- 1Department of Bioengineering, Imperial College London, London, United Kingdom
- 2Centre for Blast Injury Studies, Imperial College London, London, United Kingdom
- 3Skeletal Biology Group, Comparative Biomedical Sciences, Royal Veterinary College, London, United Kingdom
Osteoarthritis (OA) is a common chronic disease largely driven by mechanical factors, causing significant health and economic burdens worldwide. Early detection is challenging, making animal models a key tool for studying its onset and mechanically-relevant pathogenesis. This review evaluate current use of preclinical in vivo models and progressive measurement techniques for analysing biomechanical factors in the specific context of the clinical OA phenotypes. It categorizes preclinical in vivo models into naturally occurring, genetically modified, chemically-induced, surgically-induced, and non-invasive types, linking each to clinical phenotypes like chronic pain, inflammation, and mechanical overload. Specifically, we discriminate between mechanical and biological factors, give a new explanation of the mechanical overload OA phenotype and propose that it should be further subcategorized into two subtypes, post-traumatic and chronic overloading OA. This review then summarises the representative models and tools in biomechanical studies of OA. We highlight and identify how to develop a mechanical model without inflammatory sequelae and how to induce OA without significant experimental trauma and so enable the detection of changes indicative of early-stage OA in the absence of such sequelae. We propose that the most popular post-traumatic OA biomechanical models are not representative of all types of mechanical overloading OA and, in particular, identify a deficiency of current rodent models to represent the chronic overloading OA phenotype without requiring intraarticular surgery. We therefore pinpoint well standardized and reproducible chronic overloading models that are being developed to enable the study of early OA changes in non-trauma related, slowly-progressive OA. In particular, non-invasive models (repetitive small compression loading model and exercise model) and an extra-articular surgical model (osteotomy) are attractive ways to present the chronic natural course of primary OA. Use of these models and quantitative mechanical behaviour tools such as gait analysis and non-invasive imaging techniques show great promise in understanding the mechanical aspects of the onset and progression of OA in the context of chronic knee joint overloading. Further development of these models and the advanced characterisation tools will enable better replication of the human chronic overloading OA phenotype and thus facilitate mechanically-driven clinical questions to be answered.
1 Introduction
Osteoarthritis (OA) is a highly prevalent chronic disease that affects the joints and contributes significantly to a loss of physical function (Kloppenburg and Berenbaum, 2020). It is characterised by pain, sometimes linked to cartilage disintegration, bone remodelling and inflammation of the synovium. In the United Kingdom, the incidence of any type of OA in 2017 was 10.7%, with a 1-year consistent frequency of 6.8/103 adults (≥20 years) and 40.5/103 adults (≥45 years) (Swain et al., 2020; Allen et al., 2022). Pain associated disability as a result of OA represents a significant burden (22% of total ill health burden) in societal and personal costs (Versus Arthritis, 2019; Wolf et al., 2019). Internationally, hip and knee OA is the 11th highest cause of disability (GBD, 2017 Disease and Injury Incidence and Prevalence Collaborators, 2018). Knee OA (KOA) is the most prevalent type of OA and is increasing in incidence as obesity and life span increase (Wallace et al., 2017; Hunter and Bierma-Zeinstra, 2019). The latest musculoskeletal calculator (published in 2019) estimated that in 2012 18.2% of the English population over 45 years old had clinically diagnosed KOA, 1/3 of which was severe (Versus Arthritis, 2019).
KOA is historically categorised into two main types, primary OA- also known as idiopathic OA- which is characterised by natural cartilage degeneration during ageing as a result of unidentified reasons, and secondary OA which occurs as a result of known medical conditions or risk factors (Altman et al., 1986). However, with extensive research over the past 2 decades dedicated to understanding risk factors and aetiologies, primary OA has been further divided into genetic, aging and hormonal (estrogen-deficiency) subsets (Herrero-Beaumont et al., 2009). Secondary OA is classified into subsets like metabolic disorders, anatomical abnormalities, traumatic injury and inflammation (Arden and Nevitt, 2006).
