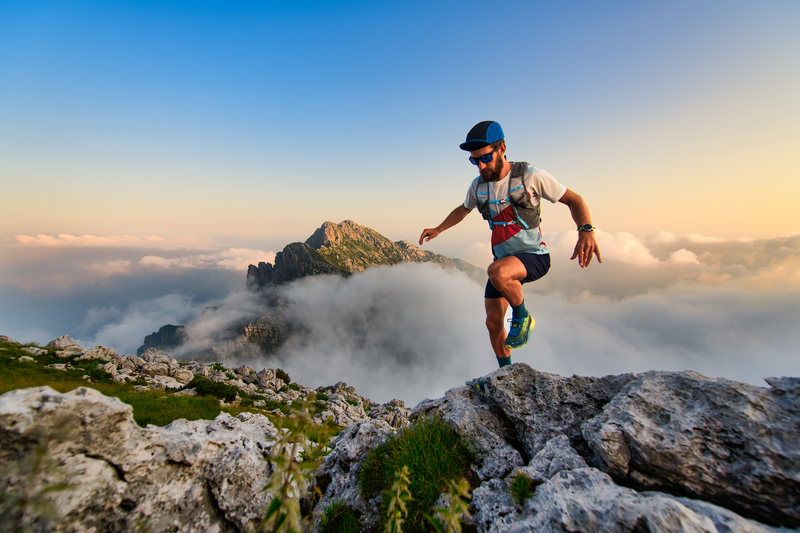
95% of researchers rate our articles as excellent or good
Learn more about the work of our research integrity team to safeguard the quality of each article we publish.
Find out more
ORIGINAL RESEARCH article
Front. Bioeng. Biotechnol. , 26 March 2025
Sec. Nanobiotechnology
Volume 13 - 2025 | https://doi.org/10.3389/fbioe.2025.1568719
This article is part of the Research Topic Dendritic Nanoparticles Synthesis and Application View all articles
The accurate and rapid detection of cardiac troponin I (cTnI) at the point of care is crucial for the timely diagnosis of myocardial infarction (MI). This study introduces an advanced lateral flow immunoassay (LFIA) platform for cTnI detection. We employed small-sized, large-pore dendritic mesoporous silica nanoparticles (DMSN-2) to encapsulate quantum dots (QDs), achieving an enhanced QD loading capacity of 1.427 g QD/g silica, compared to 0.881 g QD/g silica for smaller pore counterparts (DMSN-1). This nano-LFIA was further integrated with “AdaptiScan”, a smartphone-based detection system that uses adaptive detection algorithms to automatically extract and analyze fluorescence signals from LFIA strips. This integration of pore-size tailored DMSNs and “AdaptiScan” resulted in a limit of detection for cTnI of 42.6 ng/L, which meets clinical diagnostic requirements. The platform offers a sensitive, cost-effective, and portable solution for rapid detection of MI, potentially transforming point-of-care testing in resource-limited settings.
Cardiovascular diseases (CVDs) remain the leading cause of global mortality, accounting for an estimated 17.9 million deaths each year, with myocardial infarction (MI) being a predominant contributor due to acute myocardial ischemia and necrosis (Vaduganathan et al., 2022). The cornerstone of MI diagnosis involves electrocardiography and the quantification of cardiac biomarkers, specifically cardiac troponin (cTn) (Thygesen et al., 2019; Byrne et al., 2024), which are present at extremely low levels (2–5 ng/L) in healthy individuals (Clerico et al., 2023). High-sensitivity cardiac troponin (hs-cTn) assays, with a limit of detection (LoD) ≤3 ng/L (Clerico et al., 2023), are typically executed using complex, automated laboratory platforms (Neumann et al., 2019). However, the urgent diagnostic needs in resource-limited settings underscore the necessity for high-sensitivity point-of-care testing (POCT). Lateral flow immunoassays (LFIA) are favored for their simplicity, affordability, and adherence to the WHO’s “ASSURED” criteria for diagnostics (Budd et al., 2023). Nonetheless, conventional LFIA utilizing gold nanoparticles for colorimetric detection often exhibit a higher LoD (>0.1 mg/L) (Liu et al., 2021; Awiaz et al., 2023; Truong et al., 2023), inadequate for hs-cTn detection, and their reliance on visual or standard commercial readers limits quantitative precision at the point of care. This scenario necessitates the development of advanced nano-platforms to enhance the sensitivity and specificity of LFIA for low concentration cTn detection, thereby improving the timeliness and accuracy of MI diagnosis (Rink and Baeumner, 2023).
Quantum dots (QDs) have been identified as superior alternatives to gold nanoparticles in LFIA systems due to their exceptional quantum yield, narrow emission spectra, and tunable optical properties (Lin et al., 2024; Gao et al., 2022). Encapsulating multiple QDs within a single carrier not only amplifies signal intensity but also reduces environmental interference and improves stability (Fang et al., 2022; Gao et al., 2024). Recent studies have delved into various encapsulation techniques, such as soft-template methods involving the self-assembly of amphiphilic polymers to envelop QDs (Huang et al., 2024), and hard-template methods where QDs are sequentially assembled on the surface of silica nanoparticles via a layer-by-layer approach (Li et al., 2025; Yang et al., 2023). Notably, dendritic mesoporous silica nanoparticles (DMSNs) offer a high surface area that significantly boosts QD loading capacity (Gao et al., 2021a; Zhu et al., 2024). However, the efficacy of LFIA is influenced by a multifaceted interaction of physical, chemical, and optical properties which impact signal intensity and analyte capture efficiency (Zhan et al., 2017; Bishop et al., 2019). Our previous work indicated that while larger DMSNs can accommodate more QDs, they might degrade assay performance by elevating background noise and diminishing capture efficiency through slower diffusion rates. Conversely, smaller DMSNs, while cost-effective and stable, have a limited QD loading capacity due to reduced surface area (Gao et al., 2021b). To address this, enhancing the pore size of these smaller DMSNs presents a viable solution. The application of sodium trifluoroacetate (FC2) as a structure-directing agent has shown promise in synthesizing small-sized, large-pore DMSNs for enzyme loading (Wang et al., 2016), yet its application in enhancing QD loading for LFIA is largely unexplored.
