- State Key Laboratory of Crop Stress Biology for Arid Areas, College of Agronomy, Northwest A&F University, Yangling, Shaanxi, China
With the rapid development of next-generation sequencing (NGS), multi-omics techniques have been emerging as effective approaches for crop improvement. Here, we focus mainly on addressing the current status and future perspectives toward omics-related technologies and bioinformatic resources with potential applications in crop breeding. Using a large amount of omics-level data from the functional genome, transcriptome, proteome, epigenome, metabolome, and microbiome, clarifying the interaction between gene and phenotype formation will become possible. The integration of multi-omics datasets with pan-omics platforms and systems biology could predict the complex traits of crops and elucidate the regulatory networks for genetic improvement. Different scales of trait predictions and decision-making models will facilitate crop breeding more intelligent. Potential challenges that integrate the multi-omics data with studies of gene function and their network to efficiently select desirable agronomic traits are discussed by proposing some cutting-edge breeding strategies for crop improvement. Multi-omics-integrated approaches together with other artificial intelligence techniques will contribute to broadening and deepening our knowledge of crop precision breeding, resulting in speeding up the breeding process.
Introduction
Food security is the major issue for humans in the world nowadays. The three major grain crops, rice, wheat, and maize, have fed billions of people. However, the climate change, lack of arable land, and population expansion have led to food shortages, which require constant improvement in technologies of plant breeding (Huang et al., 2022). To date, crop breeding and improvement have achieved three major stages: phenotype-based artificial selection, hybrid breeding, and molecular breeding [marker-assisted selection (MAS) and genetically modified (GM)] (Shen et al., 2022). Thus, to feed the increasing population, new technologies, such as multi-omics, artificial intelligence (AI), and genome editing, are gradually widely used for plant breeding (breeding 4.0) to precise design. The goal of crop improvement is to select favorable alleles with high yield, good quality, and tolerance to biotic and abiotic stresses to promote the breeding of elite varieties. Using the omics technologies, new breeding strategies were further developed, such as genomic selection (GS) technology which is based on genomic estimated breeding value (GEBV) (Crossa et al., 2017).
Next-generation sequencing (NGS) technologies, including genomics, resequencing, functional genomics, transcriptomics, metabolomics, and epigenomics, have been widely applied in crop improvement. Clearly, the single-omics approach suffers from limitations that affect its sensitivity or specificity. Integration of multiple-omics technologies can overcome some of these limitations. With the acquisition of abundant sequencing data, the integrative analysis of multi-omics has become a usual method to study the genes that control important agronomic traits in crops. The association analysis combined with multi-omics makes full use of the data of comprehensive analysis and the verification of the selected core data with application in breeding. Multi-omics approaches with cutting-edge technologies such as precise genome editing tools will not only identify functional genes in a large scale to reveal the molecular mechanism of plant development and response to stress, but also provide new strategies for crop improvement. Here, we summarize current progress in this field made by multiplex omics technologies and provide a perspective for the future.
Integration of genomics and phenomics
During the past decades, sequencing technologies have been greatly reformed and developed. Thus, high-quality reference genome sequences of many crops have been generated and improved. Based on these reference genomes, resequencing of lots of crop accessions can obtain millions of genetic variations and identify functional genes for agronomic traits during the crop domestication and improvement, such as rice, soybean, maize, cotton, and so on (Xu et al., 2012; Zhou et al., 2015; Wang et al., 2017; Wang et al., 2020).
In recent years, dozens of powerful tools for quantitative trait loci (QTL) mapping have been developed based on deep sequencings, such as restriction-site associated DNA sequencing (RAD-seq), genotyping-by-sequencing (GBS), bulked segregant analysis sequencing (BSA-seq), and specific locus amplified fragment sequencing (SLAF-seq) (Bundo et al., 2022). These mapping approaches used the genetic map or limited number of SNPs to seek the candidate genomic regions and genes for the traits. The disadvantage of these strategies is that the candidate region is large and mainly dependent on the parental traits as well as not precise enough.
Combining the genetic variations (genotype) with phenotype, a genome-wide association study (GWAS) could dissect complex traits and identify candidate genes with natural variations based on millions of SNPs (Fang et al., 2017a; Fang et al., 2017b). In rapeseed, a total of 628 associated loci were detected for 56 agronomically important traits in 403 diverse accessions, including the BnRRF gene for 1000-seed weight (Hu et al., 2022). These genetic loci and causative candidate genes provide a valuable genomic resource for important traits in crops, which will facilitate crop improvement and variety development. Furthermore, pan-genome could cover much more genetic variations, and graph-based pan-genome can provide abundant genetic resources for plant breeding (Shen et al., 2022).
The genotyping platforms combined with high-throughput phenotyping could achieve valuable genetic information for complex traits in crops with standardization and high reproducibility (Zhang et al., 2017). For plant phenomics, advanced sensors, machine vision, and automation technology have been used for phenotyping, including unmanned aerial vehicles (UAV), hyperspectral imaging, and computed tomography (CT) (Yang et al., 2020). Using the automatic phenotyping platform, high-throughput phenotyping data were obtained in several crops such as rice, maize, and rapeseed (Yang et al., 2014; Guo et al., 2018; Li et al., 2020; Wu et al., 2021). For instance, using a high-throughput multiple optical phenotyping system, image-based traits (i-traits) were extracted and detected 2,318 candidate genes by GWAS for drought response in maize (Wu et al., 2021). Also, based on the time-resolved i-traits in rapeseed, the genetic architecture of plant growth and yield were dissected (Li et al., 2020). Using CT, tillering in rice was modeled for approximately 700 associated traits (Wu et al., 2019). High-throughput phenotyping with deep learning analysis pipelines, such as deep plant phenomics (DPP), made the phenotypic identification more precise and faster (Ubbens and Stavness., 2017) (Table 1).
Integration of genomics and transcriptomics
Generally, the candidate gene interval detected by GWAS or QTL mapping was large. Furthermore, combining with transcriptome data, analyzing the expression level of the candidate genes could better determine the key genes for complex quantitative traits. Using the integrative approach of GWAS for upland rice with transcriptomic profiles, the natural variation in the promoter of DROUGHT1 (DROT1) was identified to confer drought resistance in rice (Sun et al., 2022). MADS26 was identified by GWAS and transcriptomic to affect seed germination in maize (Ma et al., 2022). Moreover, integrating genetic and transcriptomic analysis in potato has fine mapped the Ro locus for tuber shape (Fan et al., 2022). Combining BSA-seq and RNA-seq, a WOX gene associated with plant architecture in rapeseed was identified for the compact phenotype (Ye et al., 2022).
