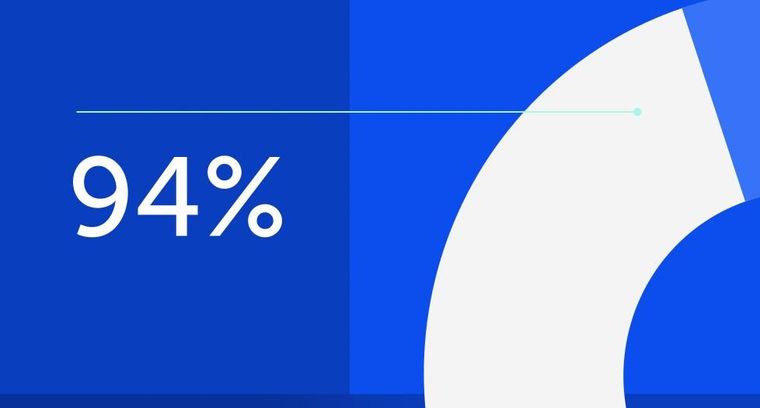
94% of researchers rate our articles as excellent or good
Learn more about the work of our research integrity team to safeguard the quality of each article we publish.
Find out more
ORIGINAL RESEARCH article
Front. Bioeng. Biotechnol.
Sec. Biosafety and Biosecurity
Volume 13 - 2025 | doi: 10.3389/fbioe.2025.1430222
The final, formatted version of the article will be published soon.
Select one of your emails
You have multiple emails registered with Frontiers:
Notify me on publication
Please enter your email address:
If you already have an account, please login
You don't have a Frontiers account ? You can register here
Introduction: There has been an increasing focus on object segmentation within remote sensing images in recent years due to advancements in remote sensing technology and the growing significance of these images in both military and civilian realms. In these situations, it is critical to accurately and quickly identify a wide variety of objects. In many computer vision applications, scene recognition in aerial-based remote sensing imagery presents a common issue. Method: However, several challenging elements make this work especially difficult: (i) Different objects have different pixel densities; (ii) objects are not evenly distributed in remote sensing images; (iii) objects can appear differently depending on viewing angle and lighting conditions; and (iv) there are fluctuations in the number of objects, even the same type, in remote sensing images. Using a synergistic combination of Markov Random Field (MRF) for accurate labeling and Alex Net model for robust scene recognition, this work presents a novel method for the identification of remote sensing objects. During the labeling step, the use of MRF guarantees precise spatial contextual modeling, which improves comprehension of intricate interactions between nearby aerial objects. By simultaneously using deep learning model, the incorporation of Alex Net in the following classification phase enhances the model's capacity to identify complex patterns in aerial images and adapt to a variety of object attributes. Results: Experiments show that our method performs better than others in terms of classification accuracy and generalization, indicating its efficacy analysis on benchmark datasets such as UC Merced Land Use and AID. Discussion: Several performance measures were calculated to assess the efficacy of the suggested technique, including accuracy,error, and F1-Score. The assessment findings show a remarkable recognition rate of around 97.90% and 98.90%, on the AID and the UC Merced Land datasets, respectively. This paper introduces a novel hybrid approach combining Markov Random Field (MRF)-based segmentation and AlexNet convolutional neural network architecture for multi-object recognition in high-resolution remote sensing data. Experiments on benchmark datasets, including UC Merced and AID, demonstrate the efficacy of the system, achieving segmentation accuracies of 91.18% and 91.67%, and scene classification accuracies of 98.90% and 97.90%, respectively.
Keywords: Multi-Modal, remote sensing, Multi-objects, autonomous devices Deep learning, Computer Vision, posterior probability, Likelihood estimation, scene analysis
Received: 09 May 2024; Accepted: 15 Apr 2025.
Copyright: © 2025 Naseer, Almudawi, Aljuaid, Alazeb, Alqahtani, Algarni, Jalal and Liu. This is an open-access article distributed under the terms of the Creative Commons Attribution License (CC BY). The use, distribution or reproduction in other forums is permitted, provided the original author(s) or licensor are credited and that the original publication in this journal is cited, in accordance with accepted academic practice. No use, distribution or reproduction is permitted which does not comply with these terms.
* Correspondence:
Ahmad Jalal, Air University, Islamabad, Pakistan
Hui Liu, University of Bremen, Bremen, 28359, Bremen, Germany
Disclaimer: All claims expressed in this article are solely those of the authors and do not necessarily represent those of their affiliated organizations, or those of the publisher, the editors and the reviewers. Any product that may be evaluated in this article or claim that may be made by its manufacturer is not guaranteed or endorsed by the publisher.
Supplementary Material
Research integrity at Frontiers
Learn more about the work of our research integrity team to safeguard the quality of each article we publish.