The integration of smartphones into LFIA systems has transformed the landscape of POCT by enhancing system portability and accessibility. Smartphones serve as multifunctional tools for control, analysis, and display, leveraging their high-resolution cameras and computational power to analyze test images, often rivaling benchtop readers (Xiao et al., 2022). Innovations include a smartphone-based system for Zika virus NS1 protein detection using a 3D-printed accessory for noise reduction and ImageJ for data analysis (Rong et al., 2019), and the use of an R Shiny application with a 3D-printed photo box for a more tailored analytical workflow (Schary et al., 2022). Moreover, Python-based tools have been developed for automated POCT quantification, emphasizing automation, reproducibility, and cost-effectiveness (Cuny et al., 2021). Despite these advancements, a critical gap remains in Python-based algorithms specifically designed for detecting faint signals on smartphone-adapted LFIA platforms, particularly when test strip signals are weak or when background autofluorescence and noise interferes with detection. This limitation constrains the minimum detectable concentration of analytes and underscores the urgent need for tools capable of intelligent image processing and adaptive detection of fluorescent test lines, thereby facilitating the sensitive detection of low-concentration analytes for early-stage diagnosis.
In this study, we present an innovative LFIA platform for detecting cardiac troponin I (cTnI), utilizing small-sized, large-pore DMSNs (DMSN-2) with an average diameter of 122.3 nm and a pore size of 22.9 nm to significantly increase QD loading (Scheme 1A). This approach enhanced the QD loading capacity from 0.881 to 1.427 g QD/g silica compared to smaller pore DMSNs (DMSN-1, pore size 11.2 nm). We combined this nano-LFIA with a cost-effective miniaturized smartphone-based detection system named “AdaptiScan” (Scheme 1B). Using a Samsung Galaxy S9 in PRO mode (F1.5 aperture, ISO 640), we captured and processed fluorescence signals through a custom mobile application featuring adaptive detection algorithms, achieving an exceptional LoD of 42.6 ng/L for cTnI. This system also supports batch processing, thereby advancing the sensitivity and practicality of LFIA for cardiac biomarker detection in resource-constrained environments.
Scheme 1. (A) Schematic illustration of the enhanced loading capacities of QDs in large-pore DMSN as compared to small-pore DMSN, along with the design of a DMSN-QD-based LFIA test strip for the detection of cTnI. (B) Schematic illustration of our custom-developed smartphone-based detection system equipped with adaptive detection algorithms for improved signal processing, named “AdaptiScan”, in comparison with the conventional ImageJ software.
All chemicals were used as received, without further purification. CdSe/ZnS QDs emitting at 620 nm were sourced from Xiamen Bohr Science & Technology Co., Ltd. Triethanolamine (TEA), cetyltrimethylammonium bromide (CTAB), (3-mercaptopropyl)trimethoxysilane (MPTMS), 3-mercaptopropionic acid (3-MPA), D-(+) sucrose, polyvinylpyrrolidone (PVP, Mw ≈ 24 K) and bovine serum albumin (BSA) were obtained from Adamas-beta. Phosphate-buffered saline (PBS) and FC2 were received from Sigma-Aldrich. Chloroform and hydrochloric acid (37% wt) were received from Sinopharm Chemical Reagent Co., Ltd. Poly(ethylene glycol) (PEG, Mw ≈ 1,500), tetraethyl orthosilicate (TEOS), sodium salicylate (NaSal), methanol, and ethanol were obtained from General-reagent, Tween 20 was purchased from TCI (Shanghai) Development Co., Ltd. Dimethyl sulfoxide (DMSO) was purchased from Shanghai Aladdin Bio-Chem Technology Co., Ltd. Ammonium hydroxide (28 wt.%) were received from Chem Supply Pty Ltd. The cardiac troponin I (cTnI) antigen (No. CTNI-Ag5) was obtained from FAPON Biotech Inc. Capture antibodies, including recombinant monoclonal anti-cTnI antibodies (anti-cTnI mAb, No. RC4T21-Y302) and human native cardiac troponin complex antibody (cTn complex Abs, No. 4TC2-20C6cc), along with detection antibodies such as recombinant anti-cTnI mAb (No. RC4T21-RecR85) and troponin C cardiac (TnC, No. 4T27cc-7B9cc), were obtained from HyTest. Human Immunoglobulin G (Human IgG) and goat anti-mouse (GAM) IgG were purchased from Changsha Bioadvantage Co. Ltd. Carcinoembryonic antigen (CEA), human alpha-fetoprotein (AFP), prostate-specific antigen (PSA), and C-reactive protein (CRP) antigens were obtained from Shanghai Linc-Bio Science Co., Ltd. Human serum was obtained from Jiangsu Kewei Biotechnology Co., Ltd. Materials for lateral flow assays included nitrocellulose (NC) membrane (CN140), absorbent pad, sample pad, conjugate pad and PVC backing card, all of which were purchased from Shanghai Kinbio Tech Co., Ltd. Materials for the smartphone-based detection device include UV lamps, which were sourced from Shenzhen Orange Purple Lighting Optoelectronics Co., Ltd., and filter glasses and prisms, which were purchased from Shenzhen Hengyang Optical Co., Ltd. Deionized water (DI water) used for all experiments was prepared using a Milli-Q system (Millipore, Bedford, MA).
