverse plant diseases worldwide (Bertaccini et al., 2014). Phloem-feeding insects, specifically leafhoppers and plant hoppers, serve as the principal vectors of phytoplasma transmission (Bertaccini et al., 2014). Phytoplasmas disease are associated with a variety of symptoms, including little leaves, virescence, large buds, shorter internodes, witches’ broom, massive calyx, phyllody, vascular discoloration, and floral abnormalities. The ability to classify phytoplasmas into groups and subgroups was made possible by the development of molecular techniques; this process mostly relied on the examination of the 16S rRNA gene sequence (Lee et al., 1998a; IRPCM, 2004). As the fundamental elements of the Sec translocation protein system, secA, secE, and secY have been found in onion yellow phytoplasma (OY) (Economou, 1999; Kakizawa et al., 2001). They are crucial for both protein movement and cell survival in Escherichia coli. Phytoplasma diseases have existed in India for over a century. Coconut root wilt disease was first observed in South Kerala in 1874 (Varghese, 1934), whereas first phytoplasma disease in chilli was reported in India by Singh and Singh (2000) and ‘Candidatus Phytoplasma trifolii’ causing witches broom disease in chillies was also reported by Rao et al. (2017). According to a recent study, the 16SrVI-D phytoplasma subgroup was associated with Capsicum chinense in India (Dutta et al., 2022).
MicroRNAs (miRNAs) are short (19–25 nucleotide) non-coding, single-stranded RNA molecules that exist naturally in plants and have evolved to be conserved (Finnegan and Matzke, 2003). In higher plants, the synthesis of miRNA gene (MIR) is controlled by RNA polymerase II. The miRNA gene is translated and generates single-standard polycistronic primary transcripts or primary miRNAs. These miRNAs regulate a wide range of biological activities in plants, including gene expression, differentiation, development, cell growth, and host-pathogen interactions (Millar, 2020; Islam et al., 2022). The post-transcriptional gene-silencing (PTGS) process known as miRNA-mediated RNA interference (RNAi) regulates or inhibits viral or non-viral infection by regulating host-virus interactions and providing antimicrobial innate immunity (Jin et al., 2022). Profiling miRNAs in mulberry phloem saps due to phytoplasma infection can help evaluate the molecular mechanisms underlying phytoplasma pathogenicity (Gai et al., 2018). The “Ca. P. trifolii”s’ gene were used as the target binding sites for chilli genome-encoded miRNAs, using a comprehensive multi-network strategy based on “Ca. P. trifolii” infection evaluation.
The major purpose of this study is to discover multiple host-derived miRNA binding sites in the 16S rRNA and secA genes that may be used to create transgenic chilli cultivars resistant to “Ca. P. trifolii”. This study used several miRNA prediction algorithms to detect microRNA-mRNA binding locations in the 16S rRNA and secA gene. These loci may be used to create hybrid/non-hybrid chilli plants resistant to “Ca. P. trifolii” and similar phytoplasma.
To get an in-depth comprehension of phytoplasma plant interactions during infection, it was also interesting to identify relevant targets for the most efficient CA-miRNAs. There have been no investigations on using amiRNA-based techniques to establish phytoplasma resistance in chilli plants, considering its potential for silencing “Ca. P. trifolii”. Further analysis of the anticipated locus-derived CA-miRNAs in the chilli genome was conducted to uncover new antiviral targets and comprehend the complicated relationships between the phytoplasma “Ca. P. trifolii” and the chilli host plants.
The miRNA sRNAanno database was used to retrieve 76 mature chilli microRNAs (CA-miRNAs) that have been experimentally confirmed with high confidence from chr1 to chr5 (Supplementary Table S1). The miRNA targets chosen for this analysis were phytoplasma 16S rRNA (Accession no. MZ557805) and secA (Accession no. MZ620707) gene sequences identified in our previous study of mixed infection in the chilli plant. The sequences were collected from the NCBI GenBank database (Supplementary Figure S1).
Target prediction is a crucial factor in establishing reliable miRNA-mRNA interaction hybridization. Many target prediction algorithms have been used to identify the best miRNA target candidates. Each tool utilizes distinct criteria and methodologies to make predictions. We assessed five target prediction techniques documented in the literature to determine the most relevant CA-miRNAs for phytoplasma components silencing: RNAhybrid (Krüger and Rehmsmeier, 2006), TAPIR (Bonnet et al., 2010), RNA22 (MiRanda et al., 2006; Loher and Rigoutsos, 2012), MiRanda (Enright et al., 2003; John et al., 2004) and psRNATarget (Dai and Zhao, 2011; Dai et al., 2018). These tools calculate complementarity-based miRNA-mRNA binding. An effective computational method was employed to evaluate miRNA targets by examining three different prediction levels: individual, union and intersection (Supplementary Figure S1).
A large number of plant miRNAs bind to their targets with perfect or almost perfect sequence complementarity (Llave et al., 2002; Reinhart et al., 2002). RNAHybrid, an online programme, allows users to identify miRNA targets using mRNA and miRNA minimum free energy (MFE) matching easily. We accepted the default parameters that were specified with hit per target of 1 with MFE threshold of −20 kcal/mol to get the more stable miRNA and mRNA heteroduplex. The Tapirhybrid method evaluates plant miRNAs in the target region for their seed-based interactions. With FASTA and RNAhybrid search capabilities, it is utilised to provide accurate miRNA target predictions, including target mimics. The free energy ratio of 0.2 and score of 9 were selected to increase the accuracy in the result (Table 1). Using RNA22, target locations with appropriate hetero-duplexes was predicted. Among the most delicate algorithmic components are non-seed interactions, pattern detection, MFE, and site compatibility (MiRanda et al., 2006). The study was conducted with sensitivity and specificity of 63% and 61% respectively, the GU region allowed in seed region with no limit and MFE for heteroduplex was −12 kcal/mol for identifying more than 60% accurate and consistent interactions. MiRanda is the most extensively used standard computational approach for predicting miRNA targets (Table 1). The MiRanda method was executed using free energy of −15 kcal/mol and score threshold of 140 led to better alignment and sustained interactions (Table 1). The psRNATarget algorithm, finds that the target phytoplasma components mRNA region and CA-miRNAs are reversely complementary (Dai et al., 2018). Target-site accessibility was evaluated by calculating the unpaired energy (UPE) using the psRNATarget approach. The interaction between miRNA and mRNA was computed using user-specified factors and an expected value cut-off of 5 (Table 1) determining the most probable binding locations while reducing the risk of false positives.
