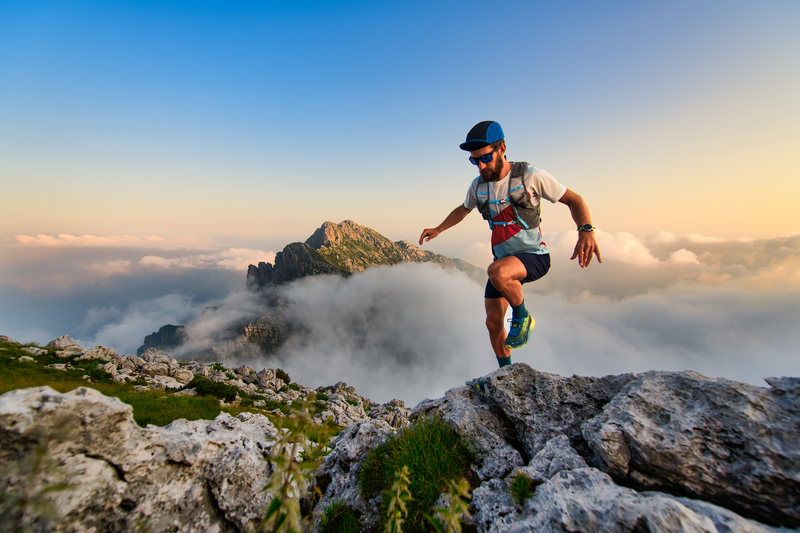
95% of researchers rate our articles as excellent or good
Learn more about the work of our research integrity team to safeguard the quality of each article we publish.
Find out more
ORIGINAL RESEARCH article
Front. Bioeng. Biotechnol.
Sec. Biosensors and Biomolecular Electronics
Volume 13 - 2025 | doi: 10.3389/fbioe.2025.1554432
The final, formatted version of the article will be published soon.
You have multiple emails registered with Frontiers:
Please enter your email address:
If you already have an account, please login
You don't have a Frontiers account ? You can register here
Purpose: Modalities for myopia control, such as orthokeratology, repeated lowintensity red light (RLRL) treatment, and low-concentration atropine, have become popular topics. However, the effects of these 3 modalities on ocular surface health remain unclear. The tear meniscus height (TMH), a crucial criterion for evaluating ocular surface health and diagnosing dry eye, is conventionally measured via manual demarcation of ocular surface images, which is inefficient and involves subjective judgment. Therefore, this study sought to establish a deep learning model for automatic TMH measurement on ocular surface images to improve the efficiency and accuracy of the initial screening of dry eye associated with myopia control modalities.Methods: To establish a model, 1200 ocular surface images captured with an OCULUS Keratograph 5M were collected. The tear meniscus area on the image was initially marked by one experienced ophthalmologist and verified by the other. The whole image dataset was divided into a training set (70%), a validation set (20%), a test set (10%), and an external validation set (100 ocular surface images) for model construction. The deep learning model was applied to ocular surface imaging data from previous clinical trials using orthokeratology, RLRL therapy, and 0.01% atropine for myopia control. TMHs at follow-ups were automatically measured by the deep learning model.Two hundred training iterations were performed to establish the model. At the 124th iteration, the IoU of the validation set peaked at 0.913, and the parameters of the model were saved for the testing process. The model IoU was 0.928 during testing. The AUC of the ROC curve was 0.935, and the R2 of the linear regression analysis was 0.92. The good performance and comprehensive validation of the model warrants its application to automatic TMH measurement in clinical trials of myopia control. There were no significant changes in the TMH during the follow-up period after treatment with orthokeratology, RLRL, or 0.01% atropine.A deep learning model was established for automatic measurement of the TMH on Keratograph 5M-captured ocular surface images. This model demonstrated high accuracy, great consistency with manual measurements, and applicability to the initial screening of dry eye associated with myopia control modalities.
Keywords: deep learning, Tear meniscus height, Automation, ocular surface image, Myopia control, dry eye
Received: 02 Jan 2025; Accepted: 02 Apr 2025.
Copyright: © 2025 Zhang, Rong, Hei, Liu, He, Du, Wei and Zhang. This is an open-access article distributed under the terms of the Creative Commons Attribution License (CC BY). The use, distribution or reproduction in other forums is permitted, provided the original author(s) or licensor are credited and that the original publication in this journal is cited, in accordance with accepted academic practice. No use, distribution or reproduction is permitted which does not comply with these terms.
* Correspondence:
Yan Zhang, Tianjin Medical University Eye Hospital, Tianjin, China
Disclaimer: All claims expressed in this article are solely those of the authors and do not necessarily represent those of their affiliated organizations, or those of the publisher, the editors and the reviewers. Any product that may be evaluated in this article or claim that may be made by its manufacturer is not guaranteed or endorsed by the publisher.
Research integrity at Frontiers
Learn more about the work of our research integrity team to safeguard the quality of each article we publish.