In a typical synthesis, uniform nonporous Stöber spheres (SNPs) were synthesized according to a previously reported method (Yu et al., 2016). Absolute ethanol (17.6 mL) was mixed with Milli-Q water (6.8 mL) and ammonium hydroxide (28 wt.%, 0.7 mL) at 25°C. Subsequently, a mixture of TEOS (2.8 mL) and ethanol (22.2 mL) was added under vigorous magnetic stirring. After 13 h, the synthesized SNPs were separated by centrifugation and washed with ethanol. The SNPs were finally dispersed in ethanol for further use.
Monodispersed DMSN-1 were synthesized with modifications to a reported method (Gao et al., 2021b). TEA (408 mg) was added to 150 mL of water and stirred at 80°C for 30 min. Afterwards, 2.28 g of CTAB and 0.403 g of NaSal were introduced and stirred for another hour. 24 mL of TEOS and 0.76 mL of ethanol were then added, maintaining stirring for an additional 2 h. The DMSN-1 were collected via centrifugation and washed with ethanol to remove residual reactants. Subsequently, the surfactant was extracted using acidic methanol (3 mL of 37% HCl in 50 mL of absolute methanol) at 60°C for three 6-h sessions. The resulting DMSN-1 were dispersed in ethanol.
DMSN-2 were synthesized similarly to DMSN-1 but with a different porogen (Wang et al., 2016; Wang et al., 2018). TEA (408 mg) was dissolved in 150 mL of water and stirred at 80°C for 30 min followed by the addition of CTAB (2.28 g) and FC2 (0.8509 g), stirring for 1 h. After the addition of 24 mL of TEOS, the solution was further stirred for 1 h before product was collected. Subsequently, the surfactant was extracted with acidic methanol as described for DMSN-1. DMSN-2 were then dispersed in ethanol.
For surface modification with thiol groups, 40 mL of ethanol solution containing 400 mg of nanomaterials was mixed with 1 mL of ammonia and 480 μL of MPTMS. This mixture was stirred at room temperature for 12 h. The modified nanoparticles were collected by centrifugation at 10,000 rpm for 20 min and washed three times with ethanol. The obtained thiolated DMSN (T-DMSN) were dispersed in 40 mL of ethanol.
To immobilize QDs in T-DMSN, a volume of CdSe/ZnS QDs in chloroform and T-DMSN in ethanol were sonicated for 10 min (Gao et al., 2021b). The resulting DMSN-QD composites were centrifuged and washed multiple times with chloroform to remove excess QDs. The precipitate was redispersed in 3.5 mL of chloroform, mixed with an equal volume of DMSO and 25 μL of 3-MPA, and sonicated for 30 min to facilitate phase transfer (Gao et al., 2021b). The carboxyl-terminated DMSN-QD-COOH were then collected, washed with DI water, and dispersed in DI water for further use.
DMSN-QD-COOH were functionalized with anti-cTnI mAbs and TnC through physical adsorption (Gao et al., 2021b). A quantity of DMSN-QD-COOH was suspended in 1 mL of PBS buffer (10 mM, pH = 7.4), followed by the addition of 10 μl of anti-cTnI mAbs and TnC (1 mg/mL each). After 2 h of gentle agitation at room temperature, the immuno-fluorescent DMSN-QD-COOH (I-DMSN-QD-COOH) were harvested by centrifugation, washed once with PBS containing 0.05% Tween-20 (PBST), and blocked using a buffer containing 10 mM PBS, 10% BSA, and 0.5% Tween-20 for 2 h. The final preparation was redispersed in 20 mM PBS buffer with 1% sucrose, 2.5% BSA and 2% PEG-1500 and stored at 4°C.
The sample pads and conjugation pads were treated with 20 mM phosphate buffer (pH = 7.4) containing 1% BSA (w/v), 1% Tween 20 (v/v), 2.5% sucrose and 0.3% PVP K24 (w/v) for 1 min and dried at 37°C. For the test line (T line), a mixture of cTn complex Abs and anti-cTnI mAbs (0.5 mg/mL each) was dispensed onto the NC membrane at 1.0 μL/cm. The C line was prepared with goat anti-mouse IgG (GAM IgG, 1 mg/mL) at the same rate. The NC membrane was then dried at 37°C for 2 h. The pads and NC membrane were assembled onto a PVC backing card and cut into 4 mm wide strips.
The smartphone-based detection device consists of a 3D-printed holder that integrates several critical optical components: an ultraviolet light-emitting diode (UV LED) with a wavelength of 365–370 nm, an aluminum UV reflective mirror, a 600 nm long-pass filter, and a Samsung Galaxy S9 smartphone (Figure 4A). The holder is designed with a base slot for the exact placement of the test strip. The long-pass filter serves to attenuate interference from both the UV excitation light and background autofluorescence. The UV LED sits on an adjustable base to achieve optimal collimation of light. For image acquisition, the Samsung Galaxy S9’s camera utilizes manual photographic controls, with images taken from the top of the holder. A 25 mm × 25 mm reflective mirror is employed to provide even illumination by redirecting horizontally scattered excitation light onto the test strips positioned at the bottom of the holder.
A mixture of 97 µL cTnI standard solution and 3 µL I-DMSN-QD-COOH was applied to the sample pad of the LFlA, allowing all liquid to migrate to the absorbent pad by capillary action. After 15 min, the assay was evaluated using the smartphone-based test strip detection device. All groups performed in triplicate.
