- 1Graduate School of Engineering Science, Osaka University, Toyonaka, Japan
- 2Graduate School of Information Sciences, Hiroshima City University, Hiroshima, Japan
- 3Faculty of Human Ecology, Yasuda Women’s University, Hiroshima, Japan
Introduction: Many studies have investigated the manipulation of a virtual upper arm using electromyogram (EMG); however, these studies primarily used a machine learning model or trigger control for this purpose. Furthermore, most of them could only display the constant motion of the virtual arm because the motion to be displayed was selected by pattern recognition or trigger control. In addition, these studies did not examine changes in the electromyographic signals after experiencing the virtual arm. By contrast, we propose a real-time, continuous, learning-free avatar that manipulates the virtual arm with electromyogram signals or physio-avatar EMG biofeedback (EB). The goal of the physio-avatar EB system is to induce physiological changes through experiential interactions.
Methods: We explored the possibility of changing motor control strategies by applying the system to healthy individuals as a case study. An intervention method that provided an experience of a body different from one’s own was conducted on seven participants using a time-invariant calculation algorithm to determine the joint angles of the avatar. Control strategies for an indicator of the equilibrium point in the baseline and adaptation phases were determined to evaluate the physio-avatar EB intervention effect. The similarity of these BL and adaptation control strategies compared to those used during the washout period was assessed using the coefficient of determination. The accuracy and reliability of the virtual reality (VR) system were evaluated by comparison with existing studies and the required specs.
Results and Discussion: Changes in motor control strategies due to the physio-avatar EB system were observed in four experiments, where the participants gradually returned to their pre-intervention control strategies. This result can be attributed to the aftereffects caused by error learning. This implies that the developed system influenced their motor control strategies. The number of EMG acquisition bits was 16 bits, and the sampling rate was 1,000 Hz. The refresh rate of the head-mounted display was 90 Hz, and its resolution was
1 Introduction
The advancement of virtual reality (VR) has made it easier for general consumers to operate avatars representing themselves in VR spaces, leading to an increase in avatar-based research. With the diversification of technologies using avatars, the physical and cognitive modifications induced by avatar experiences need to be investigated (Hagita, 2022). Previous studies have suggested the possibility of cognitive modifications resulting from avatar experiences. Keizer et al. (2016) aimed to resolve the discrepancy between the actual body and the self-body image, which causes eating disorders, by inducing a sense of body ownership toward avatars with different body types. Ratan and Sah (2015) experimentally investigated the stereotype threat effect after gaming based on the avatar gender and found an improvement in math scores among women using male avatars. These studies indicate that the impact of avatar experiences can be retained in real spaces, potentially affecting brain processing. Avatar use can induce changes in the brain concerning bodily movement control and can thus be applied to stroke rehabilitation. This is supported by reports stating that avatar experiences induce a sense of body ownership and agency (Preston and Ehrsson, 2016; Waltemate et al., 2018; Tieri et al., 2015), suggesting the possibility of mistaking an avatar for one’s own body. An individual may undergo “error learning” or “reinforcement learning” by experiencing their avatar.
In the error learning process, one’s internal model, believed to be in the cerebellum, is modified to reduce discrepancies between the executed (or outcome) motion trajectory and the expected motion trajectory. During the learning process, stiffness is high initially; virtual trajectory control is prioritized, but model-like control is subsequently implemented, thus reducing performance error and enabling feedforward motion control (Osu et al., 2002). The aftereffects of error learning are those previously induced for rehabilitation through error augmentation therapy using robots or VR (Lo et al., 2010; Liu et al., 2018; Wei et al., 2005; Abdollahi et al., 2013; Porta et al., 2021). Avatar experiences can be used for rehabilitation if the aftereffects of error learning occur. Furthermore, amplifying weak electromyogram (EMG) signals can enable the use of reinforcement learning in systems applied to patients with paralysis. Reinforcement learning focuses on the appropriateness of the executed motion outcome rather than errors during motion; successful task completion is rewarded, such as with a sense of achievement. Important factors in reinforcement learning include the difference between actual and predicted rewards, task difficulty, and exploration opportunities. Learning advances when the obtained reward exceeds the predicted reward, so task difficulty should be set appropriately, and movement methods should be explored to maximize rewards (Schultz et al., 1993; Schultz et al., 1997; Tanaka et al., 2016; Dobkin et al., 2010). Thus, amplifying weak EMG signals to enable an individual to experience movement through an avatar as a substitute for paralyzed limbs can lead to reinforcement learning. Many studies have used EMG signals in virtual reality (Tigrini et al., 2024; Tigrini et al., 2023); KiNvis™ is a reinforcement learning-based therapeutic method that utilizes EMG signals as triggers for avatar motion. The avatar moves when the EMG signals obtained from the muscles performing the set movement exceed a set threshold (Kaneko, 2016).
We propose an EMG biofeedback (EB) system that projects an arm-shaped avatar in the VR space within a head-mounted display (HMD), allowing for the continuous, real-time EMG-based control of the avatar. This system is modeled based on the findings of our previous studies, where we obtained a human neuromuscular skeletal model by expressing stimulation intensities to opposing muscles as two variables: the electrical agonist–antagonist (EAA) muscles sum and ratio (Nagai et al., 2019; Matsui et al., 2014; Matsui et al., 2015; Matsui et al., 2022; Suzuki et al., 2024). Error learning can be implemented using this system by setting the avatar’s characteristics to differ from the user’s own, whereas reinforcement learning can be executed by amplifying the EMG signals for patients with weak signals. Several studies demonstrated the acquisition of EMG signals and controlling of virtual hand models supporting hand motion rehabilitation. Gieser et al. (2017), Yang et al. (2017), and MINDROVE (2024) used an armband device that acquires the EMG signals of eight muscles, estimates hand movements using a machine learning approach, and displays constant movements, like the KiNvis™, according to estimated results. The number of bits corresponding to the accuracy of the EMG data measurement was unknown, and the EMG acquisition rates ranged from 200 to 500 Hz. Because Gieser said that the EMG activity rate could be as high as 450 Hz (Gieser et al., 2017), this sampling rate was insufficient based on the sampling theorem. Suryanarayanan et al. acquired EMG signals for a single biceps muscle and estimated joint angles using a learning method (Suryanarayanan and Reddy, 1997) and displayed the angles of the virtual arm by EMG changes. EMG signals were acquired with 12 bits, and the sampling rate was 2000 Hz. This sampling rate is somewhat high. These reported devices do not have a fixed display system, and their refresh rate depends on the used display unit. In contrast to these studies, our physio-avatar EB is unique, in that it uses a real-time, continuous, learning-free model to manipulate the virtual arm using EMG with a sampling rate of 1,000 Hz.
