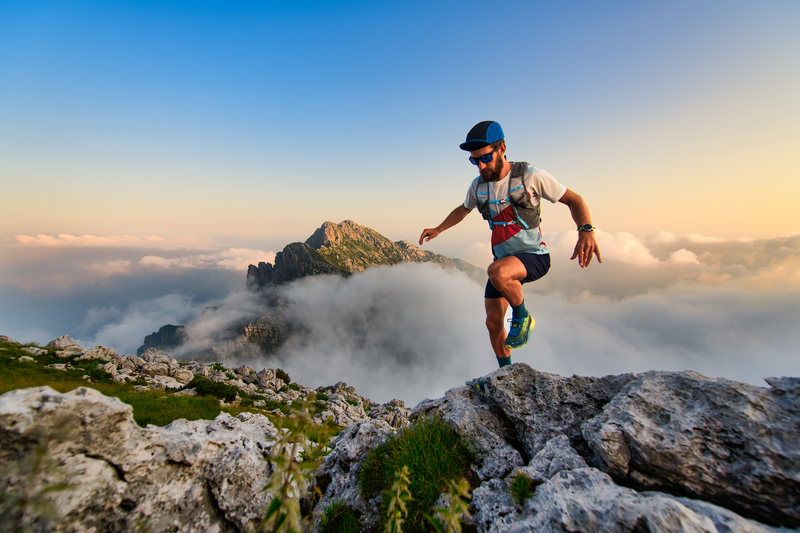
94% of researchers rate our articles as excellent or good
Learn more about the work of our research integrity team to safeguard the quality of each article we publish.
Find out more
ORIGINAL RESEARCH article
Front. Bioeng. Biotechnol.
Sec. Biomechanics
Volume 13 - 2025 | doi: 10.3389/fbioe.2025.1418940
The final, formatted version of the article will be published soon.
You have multiple emails registered with Frontiers:
Please enter your email address:
If you already have an account, please login
You don't have a Frontiers account ? You can register here
Physical action prediction or exercise recognition is an important factor for the healthcare industry around the globe. It is prevalent for improved human lifestyle outcomes. This research brings an innovation for physical action prediction using multimodal methodology and machine learning techniques, where we integrate novel descriptor extraction methods to improve the predictive power and ability in healthcare domain.Methods: This article presents a unique model for processing physical activities-based data, including cameras-based captures, wearable sensors-based signals, and ambient-based gestures. A machine learning-related method named the hidden Markov model has been incorporated to speed up the prediction of physical action. Additionally, we have proposed unique methods to extract the descriptors from multiple types of health data. A few experiments over benchmarked datasets have been conducted for data training and validation. The physical exercise prediction system has been assessed using multiple evaluation criteria, such as, accuracy, precision, recall, and F1-score.Our system outperformed the conventional methods, achieving accuracy rates of 81.67% and 80.00% over Berkeley-MHAD and LARa datasets, respectively. The precision and recall metrics demonstrated the system's ability to recognize the exercises performed. The wide-ranging F1-score has also shown outstanding performance by obtaining better results.The proposed system reveals the prospects of multisensory devices and machine learning application for physical action prediction. The consistent efficiency of our system across different datasets has offered new possibilities for the detection and interpolation of the unwanted event for quality lifestyle and good healthcare. This system promises to provide significant application projections and considerable progressions in healthcare domain.
Keywords: Exercise recognition, Hidden markov model, machine learning, Multimodal system, Multi-sensors, Physical Action Prediction
Received: 22 Apr 2024; Accepted: 19 Feb 2025.
Copyright: © 2025 Javeed, Shorfuzzaman, Sadiq, Rahman, Algarni and Jalal. This is an open-access article distributed under the terms of the Creative Commons Attribution License (CC BY). The use, distribution or reproduction in other forums is permitted, provided the original author(s) or licensor are credited and that the original publication in this journal is cited, in accordance with accepted academic practice. No use, distribution or reproduction is permitted which does not comply with these terms.
* Correspondence:
Touseef Sadiq, University of Agder, Kristiansand, 4604, Vest-Agder, Norway
Hameedur Rahman, Air University, Islamabad, Pakistan
Disclaimer: All claims expressed in this article are solely those of the authors and do not necessarily represent those of their affiliated organizations, or those of the publisher, the editors and the reviewers. Any product that may be evaluated in this article or claim that may be made by its manufacturer is not guaranteed or endorsed by the publisher.
Research integrity at Frontiers
Learn more about the work of our research integrity team to safeguard the quality of each article we publish.