Beyond these classic subsets centred on aetiological risk, recent studies propose use of clinical phenotype and endotype concepts to categorize this heterogeneous disease (Deveza and Loeser, 2018; Deveza et al., 2019; Mobasheri et al., 2019); a clinical phenotype refers to observable characteristics of an individual resulting from the interplay between genotype and environment (Mobasheri et al., 2019). Dell’Isola et al classified knee OA into six clinical phenotypes based on a qualitative meta-analysis of existing data from 24 studies: 1) long-lasting pain; 2) upregulation of inflammatory markers; 3) metabolic disorder (estrogen imbalance, dyslipidemia, diabetes, and obesity); 4) changes in bone and cartilage homeostasis; 5) altered joint mechanics (medial meniscus overload or varus malalignment) (Figure 1B); and 6) minor joint disease (slight clinical symptoms with chronic progression) (DellIsola et al., 2016). The complexity is confounded by these phenotypes not necessarily being discrete, with multiple phenotypes potentially co-existing (Bierma-Zeinstra and van Middelkoop, 2017). As a result, reaching a consensus on diagnosing each specific subgroup is challenging due to phenotype overlaps, meaning no specific biomarkers or tests can be uniquely applied. OA endotypes (or mechanistic phenotypes) are defined by distinct pathobiological molecular mechanisms and signaling pathways, making them identifiable by specific biomarkers (Anderson, 2008). This distinction is valuable for targeted anti-cytokine therapy. Inflammation, metabolism, cell senescence, and bone and cartilage endotypes are considered constituent molecular endotypes of each phenotype (Mobasheri et al., 2019).
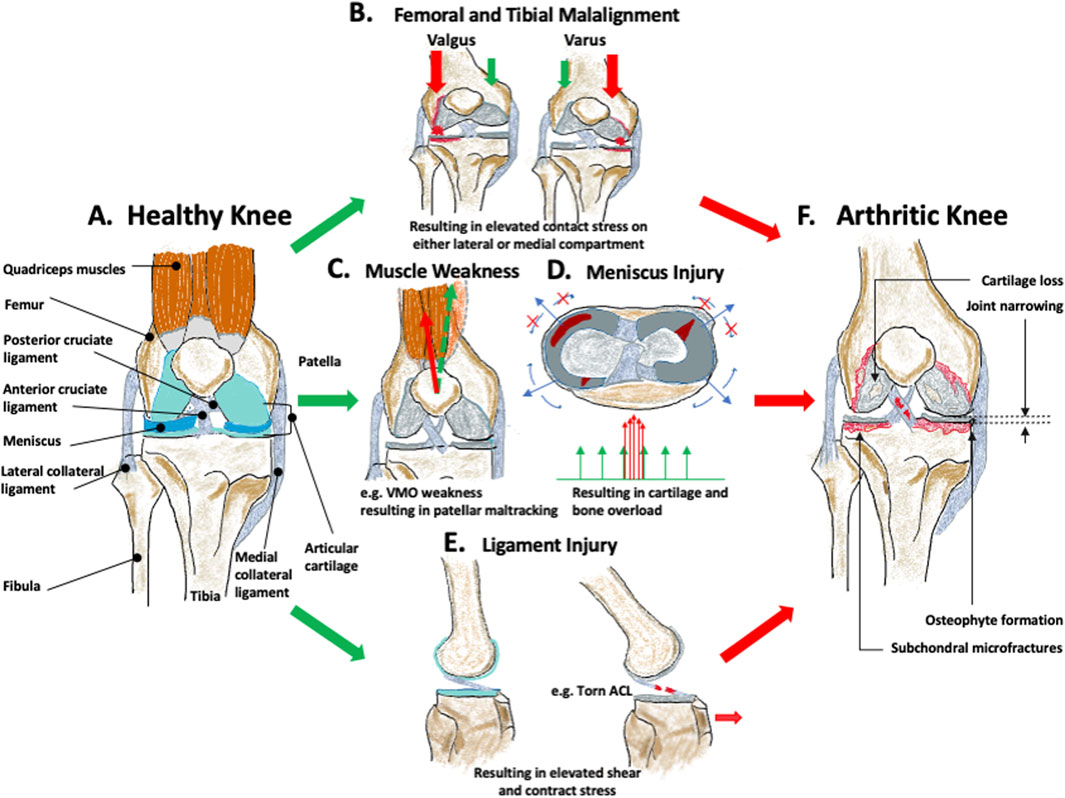
Figure 1. Biomechanical factors leading to osteoarthritis (OA). (A) represents the healthy knee. (B) femoral and tibial malalignment with resulting varus or valgus angulation and medial or lateral joint overload, (C) quadriceps muscular weakness leading to muscle imbalance and local joint overload, (D) meniscus injury leading to increased contact stress, (E) ligament injury leading to changes in the overall knee mechanics. All of these will eventually contribute to increasing the mechanical stress on the healthy knee and induce joint structural changes, including cartilage loss, joint narrowing, osteophyte formation, and subchondral microfractures resulting in, (F) arthritic knee.
