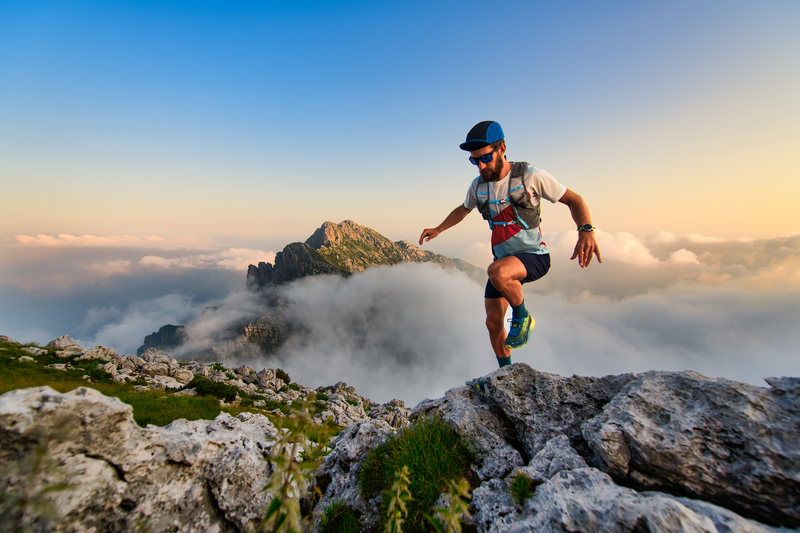
94% of researchers rate our articles as excellent or good
Learn more about the work of our research integrity team to safeguard the quality of each article we publish.
Find out more
OPINION article
Front. Bioeng. Biotechnol.
Sec. Cell and Gene Therapy
Volume 13 - 2025 | doi: 10.3389/fbioe.2025.1563878
This article is part of the Research Topic Design Strategies and Equipment Requirements for Efficient Process Development and Robust Manufacturing of Cell Therapies View all articles
The final, formatted version of the article will be published soon.
You have multiple emails registered with Frontiers:
Please enter your email address:
If you already have an account, please login
You don't have a Frontiers account ? You can register here
1IntroductionCell and gene therapies (CGTs) are new treatment modalities with demonstrated clinical results against a wide range of hard-to-treat diseases.[1, 2] They are either ex vivo treatments, obtained by manipulating cells in a laboratory before returning them to the patient, or in vivo applications, that involve direct injection of genetic material into the bloodstream or a target organ.[3] Cell and gene therapy (CGT) products are significantly different from previous generations of biologics, such as recombinant proteins or vaccines, and have been challenging the production capabilities, supply chain and business models of the pharmaceutical industry.[4, 5] With the CGT market still in its infancy, even a decade after the first market approvals, three factors related to their manufacturing have been limiting the broad adoption of CGT products in the clinic: 1) the highly variable starting materials due to the personalised nature of the treatments[6, 7], 2) the diversity and complexity of their production processes[8], and 3) the lack of fit-for-purpose tools supporting scalable supply at the commercial stage[9]. The combination of these three factors leads to limited availability and high cost of CGTs, making it challenging to reach commercial success while supporting broad and equitable patient access.[3, 10]To tackle this challenge, the authors of this article partnered in the PAT4CGT consortium that aims to develop a miniaturised process analytical technology (PAT) platform, tailored specifically for CGT manufacturing. Advanced PAT is critical for process understanding in R&D and is also becoming a key tool for process monitoring during clinical and commercial manufacturing.[11, 12] This trend is aligned with the concept of Industry 4.0, that aims to transform manufacturing and production systems through the integration of advanced digital technologies.[13] Adoption of Industry 4.0 principles in CGT is a paradigm shift, introducing cutting-edge, digital technologies, and moving away from centralised, manual and paper-led, towards distributed, automated and knowledge-driven CGT manufacturing processes.[14] In our opinion, digital solutions and innovative approaches to PAT that support scalable production are essential to deliver on the promise of these novel treatment modalities. Thus, the goal of the PAT4CGT project is to lay the foundation for minimally-invasive monitoring of critical process parameters (CPPs) in CGT. To this end, we develop a standalone, closed, automated, and miniaturised sensor technology platform for at line monitoring of CPPs, suited for applications in CGT manufacturing.2Closed, automated and single-use tools for cell culture and processingWe believe that three layers of innovation are necessary to achieve the transformation towards CGT 4.0 (Figure 1). The first and fundamental layer consists of closed, automated and single-use tools that perform two unit operations at the core of all bioprocesses: cell culture[15] and cell processing[16]. These key steps are traditionally performed in bioreactors and centrifugation systems, respectively. Single-use and closed tools have become the standard in small- and mid-scale production of biologics and are increasingly used in large scale processes.[17] While there are single-use technologies implemented in large-scale upstream processes, single-use centrifugation systems remain hard to scale. Overall, there is a critical need for novel approaches[18] that take into consideration the unique properties that set CGT products apart from previous generations of biopharmaceutical products.[19, 20]In the case of autologous, gene-edited cell therapies, innovative solutions are needed to perform the complex, multi-step processes in a streamlined sequence of unit operations. Replacing manual operations with automation in closed systems[21, 22] has the potential to increase process standardisation, robustness, scalability and reproducibility while lowering labour and clean room infrastructure costs.[23-25] There is empirical evidence[26] that process automation[27] can substantially reduce the “hands-on” operator time required for their manufacturing of CAR T-cell therapies.[28] Similarly, higher transduction and lower variability between batches were obtained for the production of a haemopoietic stem cell therapy using a closed, semi-automated approach, when compared to the standard manual process.