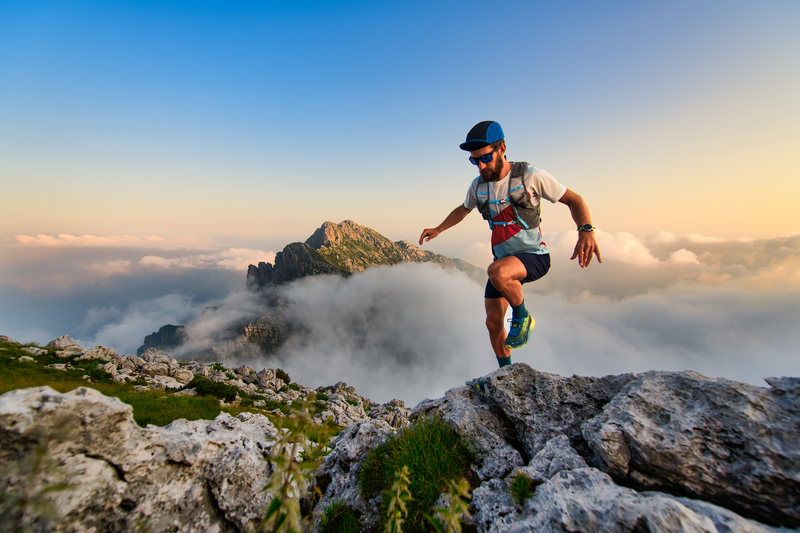
95% of researchers rate our articles as excellent or good
Learn more about the work of our research integrity team to safeguard the quality of each article we publish.
Find out more
EDITORIAL article
Front. Bioinform. , 05 January 2024
Sec. Protein Bioinformatics
Volume 3 - 2023 | https://doi.org/10.3389/fbinf.2023.1338560
This article is part of the Research Topic Expert Opinions in Protein bioinformatics: 2022 View all 5 articles
Editorial on the Research Topic
Expert opinions in protein bioinformatics: 2022
In 2021, protein bioinformatics underwent a profound and irreversible transformation with the publication of AlphaFold2, a method for predicting protein tertiary structures. AlphaFold2 (Jumper et al., 2021) demonstrated significantly higher accuracy compared to existing methods during the 14th Critical Assessment of Techniques for Protein Structure Prediction (CASP14). Notably, its accuracy often rivals that of experimental structure determination techniques like X-ray crystallography, which has proved to be exceptionally valuable in various practical applications.
An additional noteworthy aspect of AlphaFold2 is that it represents the first successful end-to-end deep neural network for protein structure prediction. This achievement is particularly remarkable given that protein structure prediction has long been recognized as one of the most challenging problems in bioinformatics and computational biophysics. Unlike existing methods with complex architectures involving intricate steps in a computational modeling pipeline, AlphaFold2 demonstrated that the entire process can be encapsulated within a single neural network.
The success of Alphafold2 is resulted from convergence of crucial elements, including the maturation of the protein structure prediction problem, the availability of a substantial dataset, the rapid advancements in deep learning, and the presence of adequate computational resources. This also indicates that the same approach would be possible for other molecular modeling problems, too.
Following AlphaFold2, a cascade of related developments ensued, spanning protein docking prediction, algorithms for protein design and drug design, RNA structure prediction, and predictions of the effects of missense mutations, all using deep learning. Consequently, the emergence of AlphaFold2 will be remembered not only as a singular achievement but also as the beginning of the end for the development of general-purpose modeling methods that aim to be applied to any proteins, drugs, RNA, and complexes.
In the era of the end of general-purpose modeling problems, an increasing emphasis will be placed on the accurate solutions to individual, fine-grained, and specific tasks. This compilation serves as a testament to the significant research topics that define the post-general-purpose problems era. Gomez et al. explored the applicability of AlphaFold2 models within the context of force spectroscopy experiments (Gomez et al.). Chang et al. delved into the key factors crucial for the successful computational design of peptides, highlighting an important application of protein design methods (Chang et al.). The article by Neumann et al. the Ben-Hur group specifically addresses the importance of the appropriate selection of negative examples in predicting host-pathogen protein interactions (Neumann et al.). Lastly, Jeffery provides insights into the various challenges that make protein function prediction a complex task (Jeffery).
As we navigate the landscape of the post-general-purpose method development, the contributions highlighted in this Research Topic reflect the profound impact and continued evolution of methodologies addressing specific molecular tasks. The success of future protein bioinformatics projects will hinge on the seamless integration of a detailed understanding of biological problems and background, coupled with the proper application of machine learning methods, to accomplish specific tasks.
DK: Conceptualization, Writing–original draft, Writing–review and editing.
The author(s) declare financial support was received for the research, authorship, and/or publication of this article. DK acknowledges funding from the National Institutes of Health (R01GM133840) and the National Science Foundation (DBI2003635, DBI2146026, IIS2211598, DMS2151678, CMMI1825941, and MCB1925643).
The author declares that the research was conducted in the absence of any commercial or financial relationships that could be construed as a potential conflict of interest.
The author(s) declared that they were an editorial board member of Frontiers, at the time of submission. This had no impact on the peer review process and the final decision.
All claims expressed in this article are solely those of the authors and do not necessarily represent those of their affiliated organizations, or those of the publisher, the editors and the reviewers. Any product that may be evaluated in this article, or claim that may be made by its manufacturer, is not guaranteed or endorsed by the publisher.
Keywords: protein bioinformatics, editorial, protein structure prediction, general purpose modeling methods, AlphaFold2
Citation: Kihara D (2024) Editorial: Expert opinions in protein bioinformatics: 2022. Front. Bioinform. 3:1338560. doi: 10.3389/fbinf.2023.1338560
Received: 14 November 2023; Accepted: 26 December 2023;
Published: 05 January 2024.
Edited and reviewed by:
Sebastian Kmiecik, University of Warsaw, PolandCopyright © 2024 Kihara. This is an open-access article distributed under the terms of the Creative Commons Attribution License (CC BY). The use, distribution or reproduction in other forums is permitted, provided the original author(s) and the copyright owner(s) are credited and that the original publication in this journal is cited, in accordance with accepted academic practice. No use, distribution or reproduction is permitted which does not comply with these terms.
*Correspondence: Daisuke Kihara, ZGtpaGFyYUBwdXJkdWUuZWR1
Disclaimer: All claims expressed in this article are solely those of the authors and do not necessarily represent those of their affiliated organizations, or those of the publisher, the editors and the reviewers. Any product that may be evaluated in this article or claim that may be made by its manufacturer is not guaranteed or endorsed by the publisher.
Research integrity at Frontiers
Learn more about the work of our research integrity team to safeguard the quality of each article we publish.