The Circos method was used in the R programme to construct an interaction map between 16S rRNA, secA, and CA-miRNAs (Krzywinski et al., 2009) (Supplementary Figure S1) to enable the detection and study of similarities and differences resulting from miRNA and mRNA interaction. Circos method allows for effective visualisation of sequence alignments, genome mapping, hybridisation arrays, and genotyping experiments (Krzywinski et al., 2009).
Sequence alignment is beneficial in predicting miRNA-mRNA interactions, but the thermodynamic aspects of miRNA-mRNA complexes provide critical information for determining hybridization durability (Riolo et al., 2020). Most miRNA-targeting prediction approaches use the free energy (ΔG) of the expected interaction to assess the thermodynamic characteristics of the miRNA-mRNA complex. RNAcofold, an online tool (http://rna.tbi.univie.ac.at/cgi-bin/RNAWebSuite/RNAcofold.cgi), predicts the duplex (miRNA and mRNA) free energy (ΔG) of interactions (Bernhart et al., 2006). Using the miRNA-target pair from psRNATarget, the necessary 16S rRNA and secA sequences, as well as CA-miRNAs, were studied with the RNAcofold default parameters.
miRNAs with a precise or near-perfect match to their target mRNAs regulate post-transcriptional gene expression through mechanisms such as translation inhibition and cleavage. The microRNA causes mRNA cleavage and subsequent degradation by binding with complementarity in the seed region and base pairing in the central section (Pasquinelli, 2012). This degradation, which is sequence-specific, relies on RNA hydrolysis, leading to effective silence (Dykxhoorn et al., 2003). Limited compatibility, on the other hand, typically results in lower gene expression because it prevents the host from translating the targeted mRNA (Bonnet et al., 2004). This study revealed host miRNAs capable of selectively targeting known phytoplasma 16S rRNA and secA isolates in chilli plants. Because miRNA binding to target RNA genomes is quite diverse, we employed five algorithmic approaches (RNAHybrid, Tapirhybrid, RNA22, MiRanda and psRNATarget) to determine the binding strength and phytoplasma relevance of the 76 known CA-miRNAs (Supplementary Table S1). When numerous in silico approaches were employed to establish target alignment with phytoplasma 16S rRNA and secA phytoplasma components, around 48 target transcripts were identified to be targeted by these 76 known CA-miRNAs (Figure 1). Out of the 76 known miRNAs, three algorithms identified one CA-miRNA (i.e., CA_miR169b_2) (Table 2). RNAHybrid predicted seventeen miRNA targets. Similarly, Tapirhybrid identified six miRNAs that target 16S rRNA. Both RNAHybrid and Tapirhybrid revealed no miRNA with the binding affinity to the secA gene. Furthermore, four miRNAs in RNA22 showed an interaction for their target, each having one target within the 16S rRNA, whereas secA had three target sites (Table 2). Similarly, MiRanda confirmed that both 16S rRNA and secA were targeted by four distinct miRNAs (Table 2). While evaluating the psRNATarget data, we observed ten and four high-probability miRNA binding sites for 16S rRNA and secA, respectively, whereas CA-miR5300_2 targets two different locations in 16S rRNA.
Figure 1. The Venn diagram plot of chilli-encoded miRNAs has been created for all five methods. Chilli-encoded miRNAs target 48 locations on “Ca.P.trifolii” 16S rRNA and secA. Furthermore, the computational tools used in this work confirm the total number of targeting sites for 33 CA-miRNAs that interact with 16S rRNA and secA. Three mathematical approaches (Tapirhybrid, RNA22, and miRanda) predicted the presence of a single CA-miRNA (CA_miR169b_2).
Table 2. List of chilli known miRNA showing target within “Candidatus phytoplasma trifolii” 16S rRNA and secA through a different algorithm.
This analysis found that thirty-four of the seventy-six known CA-miRNA transcripts encoded by chr1 to chr5 had targets in ‘Ca. P. trifolii’ 16S rRNA gene. RNAHybrid showed a total of seventeen miRNA transcripts targeting the 16S rRNA, with CA-miR399 transcripts indicating four targets (Figure 2A). Likewise, tapirhybrid predicted six targeting sites, including two transcripts of CA-miR171 (Figure 2B). In RNA22, four separate miRNA members (i.e., CA-miR6026_1, CA-miR399e_2, CA-miR169b_2, and CA_miR482a_2) targeted the four different prediction sites (Figure 2C). CA-miR403a_2 and CA-miR169b_2 showed cleavage affinity for 16S rRNA in miRanda (Figure 2D). psRNATarget identified ten targeting sites for nine miRNA transcript each targeting one sites except CA-miR5300_2 which individually targeted at two different loci in 16S rRNA (Figure 2E) (Figure 3). MiRanda confirmed CA-miR403a_1 and CA-miR172b_2 as targeting two distinct loci (Figure 3).