KOA (Figure 1A) is insidious and is defined as the degeneration of the articular cartilage in the weight-bearing region, with structural changes of the surrounding bone, and joint inflammation, irrespective of the presence of clinical symptoms (Kraus et al., 2015; Lespasio et al., 2017) (Figure 1F). This joint degeneration is affected by a combination of mechanical and biological effects (Sandell and Aigner, 2001). Epidemiologically, OA risk factors can be categorised into individual-centred (or systemic) factors such as age, sex, race, genetic background, metabolic disorder, endocrine, diet, bone density, and joint-related factors such as different shapes of the articulating surfaces, muscle weakness, occupation/sports, and injury and joint overloading (Vina and Kwoh, 2018). Biomechanical factors are the predominant theme associated with these joint-related factors, and this is the focus of much recent KOA research (Glyn-Jones et al., 2015; van Tunen et al., 2016). There is also increasing evidence that OA onset is mechanically driven and the onset is strongly correlated with altered mechanical properties of subchondral bone (Day et al., 2001; Hayami et al., 2006).
Biomechanical factors play an important role in understanding the pathogenic mechanism of all forms of KOA and in helping to address clinical questions. For example, it is reported that frontal plane knee malalignment has the strongest relation with KOA development (Palazzo et al., 2016). The natural knee alignment is around 1° varus (Cooke et al., 2007) and the ideal realignment angle ranges from 1° to 13° valgus (Dugdale et al., 1992; Cooke et al., 2007; Tsukada and Wakui, 2017; Sethi et al., 2020). However, these data are all derived from retrospective studies, which are population-based and laboratory experiments to confirm these findings are lacking. Moreover, despite OA (Figure 1) being a progressive disease, symptomatic OA is usually only diagnosed in patients with clinical symptoms and imaging evidence at an advanced stage (Hayami et al., 2006), which is likely irreversible. Therefore, it is imperative to devote research efforts to detect biomechanical mechanisms at the initial and early stages of OA. This is key not only for studying disease aetiology, but would also represent a big step towards developing preventative treatment and in diagnosing pre-radiographic OA.
Experiments to investigate these biomechanical factors are difficult in human studies as the mechanical intervention and direct outcomes are hard to perform and observe independently (Wendler and Wehling, 2010), and the available human tissue diagnosed as OA is not in the early stage (Hayami et al., 2006). Ex vivo joint models cannot truly mimic musculoskeletal mechanics and the arthritic environment. Thus, in vivo experimental animal models are important in studying the onset and early stages of this disease, particularly when investigating biomechanical aspects (Kuyinu et al., 2016). The two main goals of using OA in vivo models are: 1) to understand the pathophysiology of the disease, especially in the early stages, and 2) to test the safety and efficacy of different therapeutics developed before entering into clinical trials (Kuyinu et al., 2016). Recent advances in measurement techniques for in vivo animal studies have enabled and improved the analysis of OA and reduced animal usage (Teeple et al., 2013). In the light of recent work on in vivo animal models and methodological advances on biomechanical aspects of KOA, the purpose herein is to conduct a narrative review on the use of preclinical in vivo models, and advanced measurement techniques focusing specifically on biomechanical aspects in the context of the identified human clinical sub-division of OA.
2 Biomechanical factors in OA
Biomechanical factors are defined herein as joint-related, OA-linked, features based on epidemiological statistics. These are subdivided into either those anatomical relationships influencing the joint, or those that are the consequence of functional joint usage (Glyn-Jones et al., 2015; van Tunen et al., 2016). The following section presents current evidence on biomechanical-related risk factors associated with OA.