This study aims to validate the system’s effectiveness as a precursor to future applications for patients with post-stroke paralysis. We conducted an experiment on seven healthy individuals to examine the impact of avatar experiences on motor control strategies before and after the intervention as a case study. Although reinforcement learning cannot be confirmed without testing with patients with paralysis, we first used this system to verify whether healthy individuals can adapt to the movement characteristics of the model-defined avatar and learn the characteristics associated with the defined model in the internal model. The system aims to enable both error learning and reinforcement learning using avatars. In addition, we evaluate the developed physio-avatar EB by comparing it with existing medical devices. We used the number of EMG acquisition bits and sampling rate for accuracy and reliability and used the HMD refresh rate and resolution for realistic quality.
1.1 Physio-avatar EB
Avatar therapy is the use of avatars for rehabilitation. A physio-avatar, as we have named it, is an avatar designed to induce physiological changes in humans through experience. In this study, we use EB with a physio-avatar, so the studied avatar is called a physio-avatar EB.
1.2 System
We developed the physio-avatar EB system (Figure 1), which enables users to experience a first-person avatar for one-degree-of-freedom (1-DOF) elbow joint movements (Okamoto et al., 2024). This system is primarily divided into a computational unit and a display unit. The computational unit is constructed using LabVIEW (NI) (NATIONAL INSTRUMENTS CORP., 2024a) on a computational PC and a data acquisition board PXIe6363 (NI) for 16-bit analog–digital conversion (NATIONAL INSTRUMENTS CORP., 2024b). Specifically, analog signals from an EMG acquisition device (WEB-5000, Nihon Kohden) (Masumoto et al., 2004) and an angle acquisition sensor goniometer (SG150, Biometrics Ltd.) (Biometrics Ltd, 2024) are converted into digital signals. These signals are then processed and computed in LabVIEW. The display unit consists of Unity (Unity Technologies) (Unity Technologies, 2024) installed on a display PC, Azure Kinect DK (Microsoft) (Microsoft, 2024), and a VIVE Pro (HTC) (HTC Corporation, 2024) HMD. The goal is for a user to achieve a sense of body ownership toward the avatar. The Azure Kinect DK kit is used to align the avatar in the VR space in the HMD according to the participant’s shoulder joint position and angle. The angle, dependent on the avatar characteristics calculated by the PC based on the EMG input, is sent to the display unit via transmission control protocol/internet protocol communication. In this study, the biceps brachii muscle was used as the flexor muscle and the triceps brachii muscle as the extensor muscle, and the positions of the surface electrodes were fixed based on the literature (Doheny et al., 2008). The ground electrode was attached to the head of the elbow.
Based on our previous research (Nagai et al., 2019; Matsui et al., 2014; Matsui et al., 2015; Matsui et al., 2022; Suzuki et al., 2024), the agonist–antagonist muscle ratio (AA ratio), an indicator of the equilibrium point in the human motion control, is input to the neuromuscular system (NMS) + musculoskeletal system (MSS) model (NMSS model) to calculate the virtual angle. The NMSS model integrates the transfer function of the NMS
Here,
In this system, due to the time needed to calculate the virtual angle from the AA ratio input (approximately 3
%40 NVC normalizes the EMG of flexors and extensors during activity to the EMG at 40
The calculated AA ratio and its difference from the initial AA ratio,
For the accuracy and reliability of physio-avatar EB, the number of EMG acquisition bits was 16, and the sampling rate was 1,000 Hz. This number of acquisition bits for an EMG was considered sufficient because 16-bit resolution is used in commercial medical devices, for example, the Tringo series (Delsys Incorporated) (Gieser et al., 2017; TRIGNO CENTRO, 2024). We estimated that the sampling rate was sufficient according to a sampling theorem. To achieve life-like quality, our HMD’s refresh rate was 90 Hz, which was the same as that of the commercial VR medical device KAGURA (mediVR Inc.) (medivr, 2024). The resolution was
2 Methods
2.1 Experimental method
The experiments in this study, which involved human participants, were conducted with approval from the Ethics Committee for Research Involving Human Subjects at the Graduate School of Engineering Science, Osaka University (Approval number: R3-3). Intervention using the physio-avatar EB system was performed on the participants, during which the following measurements were recorded: the AA ratio, AA sum, and elbow joint angle during identical cyclic movements before the intervention, after adaptation during the physio-avatar EB intervention (ADP), and immediately after the intervention [washout (WO)] (Figure 2). The abovementioned adaptation process occurs during physio-avatar EB intervention before ADP. The HMD was only worn during the intervention, and only data recording was conducted.
The cyclic movements before the intervention and WO were reciprocal movements performed with the eyes open. Target A was set at a point where the joint angle was 90
During the adaptation process in the physio-avatar EB intervention, given the lack of a function to place markers within the VR space, the participants were instructed to remember the hand positions where the avatar’s joint angle reached 90
All experiments were conducted with the physio-avatar EB’s NMSS model parameters, as shown in Table 1. Preliminary experiments showed that physio-avatar EB intervention with high natural frequencies of the NMSS model, namely,
Previous studies have qualitatively demonstrated changes in motor control strategies before and after interventions when the real body’s elbow joint could extend and flex during intervention under conditions where the hand was free (Ando et al., 2023; Ando et al., 2024; Ando et al., 2022). However, the effect on motor control strategies of somatosensory input, such as counterforce, also needs to be verified, necessitating the examination of the physio-avatar intervention effect when the hand position is fixed during the intervention. Therefore, the experiments in this study were conducted under both conditions (fixed and free hand position during intervention) (Figure 5). Participants E and G only participated under the fixed-hand-position intervention condition.
In the experiment, each participant was in a sitting position. The hand rest shown in Figure 3, which had fixed and movable platforms, was used to keep the upper arm immobile and the forearm movable. This setup enabled 1-DOF motion within the horizontal plane while compensating for gravity and maintaining a constant elbow joint position. The trunk was fixed using the belt shown in Figure 3 to eliminate the influence of posture differences on motor control strategies between BL and WO. The right shoulder joint was positioned at a horizontal flexion angle of 45
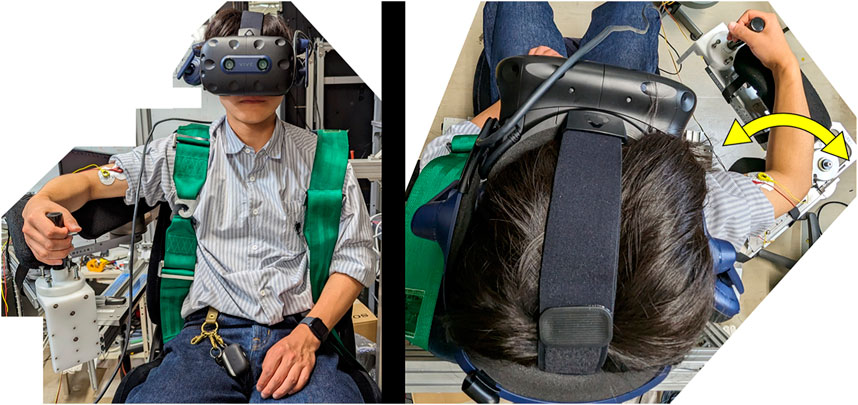
Figure 6. Actual images of a participant using the physio-avatar EB system (left: front view, right: top view).