Transmission electron microscopy (TEM), high-resolution TEM (HRTEM) images and energy-dispersive X-ray spectroscopy (EDS) elemental mappings were acquired with a Thermo Talos F200X instrument. For TEM analysis, samples were prepared by dispersing the powders in ethanol with sonication, followed by deposition and drying on the holey carbon film supported by copper grids. The morphology of the samples was observed with a Hitachi SU8010 field-emission scanning electron microscopy (FE-SEM) operated at 5 kV. SEM specimens were prepared by dispersing the powders in ethanol, then depositing them onto aluminum foil pieces, which were subsequently attached to a conductive carbon film on the SEM mount. The SEM mount was dried in a vacuum oven at 60°C for 12 h prior to characterization. The specific surface area and pore size distribution were determined using the Brunauer–Emmett–Teller (BET) method and the Barrett–Joyner–Halenda (BJH) method derived from the adsorption branch of the nitrogen isotherms, respectively. The loading amount of QDs was evaluated by measuring the concentration of cadmium (Cd) atoms in the synthesized materials using Thermo Fisher Scientific iCAP RQ inductively coupled plasma mass spectrometry (ICP-MS). Zeta potential and dynamic light scattering (DLS) measurements were carried out in DI water at 25°C using a Malvern Zetasizer Nano ZEN3700. Fluorescence emission spectra were record on a Hitachi F-2710 spectrofluorometer, with scanning from 550 to 700 nm under a fixed excitation wavelength of 400 nm. UV-vis absorption spectra were acquired with a Shimadzu UV-3600i plus over the range of 550–700 nm at room temperature.
To investigate the effect of pore size on QD loading, three types of silica nanoparticles were synthesized: non-porous silica nanoparticles (SNP), small pore-sized mesoporous silica nanoparticles (DMSN-1), and large pore-sized mesoporous silica nanoparticles (DMSN-2). TEM images (Figures 1A1–A3) demonstrate that all nanoparticles are monodisperse with similar overall sizes but distinct pore structures. The average particle sizes from TEM analysis are 116.7 ± 5.8 nm for SNP, 143.6 ± 10.8 nm for DMSN-1, and 122.3 ± 8.7 nm for DMNS-2 (Figures 1B1–B3). The SNPs were prepared using the Stöber method (Yu et al., 2016), known for producing homogenous, non-porous silica spheres. DMSN-1 was synthesized with CTAB and NaSal as structure-directing agents. The salicylate anions (Sal−) from NaSal facilitate pore formation by inducing micelle swelling, resulting in pores with an average size of approximately 12.4 nm (Yang et al., 2016). In contrast, the synthesis of DMSN-2 employed CTAB alongside a fluorocarbon surfactant (FC2) as structure-directing agents (Wang et al., 2016), leading to an increase in pore size to 22.9 nm due to enhanced micelle interactions. The fluorocarbon anions interact with the positively charged head groups of CTA+ in CTAB, penetrating the hydrophobic core of the micelles. This interaction increases the packing parameter (g), leading to a structural transition from swollen micelles to lamellar structures, thus promoting the formation of larger pores. Comparatively, NaSal, with its phenolic group and lower hydrophobicity, forms smaller pores due to a more compact micelle structure. SEM images (Figures 1C1–C3) support these findings, showing a transition from a smooth surface in SNP to increasingly porous surfaces in DMSN-1 and DMSN-2. BET analysis (Figures 1D1–D3; Supplementary Table 1) reveals an increase in specific surface areas from 380.9 m2/g for SNP (no pores) to 549.8 m2/g for DMSN-1 (12.4 nm pores) and 666.3 m2/g for DMSN-2 (22.9 nm pores), suggesting a direct correlation between pore size and surface area, which can enhance QD loading capabilities. DLS measurements reveal hydrodynamic diameters of 144.9 ± 2.1 nm, 170.5 ± 2.5 nm, and 185.0 ± 8.6 nm, with polydispersity indices (PDI) of 0.031 ± 0.019, 0.040 ± 0.013, and 0.262 ± 0.009 for SNP, DMSN-1, and DMSN-2, respectively. The zeta potential values are measured to be −25.6 ± 1.3 mV, −25.0 ± 0.9 mV, and −22.6 ± 1.3 mV (Supplementary Table 1).
Figure 1. TEM images of (A1) SNP, (A2) DMSN-1 and (A3) DMSN-2. Corresponding TEM size distributions and Gaussian fittings for (B1) SNP, (B2) DMSN-1 and (B3) DMSN-2. SEM images of (C1) SNP, (C2) DMSN-1 and (C3) DMSN-2. N2 sorption isotherms and pore size distribution curves for (D1) SNP, (D2) DMSN-1 and (D3) DMSN-2.
The QD loading capacity was assessed for silica nanoparticles with varying pore sizes. The QDs, which emit at 620 nm (Supplementary Figure 1A) and have a TEM size distribution of 12.5 ± 2.4 nm (Supplementary Figure 1B), were immobilized via thiol-metal coordination. As illustrated in Figures 2A–C, QD loading increased with feeding amount across all groups until reaching saturation. Quantitative analysis by ICP-MS reveals maximum QD loading capacities of 0.620, 0.881, and 1.427 g QD/g silica for SNP, DMSN-1, and DMSN-2, respectively. This enhancement in loading capacity aligns with the increase in the surface area provided by larger pores, consistent with previous studies (Zhu et al., 2024; Gao et al., 2021b; Huang et al., 2018). Specifically, DMSN-2 exhibits the highest capacity due to its larger pore size. As can be seen from Figure 2D, the QDs are on the surface of the non-porous SNP. With an increase in pore size to 12.4 nm for DMSN-1, more QD can be immobilized (Figure 2E). Pore blocking in DMSN-1 is evidenced by the SEM image presented in Supplementary Figure 2A due to similar QD and pore size. An increase in pore size to 22.9 nm allows DMSN-2 to maintain open pores for further QD loading (Supplementary Figure 2B; Figure 2F). Given its lower QD loading capacity, SNP was excluded from further studies.