Anatomical factors include those derived from individual joint morphology and limb alignment (Glyn-Jones et al., 2015), where these can be variations in the shape of the tibia, femur, or patella. The anatomy is the product of genetics and adaptation to use, and the reasons for the adaptive changes are not fully known, but are likely to align with Wolff’s law, which describes how bone tissue can be remodelled in response to prevailing mechanical environment (Chen et al., 2010). These morphological variations can, therefore, be created by habitual joint loading experiences, but will also then induce corresponding shifts in joint loading and so may initiate or accelerate OA (Neogi et al., 2013). The morphological variation can predict the onset of OA, a year earlier than visible radiographic changes (Neogi et al., 2013). Extensive variation, recognized as knee malalignment (i.e., varus or valgus alignment) is also a strong risk factor in OA onset and progression (Tanamas et al., 2009). It is defined as a shift from the collinear mechanical alignment of hip, knee and ankle to either a medialised (varus) or lateralised (valgus) loading distribution (Sharma et al., 2001). The alignment changes can be a result of localised morphological deviation. In the varus knee, the mechanical axis (from mid femoral head to mid ankle) will pass medial to the centre of the knee. This induces an adduction moment that increases the force on the medial tibiofemoral compartment; the opposite happens in the valgus knee (Tetsworth and Paley, 1994). This loading imbalance contributes to OA progression (Sharma et al., 2001), with a 1° varus deviation out of the natural physiological range of alignment, disproportionally increasing the medial load by 5% (Schipplein and Andriacchi, 1991; Halder et al., 2012), As such, varus and valgus malalignment (Figure 1B) is a known independent risk factor in the progression of medial and lateral OA (Tanamas et al., 2009). Of these, varus alignment is more common in the knee OA population and is significantly linked with OA development (Brouwer et al., 2007).
Functional factors that are a consequence of challenging joint usage include previous knee injury, poor muscle function, reduced proprioceptive acuity, activities (occupational activity and sports activity), and joint laxity (Bennell et al., 2012; Richmond et al., 2013; Glyn-Jones et al., 2015; van Tunen et al., 2016). Knee injury mainly includes knee ligament ruptures, sprains or meniscal tears (Thomas et al., 2017), which carry a high risk of post-traumatic OA (PTOA) onset compared to uninjured individuals (Thomas et al., 2017). There is some evidence of the high OA incidence in athletes being mainly due to joint injury (Bennell et al., 2012). Meniscal and anterior cruciate ligament (ACL) injuries each account for one-quarter of all knee injuries (Thomas et al., 2017). Ligament injuries can cause changes in joint mechanics, including translation and shear, leading to overload (Figure 1E) (Kernozek et al., 2013). Simply, meniscal tears decrease the contact area between cartilaginous surfaces thus increasing contact stresses during load transfer (Figure 1D) (Masouros et al., 2008). Muscle weakness (Figure 1C), including athrogenic muscle inhibition, was previously thought to be a secondary factor that results from progression of OA damage, but recent population-based evidence shows that muscle dysfunction can be a primary risk of OA initiation, preceding knee pain and muscle atrophy (Slemenda et al., 1997). Quadriceps weakness specifically is a better predictor of joint pain and joint space narrowing than radiographic evidence (Palmieri-Smith et al., 2010).
Impaired knee proprioception refers to a deteriorated accuracy in position and motion sense, caused by impaired articular mechanoreceptors (Knoop et al., 2011). Reduced proprioceptive accuracy has been found in OA patients and this causes a decrease in knee stabilization and joint movement coordination, both of which are directly related to mechanics, and has a significant association with knee pain (Knoop et al., 2011; van Tunen et al., 2018). These factors are linked, as the loss of muscle strength can be attributed to afferent sensory dysfunction (i.e., impaired proprioception) and the subsequent reduction in efferent neuron stimulation (Slemenda et al., 1997). Although there is currently no robust evidence that dysfunctional proprioception causes OA onset, it has been proposed as a putative OA risk factor (Roos et al., 2011). Activities (e.g., physical, occupational and sporting) are controversial factors for knee OA, as cartilage homeostasis is known to rely upon moderate loading but could change to catabolic metabolism in high intensity and long duration, abnormal mechanics (Griffin and Guilak, 2005). Heavy occupational lifting, frequent deep knee flexion (squatting, kneeling) and sports activity (at elite level) with significant impact, twisting, turning and running have a demonstrated higher OA risk (Richmond et al., 2013). In contrast, there is no evidence that physical activity (moderate sports) and recreational sports activity, without suffering injury, increase the risk of OA (Bennell et al., 2012; Richmond et al., 2013). Some large studies have found that ice hockey and soccer players, wrestlers and weightlifters have a much higher incidence of OA than endurance sport athletes (Kujala et al., 1994; Tveit et al., 2012). This aligns with the notion that there is a biomechanical tolerance level in weight-bearing joints; this level is not currently known (Thelin et al., 2006). However, there could simply be a higher incidence of injury in those sports in which higher biomechanical loads are engendered.