GWAS combined with transcriptome-wide association study (TWAS) was also recently developed to detect causal genes for agronomic traits, which explained more heritable variation. Integrating GWAS and TWAS, the genetic basis of seed oil content and glucosinolate content were revealed in rapeseed (Tang et al., 2021; Tan et al., 2022). In maize, the genetic architecture of leaf cuticular conductance was elucidated by GWAS and TWAS (Lin et al., 2022). The expression QTL (eQTL) analysis is the association between the genetic variant and gene expression, which is also an important tool to elucidate complex phenotypes. Combining GWAS and TWAS with the eQTL analysis, the genetic regulatory network for cell wall biosynthesis in cotton has been uncovered (Li et al., 2020). Integrated QTL and eQTL mapping revealed candidate genes for fatty acid composition and flowering time in Brassica napus (Li et al., 2018a).
Integration of genomics, transcriptomics, and metabolomics
The genome-wide association study based on metabolomics analysis (mGWAS) is another powerful complementary tool for phenotypic trait mapping, which has been widely used in crops, including rice, maize, wheat, barley, and tomato (Chen et al., 2014; Zhu et al., 2018; Chen et al., 2020; Zeng et al., 2020). The information of metabolites and metabolic pathways with genetic variations could elucidate the metabolic diversity and their relevance to complex traits for metabolomics-associated breeding in crops (Chen, et al., 2021).
Integrated metabolomics and transcriptomics analysis can establish the metabolite–gene correlation network and screen candidate genes for involving the metabolic pathways (Wen et al., 2014). For instance, the non-targeted metabolomics analysis of leaves for 385 maize lines was conducted with the eQTL analysis to identify drought tolerance hub genes (Zhang et al., 2021). Combined genomes, transcriptomes, and metabolomes reveal how the fruit metabolite content alterations occur in tomatoes during breeding. Also, the results suggested that the selection of genes associated with larger fruits changed the metabolite profiles, and the selection of five major loci that reduced antinutritional compounds rendered the fruit more edible (Zhu et al., 2018). Moreover, through RAD-seq and mGWAS via integrating metabolome and transcriptome data, novel candidate genes for seed coat color were identified, revealing the molecular mechanism of yellow seed in Brassica rapa (Zhao et al., 2022). Integrating QTL mapping, transcriptome, and metabolic profiling, two homologs of EIN4 and TRN1 in loquat were identified as promising candidate genes for fruit weight (Peng et al., 2022).
Integration of genomics, transcriptomics, and microbiomics
The plant microbiome is the basis of plant growth and stress tolerance, including drought and disease resistance. The rhizosphere microorganisms which are the interface between root and soil, can not only promote the absorption of mineral nutrients by plants but also help plants resist the invasion of pathogens. The metagenomic analysis combined with transcriptome profiles revealed that soil microbiota affecting nitrogen metabolism contribute to the ultrahigh yield of rice (Zhong et al., 2020). Microbiome-wide association studies in cassava revealed Lactococcus sp. played an important role in disease resistance, which could be used for control of cassava disease (Zhang et al., 2021). Metagenomic and metatranscriptomic analyses of different watermelon cultivars suggested that the fruit-associated microbiome might play an important role in the carbohydrate metabolism of ripe fruits (Saminathan et al., 2018). Genome-resolved metagenomics with time-series root transcriptome implicated iron metabolism in the root microbiome dynamics in response to drought stress (Xu et al., 2021). Lately, using metagenomics information as an external quantitative phenotype with genomic and transcriptomic data, candidate genes in barley were identified for shaping microbiota composition (Escudero-Martinez et al., 2022). Also, in tomatoes, the bacterial genes involved in the metabolism of iron, sulfur, and vitamins were reported to associate with specific QTLs (Oyserman et al., 2022). The discovery of plant probiotics can increase crop yield, resist to biotic or abiotic stresses, and minimize chemical input (Levy et al., 2018). Thus, the microbiome could be another useful information to incorporate into crop breeding programs.
Integration of genomics, epigenomics, and transcriptomics
Epigenetic variations are mainly dependent on the environments (e.g., biotic and abiotic stresses), which reprogram the transcriptome and maintain the genome stability to adapt to dynamic environments. Epigenetic diversity could produce new heritable phenotypes to widen the source of genetic and phenotypic variations, which has potential for crop improvement (Tirnaz and Batley, 2019; Hou and Wan, 2021). Integrating epigenomic information and transcriptome in 20 representative rice varieties provides comprehensive rice functional DNA elements maps for transcriptional regulation (Zhao et al., 2020). The epigenome maps combined with transcriptomes of B. napus also lay a foundation for the genetic regulation of traits in crop improvement (Zhang et al., 2021). Epigenetic modifications can regulate fertility transition and heterosis via altering the gene expression (Hu et al., 2015). Global DNA methylation, transcriptome, and small RNA profiling analysis revealed the regulatory networks and genes related to hybrid vigor in pigeon peas (Sinha et al., 2020). In wheat, through comprehensive analyses of the open chromatin, DNA methylome, and transcriptomic data elucidated the roles of cis-regulatory elements affecting transcription on a genome scale (Li et al., 2019). Also, combined with nascent RNA sequencing and epigenome profiling, the active transcription of enhancers in the wheat genome was revealed to regulate gene expression (Xie et al., 2022). Comparing the epigenomes and transcriptomes from various tissues under different developmental and environmental conditions provides valuable resources for regulomics in wheat (Wang et al., 2021). In addition, the epigenome and transcriptome changes in response to Magnaporthe oryzae infection implied that epigenomics is involved in rice fungal pathogens (Cui et al., 2021). Therefore, epigenetic variations can be used to reprogram their transcriptome for balancing the various important agronomic traits, and epigenetic diversity is a necessity in crop breeding programs (Tirnaz and Batley, 2019).