2.2 Analysis method
The recorded AA ratio, AA sum, and joint angle (the virtual angle is used instead during ADP) were analyzed separately for the extension and flexion movements. A 1.8
The coefficient of determination (CoD) (square of Pearson’s product–moment correlation coefficient), an indicator of similarity between two datasets in statistics, was used for evaluation. The CoD was used to determine whether the motor control strategies were similar to those before the intervention. The waveform after averaging the BL AA ratio was defined as the BL motor control strategy. The CoD with the BL motor control strategy was calculated for all BL and WO cycles. Additionally, the average CoD for each BL cycle with respect to the BL motor control strategy was determined. The trajectories of the AA ratio and joint angle should generally align with the fact that the AA ratio approaches 1 (0) during extension (flexion); thus, the trajectory of the AA ratio should be somewhat similar for the same movement. Therefore, an excessively low average CoD for the BL motor control strategy for each BL cycle indicated possible issues with EMG acquisition, such as insufficient signal levels or noise. A threshold value of 0.25 was set for the average CoD, and evaluations were performed on experimental results where the average CoD of the BL motor control strategy for each BL cycle during flexion and extension exceeded 0.25. Although this threshold lacked a clear basis, it was set according to a previously published guideline that a “correlation coefficient up to 0.5 is considered sufficient” (Akoglu, 2018).
In the learning of the internal model, systems are used for the short- and long-term memory of motor control strategies (Osu et al., 2002). Time constants are used to explain the results of these memories, so the time constants in this study were determined by performing exponential approximation on all 100 WO cycles. The approximate exponential function is shown in Equation 5.
Because the AA sum was not a control variable during the physio-avatar EB intervention, the motor control strategy appeared not to change due to the physio-avatar EB intervention. However, studies on variability between movement cycles state that the variability of task-related control variables decreases, whereas the variability of non-task-related control variables does not decrease (Dal’Bello and Izawa, 2021). Human movement involves control variables, such as the equilibrium point and stiffness. During adaptation to the physio-avatar EB system, that equilibrium point is a task-related control variable, and stiffness is a non-task-related control variable. The variability of indicators related to a control variable (equilibrium point) may decrease, whereas the variability of indicators related to a non-control variable (stiffness) may increase. The waveform of the AA ratio was evaluated for the equilibrium point; for stiffness, the total sum of all samples of the AA sum was utilized as an evaluation index for BL and ADP. For the equilibrium point, the standard deviation of each sample of the AA ratio across all cycles was calculated during BL and ADP, and its average value served as an indicator of variability. As for stiffness, EMG variations increase at high intensities of muscle activity; the variation of the AA sum, which is the sum of the EMG signals, also increases at high intensities (Gasparic et al., 2023). Therefore, the coefficient of variation was utilized as an indicator of variability; the standard deviation of the total sum of the AA sum was normalized across all cycles using the average value for BL and ADP.
The variability indicators for the equilibrium point in BL and ADP were denoted as
Finally, whether the same movements were being performed during the BL and WO periods should be confirmed during the evaluation of the changes in motor control strategies due to avatar intervention. As in the analysis of the AA ratio, the waveform after averaging the joint angle was defined as the BL joint angle, and the CoD of the joint angle for all WO cycles was calculated. The trajectory of the joint angle had small errors between cycles. Therefore, only cycles where the CoD of the BL joint angle exceeded 0.95 were determined to include movements similar to the BL ones.