Joint laxity–loss of stability with deficiencies in the primary soft-tissue stabilisers such as the cruciate ligaments, secondary stabilisers such as the capsule, or in alignment–is another biomechanical-related OA risk factor (van Tunen et al., 2016). Evidence has shown that patients with medial knee OA always have laxity, usually in the varus-valgus direction (van Tunen et al., 2018). All of these factors are related to biomechanics (loading, motion) and in general, alterations in these factors will change knee joint loading (van Tunen et al., 2016). Acute or long-term overuse changes in loading will subsequently induce the structural, biological and mechanical level changes and present as clinical symptoms.
Although biomechanical risks can be independent, direct-causative factors in some cases of knee OA, all OA phenotypes have a mechanical component, and all risk factors can induce shifts in mechanical stress in chondrocyte pericellular matrix (Brandt et al., 2009; Zhao et al., 2020). For example, obesity is an important OA risk factor; not only because the systemic inflammation arising from adipokines can promote local joint inflammation (Berenbaum et al., 2013; Robinson et al., 2016), but because obesity can also interact with confounding biomechanical factors, evidenced by less frequent cyclic physiologic joint loading (lowering risk) and altered gait and increased peak knee force (which both increase risk) (Aaboe et al., 2011; Issa and Griffin, 2012). At the molecular and cellular level, cartilage degradation is generally affected by biological and mechanical stimuli (Sandell and Aigner, 2001) and many risk factors induce OA by combined inflammation and stress-induced mechanotransduction signaling pathways in chondrocytes (Guilak, 2011). Recent advances in our grasp of chondrocyte mechano-signaling make the mechanism by which biomechanical factors may contribute to OA clearer. It is now evident that the pericellular matrix of the cartilage chondrocyte transmits the physiological mechanical stimulus (including abnormal stress, compression, fluid pressure) to cell surface mechanoreceptors (ion channels, integrins) which convert these outside physical signals to intracellular signals, to regulate a series of downstream pathways (Guilak, 2011; Gilbert and Blain, 2018; Zhao et al., 2020). This suggests that this biomechanical stress may be critical in the initiation and progression of OA, independently of the influence of inflammation. Therefore, although there is tantalising information, there is still no consensus in the understanding of the biomechanical factors underlying OA initiation and progression (Guilak, 2011; Saxby and Lloyd, 2017; Hunt et al., 2020). Some experts propose that any efforts to subdivide OA should largely consider the abnormal mechanical stress loading on the joint, and advise subclassifying OA based on mechanical abnormalities (Radin et al., 1991; Deveza et al., 2019). This highlights the need to accurately define the various mechanical factors, and the abnormal mechanical stress, contributing to the different phenotypes of OA. To identify these factors and to address this fundamental gap of mechanical stress mechanism, preclinical models should be developed that are clinically representative of biomechanically induced OA (McCoy, 2015; Kuyinu et al., 2016; Serra and Soler, 2019).
3 Classifying preclinical in vivo models of OA
As OA is a multifactorial disease driven by genetic, biological, and biomechanical factors (Glyn-Jones et al., 2015), there are several different in vivo models that provide a means to study its various distinct features. Each model replicates a unique OA aetiology and pathogenesis that reflects the specific mechanism of interest as well as the target uses for drug treatment (Cope et al., 2019). Generally, small mammals (nearly always rodents), including mice, rats, rabbits and guinea pigs, are considered for primary basic research (Serra and Soler, 2019), such as the study of OA pathology/pathophysiology, and assessing the effectiveness of different therapeutics (Kuyinu et al., 2016). Larger animals (dog, horse, goat, cattle) develop the disease more slowly, are more expensive, less reproducible and harder to handle (Serra and Soler, 2019), yet can be more relevant to disease heterogeneity and the anatomy, dimensions and biomechanics of human joints (Lampropoulou-Adamidou et al., 2014; Meeson et al., 2019b). Therefore, they are more widely used in translational preclinical studies, where the clinical progression of the disease and treatment are being considered.