Figure 2. The “five algorithms” strategy predicted unique chilli CA-miRNAs and their high-confidence binding regions across Ca.P.trifolii’s 16S rRNA. (A) CA-miRNA binding sites were identified using RNAhybrid. (B) Tapirhybrid reported the target’s CA-miRNA positions and MFE ratio. (C) RNA22 predicts miRNA binding affinity sites. (D) miRanda reported the target’s CA-miRNA spots. (E) psRNATarget indicates CA-miRNA binding sites.
Figure 3. The union plot depicts every predicted binding region identified by each method used. Coloured dots represent numerous copies of the binding spots for miRNA targets by different computational methods.
Bacterial Sec protein transfer involves the secA protein. The translocation of proteins across the cell membrane that is dependent on ATP is mediated by it. According to Xue et al. (2023) secA most likely aids in the survival of phytoplasmas by moving proteins across the cell membrane. We obtained data for the secA gene from three target prediction algorithms. MiRanda, RNA22, and psRNATarget each predicted two, three, and four secA target sites, respectively (Figures 4A–C). In psRNATarget, transcripts of CA-miR159 targeted three of the targeting sites. However, RNA22 predicted three different binding sites by CA_miR319c_2, CA_miR399e_2, and CA_miR482a_1.
Figure 4. The “three algorithms” strategy predicted unique chilli CA-miRNAs and their high-confidence binding regions across ‘Ca.P.trifolii’ secA. (A) RNA22 predicts miRNA binding affinity sites. (B) miRanda reported the target’s CA-miRNA sites. (C) psRNATarget indicates CA-miRNA binding sites along with expectation scores.
The current study was carried out primarily on the consensus of the target binding loci of CA-miRNAs obtained through multiple approaches. We chose four CA-miRNAs, CA-miR169b_2, CA-miR166c_2, CA-miR168a_1, and CA-miR5300_2, considering consensus nucleotide spots 1,006, 533, 906, and 131, respectively (Tables 3) (Figure 5). Only one CA-miRNA, miR169b_2, was identified by combining nucleotide consensus sites at location 1,006 using three approaches (RNA22, TapirHybrid, and MiRanda).
Table 3. High-confidence binding sites for consensus CA-miRNAs that target the 16S rRNA gene of “Candidatus phytoplasma trifolii” that were predicted using several computational approaches.
Figure 5. An intersection graph illustrates the most prevalent CA-miRNAs found using at least twodistinct approaches at homologous loci. Colour codes are provided in the figure showing algorithms.The expected cut-off score (psRNATarget), the minimum free energy (MFE) (RNAhybrid, miRanda, and RNA22), and the MFE ratio (Taphirhybrid) are presented.
To correctly integrate biologically valid data for investigating the miRNA-host gene, we utilised the R-tool to create circos plots for miRNA targets (Table 2). To enable best visualisation and readability, this mapping between the CA-miRNAs with their 16S rRNA and secA gene targets were done (Figure 6).
Figure 6. The “Ca.P.trifolii” 16S rRNA and secA gene schematic representation for the chilli-target interaction. The known CA-miRNAs retrieved from sRNAanno dataset and their targets against the 16S rRNA and secA gene are summarized in a circular plot (Circos) constructed with the R-program. The outer ring represents the genetic components of “Ca. P. trifolii” and known CA-miRNAs. The coloured lines reflect the interaction of both 16S rRNA and secA with the target.
The free energy (ΔG) of miRNA-mRNA duplex for those miRNAs that were supported by at least two predicted tools were evaluated. The miRNA-mRNA complex is thought to be highly thermodynamically stable, with as stronger miRNA-mRNA association when the ΔG of the complex is low (i.e., greater negative ΔG) which strengthens the miRNA’s regulatory influence on the target mRNA (Bernhart et al., 2006). This constitutes essential information because it increases the likelihood that stable miRNA-mRNA binding will be recognized as an actual interaction (Riolo et al., 2020). The RNAcofold algorithm’s free energy (ΔG) estimation was based on the alignment (miRNA-mRNA) result of psRNATarget. Four duplexes were identified, with the lowest free energy (ΔG) of > −15 kcal/mol for CA-miR166c_2, CA-miR166c_2, CA-miR168a_1 and CA-miR168b_2 for 16S rRNA (Table 4). CA-miR6026_1 had the lowest binding energy for secA, which was −12.34 kcal/mol.
Table 4. Duplex free energy (ΔG) of top four known CA-miRNAs, including binding range, length of target, with miRNA alignment:Target duplex.
The sRNAanno database (Chen et al., 2021) was used to predict stable secondary structures for known CA-miRNAs (Figure 7). Precursors for mature CA-miRNAs were retrieved from same database. The secondary structures of four pre-miRNA precursors as predicted by the intersection of two consensus algorithms at the same locus were identified. We identified the important attributes of thirty-three precursor miRNAs that showed targets for either 16S rRNA or secA, including MFE, Adjusted Minimum Folding Free Energy (AMFE), Minimum Folding free Energy Index (MFEI), length precursor, length of mature miRNA, nucleotide and GC content (Figure 8). The MFE is the most important determinant for assessing precursors’ stable secondary structures. According to Bonnet et al. (2004), precursor microRNAs must have less folding energy compared to different non-coding RNAs. The RNAfold tool were used to accessed the MFE value of precursor miRNA (Lorenz et al., 2011). These known CA-miRNAs precursors were found to have lowered MFE values (range from −27.00 to −134.20 kcal/mol) (Table 5). In this work, the precursor length ranged from 116–319 nucleotides (Figure 8), and the (G + C) % varied from 34.9% to 54.8%. The AMFE measured between −26.54 and −49.52 kcal/mol, with an MFEI of −0.58 to −1.24 kcal/mol. Using standard characteristics, the topmost stable secondary structure of precursor was CA-miR6026_1 (MFE: 134.20 kcal/mol, MFEI: 1.16 kcal/mol).