3 Results
The following indices are used to discuss the experimental results for both flexion and extension movements.
For CoD Angle (FX) and CoD Angle (EX), if the value of the first cycle is small, then the value of the second cycle is shown in parentheses. Additionally, experimental data that do not fit the exponential approximation are denoted with a “-” for the time constant. In that case, the Initial Value (BL) or Initial Value (ADP) and Constant Value (BL) or Constant Value (ADP) are shown as average values during WO. Additionally, for Participants C, D, E, and G under the Free condition and Participant F under both conditions, the average CoD values at BL are below 0.25, leading to their exclusion from evaluation. Thus, the experimental results considered for evaluation are those of Participants A and B under both conditions and those of Participants C, D, and E under the Fixed condition. The results are shown in Tables 2–6.
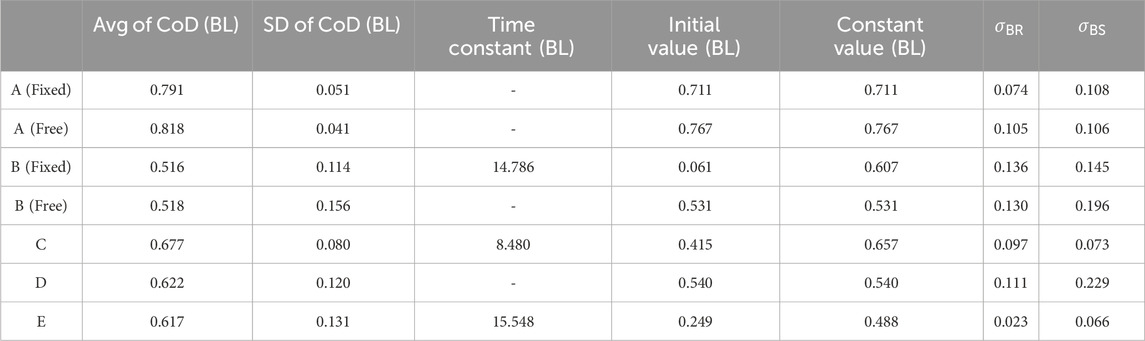
Table 3. Results during flexion movement: Avg of CoD (BL), SD of CoD (BL), Time Constant (BL), Initial Value (BL), Constant Value (BL),
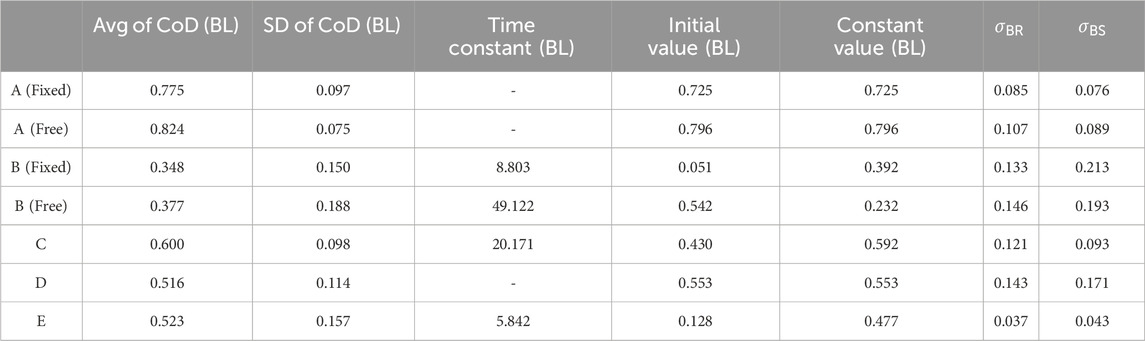
Table 4. Result during extension movement: Avg of CoD (BL), SD of CoD (BL), Time Constant (BL), Initial Value (BL), Constant Value (BL),
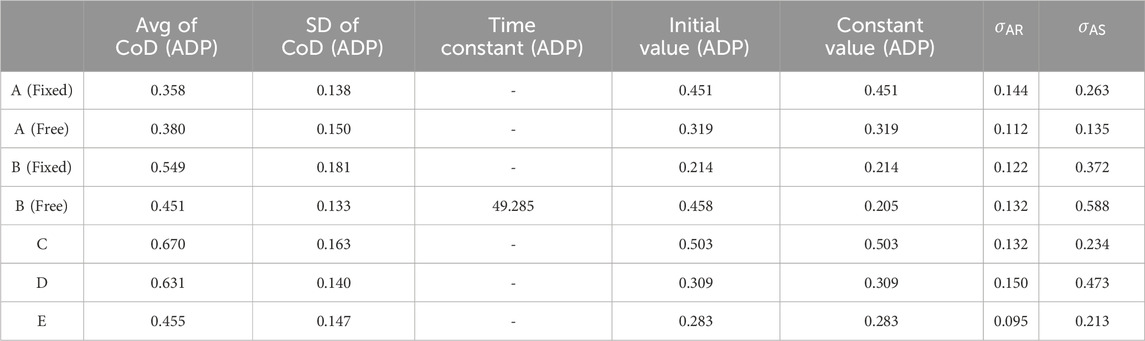
Table 5. Results during flexion movement: Avg of CoD (ADP), SD of CoD (ADP), Time Constant (ADP), Initial Value (ADP), Constant Value (ADP),
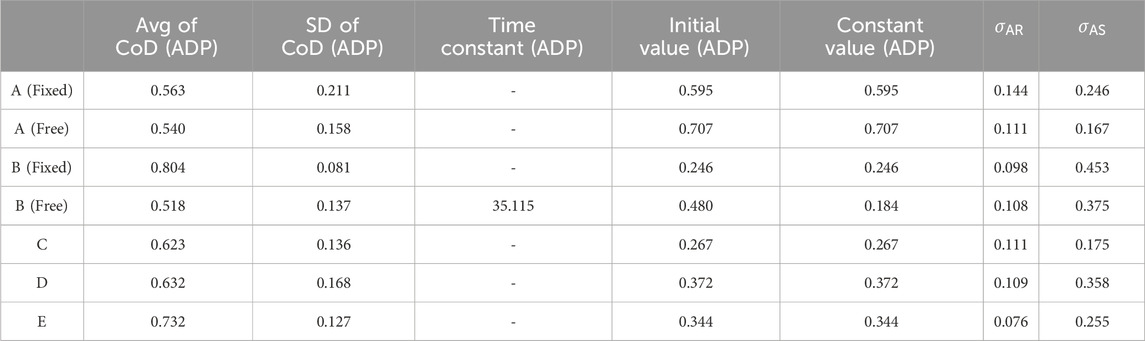
Table 6. Results during extension movement: Avg of CoD (ADP), SD of CoD (ADP), Time Constant (ADP), Initial Value (ADP), Constant Value (ADP),
Figure 7 shows sample experimental results under a converged exponential approximation curve of the CoD of the BL motor control strategy during WO. These are the results during flexion for Participant B (Fixed). The graph shows the CoD of the AA ratio of the BL motor control strategy during WO, the exponential approximation curve calculated from that CoD, Avg of CoD (BL), and Avg of CoD (BL)
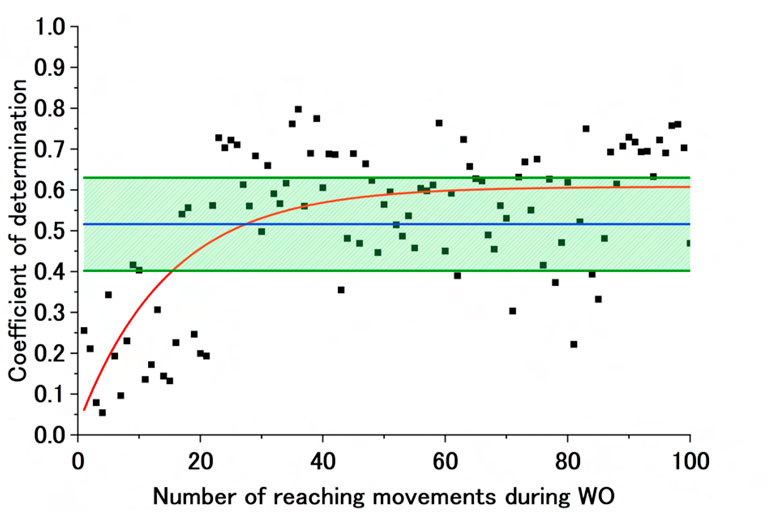
Figure 7. Sample exponential approximation curve (calculated) of the CoD of the BL motor control strategy during WO. The black dots represent the CoD of the BL motor control strategy, and the red line represents the failed approximated exponential function of the CoD of the BL motor control strategy. The blue line represents Avg of CoD (BL), and the green lines represent Avg of CoD (BL) + SD of CoD (BL) and Avg of CoD (BL)
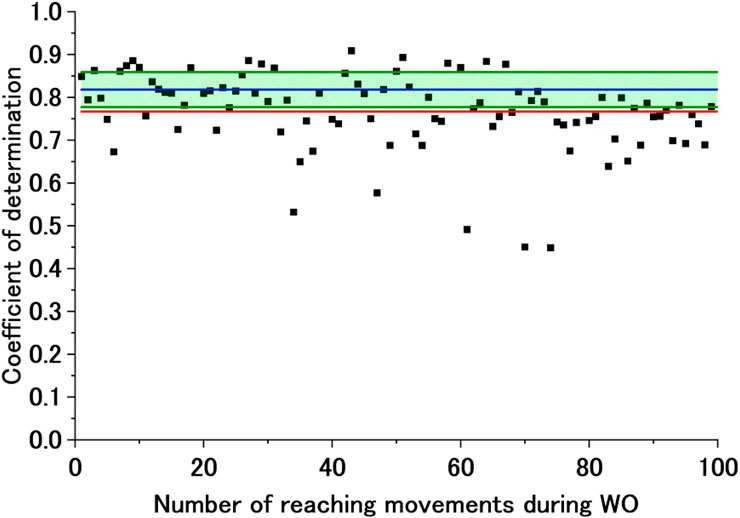
Figure 8. Sample exponential approximation curve (not calculated) of the CoD of the BL motor control strategy during WO. The black dots represent the CoD of the BL motor control strategy, and the red line represents the failed approximated exponential function of the CoD of the BL motor control strategy. The blue line represents Avg of CoD (BL), and the green lines represent Avg of CoD (BL) + SD of CoD (BL) and Avg of CoD (BL)
Figure 9 shows sample experimental results under a converged exponential approximation curve of the CoD of the ADP motor control strategy during WO. These are the results during extension for Participant B (Free). The graph shows the CoD of the AA ratio of the ADP motor control strategy during WO, the exponential approximation curve calculated from that CoD, and Avg of CoD (ADP)
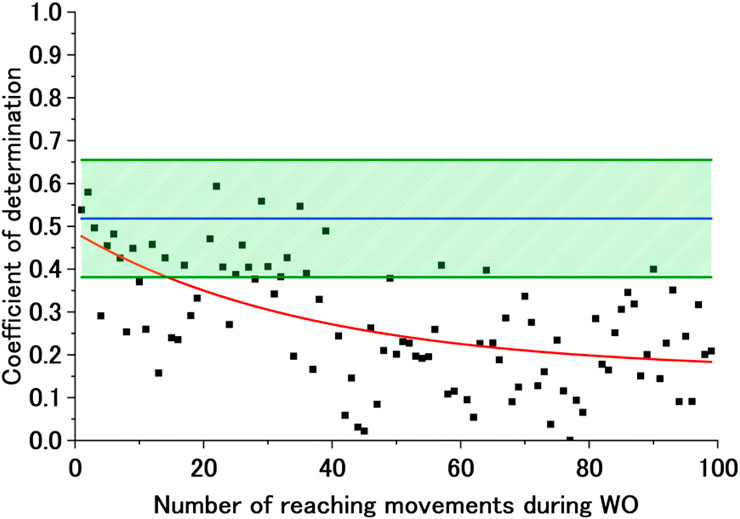
Figure 9. Sample exponential approximation curve (calculated) of the CoD of the ADP motor control strategy during WO. The black dots represent the CoD of the ADP motor control strategy, and the red line represents the failed approximated exponential function of the CoD of the ADP motor control strategy. The blue line represents Avg of CoD (ADP), and the green lines represent Avg of CoD (ADP) + SD of CoD (ADP) and Avg of CoD (ADP)
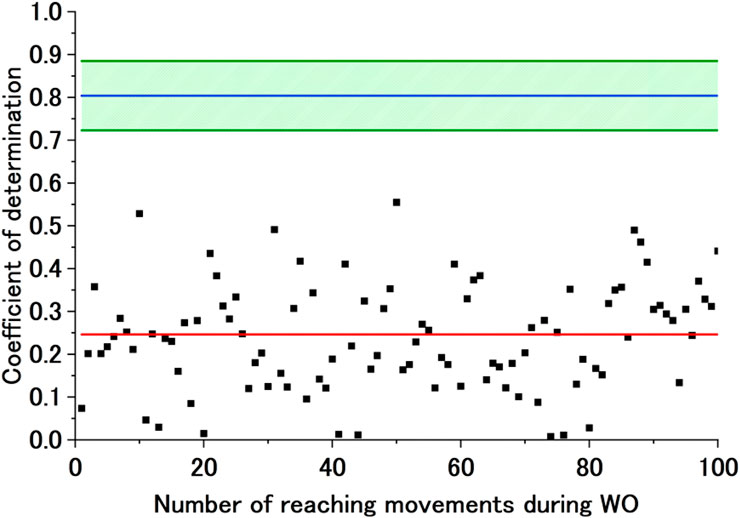
Figure 10. Sample exponential approximation curve (not calculated) of the CoD of the ADP motor control strategy during WO. The black dots represent the CoD of the ADP motor control strategy, and the red line represents the failed approximated exponential function of the CoD of the ADP motor control strategy. The blue line represents Avg of CoD (ADP), and the green lines represent Avg of CoD (ADP) + SD of CoD (ADP) and Avg of CoD (ADP)