These in vivo OA models are characteristically classified as: spontaneous, which include naturally occurring for uncertain/unknown reasons and genetically modified models, and induced models which can be provoked by chemical or surgical intervention (Table 1). Recently, some non-invasive induced models have been developed as alternatives to surgical models (Christiansen et al., 2015; Poulet, 2016). These OA models are typically categorised in terms of classical primary and secondary OA types. Spontaneous models characterise to a certain extent the natural progression of human primary OA with or without genetic modification. Accordingly, induced models are developed as a result of replicating specific known risk factors of secondary OA and these models are usually surgically induced, and sometimes less appropriately chemically induced (Vincent et al., 2012; McCoy, 2015). The induced methods can stimulate acute inflammation and/or alter the joint mechanics (Serra and Soler, 2019).
The Dunkin Hartley guinea pig is the most used spontaneous model to develop naturally occurring, age-related OA. This has a high incidence and occurs at a reasonably young age compared to humans (Jimenez et al., 1997). It has similar histopathology to the human disease and the histological changes can normally be observed at 3 months of age (Kraus et al., 2010). The Str/ort mouse, derived from selective breeding with susceptibility to OA, is a well-recognized spontaneous OA model where >85% of all male mice have very mild OA lesions in the medial tibial plateau from 10 weeks of age (Mason et al., 2001; Staines et al., 2017a). Similarly, OA can arise spontaneously in rabbits, where natural disease can be found in >50% by the sixth year of age (Arzi et al., 2012). Spontaneous dog OA has also been proposed as a model, as OA can have high prevalence (>20%) over 1-year of age, and dogs have more human-like anatomy and OA heterogeneity than rodents (Meeson et al., 2019a). Genetically modified models, mainly in mice, are designed to knock down, knockout, knock-in or mutate specific genes to breed strains with modified OA susceptibility (Little and Hunter, 2013).
The induced methods aim to generate joint destabilization and increase joint contact force, and/or create an intra-articular inflammation, and so consequently alter cell metabolism and induce an OA lesion (Teeple et al., 2013). Chemically induced models mostly include intra-articular injection of an agent to trigger acute local inflammation, extracellular matrix degradation and chondrocyte death (Bendele, 2001). The chemical agents generally used in induced animal models of ‘OA-like’ joint pain are papain (Sukur et al., 2016), quinolone (Rodrigues-Neto et al., 2016), collagenase (Lee et al., 2020), carrageenan (Korotkyi et al., 2019), Freund’s adjuvant (Robin, 2017) and, most commonly, sodium mono-iodoacetate (MIA) (Adeyemi and Olayaki, 2017). Surgically induced models mainly use intra-articular invasive surgery to generate joint instability or create chondral defects to induce OA. PTOA is the most widely studied secondary OA model, commonly used to analyse mechanical aspects of OA. Currently, the most often used surgical models are destabilization of the medial meniscus (Arzi et al., 2012)), anterior cruciate ligament transection (ACLT; (Lampropoulou-Adamidou et al., 2014), collateral ligament transection (Bendele, 2001) other meniscal injury (meniscectomy (Ali et al., 2018) or medial meniscal tear/transection (Brederson et al., 2018; Pucha et al., 2020; Temp et al., 2020)), and chondral/osteochondral defects (Mastbergen et al., 2006; Flanigan et al., 2010; McNulty et al., 2012; Figueroa et al., 2014; Serra et al., 2014), or ovariectomy (oestrogen deficiency causing subchondral osteoblast changes) (Xu et al., 2019). Additionally, a novel non-articular invasive method was raised again recently, using tibial osteotomy (TO) to induce a varus tibial malalignment and joint mechanical overload (Britzman et al., 2018); this is more representative of the human primary chronic overloading OA condition, or a means of replicating the susceptibility to OA based on anatomical deviation, without any intra-articular damage or destabilization of internal joint mechanics (Britzman et al., 2018).