Figure 7. Secondary structure of known CA-miRNAs: those with a greater abundance are coloured red, whereas those with a low abundance are coloured green.
Figure 8. Mature miRNA length and precursor miRNA length were measured. Pink indicates mature miRNAs, whereas blue indicates the length of miRNA precursors. Nucleotide composition of the precursor miRNA was also determined.
Chilli fruit and its supplementary components have significant applications and a diverse range of bioactive chemicals in farming, nourishment, pharmaceuticals, healthcare, and skincare sector. Its by-products are also useful in the field of textile (Havsteen, 2002; Dixon and Pasinetti, 2010; Liu et al., 2013). Aside from its restricted genetic base, chilli revenue is severely affected due to its susceptibility against to biotic and abiotic pressures. Phytoplasmas are non-culturable prokaryotic bacteria responsible for a variety of plant diseases and are spread by insect’s feed on phloem. Chilli is prone to a variety of diseases, among which little leaf disease, caused by phytoplasmas, responsible for major economic losses (Singh and Singh, 2000).
In eukaryotes, microRNAs (miRNAs) are well-conserved, short endogenous non-coding RNAs that use sequence complementarity to target and destroy mRNA. In plant miRNAs often exhibit perfect base-pairing with target sites whereas animal miRNAs establish imperfect duplexes with target sequences, hence confounding the prediction of direct targets (Pasquinelli, 2012). MiRNAs typically suppress target expression in plants and animals by causing mRNA de-adenylation and degradation, as well as limiting translation (Pasquinelli, 2012). Research has explored complex host-virus interactions and employed computational approaches to study miRNAs targeting plant viruses (Akhter and Khan, 2013; Ashraf et al. 2022; 2023; Iqbal et al., 2017; Jabbar et al., 2019; Shahid et al., 2022). In our earlier study, we predicted and examined the mature locus-derived microRNAs in the chilli and papaya genome that were expected to be chilli leaf curl virus (ChiLCV) and papaya leaf curl virus (PaLCuV) targets based on in silico criteria (Pandey et al., 2024; Srivastava et al., 2024).
In this in silico research, we attempted for the first time to align mature chilli CA-miRNAs with the genomic sequence of the 16S rRNA and secA gene of ‘Ca. P. trifolii’ targets in order to identify miRNA-mRNA binding loci hypothesised for comprehending complex host-phytoplasma interactions. The survival of phytoplasma relies on its two primary components, 16S rRNA and sec (A, Y, and E) genes. The 3′end of 16S rRNA interacts with proteins S1 and S21, which are believed to be associated with protein synthesis beginning (Czernilofsky et al., 1975). The 16S rRNA gene is frequently used in phylogenetic investigations (Weisburg et al., 1991) because it is primarily conserved across diverse bacteria and archaea (Coenye and Vandamme, 2003). Similarly, proteins released via the Sec system are anticipated to be crucial throughout the infection process as they facilitate protein translocation. So, this work employs “Ca. P. trifolii” 16S rRNA and secA as CA-miRNA targets, which might be useful for similar phytoplasma sequences.
We investigated the effectiveness of computational strategies for assessing miRNA target prediction data to filter out false-positive outcomes. We developed a reliable method for validating these predictions at the individual, union, and intersection stages. Algorithmic prediction provides quick ways for identifying putative host-derived target regions for miRNA in phytoplasma genomes. The parameters vary depending on the algorithm or tool and may be adjusted to fine-tune the settings or increase the degree of sensitivity for expected spots. Five different approaches were utilised for target prediction: RNAHybrid, Tarpirhybrid, RNA22, miRanda, and psRNATarget. We applied all five approaches to determining the MFE and target inhibition sites.
Two or more algorithms may jointly identify a number of putative CA-miRNAs targets and miRNA-mRNA duplexes (Figure 3). Target gene destruction is induced by plant miRNAs by the application of perfect or near-perfect complementary base pairing (Jones-Rhoades et al., 2006). The present study shows that a collection of consensus CA-miRNAs may target ‘Ca. P. trifolii’ genomic components (16S rRNA and secA gene). Furthermore, three algorithms identified CA-miR169b_2 as targeting 16S rRNA at the same consensus hybridisation site (i.e., 1,006), and because this specific miRNA’s target region was proven by three approaches, more research could be undertaken on it (Figure 5). miR169 is largely conserved across plant species and may be activated by drought and salt environments in rice (Sunkar and Jagadeeswaran, 2008). Free energy estimation is a dynamic characteristic of miRNA and target binding. Previous research has identified a strong link between free energy and both translational repression and seed hybridization binding (Doench and Sharp, 2004). The thermodynamic stability of the miRNA-mRNA heterodimer was assessed using free energy analysis to track site availability for secondary structure duplex identification (Peterson et al., 2014). To validate miRNA-mRNA interactions, we calculated the free energy of the heterodimer (Table 4). Our prediction indicates that the chilli-encoded miRNA-phytoplasma-mRNA duplex is highly stable at low free energy levels (Table 4). The increased stability of the RNA duplex is attributed to the stronger interaction between the miRNA and mRNA (Lewis et al., 2005; Huang et al., 2010).