Integration of other multi-omics approaches
To comprehensively reveal the potential mechanism at genetic and protein levels in crops under biotic and abiotic stresses, transcriptomics and proteomics were often used to analyze the global changes. Transcriptomics and proteomics analysis of soybean symbiosis with arbuscular mycorrhizal fungi (AMF) gives some insights into the molecular basis of disease resistance (Zhang et al., 2020). Using transcriptomics- and proteomics-based screening, small secreted proteins (SSPs) were identified to regulate rice immunity by rice blast (Wang et al., 2020). Integration of proteomic and transcriptomic profiles, systematic salt tolerance in cotton, and the alternative molecular network of N-deficiency stress in rice were revealed (Peng et al., 2018; Liang et al., 2021). In addition, the genome-scale metabolic pathways integrated with other databases (eg., PMN) were constructed (Hawkins et al., 2021) (Table 1).
Integrated gene regulatory network of microRNAs (miRNAs) and transcription factors (TFs) and genes revealed that intertwined miRNA-containing FFLs are associated with miRNA hubs in Arabidopsis (Gao et al., 2021). Lipidomic and transcriptomic analysis enable the understanding of citrus fruit quality maintenance (Wan et al., 2020). In rice, the genetic architecture of ionome variations has been elucidated via GWAS analysis of 17 mineral elements in grains (Yang et al., 2018).
Integrative methodologies and databases
Due to a large amount of high-throughput data, multi-omics system biology, such as software tools, databases, and approaches are required for multi-omics integration. Recently, these large data sets can be comprehensively assimilated, annotated, as well as modeling using a systematic multi-omics integration (MOI) (Jamil et al., 2020). Machine learning (ML) and deep learning (DL) have been widely used to integrate omics datasets to reveal the functional relationships with these data. Lately, target-oriented prioritization (TOP) was developed to learn the inherent correlations among traits and balance the selection of multiple traits simultaneously (Yang et al., 2022) (Table 1). Several unsupervised clustering methodologies were developed to integrate the multi-omics data, such as GpemDB, IOMA, mixOmics, OmicsPLS, MODAS, multiple co-inertia analysis (MCIA), and similarity network fusion (SNF) (Yizhak et al., 2010; Meng et al., 2014; Wang et al., 2014; Rohart et al., 2017; Bouhaddani et al., 2018; Tini et al., 2019; Gong et al., 2022; Liu et al., 2022) (Table 1). This multiple molecular level (omics) data analysis can extract more knowledge from the available data. Thus, we should consider integrating the omics data simultaneously and reduce false-positive results by adding a priori information (Tini et al., 2019).
A comprehensive database integrates multi-omics data from the same crop and provides a valuable resource for gene cloning or study of the regulatory network, promoting crop improvement, such as MBKbase, WheatOmics, ZEAMP, and so on (Gui et al., 2020; Peng et al., 2020; Ma et al., 2021). On the other hand, many web database platforms were also developed to explore the functional information from multi-omics data, including CARMO, OmicsAnalyst, MapMan4, KBCommons, and Plant regulomics (Wang et al., 2015; Schwacke et al., 2019; Ran et al., 2020; Zeng et al., 2020; Zhou et al., 2021). Lately, an integrative platform in the ENCODE standards, ChIP-Hub, has provided rich resources from the regulome and epigenome data in plants (Fu et al., 2022). Moreover, the integration of regulome and genetic variations leads CRISPR-cereal to promote precise gene editing for wheat, rice, and maize (He et al., 2021). In addition, integrative pipelines for transcriptome or epitranscriptome sequencing data could also offer a clue to the discovery of candidate genes. For instance, to identify the key genes across multiple tissues for yield in rice, a novel dynamic cross-tissue (DCT) network analysis based on the transcriptome was developed to map genotype to phenotype by gene networks (or modules), namely, genotypes → network → phenotypes (Hu et al., 2020). Also, deepEA is a containerized web server for the integration of epitranscriptome with different chemical modifications, including 5-methylcytidine (m5C), N6-methyladenosine (m6A), and so on (Zhai et al., 2021). Using an algorithm, gene co-expression network differential edge-like transformation (GRN-DET), the key regulatory miRNAs for plant development and important traits can be identified by co-variances of miRNA-mRNA (Hu et al., 2018).
Since the features of the large scale, high dimension, high noise, and strong heterogeneity of multi-omics data, more software or algorithms should be developed for gene discovery in crop improvement.
Discussion and future perspectives
Due to NGS technologies generating large-scale sequence data, the collation and utilization of this vast data would require an interdisciplinary approach to integrate, which will be precise tools for crop improvement. GS with doubled-haploid (DH) technology (haploid breeding) could accelerate the breeding process to obtain the elite varieties (Hu et al., 2019; Fu et al., 2022). Multi-omics technologies including genomics, transcriptomics, proteomics, and metabolomics can link the genotype and phenotype, and integrate more information for systematic analysis to largely identified candidate genes for crop improvement (Figure 1). Multi-omics data will be analyzed in a more systematic and integrated way for accelerating crop improvement, such as an intelligent seed selection system. When integrating the multi-omics datasets, “phenotype to genotype” and “genotype to phenotype” as well as the genotype and environment interaction should be considered with the system biology approach to provide the basis for crop genetic improvement. Epigenetic diversity should also be considered in crop breeding programs due to desirable phenotypes by epigenetic modifications. De novo domestication based on the high-quality genome could speedily domesticate wild crops with retaining genetic diversity and elite alleles, which is a new breeding strategy to meet future agricultural challenges, such as rice and tomato (Li et al., 2018b; Yu et al., 2021). The utilization of omics technologies with genome editing, genomic selection, and haploid induction augmented by multi-scale “pan-omics” data will promote crop improvement to obtain a high yield, good quality and to enhance the tolerance of stresses (Figure 1) (Ghosh et al., 2018, Weckwerth et al., 2020).