Table 2 shows VEPFX, VEPEX, VSTFX, VSTEX, CoD angle (FX), and CoD angle (EX) in the first (or second) cycle of the first set during WO, along with SoA and SoO. Table 3 shows the indicators (Avg of CoD (BL), SD of CoD (BL), Time Constant (BL), Initial Value (BL), and Constant Value (BL)),
The Time Constant (BL) and Time Constant (ADP) values of Participants B (Fixed), C, and E are calculated through exponential approximation for both flexion and extension movements. Although the Initial Values (BL) are outside the range of Avg of CoD (BL)
Table 5 shows the indicators (Avg of CoD (ADP), SD of CoD (ADP), Time Constant (ADP), Initial Value (ADP), and Constant Value (ADP)),
4 Discussion
Adaptation to the physio-avatar EB system was demonstrated in six of seven experiments, indicating that the trajectory of the joint angles during flexion and extension movements returned to the BL movement strategy by the second cycle of the first set during WO. Additionally, the results of the SoA and SoO surveys in response to the physio-avatar EB system were presented. Adaptation to the avatar was confirmed by the reduced variability in control variables compared with the non-control variables. Hence, humans may be able to control variables independently, such as the equilibrium point (control variable) and stiffness (non-control variable). Moreover, the WO motor control strategy was analyzed against the BL and ADP strategies using the CoD. The (1) presence or absence of aftereffects due to the physio-avatar EB intervention and (2) its impact on SoA and SoO aftereffects were demonstrated. First, the existence of aftereffects from the WO strategy compared to the BL strategy was examined. The purpose of this study was to verify whether physio-avatar EB intervention could influence motor control strategies, so BL and WO movements had to be equivalent to evaluate the same motor control strategies. Therefore, as an indicator of the occurrence of aftereffects, the time constant of the exponential approximation of the CoD of the AA ratio trajectory during WO must include at least two cycles.
For Participants B (Fixed), C, and E during both flexion and extension movements and Participant B (Free) during extension movements, the time constant of exponential approximation was greater than 2. In these experiments, Constant Value (BL) was within the range of Avg of CoD (BL)
The presence or absence of aftereffects from the WO control strategy compared to the ADP control strategy was examined. The continuation or divergence of the ADP motor control strategy during WO may indicate that the ADP control strategy was maintained as an aftereffect during WO. The divergence of the results from the ADP control strategy during the flexion and extension movements of Participant B (Free), where a Time Constant (ADP) was calculated, and the fact that Initial Value (ADP) exceeded Constant Value (ADP) suggest an aftereffect of returning from a virtual-trajectory-control-like control strategy to a non-virtual-trajectory-control-like control strategy. However, for flexion movements, despite the observed divergence from the ADP control strategy, the maintenance of the BL motor control strategy from the early WO period does not definitively indicate aftereffects. Hence, this is considered an example where aftereffects did not occur. Participant A maintained the ADP control strategy during flexion and extension movements under both hand conditions. However, they returned to the BL motor control strategy from the early WO period. Therefore, rather than maintaining the ADP motor control strategy during WO, they controlled the avatar while implementing the BL motor control strategy, leading to a high CoD of the ADP control strategy during WO. Thus, aftereffects did not occur in these four examples. For Participants C and D, the return to the BL motor control strategy from the early WO period and the divergence from the ADP control strategy indicate the absence of aftereffects.