We applied union and intersection methods to decrease false positive predictions. When it comes to detecting genuine and false targets, union techniques rely on merging many target prediction tools. An intersecting method is fundamentally different, relying on the integration of two or more computational algorithms to increase the specificity of anticipated targets by reducing insensitivity (Witkos et al., 2011). Our target prediction outcomes showed that both computational methods performed optimally when identifying and estimating the optimum targets (Figures 3, 5). Based on the manner of miRNA-target identification, MFE is another significant component that influences miRNA-target interaction during result validation (Pinzón et al., 2017). Setting a lower MFE value increases the possibility of miRNA-target building complexes (Kertesz et al., 2007). For miRanda analysis, a strict cut-off value of −15 kcal/mol was used to filter out miRNA candidates. Similarly, to confirm host-phytoplasma interaction, RNA hybrid analysis was performed with an MFE cut-off value of −20 kcal/mol present investigation, we identified 17 candidate miRNA hybridization binding sites with low MFEs and free energy for duplex formation (Enright et al., 2003). Although MFE plays an important role in the formation of miRNA-mRNA duplexes, it fails to guarantee that interactions result in functional alterations. In the present investigation, we identified 14 candidate miRNA hybridization binding sites with low MFEs and free energy values for duplex formation by using psRNATarget and RNAcofold.
These candidate CA-miRNAs have potential transgenic targets for the 16S rRNA and secA genomes, as well as a greater possibility of forming miRNA-phytoplasma mRNA complexes. We selected best four experimentally confirmed CA-miRNAs with identified high-confidence targets from ‘Ca. P. trifolii’ (Table 3) (i.e., CA-miR169b_2, CA-miR166c_2, CA-miR168a_1 and CA-miR5300_2) and predict their secondary structure through sRNAanno database. The amiRNA-based silencing technique has been effectively proven in numerous agricultural plants for controlling emerging plant pathogens (Niu et al., 2006; Ali et al., 2013; Petchthai et al., 2018).
To the best of our knowledge, this is the first-time known CA-miRNAs have targeted at phytoplasmic components. Our computational study of “Ca. P. trifolii” gene silencing may provide a novel strategy for the creation of anti-phytoplasma agents. Furthermore, we developed a technique for minimising the new anti-phytoplasma impacts of host-derived miRNAs on “Ca. P. trifolii”. The in silico research aimed to provide a basis for experimental validation to determine whether known CA-miRNAs could confer resistance to “Ca. P. trifolii” in plants. The expression of CA-miR169b_2 in transgenic chilli varieties to silence “Ca. P. trifolii” target genes might help us gain insight into crucial host-virus interactions.
In India, phytoplasma has emerged as a major agricultural threat, affecting a wide range of crops and “Ca. P. trifolii” lowers the quantitative production of chilli cultivars. In this study, we used computational techniques to predict and thoroughly investigate possible miRNA from chilli against “Ca. P. trifolii” 16S rRNA and secA gene. The best CA-miRNA for interacting with the “Ca. P. trifolii” was discovered to be miR169b_2. Our findings suggest that miR169b_2 may be a viable and successful treatment strategy for “Ca. P. trifolii” infection in chilli cultivars. Large-scale transgenic chilli cultivar development must be substantiated by pathological implications. As a result, the next challenge will be to find the crucial miR169b_2 targets involved in silencing the “Ca. P. trifolii” genome’s 16S rRNA gene, as well as determining their involvement in a genome-editing-based conversion system. Using chilli transformation procedures, predicted new targets can be created to create “Ca. P. trifolii” -resistant chilli cultivars. Chilli transformation processes can be used to generate expected new objectives for “Ca. P. trifolii” -resistant chilli cultivars.
The original contributions presented in the study are included in the article/Supplementary Material, further inquiries can be directed to the corresponding authors.
VP: Formal Analysis, Methodology, Software, Validation, Writing–original draft. AS: Formal Analysis, Methodology, Software, Validation, Writing–original draft. RGu: Conceptualization, Resources, Visualization, Writing–review and editing. HZ: Data curation, Formal Analysis, Software, Writing–review and editing. MS: Conceptualization, Project administration, Supervision, Validation, Writing–review and editing. RGa: Conceptualization, Investigation, Project administration, Supervision, Writing–review and editing.
The author(s) declare that no financial support was received for the research, authorship, and/or publication of this article.
Author HZ thanks and acknowledges the Department of Research and Consultation at the University of Technology and Applied Sciences-Sur, Oman, for their ongoing support and facilities.
The authors declare that the research was conducted in the absence of any commercial or financial relationships that could be construed as a potential conflict of interest.
All claims expressed in this article are solely those of the authors and do not necessarily represent those of their affiliated organizations, or those of the publisher, the editors and the reviewers. Any product that may be evaluated in this article, or claim that may be made by its manufacturer, is not guaranteed or endorsed by the publisher.
The Supplementary Material for this article can be found online at: https://www.frontiersin.org/articles/10.3389/fbinf.2024.1493712/full#supplementary-material
SUPPLEMENTARY FIGURE S1 | Flow chart showing all computational tools used in this research.
SUPPLEMENTARY TABLE S1 | List of 76 mature known CA-miRNAs retrieved from sRNAanno database, along with its location on chromosomes.