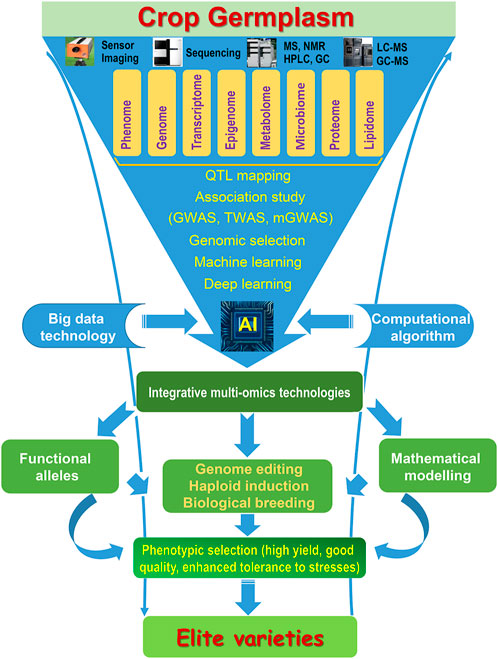
FIGURE 1. Integration of multi-omics technologies accelerates crop improvement. MS, mass spectrometry; NMR, nuclear magnetic resonance; HPLC, high-performance liquid chromatography; GC, gas chromatography; AI, artificial intelligence.
There is a challenge to integrate disparate data from different platforms and formats across the genotype–phenotype spectrum as well as analyze and interpret the final results. The other problem is how to improve the phenotypic prediction based on the large-scale multi-omics data. Novel algorithms or models should also be developed to predict heterosis or complex phenotypes with AI including machine learning and deep learning (Dan et al., 2021; Wang et al., 2021). Thus, integration of more robust visualization tools, multi-omics analysis approaches, statistical genetic models, bioinformatics tools, and cloud computing with interdisciplinary should be integrated to explore candidate variations underlying agronomic traits.
In the future, a promising frontier is the integration of multi-omics data on the single cell level, single-cell multi-omics, which has great potential for crop improvement. The single-cell analysis will be used to identify non-anatomical markers for various cell populations and map individual cell stages during the differentiation of crop plants (Luo et al., 2020). With the development of single-cell multi-omics technologies, it will be possible to conduct simultaneous analyses of the genome, transcriptome, metabolome, and epigenome from a single cell (Shaw et al., 2021). These multi-omics integrative analyses of single cells provide valuable information on how genotype to phenotype at the single-cell level occur.
Data availability statement
The datasets presented in this article are not readily available. Requests to access the datasets should be directed to aHVqaDA1QG53YWZ1LmVkdS5jbg==.
Author contributions
JH conceived the project. RZ, CZ, CY, JD, and JH collected the data. RZ and JH wrote the original draft and revised the manuscript. All the authors approved the submitted manuscript.
Funding
This study was supported by the National Natural Science Foundation of China (31901426) and the Start-Up Funds of Northwest A&F University (Z1090222020) to JH.
Conflict of interest
The authors declare that the research was conducted in the absence of any commercial or financial relationships that could be construed as a potential conflict of interest.
Publisher’s note
All claims expressed in this article are solely those of the authors and do not necessarily represent those of their affiliated organizations, or those of the publisher, the editors, and the reviewers. Any product that may be evaluated in this article, or claim that may be made by its manufacturer, is not guaranteed or endorsed by the publisher.
References
Bouhaddani, S., Uh, H., Jongbloed, G., Hayward, C., Klari´c, L., Kiełbasa, S. M., et al. (2018). Integrating omics datasets with the OmicsPLS package. BMC Bioinforma. 19, 371. doi:10.1186/s12859-018-2371-3
Bundo, M., Martin-Cardoso, H., Pesenti, M., Gomez-Ariza, J., Castillo, L., Frouin, J., et al. (2022). Integrative approach for precise genotyping and transcriptomics of salt tolerant introgression rice lines. Front. Plant Sci. 12, 797141. doi:10.3389/fpls.2021.797141
Chen, J., Hu, X., Shi, T., Yin, H., Sun, D., Hao, Y., et al. (2020). Metabolite-based genome-wide association study enables dissection of the flavonoid decoration pathway of wheat kernels. Plant Biotechnol. J. 18, 1722–1735. doi:10.1111/pbi.13335
Chen, J., Xue, M., Liu, H., Fernie, A., and Chen, W. (2021). Exploring the genic resources underlying metabolites through mGWAS and mQTL in wheat: From large-scale gene identification and pathway elucidation to crop improvement. Plant Commun. 2, 100216. doi:10.1016/j.xplc.2021.100216
Chen, W., Gao, Y., Xie, W., Gong, L., Lu, K., Wang, W., et al. (2014). Genome-wide association analyses provide genetic and biochemical insights into natural variation in rice metabolism. Nat. Genet. 46, 714–721. doi:10.1038/ng.3007
Crossa, J., Perez-Rodriguez, P., Cuevas, J., Montesinos-Lopez, O., Jarquin, D., de Los Campos, G., et al. (2017). Genomic selection in plant breeding: Methods, models, and perspectives. Trends Plant Sci. 22, 961–975. doi:10.1016/j.tplants.2017.08.011
Cui, N., Chen, X., Shi, Y., Chi, M., Hu, J., Lai, K., et al. (2021). Changes in the epigenome and transcriptome of rice in response to Magnaporthe oryzae infection. Crop J. 9, 843–853. doi:10.1016/j.cj.2020.10.002
Dan, Z., Chen, Y., Li, H., Zeng, Y., Xu, W., Zhao, W., et al. (2021). The metabolomic landscape of rice heterosis highlights pathway biomarkers for predicting complex phenotypes. Plant Physiol. 187, 1011–1025. doi:10.1093/plphys/kiab273
Escudero-Martinez, Q., Coulter, M., Terrazas, R., Foito, A., Kanpadia, R., Pietrangelo, L., et al. (2022). Identifying plant genes shaping microbiota composition in the barley rhizosphere. Nat. Commun. 13, 3443. doi:10.1038/s41467-022-31022-y
Fan, G., Wang, Q., Xu, J., Chen, N., Zhu, W., Duan, S., et al. (2022). Fine mapping and candidate gene prediction of tuber shape controlling Ro locus based on integrating genetic and transcriptomic analyses in potato. Int. J. Mol. Sci. 23, 1470. doi:10.3390/ijms23031470