Figure 2. Relationships between QD loading and QD feeding amount for (A) SNP, (B) DMSN-1, and (C) DMSN-2, with corresponding TEM images for (D) SNPQD, (E) DMSN-1-QD, and (F) DMSN-2-QD.
To ensure the suitability of the prepared DMSN-QD nanocomposites for LFIA application, a 3-MPA ligand exchange strategy was implemented to transfer DMSN-QD into an aqueous phase (Gao et al., 2021b). The substitution of hydrophobic trioctylphosphine oxide and oleic acid (TOPO/OA) ligands on the QD surface with 3-MPA can introduce surface defects and lead to fluorescence quenching (Gao et al., 2021a). Therefore, the amount of 3-MPA was carefully optimized to minimize fluorescence quenching while ensuring effective phase transfer (Supplementary Figure 3). The optimal volumes of 3-MPA for DMSN-1-QD and DMSN-2-QD are determined to be 27.5 µL and 30.0 µL, respectively. The resulting DMSN-2-QD-COOH displays both high fluorescence and excellent water dispersibility. TEM and HRTEM images (Figures 3A, B) depict a pomegranate-like structure with crystalline QDs embedded within the silica matrix. The lattice spacing of 3.3 Å corresponds to the (100) plane of wurtzite ZnS (Xia et al., 2010). The high-angle annular dark-field scanning transmission electron microscopy (HAADF-STEM) image (Figure 3C) shows numerous bright spots, indicative of high loading and homogeneous distribution of CdSe/ZnS QDs within the silica matrix. This is further confirmed by the EDS elemental mappings showing Cd, Zn, Si, Se, S, and O (Figure 3D).
Figure 3. (A) TEM image, (B) HRTEM image, (C) HAADF-STEM image, and (D) EDS elemental mapping of DMSN-2-QD-COOH.
The high fluorescence intensity and excellent aqueous dispersibility of DMSN-QD-COOH render them suitable for LFIA applications. By conjugating DMSN-QD-COOH with cTnI detection antibodies, I-DMSN-QD-COOH were synthesized for the detection of cTnI, a critical biomarker for MI. The assay procedure involves applying 100 µL of a premixed cTnI standard solution with I-DMSN-QD-COOH to the sample pad, resulting in specific antigen-antibody interactions at the T line, forming a fluorescent sandwich structure. C line validation is ensured through interactions with GAM IgG. The quantification of fluorescence signals at the T and C lines is facilitated by our “AdaptiScan” system, employing a Samsung Galaxy S9 smartphone for image capture and analysis in PRO mode, configured with an F1.5 aperture and ISO 640 settings. Fluorescence signals from the reacted test strips are recorded as RGB images by the smartphone’s camera above a long-pass filter (Figure 4A). The captured images are then processed and analyzed via a custom-developed mobile application featuring adaptive detection algorithms for precise evaluation of the fluorescent intensities at both the T and C lines (Figure 4B).
Figure 4. Schematic illustration of “AdaptiScan” system. (A) Demonstration of the smartphone-based optical device for test strip detection. (B) Flowchart of the adaptive detection algorithms for fluorescent signal extraction and image processing of the reacted test strips.
The initial processing step involves image enhancement, threshold segmentation, and contour extraction to accurately locate the test strip and define the regions of interest (ROI) for T and C lines adaptively (Figure 4B). Specifically, the contrast-limited adaptive histogram equalization (CLAHE) algorithm is applied to enhance the contrast of the red channel in the RGB images, thereby improving visibility of the test strip region. For quantitative assessment of T and C line fluorescence, a morphological opening algorithm is utlized to estimate background noise, which is then subtracted from the red channel for background correction. Adaptive thresholding using Otsu’s method further refines the identification of T and C line regions within the ROI. Subsequent steps include smoothing and morphological denoising to accurately delineate the T and C line contours while reducing background noise interference. The average grayscale value of pixels within these delineated areas is then calculated to quantify fluorescence. The above regulated procedure significantly enhances the detection of weak fluorescent signals against a complex and noisy background on FLIA test strips, demonstrating excellent robustness and stability for comprehensive POCT applications. Our application is also adept at automatically processing images from different batches and samples, thereby enhancing its practical utility.
The pore size of nanoparticles significantly influences QD loading capacity, which in turn affects the sensitivity of LFIA. To enhance detection sensitivity, the analytical performances of I-DMSN-QD-COOH with varying pore sizes were compared. Initially, the optimal concentration of the cTnI detection antibody for conjugation was determined. The optimal detection antibody concentrations for forming I-DMSN-QD-COOH are found to be 30 μg/mL for I-DMSN-1-QD-COOH and 10 μg/mL for I-DMSN-2-QD-COOH (Supplementary Figure 4). The detection capabilities of these I-DMSN-QD-COOH-labeled LFIA were then evaluated across a cTnI concentration range of 0–20 ng/mL. As illustrated in Figures 5A, B, all LFIA strips yield valid results with visible red fluorescent C lines. The T line intensity increases with cTnI concentrations from 0 to 20 ng/mL (diluted in PBS). I-DMSN-2-QD-COOH displays a lower LoD of 500 pg/mL, compared to 1 ng/mL for I-DMSN-1-QD-COOH as determined by naked eye observation. The FT/FC value is utilized for cTnI quantification, with Figures 5C, D displaying a linear relationship with concentration from 1 to 10 ng/mL for I-DMSN-1-QD-COOH and from 0.5 to 10 ng/mL for I-DMSN-2-QD-COOH. According to the 3σ/S rule (where σ is the standard deviation of the blank and S is the slope of the linearity range), the LoDs for I-DMSN-2-QD-COOH and I-DMSN-1-QD-COOH are calculated to be 42.6 ng/L and 436.7 ng/L, respectively. The combination of a low LoD (42.6 ng/L) and a wide linear detection range (0.5–10 ng/mL) make I-DMSN-2-QD-COOH the superior label for LFIA-based cTnI detection. Notably, the current assay demonstrates higher sensitivity compared with reported LFIA strips for cTnI analysis (Supplementary Table 2)
Figure 5. I-DMSN-QD-COOH-based LFIA for sensitive cTnI detection. Fluorescence images of test strips for (A) I-DMSN-1-QD-COOH and (B) I-DMSN-2-QD-COOH in the presence of varying cTnI concentrations from 0 to 20 ng/mL. Linear response curves for (C) I-DMSN-1-QD-COOH and (D) I-DMSN-2-QD-COOH-based LFIA with cTnI concentration ranges of (C) 1–10 ng/mL and (D) 0.5–10 ng/mL, respectively. Error bars represent the standard errors from three independent experiments.