In summary, aftereffects were observed in the results of Participants B (Fixed), C, and E during flexion and extension movements and Participant B (Free) during extension movements, suggesting the effectiveness of the physio-avatar EB intervention. Among the four experiments showing intervention effects, three had SoA scores exceeding 4, and two had SoO scores above 4, with their averages being 4.5 and 3.5, respectively. The degree of SoA may influence the effectiveness of the physio-avatar EB intervention. Prior researchers on SoA and SoO developed methods of artificially creating conditions with SoO but without SoA, or vice versa, and found that only SoA is associated with improved motor skills. Therefore, enhancing SoA can be effective in rehabilitation systems (Matsumiya, 2021).
The results of the current study are similar to those of previous studies. With an increased sample size, future research should explore the relationship between NMSS model parameters or experimental conditions and SoA and verify whether the intervention effects are related to SoA and not dependent on SoO. In addition, the results were compared with those of previous studies. No error learning was observed for the rehabilitation device with the EMG and a virtual arm in existing studies (Gieser et al., 2017; Yang et al., 2017; MINDROVE, 2024; Suryanarayanan and Reddy, 1997). Furthermore, the research group cited in the introduction, which had reported that error learning did occur, did not use EMG to generate errors in the muscle space (Lo et al., 2010; Liu et al., 2018; Wei et al., 2005; Abdollahi et al., 2013; Porta et al., 2021). This is because, in these studies, errors such as the finger-tip position error were introduced into the task space. The greatest contribution of the present study is that it confirms that error learning does, in fact, occur in the muscle space when avatars that can be manipulated with EMG signals continuously and in real time are used.
Future challenges include issues with the system and analytical methods. In terms of system-related issues, forearm pronation and supination are important for elbow flexion–extension movements when the shoulder joint is not horizontal. However, in this system, while the avatar is limited to 1-DOF movements within a horizontal plane, the actual body assumes an internal rotation position at the shoulder joint, failing to realize complete horizontal-plane movement. SoA requires a match between the prediction of action outcomes and the feedback of actual results (Watanabe and Nobuyuki, 2016), and the inability to achieve a complete horizontal-plane movement in this work may have reduced SoA values. Future developments should consider the relationship between the shoulder joint posture and movement direction during flexion movements to achieve complete horizontal-plane movement. Regarding issues related to the analytical methods, the discussion centers around the AA ratio trajectory and determining the degree of change compared to before the physio-avatar EB intervention based on the CoD. This article focuses on whether the control strategy returns to the pre-intervention state immediately after the intervention. Results that do not indicate a return to the pre-intervention control strategy are not discussed and should, therefore, be further explored. Additionally, as the CoD may be insufficient for evaluating the trajectory shape, other indicators should be introduced for evaluating trajectories. These issues will be addressed in future work, which will also conduct further verification with healthy individuals and evaluate the clinical efficacy of using the developed system with actual patients.
5 Conclusion
In this study, we explored the possibility of changing motor control strategies by applying the physio-avatar EB system to healthy individuals as a case study. This is a preliminary step toward confirming the applicability of the developed system to patients. The intervention, providing an experience of a body different from one’s own, was conducted on seven participants using a time-invariant calculation algorithm to determine the avatar joint angles. The participants’ motor control strategies for identical cyclical movements before the intervention (five sets) and immediately after the intervention (five sets) were compared. Additionally, fixed and free-hand conditions were set during ADP, so 12 experiments were conducted. The data from seven experiments were evaluated after excluding data believed to have been affected by EMG acquisition issues.
The evaluation focused on adaptation to the physio-avatar EB system, the effect of physio-avatar EB intervention, and the impact of SoA and SoO on the intervention effect. Evaluations were conducted separately for extension and flexion movements. To evaluate adaptation to the physio-avatar EB system, the BL and ADP variability indices for the equilibrium point (a control variable of the physio-avatar EB system) and stiffness (a non-control variable) were determined. The degree of increase from BL to ADP was assessed, and findings confirmed such adaptation in all but one experiment. The adaptation indicators of the experiment that failed to confirm adaptation may have been undervalued. In the evaluation of the physio-avatar EB intervention effect, the BL and ADP control strategies for the AA ratio, an indicator of the equilibrium point in human motor control, were determined. The similarity of these control strategies during WO was assessed using the CoD. Exponential approximation of the CoD compared to the control strategies at BL and ADP during WO was performed to determine the time constant, initial value, and convergence value of the exponential approximation. The results then served as indicators for assessing the intervention effect. Changes in motor control strategies due to physio-avatar EB intervention were observed in four experiments, which showed a gradual return to the pre-intervention control strategy. Thus, the physio-avatar EB system affected motor control strategies. Furthermore, the four experiments showing an intervention effect suggest that SoA may influence the presence or absence of such an effect. The employed system specs were considered sufficient when compared with existing studies and required specifications.
Data availability statement
The raw data supporting the conclusions of this article will be made available by the authors, without undue reservation.
Ethics statement
The studies involving humans were approved by the Ethics Committee for Research Involving Human Subjects at the Graduate School of Engineering Science, Osaka University. The studies were conducted in accordance with the local legislation and institutional requirements. The participants provided their written informed consent to participate in this study.
Author contributions
TA: writing–original draft, visualization, software, methodology, investigation, formal analysis, and data curation. KM: writing–review and editing, writing–original draft, visualization, validation, supervision, software, project administration, methodology, investigation, funding acquisition, formal analysis, data curation, and conceptualization. YO: writing–review and editing, software, and investigation. KA: writing–review and editing, validation, and supervision. KT: writing–review and editing, validation, and supervision. HH: writing–review and editing, validation, and supervision. AN: writing–review and editing, validation, supervision, project administration, and conceptualization.
Funding
The author(s) declare that financial support was received for the research, authorship, and/or publication of this article. This work was supported by JSPS KAKENHI JP20K14693, the Future Social Value Co-Creation Project, Osaka University, and the Hattori Hokokai Foundation 23-012.
Conflict of interest
The authors declare that the research was conducted in the absence of any commercial or financial relationships that could be construed as a potential conflict of interest.
Publisher’s note
All claims expressed in this article are solely those of the authors and do not necessarily represent those of their affiliated organizations, or those of the publisher, the editors, and the reviewers. Any product that may be evaluated in this article, or claim that may be made by its manufacturer, is not guaranteed or endorsed by the publisher.