Akhter, Y., and Khan, J. A. (2013). Genome wide identification of cotton (Gossypium hirsutum)-encoded microRNA targets against Cotton leaf curl Burewala. virus gene. 638, 60–65. doi:10.1016/jgene201709061
Ali, I., Amin, I., Briddon, R. W., and Mansoor, S. (2013). Artificial microRNA-mediated resistance against the monopartite begomovirus Cotton leaf curl Burewala virus. Virol. J. 10, 231. doi:10.1186/1743-422X-10-231
Ashraf, M. A., Ali, B., Brown, J. K., Shahid, I., and Yu, N. (2023). In silico identification of cassava genome-encoded MicroRNAs with predicted potential for targeting the ICMV-Kerala begomoviral pathogen of cassava. Viruses 15, 486. doi:10.3390/v15020486
Ashraf, M. A., Tariq, H. K., Hu, X.-W., Khan, J., and Zou, Z. (2022). Computational biology and machine learning approaches identify rubber tree (hevea brasiliensis muell arg) genome encoded MicroRNAs targeting rubber tree virus 1. Appl. Sci. 12, 12908. doi:10.3390/app122412908
Bal, S., Maity, T. K., and Maji, A. (2020a). Genetic divergence studies for yield and quality traits in onion (Allium cepa L). Int. J. Curr. Microbiol. Appl. Sci. 9, 3201–3208. doi:10.20546/ijcmas2020906383
Bal, S., Maity, T. K., and Maji, A. (2020b). Evaluation of onion genotypes for growth, yield and quality traits under gangetic alluvial plains of West Bengal. Int. J. Chem. Stud. 8, 2157–2162. doi:10.22271/chemi2020v8i4x9948
Bal, S., Maity, T. K., Sharangi, A. B., and Majumdar, A. (2019). Quality assessment in association with yield attributes contributing improved yield in onion (Allium cepa L). J. Crop Weed 15, 107–115. doi:10.22271/09746315.2019.v15.i3.1245
Bernhart, S. H., Tafer, H., Mückstein, U., Flamm, C., Stadler, P. F., and Hofacker, I. L. (2006). Partition function and base pairing probabilities of RNA heterodimers. Algorithms Mol. Biol. AMB 1 (1), 3. doi:10.1186/1748-7188-1-3
Bertaccini, A., Duduk, B., Paltrinieri, S., and Contaldo, N. (2014). Phytoplasmas and phytoplasma diseases: a severe threat to agriculture. Am. J. Plant Sci. 5, 1763–1788. doi:10.4236/ajps2014512191
Bonnet, E., He, Y., Billiau, K., and Van de Peer, Y. (2010). TAPIR, a web server for the prediction of plant microRNA targets, including target mimics. Bioinformatics 26, 1566–1568. doi:10.1093/bioinformatics/btq233
Bonnet, E., Wuyts, J., Rouzé, P., and Van de Peer, Y. (2004). Detection of 91 potential conserved plant microRNAs in Arabidopsis thaliana and Oryza sativa identifies important target genes. Proc. Natl. Acad. Sci. U. S. A. 101, 11511–11516. doi:10.1073/pnas0404025101
Bosland, P. W. (1996). Capsicum: innovative uses of an ancient crop in progress in new crops. Arlington, VA, USA: Academic Press, 479–487.
Chen, C., Li, J., Feng, J., Liu, B., Feng, L., Yu, X., et al. (2021). sRNAanno-a database repository of uniformly annotated small RNAs in plants. Hort. Res. 8 (1), 45. doi:10.1038/s41438-021-00480-8
Coenye, T., and Vandamme, P. (2003). Intragenomic heterogeneity between multiple 16S ribosomal RNA operons in sequenced bacterial genomes. FEMS microbio. Let. 228 (1), 45–49. doi:10.1016/S0378-1097(03)00717-1
Czernilofsky, A. P., Kurland, C. G., and Stöffler, G. (1975). 30S ribosomal proteins associated with the 3'-terminus of 16S RNA. FEBS Lett. 58 (1), 281–284. doi:10.1016/0014-5793(75)80279-1
Dai, X., and Zhao, P. X. (2011). psRNATarget: a plant small RNA target analysis server. Nucleic Acids Res. 39, W155–W159. doi:10.1093/nar/gkr319
Dai, X., Zhuang, Z., and Zhao, P. X. (2018). psRNATarget: a plant small RNA target analysis server (2017 release). Nucleic Acids Res. 46, W49–W54. doi:10.1093/nar/gky316
Dixon, R. A., and Pasinetti, G. M. (2010). Flavonoids and iso-flavonoids: from plant biology to agriculture and neuroscience. Plant Physiol. 154, 453–457. doi:10.1104/pp110161430
Doench, J. G., and Sharp, P. A. (2004). Specificity of microRNA target selection in translational repression. Genes and Dev. 18 (5), 504–511. doi:10.1101/gad1184404
Dutta, D. S., Kumar, K. S. D. S., Kalita, M. K., and Nath, P. D. (2022). First report of ‘Candidatus Phytoplasma trifolii’ associated with little leaf disease of Capsicum chinense from the northeast of India. New Dis. Rep. 46, e12115. doi:10.1002/ndr212115
Dykxhoorn, D. M., Novina, C. D., and Sharp, P. A. (2003). Killing the messenger: short RNAs that silence gene expression. Nat. Rev. Mol. Cell Biol. 4 (6), 457–467. doi:10.1038/nrm1129
Economou, A. (1999). Following the leader: bacterial protein export through the Sec pathway. Trends Microbiol. 7 (8), 315–320. doi:10.1016/S0966-842X(99)01555-3
Enright, A., John, B., Gaul, U., Tuschl, T., Sander, C., and Marks, D. (2003). MicroRNA targets in Drosophila. Genome Biol. 5, R1. doi:10.1186/gb-2003-5-1-r1
Finnegan, E. J., and Matzke, M. A. (2003). The small RNA world. J. Cell Sci. 116, 4689–4693. doi:10.1242/jcs00838
Gai, Y. P., Zhao, H. N., Zhao, Y. N., Zhu, B. S., Yuan, S. S., Li, S., et al. (2018). MiRNA-seq-based profiles of miRNAs in mulberry phloem sap provide insight into the pathogenic mechanisms of mulberry yellow dwarf disease. Sci. Res. 8 (1), 812. doi:10.1038/s41598-018-19210-7