[29] Novel closed and automated solutions enabling end-to-end manufacturing of autologous cell therapies will be necessary to transform the field and enable point of care production. While modular approaches are being implemented, we believe bringing all unit operations into a single device, and thus removing transfer of cells between tools, will lead to higher process yields and product quality. Solutions supporting ex vivo cell manipulations across a range of volumes and cell numbers will also be instrumental in unlocking the full potential of these personalised treatments. Furthermore, scalability to a full patient dose within the same device has the potential to significantly reduce the costs and time currently invested in technology transfer during the transition from pre-clinical to commercial stage.In the case of gene therapy, the cryopreservation and thawing of the master cell bank is a critical first step in the manufacturing process. Advanced methods that allow closed handling of cell banks use either the (high cell density) cell bag-based approach or a microfluidic-based system. Immortalised cell lines (e.g. HEK-derived cells) are already characterised and automated cell culture technologies exist for viral vector manufacturing.[30] However, the industry is still lacking fully automated workflows covering all steps, from cell expansion, virus production and purification up to the final formulation.[31] The traditional tools currently used to perform these unit operations, such as flatware flask for cell culture or ultracentrifugation for virus purification, are unsatisfactory as they not only hinder scalability but also lack the possibility of continuous data acquisition. 3Miniaturised sensors for process monitoringThe middle layer of innovation towards CGT 4.0 is composed of sensor solutions that can measure CPPs in real-time, providing vital information about the process status. Monitoring capabilities are critical to capture data into a record that is used in process development or required for batch documentation but also to provide the necessary inputs for process control.[32] The information about CPPs facilitates decision making through early detection of deviations and out of specification (OOS) events. This can enable timely intervention to improve the manufacturing success rate, as well as minimisation of losses associated with batch failure. To achieve this, a variety of offline, at line and inline tools exist but their integration into CGT processes remains questionable.[12] There are currently two concurring PAT approaches: (i) measuring a sample of the process with a traditionally offline, high-precision method (e.g. HPLC-MS) but automating the sampling process, or (ii) relying on an in situ method (e.g. spectroscopy) that often has an indirect measurement principle and requires extensive data analysis but can be applied in a closed system without the risk of contamination. In our opinion, sensor arrays for the monitoring of traditional biologics’ production – often limited to temperature, pH, dissolved oxygen and CO2 – are insufficient to characterise the status of a complex CGT production batch because tracking of cell metabolism is required for process development.[33-36] While relying on historical process data to design a medium supplementation strategy, the absence of continuous – or at least frequent – monitoring of cell metabolism limits process flexibility to adjust media components when important changes occur, hindering productivity or product quality. Inline spectroscopic sensors, such as infrared, Raman and dielectric spectroscopy, offer capabilities to monitor, nutrients, metabolites[37-40] or cell count.[41, 42] However, these methods are influenced by non-specific (background) variations.[41] Moreover, data interpretation, validation and software integration into process control, as well as the physical integration of a probe into innovative, small-scale manufacturing systems of CGTs are challenging.[41]The limitations due to background signals with the above-mentioned methods can be overcome by the use of a specific recognition element, such as an antibody-based or enzymatic biosensor.[43, 44] These, however, are at line methods that require a representative sample. The sampling technique must be tailored to the unique requirements of CGT production, mainly to single-use applications, across a wide range of process scales. Sterile sampling opens the possibility for further downstream processing and offline analytics. In general, the combination of automated, in situ collection of samples from a manufacturing platform in a closed, single-use environment with a highly precise, specific method has the potential to lower the risk of contamination associated with manual sampling and provide continuous/frequent measurements. This will play an important role in increasing the availability of data for subsequent analysis. 4Digital twin for process knowledge integration and controlThis leads us to the top layer of CGT 4.0, which is a data analytics solution allowing for the transformation of the information, collected by the analytical tools, into actionable knowledge, to efficiently manage the process lifecycle. In our opinion, a mathematical model of the manufacturing process, also known as a “digital twin”, is an essential tool for knowledge integration, with the aim of enabling predictive control of the critical quality attributes (CQAs) by adjusting the CPPs.