References
Abdollahi, F., Case Lazarro, E. D., Listenberger, M., Kenyon, R. V., Kovic, M., Bogey, R. A., et al. (2013). Error augmentation enhancing arm recovery in individuals with chronic stroke: a randomized crossover design. Neurorehabilitation Neural Repair 28, 120–128. doi:10.1177/1545968313498649
Akoglu, H. (2018). User’s guide to correlation coefficients. Turkish J. Emerg. Med. 18, 91–93. doi:10.1016/j.tjem.2018.08.001
Ando, T., Okamoto, Y., Matsui, K., Atsuumi, K., Taniguchi, K., Hirai, H., et al. (2022). Visual EMG biofeedback system in a virtual reality space for relearning internal models: case studies focusing on equilibrium point velocity. IEEE/SICE International Symposium on System Integration (SII) 2022, 2A1–A12. doi:10.1109/SII55687.2023.10039153
Ando, T., Okamoto, Y., Matsui, K., Atsuumi, K., Taniguchi, K., Hirai, H., et al. (2023). “Visual EMG biofeedback system in a virtual reality space for relearning internal models,” in Case studies focusing on equilibrium point velocity. IEEE, 1–2.
Ando, T., Okamoto, Y., Matsui, K., Atsuumi, K., Taniguchi, K., Hirai, H., et al. (2024). Visual emg biofeedback system in a vr space for relearning internal models — case studies in healthy subjects using a prototype. J. Robotics Soc. Jpn. 42, 283–286. doi:10.7210/jrsj.43.283
Biometrics Ltd (2024). Advanced electronic goniometers for joint rom. Available at: https://www.biometricsltd.com/goniometer.htm.
Bizzi, E., Accornero, N., Chapple, W., and Hogan, N. (1984). Posture control and trajectory formation during arm movement. J. Neurosci. 4, 2738–2744. doi:10.1523/jneurosci.04-11-02738.1984
Dal’Bello, L. R., and Izawa, J. (2021). Task-relevant and task-irrelevant variability causally shape error-based motor learning. Neural Netw. 142, 583–596. doi:10.1016/j.neunet.2021.07.015
Dobkin, B. H., Plummer-D’Amato, P., Elashoff, R., and Lee, J.the SIRROWS Group (2010). International randomized clinical trial, stroke inpatient rehabilitation with reinforcement of walking speed (SIRROWS), improves outcomes. Neurorehabilitation Neural Repair 24, 235–242. doi:10.1177/1545968309357558
Doheny, E. P., Lowery, M. M., FitzPatrick, D. P., and O’Malley, M. J. (2008). Effect of elbow joint angle on force-emg relationships in human elbow flexor and extensor muscles. J. Electromyogr. Kinesiol. 18, 760–770. doi:10.1016/j.jelekin.2007.03.006
Gasparic, F., Jorgovanovic, N., Hofer, C., Russold, M. F., Koppe, M., Stanisic, D., et al. (2023). Nonlinear mapping from emg to prosthesis closing velocity improves force control with emg biofeedback. IEEE Trans. Haptics 16, 379–390. doi:10.1109/toh.2023.3293545
Gieser, S. N., Kanal, V., and Makedon, F. (2017). “Evaluation of a low cost EMG sensor as a modality for use in virtual reality applications,” in Proceedings of virtual, Augmented and mixed reality (VAMR 2017), 97–110.
Gong, S., Matsui, K., Fukui, R., Hirai, H., and Nishikawa, A. (2020). “Modeling and stiffness control of elbow joint movement using functional electrical stimulation,” in Proceedings of 42nd Annual International Conference of the IEEE Engineering in Medicine and Biology Society.
Hagita, N. (2022). Trends in the scientific and technological development of human-machine symbiosis for a post-covid-19 society. Available at: https://www.eaj.or.jp/eajlocal/wp-content/uploads/2022/04/eaj-report-proj-20220208.pdf.
Hirai, H., Miyazaki, F., Naritomi, H., Koba, K., Oku, T., Uno, K., et al. (2015). On the origin of muscle synergies: invariant balance in the co-activation of agonist and antagonist muscle pairs. Front. Bioeng. Biotechnol. 3, 192. doi:10.3389/fbioe.2015.00192
HTC Corporation (2024). Vive pro support. Available at: https://www.vive.com/us/support/vive-pro/.
Kaneko, F. (2016). Own-body kinesthetic illusion in the augmented reality. Jpn. J. Rehabilitation Med. 53, 234–240. doi:10.2490/jjrmc.53.234
Keizer, A., van Elburg, A., Helms, R., and Dijkerman, H. C. (2016). A virtual reality full body illusion improves body image disturbance in anorexia nervosa. PloS one 11, e0163921. doi:10.1371/journal.pone.0163921
Lawrence, J. H., and De Luca, C. (1983). Myoelectric signal versus force relationship in different human muscles. J. Appl. Physiology 54, 1653–1659. doi:10.1152/jappl.1983.54.6.1653
Liu, L. Y., Li, Y., and Lamontagne, A. (2018). The effects of error-augmentation versus error-reduction paradigms in robotic therapy to enhance upper extremity performance and recovery post-stroke: a systematic review. J. neuroengineering rehabilitation 15, 65–25. doi:10.1186/s12984-018-0408-5
Lo, A. C., Guarino, P. D., Richards, L. G., Haselkorn, J. K., Wittenberg, G. F., Federman, D. G., et al. (2010). Robot-assisted therapy for long-term upper-limb impairment after stroke. N. Engl. J. Med. 362, 1772–1783. doi:10.1056/nejmoa0911341
Masumoto, K., Takasugi, S., Hotta, N., Fujishima, K., and Iwamoto, Y. (2004). Electromyographic analysis of walking in water in healthy humans. J. physiological Anthropol. Appl. Hum. Sci. 23, 119–127. doi:10.2114/jpa.23.119
Matsui, K., Hishii, Y., Maegaki, K., Yamashita, Y., Uemura, M., Hirai, H., et al. (2014). Equilibrium-point control of human elbow-joint movement under isometric environment by using multichannel functional electrical stimulation. Front. Neurosci. 8, 164. doi:10.3389/fnins.2014.00164
Matsui, K., Maegaki, K., Yamashita, Y., Uemura, M., Hirai, H., and Miyazaki, F. (2015). Analysis of equilibrium-point control model using two-channel functional electrical stimulation to extend elbow joint movement to an unconstrained environment on the horizontal plane. Trans. Jpn. Soc. Med. Biol. Eng. 53, 14–20. doi:10.11239/jsmbe.53.14
Matsui, K., Suzuki, Y., Atsuumi, K., Nagai, M., Ohno, S., Hirai, H., et al. (2022). Earable (omega): a novel clenching interface using ear canal sensing for human metacarpophalangeal joint control by functional electrical stimulation. Sensors 22, 7412. doi:10.3390/s22197412
Matsumiya, K. (2021). Awareness of voluntary action, rather than body ownership, improves motor control. Sci. Rep. 11, 418. doi:10.1038/s41598-020-79910-x
Medivr (2024). Medivr kagura. Available at: https://www.info.pmda.go.jp/downfiles/md/PDF/784965/784965_27B2X00324201901_1_01_04.pdf.
Microsoft (2024). Azure kinect dk documentation. Available at: https://learn.microsoft.com/en-us/previous-versions/azure/kinect-dk/.
MINDROVE (2024). Emg-based hand gesture recognition in vr. Available at: https://mindrove.com/2023/01/10/emg_based_hand_gesture_recognition-_in_vr/.