Havsteen, B. H. (2002). The biochemistry and medical significance of the flavonoids. Pharmacol. Ther. 96, 67–202. doi:10.1016/s0163-7258(02)00298-x
Huang, Y., Zou, Q., Song, H., Song, F., Wang, L., Zhang, G., et al. (2010). A study of miRNAs targets prediction and experimental validation. Protein and cell 1 (11), 979–986. doi:10.1007/s13238-010-0129-4
Iqbal, M. S., Jabbar, B., Sharif, M. N., Ali, Q., Husnain, T., and Nasir, I. A. (2017). In silico MCMV silencing concludes potential host-derived miRNAsin maize. Front. Plant Sci. 8, 372. doi:10.3389/fpls201700372
IRPCM (2004). ‘Candidatus Phytoplasma’, a taxon for the wall-less, non-helical prokaryotes that colonise plant phloem and insects. Int. J. Syst. Evol. Microbiol. 54, 1243–1255. doi:10.1099/ijs002854-0
Islam, W., Waheed, A., Idrees, A., Rashid, J., and Zeng, F. (2022). Role of plant microRNAs and their corresponding pathways in fluctuating light conditions. Biochim. Biophys. Acta (BBA)-Mol. Cell Res. 1870, 119304. doi:10.1016/jbbamcr2022119304
Jabbar, B., Iqbal, M. S., Batcho, A. A., Nasir, I. A., Rashid, B., Husnain, T., et al. (2019). Target prediction of candidate miRNAs from Oryza sativa for silencing the RYMV genome. Comput. Biol. Chem. 83, 107127. doi:10.1016/jcompbiolchem2019107127
Jin, L., Chen, M., Xiang, M., and Guo, Z. (2022). RNAi-based antiviral innate immunity in plants. Viruses 14, 432. doi:10.3390/v14020432
John, B., Enright, A. J., Aravin, A., Tuschl, T., Sander, C., and Marks, D. S. (2004). Human microRNA targets. PLoS Biol. 2, e363. doi:10.1371/journalpbio0020363
Jones-Rhoades, M. W., Bartel, D. P., and Bartel, B. (2006). MicroRNAs and their regulatory roles in plants. Annu. Rev. Plant Biol. 57, 19–53. doi:10.1146/annurevarplant57032905105218
Kakizawa, S., Oshima, K., Kuboyama, T., Nishigawa, H., Jung, H. y., Sawayanagi, T., et al. (2001). Cloning and expression analysis of phy toplasma protein translocation genes. Mol. Plant Microbe. In. 14 (9), 1043–1050. doi:10.1094/MPMI20011491043
Kertesz, M., Iovino, N., Unnerstall, U., Gaul, U., and Segal, E. (2007). The role of site accessibility in microRNA target recognition. Nat. Genet. 39 (10), 1278–1284. doi:10.1038/ng2135
Krüger, J., and Rehmsmeier, M. (2006). RNAhybrid: microRNA target prediction easy, fast and flexible. Nucleic Acids Res. 34, W451–W454. doi:10.1093/nar/gkl243
Krzywinski, M., Schein, J., Birol, I., Connors, J., Gascoyne, R., Horsman, D., et al. (2009). Circos: an information aesthetic for comparative genomics. Genome Res. 19 (9), 1639–1645. doi:10.1101/gr092759109
Lee, I.-M., Gundersen-Rindal, D., Davis, R. E., and Bartoszyk, I. M. (1998a). Revised classification scheme of phytoplasmas based on RFLP analyses of 16S rRNA and ribosomal protein gene sequences. Int. J. Syst. Bacteriol. 48, 1153–1169. doi:10.1099/00207713-48-4-1153
Lewis, B. P., Burge, C. B., and Bartel, D. P. (2005). Conserved seed pairing, often flanked by adenosines, indicates that thousands of human genes are microRNA targets. Cell 120 (1), 15–20. doi:10.1016/jcell200412035
Liu, S., Li, W., Wu, Y., Chen, C., and Lei, J. (2013). De novo transcriptome assembly in chilli pepper (Capsicum frutescens) to identify genes involved in the biosynthesis of capsaicinoids. PLoS ONE 8, e48156. doi:10.1371/journalpone0048156
Llave, C., Xie, Z., Kasschau, K. D., and Carrington, J. C. (2002). Cleavage of Scarecrow-like mRNA targets directed by a class of Arabidopsis miRNA. Science 297 (5589), 2053–2056. doi:10.1126/science1076311
Loher, P., and Rigoutsos, I. (2012). Interactive exploration of RNA22 microRNA target predictions. Bioinformatics 28, 3322–3323. doi:10.1093/bioinformatics/bts615
Lorenz, R., Bernhart, S. H., Höner Zu Siederdissen, C., Tafer, H., Flamm, C., Stadler, P. F., et al. (2011). ViennaRNA package 20. Algorithms Mol. Biol. AMB 6, 26. doi:10.1186/1748-7188-6-26
Millar, A. A. (2020). The function of miRNAs in plants. Plants 9 (2), 198. doi:10.3390/plants9020198
Miranda, K. C., Huynh, T., Tay, Y., Ang, Y. S., Tam, W. L., Thomson, A. M., et al. (2006). A pattern-based method for the identification of MicroRNA binding sites and their corresponding heteroduplexes. Cell 126 (6), 1203–1217. doi:10.1016/jcell200607031
Niu, Q. W., Lin, S. S., Reyes, J. L., Chen, K. C., Wu, H. W., Yeh, S. D., et al. (2006). Expression of artificial microRNAs in transgenic Arabidopsis thaliana confers virus resistance. Nat. Biotech. 24 (11), 1420–1428. doi:10.1038/nbt1255
Oh, S., Choi, C. H., and Jung, Y. K. (2010). Autophagy induction by capsaicin in malignant human breast cells is modulated by p38 and extracellular signal-regulated mitogen-activated protein kinases and retards cell death by suppressing endoplasmic reticulum stress-mediated apoptosis. Mol. Pharmacol. 78, 114–125. doi:10.1124/mol110063495