[45] Historically, experimental data is used for mechanistic modelling approaches (e.g. material balance of substrates and metabolites) to describe microbial systems.[46] These methods have been successfully adapted to more complex cases, such as Chinese hamster ovary (CHO) cells.[47, 48] The mechanistic assumptions are not universal but the different modelling workflows can be transferred between cell types.[49] However, their imminent translation to CGT processes is limited by the generally low process understanding of CGTs.[50-52] Therefore, the prerequisite of developing accurate predictive models is to clearly define, then capture the time-resolved values of the CPPs and CQAs of CGT processes,[53] for which automated, in-situ analytical solutions can play a central role.In our view, purely data-driven, neural network methods could be used to develop models of CGT processes.[54, 55] For the generation of the large amounts of data that are necessary to train such models, novel, automated cell culturing tools could resolve the bottleneck of current production capacities. Another potential solution to the limited availability of data is to apply more sophisticated approaches and algorithms, such as hybrid modelling. Hybrid modelling combines mechanistic representations with data-driven methods.[56] For instance, the long-short term memory[57] and physics informed approaches,[58] as well as the reinforced learning-based methods.[59] However, the missing connection between the variability in the starting material (e.g. variability in cell phenotype between patients) and the process outcome, in the case of autologous cell therapies, poses an extra challenge. While classical mechanistic models can potentially describe the effect of nutrients and metabolites on cell growth, completely novel mechanistic representations are needed to describe cytotoxicity and similar efficacy CQAs for other CGTs. All things considered, hybrid modelling will be the approach that, in our opinion, will eventually prevail in the field of CGT, as its ability to incorporate simpler process knowledge, unconventional process parameters and mechanistic descriptions of efficacy CQAs allows users to achieve the desired prescriptive process control, as a key element of CGT 4.0.Naturally, as with every novel technique, an acceptable solution to validate these models, depending on the model’s importance for the process, has to be found.[60] Active discussions in the filed indicate that more time is needed to establish a universal validation approach for good manufacturing practice (GMP) compliance.[61, 62] However, in our view, the definition of suitable regulation is a technical question that can be addressed through collaboration of all parties concerned.5ConclusionsWe conclude that innovation across three layers is key to enable robust and scalable manufacturing of CGT products: closed and automated tools, robust and frequent process measurements and data analytics will enable the development and execution of well-characterised and adaptive production methods. The integration of these three layers into cohesive systems requires a multidisciplinary approach combining a profound understanding of the underlying biology as well as engineering skills to identify relationships between CPPs and CQAs and compile all process knowledge in a digital twin. Considering the complexity of the problem at hand, we wish to highlight the important role of innovation management and collaboration between experts from very diverse fields. Product engineering and design, cell biology and bioprocesses, sensors development, data modelling and material sciences, as well as end users and regulators of the technologies all need to come together to guide the industry towards the adoption of meaningful Industry 4.0 concepts applicable to CGT products, in order to provide novel therapeutic options to a vast number of patients.
Keywords: Cell and Gene Therapy, Automation, Sensors, PAT, Single-use, Digital Twin, manufacturing, Viral vector
Received: 20 Jan 2025; Accepted: 07 Mar 2025.
Copyright: © 2025 Szarzynski, Spadiut, Reisbeck, Jobst, Paterson, Kamenskaya, Gateau, Lesch, Henry and Kozma. This is an open-access article distributed under the terms of the Creative Commons Attribution License (CC BY). The use, distribution or reproduction in other forums is permitted, provided the original author(s) or licensor are credited and that the original publication in this journal is cited, in accordance with accepted academic practice. No use, distribution or reproduction is permitted which does not comply with these terms.
* Correspondence:
Bence Kozma, Institute of Chemical, Environmental and Bioscience Engineering, TU Wien, Vienna, Austria
Disclaimer: All claims expressed in this article are solely those of the authors and do not necessarily represent those of their affiliated organizations, or those of the publisher, the editors and the reviewers. Any product that may be evaluated in this article or claim that may be made by its manufacturer is not guaranteed or endorsed by the publisher.
Research integrity at Frontiers
Learn more about the work of our research integrity team to safeguard the quality of each article we publish.