Although surgical models better mimic the pathogenic mechanisms than chemical models and have a shorter progression period than spontaneous models, they involve aseptic procedures, and the results are highly dependent on surgical skill. This means that ensuring repeatability and minimising variability of these invasive models is difficult. To address these issues, some studies create PTOA by using non-invasive methods through applying an external mechanical load to the relevant joint without disrupting the skin or the joint capsule (Christiansen et al., 2015) through either single-impact injury or repetitive loading (Poulet, 2016). There are five main types of non-surgically induced model: 1) intra-articular tibial plateau fracture (Lewis et al., 2011; Schenker et al., 2014; Kimmerling et al., 2015); 2) ACL rupture through tibial compression overload (Lockwood et al., 2014; Khorasani et al., 2015); 3) knee immobilization in an extended position (Videman, 1982) 4) cyclic articular cartilage tibial compression (Poulet et al., 2011; Melville et al., 2015); and 5) moderate running exercise. Compared to severe surgical PTOA models, these models are more likely to represent real biomechanical conditions in OA development.
The OA model classifications previously mentioned (spontaneous and induced) are rooted in the conventional view (primary and secondary OA) that distinguishes various aetiologies (intervention methods) (Table 1) (Esdaille et al., 2022). Notably, most pharmacotherapies derived from these animal models are symptomatic, primarily addressing the clinical phase of the disease rather than the pre-arthritis phase. A recent review article analyzed gene expression data across different OA models, emphasizing how discrepancies between models can lead to divergent conclusions regarding targeted intervention therapies (Soul et al., 2020). Therefore, it is crucial to clarify the commonalities among these models and understand their relationship to each etiopathogenesis and the six distinct OA clinical classifications currently adopted. A recent systematic review defined these clinical phenotypes as those related to: 1. chronic pain, 2. inflammation, 3. metabolic syndrome, 4. bone and cartilage homeostasis, 5. mechanical overload, and 6. minor joint disease phenotypes (DellIsola et al., 2016). This section summarises how these animal models relate to the clinical classifications.
Types 1 and 2. Responses to chronic pain and inflammation are usually conducted using chemically induced models, which induce cartilage degeneration based on triggering the inflammatory mechanism rapidly (Serra and Soler, 2019). The MIA method induces widespread cell death across many joint tissues leading to intense pain and is typically used to study ‘joint’ pain (Pitcher et al., 2016). These models should not be used to study the pathogenic mechanism of OA in humans (Vincent et al., 2012).
Type 3. Metabolic OA phenotype is usually characterized by surgical (ovariectomy) or spontaneous OA models. These models are appropriate to study diabetes, obesity and other metabolic disturbances (estrogen imbalance) causing OA (Xu et al., 2019).
Type 4. Bone and cartilage homeostasis phenotype presents an OA subgroup with significant changes in cartilage and bone metabolism (DellIsola et al., 2016), categorised to atrophic (few osteophytes with severe joint space narrowing) and hypertrophic (large osteophytes with little joint space narrowing) subsets (Roemer et al., 2012). The ACLT surgical model is usually used to study this phenotype, but the cyclic articular cartilage tibial compression models are also used. Bone osteophytes can be rapidly induced in experimental canine OA as early as a few days after ACL surgical induction (Gilbertson, 1975), and the subchondral and trabecular bone remodeling can be observed at around 2 months (Lavigne et al., 2005).
Type 5. The mechanical overloading phenotype which accounts for up to 22% of incidence (DellIsola et al., 2016), is usually modelled by surgical disruption of joint biomechanics, such as meniscectomy or ligament transection, causing joint instability. These models can simulate a human injury event: so-called PTOA models (Little and Hunter, 2013). However, as shown in a population study, PTOA comprises only approximately 12% of symptomatic OA (Brown et al., 2006). Therefore, it could be contended that there is an overuse of the PTOA models in representing the chronic overloading OA phenotype.
Type 6. Ageing or minor joint disease phenotypes are best studied by naturally occurring models (Lampropoulou-Adamidou et al., 2014).