Nagai, M., Matsui, K., Atsuumi, K., Taniguchi, K., Hirai, H., and Nishikawa, A. (2019). “Modeling of metacarpophalangeal joint movement using functional electrical stimulation by controlling the equilibrium-point,” in Proceedings of of the 41st annual international Conference of the IEEE Engineering in Medicine and biology society.
NATIONAL INSTRUMENTS CORP (2024a). Labview. Available at: https://www.ni.com/ja-jp/shop/product/labview.html.
NATIONAL INSTRUMENTS CORP (2024b). Pxie-6363 specifications. Available at: https://www.ni.com/docs/ja-JP/bundle/pxie-6363-specs/page/specs.html.
Okamoto, Y., Matsui, K., Ando, T., Atsuumi, K., Taniguchi, K., Hirai, H., et al. (2024). Pilot study of the relation between various dynamics of avatar experience and perceptual characteristics. PeerJ Comput. Sci. 10, e2042. doi:10.7717/peerj-cs.2042
OriginLab (2023). Help online - origin help - theory of nonlinear curve fitting. Available at: https://www.originlab.com/doc/ja/origin-help/nlfit-theory#Parameter_Standard_Errors.
Osu, R., Franklin, D. W., Kato, H., Gomi, H., Domen, K., Yoshioka, T., et al. (2002). Short-and long-term changes in joint co-contraction associated with motor learning as revealed from surface emg. J. neurophysiology 88, 991–1004. doi:10.1152/jn.2002.88.2.991
Porta, F., Celian, C., and Patton, J. L. (2021). “Upper extremity functional rehabilitation for stroke survivors using error-augmented visual feedback: interim results,” in 43rd Annual International Conference of the IEEE Engineering in Medicine and Biology Society (EMBC), 1318–1324. doi:10.1109/EMBC46164.2021.9630799
Preston, C., and Ehrsson, H. H. (2016). Illusory obesity triggers body dissatisfaction responses in the insula and anterior cingulate cortex. Cereb. Cortex 26, 4450–4460. doi:10.1093/cercor/bhw313
Ratan, R., and Sah, Y. J. (2015). Leveling up on stereotype threat: the role of avatar customization and avatar embodiment. Comput. Hum. Behav. 50, 367–374. doi:10.1016/j.chb.2015.04.010
Schultz, W., Apicella, P., and Ljungberg, T. (1993). Responses of monkey dopamine neurons to reward and conditioned stimuli during successive steps of learning a delayed response task. J. Neurosci. 13, 900–913. doi:10.1523/jneurosci.13-03-00900.1993
Schultz, W., Dayan, P., and Montague, P. R. (1997). A neural substrate of prediction and reward. Science 275, 1593–1599. doi:10.1126/science.275.5306.1593
Suryanarayanan, S., and Reddy, N. P. (1997). Emg-based interface for position tracking and control in vr environments and teleoperation. Presence Teleoperators and Virtual Environ. 6, 282–291. doi:10.1162/pres.1997.6.3.282
Suzuki, Y., Matsui, K., Atsuumi, K., Taniguchi, K., Hirai, H., and Nishikawa, A. (2024). Feasibility of human wrist-joint neuromuscular system identification method using functional electrical stimulation in clinical examinations. Adv. Biomed. Eng. 13, 205–213. doi:10.14326/abe.13.205
Tanaka, S. C., Doya, K., Okada, G., Ueda, K., Okamoto, Y., and Yamawaki, S. (2016). Prediction of immediate and future rewards differentially recruits cortico-basal ganglia loops. Tokyo: Springer Japan, 593–616.
Tieri, G., Tidoni, E., Pavone, E. F., and Aglioti, S. M. (2015). Mere observation of body discontinuity affects perceived ownership and vicarious agency over a virtual hand. Exp. Brain Res. 233, 1247–1259. doi:10.1007/s00221-015-4202-3
Tigrini, A., Ranaldi, S., Verdini, F., Mobarak, R., Scattolini, M., Conforto, S., et al. (2024). Intelligent human–computer interaction: combined wrist and forearm myoelectric signals for handwriting recognition. Bioengineering 11, 458. doi:10.3390/bioengineering11050458
Tigrini, A., Verdini, F., Scattolini, M., Barbarossa, F., Burattini, L., Morettini, M., et al. (2023). Handwritten digits recognition from semg: electrodes location and feature selection. IEEE Access 11, 58006–58015. doi:10.1109/access.2023.3279735
TRIGNO CENTRO (2024). Trigno lab. Available at: http://www.delsys.com/products/wireless-emg/trigno-lab/.
Unity Technologies (2024). Unity web site. Available at: https://unity.com/en.
Waltemate, T., Gall, D., Roth, D., Botsch, M., and Latoschik, M. E. (2018). The impact of avatar personalization and immersion on virtual body ownership, presence, and emotional response. IEEE Trans. Vis. Comput. Graph. 24, 1643–1652. doi:10.1109/tvcg.2018.2794629
Watanabe, S., and Nobuyuki, K. (2016). The 33rd Annual Meeting of the Japanese Cognitive Science Society, 31–40.
Wei, Y., Bajaj, P., Scheidt, R., and Patton, J. (2005). “Visual error augmentation for enhancing motor learning and rehabilitative relearning,” in Proceedings of 9th international Conference on rehabilitation robotics, 2005, 505–510. ICORR 2005.
Keywords: rehabilitation, virtual reality, error learning, internal model, electromyogram, biofeedback
Citation: Ando T, Matsui K, Okamoto Y, Atsuumi K, Taniguchi K, Hirai H and Nishikawa A (2024) Physio-avatar EB: aftereffects in error learning with EMG manipulation of first-person avatar experience. Front. Bioeng. Biotechnol. 12:1421765. doi: 10.3389/fbioe.2024.1421765
Received: 22 April 2024; Accepted: 17 September 2024;
Published: 09 October 2024.
Edited by:
Steven Truijen, University of Antwerp, BelgiumReviewed by:
Tan-Nhu Nguyen, Vietnam National University, VietnamAndrea Tigrini, Marche Polytechnic University, Italy
Copyright © 2024 Ando, Matsui, Okamoto, Atsuumi, Taniguchi, Hirai and Nishikawa. This is an open-access article distributed under the terms of the Creative Commons Attribution License (CC BY). The use, distribution or reproduction in other forums is permitted, provided the original author(s) and the copyright owner(s) are credited and that the original publication in this journal is cited, in accordance with accepted academic practice. No use, distribution or reproduction is permitted which does not comply with these terms.
*Correspondence: Kazuhiro Matsui, bWF0c3VpLmthenVoaXJvLmVzQG9zYWthLXUuYWMuanA=
†Present addresses: Kazuhiro Matsui, Graduate School of Information Science and Arts, Osaka Electro-Communication University, Shijonawate, Japan, Graduate School of Engineering Science, Osaka University, Toyonaka, Japan