The practical utility of the I-DMSN-2-QD-labeled LFIA for detecting cTnI in clinical settings was assessed using human serum samples. Consistent with the results in PBS buffer (Figure 5B), the LFIA strips labeled with I-DMSN-2-QD-COOH produce valid results with visible C and the T line intensity increased in a concentration-dependent manner with cTnI concentrations ranging from 0 to 20 ng/mL, as depicted in Figure 6A. A linear correlation between the signal ratio and cTnI concentration is observed within the range of 0.5–10 ng/mL, with a coefficient of determination (R2) of 0.991 (Figure 6B). While our assay’s LoD of 42.6 ng/L slightly exceeds the 99th percentile URL of 40 ng/L (Mariathas et al., 2019), this LoD remains suitable for identifying clinically significant elevations in acute settings. Further optimization is required to achieve the sensitivity needed for early MI detection. To evaluate the specificity of the I-DMSN-2-QD-COOH-labeled LFIA, interference tests were conducted with various proteins including CRP, CEA, AFP, PSA, and human IgG. As shown in Figure 6C, none of these interfering proteins produces a detectable signal even at concentrations 10,000 times higher than cTnI. In contrast, a cTnI concentration of 1 ng/mL resulted in a strong fluorescent signal despite the presence of high levels of mixed interfering proteins. This highlights the high specificity of the current assay for cTnI, indicating its potential for accurate clinical diagnosis of MI. Considering that low cost is one of the main strengths of LFIA, we have included a comparison with commercial products for cTnI in Supplementary Table 3. The cost of the DMSN-2-QD-COOH-labelled LFIA was estimated (see Supplementary Table 4). The low cost of ¥2.27/strip indicates the feasibility for potential commercial applications.
Figure 6. (A) Fluorescence photographs of test strips using I-DMSN-2-QD-COOH in human serum with cTnI concentrations from 0 to 20 ng/mL. (B) Linear response curve of the I-DMSN-2-QD-COOH-based LFIA for cTnI detection in human serum, spanning concentrations from 0.5 to 10 ng/mL. Error bars denote the standard errors from three independent experiments. (C) Fluorescence photographs evaluating the specificity of the I-DMSN-2-QD-COOH-labelled LFIA with various protein analytes at 10 μg/mL.
In this study, three types of silica nanoparticles with varied pore sizes were synthesized to optimize QD loading for enhancing the sensitivity of LFIA. The small-sized and large-pore mesoporous silica nanoparticles (DMSN-2) demonstrated the highest QD loading capacity of 1.427 g QD/g silica. Integration with our custom “AdaptiScan” smartphone-based detection system, which employs adaptive detection algorithms for image processing and fluorescent signal extraction, a low LoD of 42.6 ng/L for cTnI was achieved, meeting the criteria for clinical diagnostic applications. Our findings provide valuable insights into the rational design of high-quality, water-dispersible QD-based nanoplatforms and sensitive smartphone-based POCT devices, thereby facilitating the development of an ultrasensitive LFIA platform.
The original contributions presented in the study are included in the article/Supplementary Material, further inquiries can be directed to the corresponding authors.
SY: Methodology, Project administration, Writing–original draft, Writing–review and editing, Data curation, Formal Analysis, Investigation. CX: Methodology, Writing–review and editing, Formal Analysis, Investigation. HL: Writing–review and editing. SF: Writing–review and editing. YW: Writing–review and editing, Conceptualization, Methodology, Supervision. FG: Conceptualization, Methodology, Supervision, Writing–review and editing, Project administration, Resources, Writing–original draft.
The author(s) declare that financial support was received for the research, authorship, and/or publication of this article. The studies were funded by grants from the National Natural Science Foundation of China (62401569) and the Outstanding Leaders Training Program of Pudong Health Bureau of Shanghai (PWR12024-01).
This work is supported by the Center for Instrumental Analysis, University of Shanghai for Science and Technology.
The authors declare that the research was conducted in the absence of any commercial or financial relationships that could be construed as a potential conflict of interest.
The author(s) declare that no Generative AI was used in the creation of this manuscript.
All claims expressed in this article are solely those of the authors and do not necessarily represent those of their affiliated organizations, or those of the publisher, the editors and the reviewers. Any product that may be evaluated in this article, or claim that may be made by its manufacturer, is not guaranteed or endorsed by the publisher.