Pandey, V., Srivastava, A., Ali, A., Gupta, R., Shahid, M. S., and Gaur, R. K. (2024). Predicting candidate miRNAs for targeting begomovirus to induce sequence-specific gene silencing in chilli plants. Front. plant sci. 15, 1460540. doi:10.3389/fpls.2024.1460540
Pasquinelli, A. (2012). MicroRNAs and their targets: recognition, regulation and an emerging reciprocal relationship. Nat. Rev. Genet. 13, 271–282. doi:10.1038/nrg3162
Petchthai, U., Yee, C. S. L., and Wong, S. M. (2018). Resistance to CymMV and ORSV in artificial microRNA transgenic Nicotiana benthamiana plants. Sci. Rep. 8 (1), 9958. doi:10.1038/s41598-018-28388-9
Peterson, S. M., Thompson, J. A., Ufkin, M. L., Sathyanarayana, P., Liaw, L., and Congdon, C. B. (2014). Common features of microRNA target prediction tools. Front. Genet. 5, 23. doi:10.3389/fgene201400023
Pinzón, N., Li, B., Martinez, L., Sergeeva, A., Presumey, J., Apparailly, F., et al. (2017). microRNA target prediction programs predict many false positives. Genome Res. 27 (2), 234–245. doi:10.1101/gr205146116
Powis, T. G., Gallaga-Murrieta, E., Lesure, R., Lopez-Bravo, R., Grivetti, L., Kucera, H., et al. (2013). Prehispanic use of chili peppers in chiapas, Mexico. PLoS ONE 8, e79013. doi:10.1371/journalpone0079013
Rao, A., Goel, S., Kumar, M., and Gopala and Rao, G. P. (2017). First report of occurrence of Candidatus Phytoplasma trifolii-related strain causing witches’ broom disease of chilli in India. Australas. Plant Dis. Notes 12, 28–34. doi:10.1007/s13314-017-0251-8
Reinhart, B. J., Weinstein, E. G., Rhoades, M. W., Bartel, B., and Bartel, D. P. (2002). MicroRNAs in plants. Genes and Dev. 16 (13), 1616–1626. doi:10.1101/gad1004402
Riolo, G., Cantara, S., Marzocchi, C., and Ricci, C. (2020). miRNA targets: from prediction tools to experimental validation methods protoc. Methods Protoc. 4 (1), 1. doi:10.3390/mps4010001
Shahid, M. N., Rashid, S., Iqbal, M. S., Jamal, A., Khalid, S., and Shamim, Z. (2022). In silico prediction of potential mirnas to target ZYMV in cucumis melo. Pak J. Bot. 54 (18), 1319–1325. doi:10.30848/pjb2022-4(18)
Singh, D., and Singh, J. S. (2000). Chilli little leaf - a new phytoplasma disease in India. Indian phytopathol. 53, 309–310.
Srivastava, A., Pandey, V., Singh, N., Marwal, A., Shahid, M. S., and Gaur, R. K. (2024). In silico identification of papaya genome-encoded microRNAs to target begomovirus genes in papaya leaf curl disease. Front. Microbiol. 15, 1340275. doi:10.3389/fmicb20241340275
Sunkar, R., and Jagadeeswaran, G. (2008). In silico identification of conserved microRNAs in large number of diverse plant species. BMC Plant Biol. 8, 37. doi:10.1186/1471-2229-8-37
Weisburg, W. G., Barns, S. M., Pelletierm, D. A., and Lane, D. J. (1991). 16S ribosomal DNA amplification for phylogenetic study. J. Bacteriol. 173 (2), 697–703. doi:10.1128/jb1732697-7031991
Witkos, T. M., Koscianska, E., and Krzyzosiak, W. J. (2011). Practical aspects of microRNA target prediction. Curr. Mol. Med. 11 (2), 93–109. doi:10.2174/156652411794859250
Keywords: phytoplasma, ‘candidatus phytoplasma trifolii’, chilli, 16S rrna, SecA, miRNA
Citation: Pandey V, Srivastava A, Gupta R, Zaki HEM, Shafiq Shahid M and Gaur RK (2025) In silico identification of chilli genome encoded MicroRNAs targeting the 16S rRNA and secA genes of “Candidatus phytoplasma trifolii”. Front. Bioinform. 4:1493712. doi: 10.3389/fbinf.2024.1493712
Received: 09 September 2024; Accepted: 11 December 2024;
Published: 06 January 2025.
Edited by:
C. N. Lakshminarayana Reddy, University of Agricultural Sciences, IndiaReviewed by:
V. Venkataravanappa, Indian Institute of Horticultural Research (ICAR), IndiaCopyright © 2025 Pandey, Srivastava, Gupta, Zaki, Shafiq Shahid and Gaur. This is an open-access article distributed under the terms of the Creative Commons Attribution License (CC BY). The use, distribution or reproduction in other forums is permitted, provided the original author(s) and the copyright owner(s) are credited and that the original publication in this journal is cited, in accordance with accepted academic practice. No use, distribution or reproduction is permitted which does not comply with these terms.
*Correspondence: Haitham E. M. Zaki, aGFpdGhhbS56YWtpQHV0YXMuZWR1Lm9t; Muhammad Shafiq Shahid, bXNoYWhpZEBzcXUuZWR1LmNvbQ==; Rajarshi K. Gaur, Z2F1cnJhamFyc2hpQGhvdG1haWwuY29t
†ORCID: Muhammad Shafiq Shahid, orcid.org/0000-0002-3550-0000; Rajarshi K. Gaur, orcid.org/0000-0003-4550-0730
‡These authors have contributed equally to this work
Disclaimer: All claims expressed in this article are solely those of the authors and do not necessarily represent those of their affiliated organizations, or those of the publisher, the editors and the reviewers. Any product that may be evaluated in this article or claim that may be made by its manufacturer is not guaranteed or endorsed by the publisher.
Research integrity at Frontiers
Learn more about the work of our research integrity team to safeguard the quality of each article we publish.