Fang, C., Ma, Y., Wu, S., Liu, Z., Wang, Z., Yang, R., et al. (2017a). Genome-wide association studies dissect the genetic networks underlying agronomical traits in soybean. Genome Biol. 18, 161. doi:10.1186/s13059-017-1289-9
Fang, L., Wang, Q., Hu, Y., Jia, Y., Chen, J., Liu, B., et al. (2017b). Genomic analyses in cotton identify signatures of selection and loci associated with fiber quality and yield traits. Nat. Genet. 49, 1089–1098. doi:10.1038/ng.3887
Fu, J., Hao, Y., Li, H., Reif, J. C., Chen, S., Huang, C., et al. (2022). Integration of genomic selection with doubled-haploid evaluation in hybrid breeding: From GS 1.0 to GS 4.0 and beyond. Mol. Plant 15, 577–580. doi:10.1016/j.molp.2022.02.005
Fu, L., Zhu, T., Zhou, X., Yu, R., He, Z., Zhang, P., et al. (2022). ChIP-Hub provides an integrative platform for exploring plant regulome. Nat. Commun. 13, 3413. doi:10.1038/s41467-022-30770-1
Gao, Z., Li, J., Li, L., Yang, Y., Li, J., Fu, C., et al. (2021). Structural and functional analyses of hub microRNAs in an integrated gene regulatory network of Arabidopsis. Genomics Proteomics Bioinforma. doi:10.1016/j.gpb.2020.02.004
Ghosh, S., Watson, A., Gonzalez-Navarro, O. E., Ramirez-Gonzalez, R. H., Yanes, L., Mendoza-Suarez, M., et al. (2018). Speed breeding in growth chambers and glasshouses for crop breeding and model plant research. Nat. Protoc. 13, 2944–2963. doi:10.1038/s41596-018-0072-z
Gong, L., Lou, Q. J., Yu, C. R., Chen, Y. Y., Hong, J., Wu, W., et al. (2022). GpemDB: A scalable database architecture with the multi-omics entity-relationship model to integrate heterogeneous big-data for precise crop breeding. Front. Biosci. 27, 159. doi:10.31083/j.fbl2705159
Gui, S., Yang, L., Li, J., Luo, J., Xu, X., Yuan, J., et al. (2020). ZEAMAP, a comprehensive database adapted to the maize multi-omics era. iScience 23, 101241. doi:10.1016/j.isci.2020.101241
Guo, Z., Yang, W., Chang, Y., Ma, X., Tu, H., Xiong, F., et al. (2018). Genome-wide association studies of image traits reveal genetic architecture of drought resistance in rice. Mol. Plant 11, 789–805. doi:10.1016/j.molp.2018.03.018
Hawkins, C., Ginzburg, D., Zhao, K., Dwyer, W., Xue, B., Xu, A., et al. (2021). Plant metabolic network 15: A resource of genome-wide metabolism databases for 126 plants and algae. J. Integr. Plant Biol. 63, 1888–1905. doi:10.1111/jipb.13163
He, C., Liu, H., Chen, D., Xie, W., Wang, M., Li, Y., et al. (2021). CRISPR-cereal: A guide RNA design tool integrating regulome and genomic variation for wheat, maize and rice. Plant Biotechnol. J. 19, 2141–2143. doi:10.1111/pbi.13675
Hou, Q., and Wan, X. (2021). Epigenome and epitranscriptome: Potential resources for crop improvement. Int. J. Mol. Sci. 22, 12912. doi:10.3390/ijms222312912
Hu, J., Chen, B., Zhao, J., Zhang, F., Xie, T., Xu, K., et al. (2022). Genomic selection and genetic architecture of agronomic traits during modern rapeseed breeding. Nat. Genet. 54, 694–704. doi:10.1038/s41588-022-01055-6
Hu, J., Chen, X., Zhang, H., and Ding, Y. (2015). Genome-wide analysis of DNA methylation in photoperiod- and thermo-sensitive male sterile rice Peiai 64S. BMC Genomics 16, 102. doi:10.1186/s12864-015-1317-7
Hu, J., Zeng, T., Xia, Q., Huang, L., Zhang, Y., Zhang, C., et al. (2020). Identification of key genes for the ultrahigh yield of rice using dynamic cross-tissue network analysis. Genomics Proteomics Bioinforma. 18, 256–270. doi:10.1016/j.gpb.2019.11.007
Hu, J., Zeng, T., Xia, Q., Qian, Q., Yang, C., Ding, Y., et al. (2018). Unravelling miRNA regulation in yield of rice (Oryza sativa) based on differential network model. Sci. Rep. 8, 8498. doi:10.1038/s41598-018-26438-w
Hu, X., Xie, W., Wu, C., and Xu, S. (2019). A directed learning strategy integrating multiple omic data improves genomic prediction. Plant Biotechnol. J. 17, 2011–2020. doi:10.1111/pbi.13117
Huang, X., Huang, S., Han, B., and Li, J. (2022). The integrated genomics of crop domestication and breeding. Cell 185, 2828–2839. doi:10.1016/j.cell.2022.04.036
Jamil, I., Remali, J., Azizan, K., Muhammad, N., Arita, M., Goh, H., et al. (2020). Systematic multi-omics integration (MOI) approach in plant systems biology. Front. Plant Sci. 11, 944. doi:10.3389/fpls.2020.00944
Levy, A., Conway, J., Dangl, J., and Woyke, T. (2018). Elucidating bacterial gene functions in the plant microbiome. Cell host microbe 24, 475–485. doi:10.1016/j.chom.2018.09.005
Li, H., Feng, H., Guo, C., Yang, S., Huang, W., Xiong, X., et al. (2020). High-throughput phenotyping accelerates the dissection of the dynamic genetic architecture of plant growth and yield improvement in rapeseed. Plant Biotechnol. J. 18, 2345–2353. doi:10.1111/pbi.13396
Li, R., Jeong, K., Davis, J. T., Kim, S., Lee, S., Michelmore, R. W., et al. (2018a). Integrated QTL and eQTL mapping provides insights and candidate genes for fatty acid composition, flowering time, and growth traits in a F2 population of a novel synthetic allopolyploid Brassica napus. Front. Plant Sci. 9, 1632. doi:10.3389/fpls.2018.01632
Li, T., Yang, X., Yu, Y., Si, X., Zhai, X., Zhang, H., et al. (2018b). Domestication of wild tomato is accelerated by genome editing. Nat. Biotechnol. 36, 1160–1163.