The Supplementary Material for this article can be found online at: https://www.frontiersin.org/articles/10.3389/fbioe.2025.1568719/full#supplementary-material
Awiaz, G., Lin, J., and Wu, A. (2023). Recent advances of Au@Ag core–shell SERS-based biosensors. Exploration 3, 20220072. doi:10.1002/EXP.20220072
Bishop, J. D., Hsieh, H. V., Gasperino, D. J., and Weigl, B. H. (2019). Sensitivity enhancement in lateral flow assays: a systems perspective. Lab. Chip 19, 2486–2499. doi:10.1039/c9lc00104b
Budd, J., Miller, B. S., Weckman, N. E., Cherkaoui, D., Huang, D., Decruz, A. T., et al. (2023). Lateral flow test engineering and lessons learned from COVID-19. Nat. Rev. Bioeng. 1, 13–31. doi:10.1038/s44222-022-00007-3
Byrne, R. A., Rossello, X., Coughlan, J. J., Barbato, E., Berry, C., Chieffo, A., et al. (2024). 2023 ESC Guidelines for the management of acute coronary syndromes: developed by the task force on the management of acute coronary syndromes of the European Society of Cardiology (ESC). Eur. Heart J. Acute Cardiovasc. Care 13, 55–161. doi:10.1093/eurheartj/ehad191
Clerico, A., Zaninotto, M., Aimo, A., Cardinale, D. M., Dittadi, R., Sandri, M. T., et al. (2023). Variability of cardiac troponin levels in normal subjects and in patients with cardiovascular diseases: analytical considerations and clinical relevance: a consensus document by the Study Group on Cardiac Biomarkers from Italian Society of Biochemical Chemistry (SIBioC) and European Ligand Assay Society (ELAS). Clin. Chem. Lab. Med. 61, 1209–1229. doi:10.1515/cclm-2022-1285
Cuny, A. P., Rudolf, F., and Ponti, A. (2021). pyPOCQuant—a tool to automatically quantify point-of-care tests from images. SoftwareX 15, 100710. doi:10.1016/j.softx.2021.100710
Fang, B., Xiong, Q., Duan, H., Xiong, Y., and Lai, W. (2022). Tailored quantum dots for enhancing sensing performance of lateral flow immunoassay. Trends Anal. Chem. 157, 116754. doi:10.1016/j.trac.2022.116754
Gao, F., Lei, C., Liu, Y., Song, H., Kong, Y., Wan, J., et al. (2021a). Rational design of dendritic mesoporous silica nanoparticles’ surface chemistry for quantum dot enrichment and an ultrasensitive lateral flow immunoassay. ACS Appl. Mater. Interfaces 13, 21507–21515. doi:10.1021/acsami.1c02149
Gao, F., Liu, C., Yao, Y., Lei, C., Li, S., Yuan, L., et al. (2022). Quantum dots’ size matters for balancing their quantity and quality in label materials to improve lateral flow immunoassay performance for C-reactive protein determination. Biosens. Bioelectron. 199, 113892. doi:10.1016/J.BIOS.2021.113892
Gao, F., Liu, Y., Lei, C., Liu, C., Song, H., Gu, Z., et al. (2021b). The role of dendritic mesoporous silica nanoparticles’ size for quantum dots enrichment and lateral flow immunoassay performance. Small Methods 5, 2000924. doi:10.1002/smtd.202000924
Gao, F., Ye, S., Huang, L., and Gu, Z. (2024). A nanoparticle-assisted signal-enhancement technique for lateral flow immunoassays. J. Mater. Chem. B 12, 6735–6756. doi:10.1039/d4tb00865k
Huang, L., Liao, T., Wang, J., Ao, L., Su, W., and Hu, J. (2018). Brilliant pitaya-type silica colloids with central-radial and high-density quantum dots incorporation for ultrasensitive fluorescence immunoassays. Adv. Funct. Mater. 28, 1705380. doi:10.1002/adfm.201705380
Huang, Z., Huang, H., Hu, J., Xia, L., Liu, X., Qu, R., et al. (2024). A novel quantitative urine LAM antigen strip for point-of-care tuberculosis diagnosis in non-HIV adults. J. Infect. 88, 194–198. doi:10.1016/j.jinf.2023.11.014
Li, J., Li, Z., Wang, B., Yu, Q., Wu, T., Wang, C., et al. (2025). Electropositive magnetic fluorescent nanoprobe-mediated immunochromatographic assay for the ultrasensitive and simultaneous detection of bacteria. Adv. Sci., 2412421. doi:10.1002/advs.202412421
Lin, L., Liu, A., Zhao, W., Yang, Y., Zhu, D., Dong, B., et al. (2024). Multihierarchical regulation to achieve quantum dot nanospheres with a photoluminescence quantum yield close to 100%. J. Am. Chem. Soc. 146, 21348–21356. doi:10.1021/jacs.4c03308
Liu, Y., Zhan, L., Qin, Z., Sackrison, J., and Bischof, J. C. (2021). Ultrasensitive and highly specific lateral flow assays for point-of-care diagnosis. ACS Nano 15, 3593–3611. doi:10.1021/acsnano.0c10035
Mariathas, M., Allan, R., Ramamoorthy, S., Olechowski, B., Hinton, J., Azor, M., et al. (2019). True 99th centile of high sensitivity cardiac troponin for hospital patients: prospective, observational cohort study. BMJ 364, 1729. doi:10.1136/bmj.l729
Neumann, J. T., Twerenbold, R., Ojeda, F., Sörensen, N. A., Chapman, A. R., Shah, A. S. V., et al. (2019). Application of high-sensitivity troponin in suspected myocardial infarction. N. Engl. J. Med. 380, 2529–2540. doi:10.1056/NEJMoa1803377
Rink, S., and Baeumner, A. J. (2023). Progression of paper-based point-of-care testing toward being an indispensable diagnostic tool in future healthcare. Anal. Chem. 95, 1785–1793. doi:10.1021/acs.analchem.2c04442