Li, Z., Wang, M., Lin, K., Xie, Y., Guo, J., Ye, L., et al. (2019). The bread wheat epigenomic map reveals distinct chromatin architectural and evolutionary features of functional genetic elements. Genome Biol. 20, 139. doi:10.1186/s13059-019-1746-8
Li, Z., Wang, P., You, C., Yu, J., Zhang, X., Yan, F., et al. (2020). Combined GWAS and eQTL analysis uncovers a genetic regulatory network orchestrating the initiation of secondary cell wall development in cotton. New Phytol. 226, 1738–1752. doi:10.1111/nph.16468
Liang, T., Yuan, Z., Fu, L., Zhu, M., Luo, X., Xu, W., et al. (2021). Integrative Transcriptomic and proteomic analysis reveals an alternative molecular network of glutamine synthetase 2 corresponding to nitrogen deficiency in rice (Oryza sativa L.). Int. J. Mol. Sci. 22, 7674. doi:10.3390/ijms22147674
Lin, M., Qiao, P., Matschi, S., Vasquez, M., Ramstein, G., Bourgault, R., et al. (2022). Integrating GWAS and TWAS to elucidate the genetic architecture of maize leaf cuticular conductance. Plant Physiol. 189, 2144–2158. doi:10.1093/plphys/kiac198
Liu, S., Xu, F., Xu, Y., Wang, Q., Yan, J., Wang, J., et al. (2022). Modas: Exploring maize germplasm with multi-omics data association studies. Sci. Bull. (Beijing). 67, 903–906. doi:10.1016/j.scib.2022.01.021
Luo, C., Fernie, A., and Yan, J. (2020). Single-cell genomics and epigenomics: Technologies and applications in plants. Trends Plant Sci. 25, 1030–1040. doi:10.1016/j.tplants.2020.04.016
Ma, L., Wang, C., Hu, Y., Dai, W., Liang, Z., Zou, C., et al. (2022). GWAS and transcriptome analysis reveal MADS26 involved in seed germination ability in maize. Theor. Appl. Genet. 135, 1717–1730. doi:10.1007/s00122-022-04065-4
Ma, S., Wang, M., Wu, J., Guo, W., Chen, Y., Li, G., et al. (2021). WheatOmics: A platform combining multiple omics data to accelerate functional genomics studies in wheat. Mol. Plant 14, 1965. doi:10.1016/j.molp.2021.10.006
Meng, C., Kuster, B., Culhane, A., and Gholami, A. (2014). A multivariate approach to the integration of multi-omics datasets. BMC Bioinforma. 15, 162. doi:10.1186/1471-2105-15-162
Oyserman, B., Flores, S., Griffioen, T., Pan, X., Wijk, E., Pronk, L., et al. (2022). Disentangling the genetic basis of rhizosphere microbiome assembly in tomato. Nat. Commun. 13, 3228. doi:10.1038/s41467-022-30849-9
Peng, H., Wang, K., Chen, Z., Cao, Y., Gao, Q., Li, Y., et al. (2020). MBKbase for rice: An integrated omics knowledgebase for molecular breeding in rice. Nucleic Acids Res. 48, D1085–D1092. doi:10.1093/nar/gkz921
Peng, Z., He, S., Gong, W., Xu, F., Pan, Z., Jia, Y., et al. (2018). Integration of proteomic and transcriptomic profiles reveals multiple levels of genetic regulation of salt tolerance in cotton. BMC Plant Biol. 18, 128. doi:10.1186/s12870-018-1350-1
Peng, Z., Zhao, C., Li, S., Guo, Y., Xu, H., Hu, G., et al. (2022). Integration of genomics, transcriptomics and metabolomics identifies candidate loci underlying fruit weight in loquat. Hortic. Res. 9, uhac037. doi:10.1093/hr/uhac037
Ran, X., Zhao, F., Wang, Y., Liu, J., Zhuang, Y., Ye, L., et al. (2020). Plant regulomics: A data-driven interface for retrieving upstream regulators from plant multi-omics data. Plant J. 101, 237–248. doi:10.1111/tpj.14526
Rohart, F., Gautier, B., Singh, A., and Lê Cao, K. (2017). mixOmics: An R package for ‘omics feature selection and multiple data integration. PLOS Comput. Biol. 13, e1005752. doi:10.1371/journal.pcbi.1005752
Saminathan, T., García, M., Ghimire, B., Lopez, C., Bodunrin, A., Nimmakayala, P., et al. (2018). Metagenomic and metatranscriptomic analyses of diverse watermelon cultivars reveal the role of fruit associated microbiome in carbohydrate metabolism and ripening of mature fruits. Front. Plant Sci. 9, 4. doi:10.3389/fpls.2018.00004
Schwacke, R., Ponce-Soto, G., Krause, K., Bolger, A., Arsova, B., Hallab, A., et al. (2019). MapMan4: A refined protein classification and annotation framework applicable to multi-omics data analysis. Mol. Plant 12, 879–892. doi:10.1016/j.molp.2019.01.003
Shaw, R., Tian, X., and Xu, J. (2021). Single-cell transcriptome analysis in plants: Advances and challenges. Mol. Plant 14, 115–126. doi:10.1016/j.molp.2020.10.012
Shen, Y., Zhou, G., Liang, C., and Tian, Z. (2022). Omics-based interdisciplinarity is accelerating plant breeding. Curr. Opin. Plant Biol. 66, 102167. doi:10.1016/j.pbi.2021.102167
Sinha, P., Singh, V., Saxena, R., Kale, S., Li, Y., Garg, V., et al. (2020). Genome-wide analysis of epigenetic and transcriptional changes associated with heterosis in pigeonpea. Plant Biotechnol. J. 18, 1697–1710. doi:10.1111/pbi.13333
Sun, X., Xiong, H., Jiang, C., Zhang, D., Yang, Z., Huang, Y., et al. (2022). Natural variation of DROT1 confers drought adaptation in upland rice. Nat. Commun. 13, 4265. doi:10.1038/s41467-022-31844-w
Tan, Z., Xie, Z., Dai, L., Zhang, Y., Zhao, H., Tang, S., et al. (2022). Genome- and transcriptome-wide association studies reveal the genetic basis and the breeding history of seed glucosinolate content in Brassica napus. Plant Biotechnol. J. 20, 211–225. doi:10.1111/pbi.13707
Tang, S., Zhao, H., Lu, S., Yu, L., Zhang, G., Zhang, Y., et al. (2021). Genome- and transcriptome-wide association studies provide insights into the genetic basis of natural variation of seed oil content in Brassica napus. Mol. Plant 14, 470–487. doi:10.1016/j.molp.2020.12.003
Tini, G., Marchetti, L., Priami, C., and Scott-Boyer, M. (2019). Multi-omics integration—A comparison of unsupervised clustering methodologies. Brief. Bioinform. 20, 1269–1279. doi:10.1093/bib/bbx167
Tirnaz, S., and Batley, J. (2019). DNA methylation: Toward crop disease resistance improvement. Trends Plant Sci. 24, 1137–1150. doi:10.1016/j.tplants.2019.08.007
Tirnaz, S., and Batley, J. (2019). Epigenetics: Potentials and challenges in crop breeding. Mol. Plant 12, 1309–1311. doi:10.1016/j.molp.2019.09.006
Ubbens, J., and Stavness, I. (2017). Deep plant phenomics: A deep learningplatform for complex plant phenotyping tasks. Front. Plant Sci. 8, 1190. doi:10.3389/fpls.2017.01190
Wan, H., Liu, H., Zhang, J., Lyu, Y., Li, Z., He, Y., et al. (2020). Lipidomic and transcriptomic analysis reveals reallocation of carbon flux from cuticular wax into plastid membrane lipids in a glossy “Newhall” navel orange mutant. Hortic. Res. 7, 41. doi:10.1038/s41438-020-0262-z
Wang, B., Lin, Z., Li, X., Zhao, Y., Zhao, B., Wu, G., et al. (2020). Genome-wide selection and genetic improvement during modern maize breeding. Nat. Genet. 52, 565–571. doi:10.1038/s41588-020-0616-3
Wang, B., Mezlini, A. M., Demir, F., Fiume, M., Tu, Z. W., Brudno, M., et al. (2014). Similarity network fusion for aggregating data types on a genomic scale. Nat. Methods 11 (3), 333–337. doi:10.1038/nmeth.2810
Wang, J. W., Qi, M. F., Liu, J., and Zhang, Y. J. (2015). Carmo: A comprehensive annotation platform for functional exploration of rice multi-omics data. Plant J. 83, 359–374. doi:10.1111/tpj.12894
Wang, M., Li, Z., Zhang, Y., Zhang, Y., Xie, Y., Ye, L., et al. (2021). An atlas of wheat epigenetic regulatory elements reveals subgenome divergence in the regulation of development and stress responses. Plant Cell 33, 865–881. doi:10.1093/plcell/koab028
Wang, M., Tu, L., Lin, M., Lin, Z., Wang, P., Yang, Q., et al. (2017). Asymmetric subgenome selection and cis-regulatory divergence during cotton domestication. Nat. Genet. 49, 579–587. doi:10.1038/ng.3807
Wang, P., Yao, S., Kosami, K., Guo, T., Li, J., Zhang, Y., et al. (2020). Identification of endogenous small peptides involved in rice immunity through transcriptomics- and proteomics-based screening. Plant Biotechnol. J. 18, 415–428. doi:10.1111/pbi.13208
Wang, Q., Yan, T., Long, Z., Yuehuang, L., Zhu, Y., Xu, Y., et al. (2021). Prediction of heterosis in the recent rapeseed (Brassica napus) polyploid by pairing parental nucleotide sequences. PLoS Genet. 17, e1009879. doi:10.1371/journal.pgen.1009879
Weckwerth, W., Ghatak, A., Bellaire, A., Chaturvedi, P., and Varshney, R. (2020). PANOMICS meets germplasm. Plant Biotechnol. J. 18, 1507–1525. doi:10.1111/pbi.13372
Wen, W., Li, D., Li, X., Gao, Y., Li, W., Li, H., et al. (2014). Metabolome-based genome-wide association study of maize kernel leads to novel biochemical insights. Nat. Commun. 5, 3438. doi:10.1038/ncomms4438
Wu, D., Guo, Z., Ye, J., Feng, H., Liu, J., Chen, G., et al. (2019). Combining high-throughput micro CT-RGB phenotyping and genome-wide association study to dissect the genetic architecture of tiller growth in rice. J. Exp. Bot. 70, 545–561. doi:10.1093/jxb/ery373
Wu, X., Feng, H., Wu, D., Yan, S., Zhang, P., Wang, W., et al. (2021). Using high-throughput multiple optical phenotyping to decipher the genetic architecture of maize drought tolerance. Genome Biol. 22, 185. doi:10.1186/s13059-021-02377-0
Xie, Y., Chen, Y., Li, Z., Zhu, J., Liu, M., Zhang, Y., et al. (2022). Enhancer transcription detected in the nascent transcriptomic landscape of bread wheat. Genome Biol. 23, 109. doi:10.1186/s13059-022-02675-1
Xu, L., Dong, Z., Chiniquy, D., Pierroz, G., Deng, S., Gao, C., et al. (2021). Genome-resolved metagenomics reveals role of iron metabolism in drought-induced rhizosphere microbiome dynamics. Nat. Commun. 12, 3209. doi:10.1038/s41467-021-23553-7
Xu, X., Liu, X., Ge, S., Jensen, J., Hu, F., Li, X., et al. (2012). Resequencing 50 accessions of cultivated and wild rice yields markers for identifying agronomically important genes. Nat. Biotechnol. 30, 105–111. doi:10.1038/nbt.2050
Yang, M., Lu, K., Zhao, F., Xie, W., Ramakrishna, P., Wang, G., et al. (2018). Genome-wide association studies reveal the genetic basis of ionomic variation in rice. Plant Cell 30, 2720–2740. doi:10.1105/tpc.18.00375
Yang, W., Feng, H., Zhang, X., Zhang, J., Doonan, J., Batchelor, W., et al. (2020). Crop phenomics and high-throughput phenotyping: Past decades, current, challenges, and future perspectives. Mol. Plant 13, 187–214. doi:10.1016/j.molp.2020.01.008
Yang, W., Guo, T., Luo, J., Zhang, R., Zhao, J., Warburton, M., et al. (2022). Target-oriented prioritization: Targeted selection strategy by integrating organismal and molecular traits through predictive analytics in breeding. Genome Biol. 23, 80. doi:10.1186/s13059-022-02650-w