Rong, Z., Wang, Q., Sun, N., Jia, X., Wang, K., Xiao, R., et al. (2019). Smartphone-based fluorescent lateral flow immunoassay platform for highly sensitive point-of-care detection of Zika virus nonstructural protein 1. Anal. Chim. Acta 1055, 140–147. doi:10.1016/j.aca.2018.12.043
Schary, W., Paskali, F., Rentschler, S., Ruppert, C., Wagner, G. E., Steinmetz, I., et al. (2022). Open-source, adaptable, all-in-one smartphone-based system for quantitative analysis of point-of-care diagnostics. Diagnostics 12, 589. doi:10.3390/diagnostics12030589
Thygesen, K., Alpert, J. S., Jaffe, A. S., Chaitman, B. R., Bax, J. J., Morrow, D. A., et al. (2019). Fourth universal definition of myocardial infarction (2018). Eur. Heart J. 40, 237–269. doi:10.1093/eurheartj/ehy462
Truong, P. L., Yin, Y., Lee, D., and Ko, S. H. (2023). Advancement in COVID-19 detection using nanomaterial-based biosensors. Exploration 3, 20210232. doi:10.1002/EXP.20210232
Vaduganathan, M., Mensah, G. A., Turco, J. V., Fuster, V., and Roth, G. A. (2022). The global burden of cardiovascular diseases and risk: a compass for future Health. J. Am. Coll. Cardiol. 80, 2361–2371. doi:10.1016/j.jacc.2022.11.005
Wang, Y., Nor, Y. A., Song, H., Yang, Y., Xu, C., Yu, M., et al. (2016). Small-sized and large-pore dendritic mesoporous silica nanoparticles enhance antimicrobial enzyme delivery. J. Mater. Chem. B 4, 2646–2653. doi:10.1039/c6tb00053c
Wang, Y., Song, H., Yang, Y., Liu, Y., Tang, J., and Yu, C. (2018). Kinetically controlled dendritic mesoporous silica nanoparticles: from dahlia-to pomegranate-like structures by micelle filling. Chem. Mater. 30, 5770–5776. doi:10.1021/acs.chemmater.8b02712
Xia, X., Liu, Z., Du, G., Li, Y., and Ma, M. (2010). Structural evolution and photoluminescence of zinc-blende CdSe-based CdSe/ZnS nanocrystals. J. Phys. Chem. C 114, 13414–13420. doi:10.1021/jp100442v
Xiao, M., Tian, F., Liu, X., Zhou, Q., Pan, J., Luo, Z., et al. (2022). Virus detection: from state-of-the-art laboratories to smartphone-based point-of-care testing. Adv. Sci. 9, 2105904. doi:10.1002/advs.202105904
Yang, X., Cheng, X., Wei, H., Tu, Z., Rong, Z., Wang, C., et al. (2023). Fluorescence-enhanced dual signal lateral flow immunoassay for flexible and ultrasensitive detection of monkeypox virus. J. Nanobiotechnology 21, 450. doi:10.1186/s12951-023-02215-4
Yang, Y., Bernardi, S., Song, H., Zhang, J., Yu, M., Reid, J. C., et al. (2016). Anion assisted synthesis of large pore hollow dendritic mesoporous organosilica nanoparticles: understanding the composition gradient. Chem. Mater. 28, 704–707. doi:10.1021/acs.chemmater.5b03963
Yu, M., Niu, Y., Zhang, J., Zhang, H., Yang, Y., Taran, E., et al. (2016). Size-dependent gene delivery of amine-modified silica nanoparticles. Nano Res. 9, 291–305. doi:10.1007/s12274-015-0909-5
Zhan, L., Guo, S., Song, F., Gong, Y., Xu, F., R. Boulware, D., et al. (2017). The role of nanoparticle design in determining analytical performance of lateral flow immunoassays. Nano Lett. 17, 7207–7212. doi:10.1021/acs.nanolett.7b02302
Keywords: mesoporous, pore size, quantum dots, lateral flow immunoassay, smartphone-based analysis
Citation: Ye S, Xu C, Li H, Feng S, Wang Y and Gao F (2025) Enhancing lateral flow immunoassay performance for cardiac troponin I detection with pore-size tailored silica nanoparticles and smartphone-based “AdaptiScan” analysis. Front. Bioeng. Biotechnol. 13:1568719. doi: 10.3389/fbioe.2025.1568719
Received: 30 January 2025; Accepted: 06 March 2025;
Published: 26 March 2025.
Edited by:
Shevanuja Theivendran, The University of Queensland, AustraliaReviewed by:
Shanshan Shi, University of South Carolina, United StatesCopyright © 2025 Ye, Xu, Li, Feng, Wang and Gao. This is an open-access article distributed under the terms of the Creative Commons Attribution License (CC BY). The use, distribution or reproduction in other forums is permitted, provided the original author(s) and the copyright owner(s) are credited and that the original publication in this journal is cited, in accordance with accepted academic practice. No use, distribution or reproduction is permitted which does not comply with these terms.
*Correspondence: Yan Wang, eWFud2FuZ0BjYXUuZWR1LmNu; Fang Gao, Zmdhb0B1c3N0LmVkdS5jbg==
Disclaimer: All claims expressed in this article are solely those of the authors and do not necessarily represent those of their affiliated organizations, or those of the publisher, the editors and the reviewers. Any product that may be evaluated in this article or claim that may be made by its manufacturer is not guaranteed or endorsed by the publisher.
Research integrity at Frontiers
Learn more about the work of our research integrity team to safeguard the quality of each article we publish.