Yang, W., Guo, Z., Huang, C., Duan, L., Chen, G., Jiang, N., et al. (2014). Combining high-throughput phenotyping and genome-wide association studies to reveal natural genetic variation in rice. Nat. Commun. 5, 5087. doi:10.1038/ncomms6087
Ye, S., Yan, L., Ma, X., Chen, Y., Wu, L., Ma, T., et al. (2022). Combined BSA-seq based mapping and RNA-seq profiling reveal candidate genes associated with plant architecture in Brassica napus. Int. J. Mol. Sci. 23, 2472. doi:10.3390/ijms23052472
Yizhak, K., Benyamini, T., Liebermeister, W., Ruppin, E., and Shlomi, T. (2010). Integrating quantitative proteomics and metabolomics with a genome-scale metabolic network model. Bioinformatics 26, i255–i260. doi:10.1093/bioinformatics/btq183
Yu, H., Lin, T., Meng, X., Du, H., Zhang, J., Liu, G., et al. (2021). A route to de novo domestication of wild allotetraploid rice. Cell 184, 1156–1170. doi:10.1038/nbt.4273
Zeng, S., Lyu, Z., Narisetti, S. R. K., Xu, D., and Joshi, T. (2019). Knowledge base commons (KBCommons) v1.1: A universal framework for multi-omics data integration and biological discoveries. BMC Genomics 20, 947. doi:10.1186/s12864-019-6287-8
Zeng, X., Yuan, H., Dong, X., Peng, M., Jing, X., Xu, Q., et al. (2020). Genome-wide dissection of coselected UV-B responsive pathways in the UV-B adaptation of qingke. Mol. Plant 13, 112–127. doi:10.1016/j.molp.2019.10.009
Zhai, J., Song, J., Zhang, T., Xie, S., and Ma, C. deepE. A. (2021). deepEA: a containerized web server for interactive analysis of epitranscriptome sequencing data. Plant Physiol. 185, 29–33. doi:10.1093/plphys/kiaa008
Zhang, F., Wu, J., Sade, N., Wu, S., Egbaria, A., Fernie, A., et al. (2021). Genomic basis underlying the metabolome-mediated drought adaptation of maize. Genome Biol. 22, 260. doi:10.1186/s13059-021-02481-1
Zhang, L., Zhang, J., Wei, Y., Hu, W., Liu, G., Zeng, H., et al. (2021). Microbiome-wide association studies reveal correlations between the structure and metabolism of the rhizosphere microbiome and disease resistance in cassava. Plant Biotechnol. J. 19, 689–701. doi:10.1111/pbi.13495
Zhang, Q., Guan, P., Zhao, L., Ma, M., Xie, L., Li, Y., et al. (2021). Asymmetric epigenome maps of subgenomes reveal imbalanced transcription and distinct evolutionary trends in Brassica napus. Mol. Plant 14, 604–619. doi:10.1016/j.molp.2020.12.020
Zhang, X., Bai, L., Sun, H., Yang, C., and Cai, B. (2020). Transcriptomic and proteomic analysis revealed the effect of Funneliformis mosseae in soybean roots differential expression genes and proteins. J. Proteome Res. 19, 3631–3643. doi:10.1021/acs.jproteome.0c00017
Zhang, X., Huang, C., Wu, D., Diao, F., Li, W., Duan, L., et al. (2017). High-Throughput phenotyping and QTL mapping reveals the genetic architecture of maize plant growth. Plant Physiol. 173, 1554–1564. doi:10.1104/pp.16.01516
Zhao, H., Shang, G., Yin, N., Chen, S., Shen, S., Jiang, H., et al. (2022). Multi-omics analysis reveals the mechanism of seed coat color formation in Brassica rapa L. Theor. Appl. Genet. 135, 2083–2099. doi:10.1007/s00122-022-04099-8
Zhao, L., Xie, L., Zhang, Q., Ouyang, W., Deng, L., Guan, P., et al. (2020). Integrative analysis of reference epigenomes in 20 rice varieties. Nat. Commun. 11, 2658. doi:10.1038/s41467-020-16457-5
Zhong, Y., Hu, J., Xia, Q., Zhang, S., Li, X., Pan, X., et al. (2020). Soil microbial mechanisms promoting ultrahigh rice yield. Soil Biol. Biochem. 143, 107741. doi:10.1016/j.soilbio.2020.107741
Zhou, G., Ewald, J., and Xia, J. (2021). OmicsAnalyst: A comprehensive web-based platform for visual analytics of multi-omics data. Nucleic Acids Res. 49, W476–W482. doi:10.1093/nar/gkab394
Zhou, Z., Jiang, Y., Wang, Z., Gou, Z., Lyu, J., Li, W., et al. (2015). Resequencing 302 wild and cultivated accessions identifies genes related to domestication and improvement in soybean. Nat. Biotechnol. 33, 408–414. doi:10.1038/nbt.3096
Keywords: multi-omics, crop improvement, integration, artificial intelligence, precision breeding
Citation: Zhang R, Zhang C, Yu C, Dong J and Hu J (2022) Integration of multi-omics technologies for crop improvement: Status and prospects. Front. Bioinform. 2:1027457. doi: 10.3389/fbinf.2022.1027457
Received: 25 August 2022; Accepted: 28 September 2022;
Published: 19 October 2022.
Edited by:
Chuanchao Zhang, Chinese Academy of Sciences (CAS), ChinaReviewed by:
Xuehai Zhang, Henan Agricultural University, ChinaSongtao Gui, Huazhong Agricultural University, China
Copyright © 2022 Zhang, Zhang, Yu, Dong and Hu. This is an open-access article distributed under the terms of the Creative Commons Attribution License (CC BY). The use, distribution or reproduction in other forums is permitted, provided the original author(s) and the copyright owner(s) are credited and that the original publication in this journal is cited, in accordance with accepted academic practice. No use, distribution or reproduction is permitted which does not comply with these terms.
*Correspondence: Jihong Hu, amloaHVAd2h1LmVkdS5jbg==