The mechanical overloading phenotype itself has been shown to arise from 1) long-term overuse or cyclic small loads or 2) a short-term high load (impact) (Gardiner et al., 2016) and these two are suggested to have distinct pathophysiology progression (Little and Hunter, 2013). For example, a comparative study developed in guinea pigs with or without ACLT surgical intervention showed that there are several differences in expression of OA biomarkers between these two mechanical overload phenotypes (Wei et al., 2010; Rice et al., 2020). A later study characterised the subgroups of the mechanical overload phenotype into two: one having severe medial OA and varus malalignment with a high prevalence of injury history (55%), and the other having evidence of lateral OA and valgus alignment with lower body mass index (BMI) (DellIsola et al., 2016). Therefore, current PTOA models only represent the short impact (injury-history) subgroup, and there should be another type of biomechanically induced OA model which occurs in a relatively idiopathic, non-traumatic and slow-progressive condition to present the chronic overloading OA that is considered responsible for primary OA (DellIsola et al., 2016). The studies more representative of this form of chronic mechanical overloading OA are the non-invasive cyclic overloading model (Ko et al., 2013) and the extra-articular TO surgical model (Britzman et al., 2018). These will be described in more detail in section 4.2.
As the PTOA surgical model cannot cover all the mechanical overloading phenotypes, here, we define a new term, biomechanically induced animal models (biomechanical model for short) to present all the mechanical overloading phenotypes of OA, including those that mimic ‘primary’ mechanically induced slow-progressive OA associated with chronic overloading which is naturally occurring after long-term lower levels of increased loading (mechanical overuse), and ‘secondary’ PTOA that develops in response to acute high impact overloading or trauma. Unlike genetically modified or chemically induced models, which primarily focus on altering genetic expressions or chemical interactions within the joint, biomechanically induced models specifically recreate the mechanical conditions contributing to OA development. The main underpinning rationale is to alter the joint mechanical challenges based on changing the loading distribution, or creating joint instability or joint defects. The following section will focus on these biomechanical aspects identified as key in animal models of biomechanical mechanisms in OA development.
4 Biomechanical aspects of in vivo models of OA
This section summarises recent advances in the development of mechanical overloading models. It also highlights that there remains a gap in ensuring that these mimic with sufficient precision the clinical condition in terms of the biomechanical aspects. We hypothesise that there are two key considerations when studying mechanically-driven OA, which are: 1) how to develop a mechanical model without inflammatory sequelae, and 2) how to induce OA without significant and immediate experimental trauma. Appropriate tackling of these concerns will enable the discrimination of changes indicative of early-stage OA in both the presence and absence of such potential injury-related onset, enabling comprehensive identification of mechanisms underpinning slow-progressive disease.
4.1 Isolating biomechanical changes from inflammation in post-traumatic OA models
Except for some specific metabolic phenotypes (e.g., obesity) in which a systemic inflammatory state promotes local joint inflammation by adipose tissue releasing adipokines and other proinflammatory cytokines into the blood stream (Trayhurn and Wood, 2004; Berenbaum et al., 2013) to cause OA, most OA aetiology does not have a primarily inflammatory focus. The inflammation observed in most forms of OA is indeed generally chronic, low-grade inflammation and thought to be an epiphenomenon induced by mechanical derangement (Poulet et al., 2012; Sokolove and Lepus, 2013; Robinson et al., 2016; Chow and Chin, 2020). The chronic inflammation in OA is likely a secondary process induced by cartilage damage, which can be understood as a progressive cycle of local tissue damage in an initial area, failed tissue repair, and inflammatory and innate immune response, resulting in further cartilage degeneration in the surrounding area over time (Robinson et al., 2016).
The inflammation process provoked by joint trauma has two phases: an acute posttraumatic phase lasting 2 months after the mechanical impact, with joint pain and swelling due to the intraarticular bleeding, prominent inflammation and synovial effusion (Lotz and Kraus, 2010), during which the damage progresses rapidly. Then most individuals develop into the chronic OA phase, from a long clinically asymptomatic period with constant low-grade inflammation, subtle metabolic changes and structure changes in cartilage, to a symptomatic phase (Lotz and Kraus, 2010). In addition, although OA is thought to be a local disease, lacking the large scale systemic response observed in rheumatoid arthritis, the increase of serum C-reactive protein levels associated with OA symptoms suggests that there is an accompanying chronic low-grade systemic inflammatory response in these patients (Stürmer et al., 2004; Berenbaum, 2013; Cicuttini and Wluka, 2014).
As outlined, the acute injury or long-term mechanical abnormities can cause a different inflammatory process in patients. Inflammation can potentially thus arise in biomechanical OA models from any of three sources: the tissue injury from the artefacts of surgery, the acute inflammation after mechanical injury/impact and the chronic low-grade inflammation from long-term subtle mechanical changes. It has been reported that such mechanical forces can either induce or suppress inflammatory signalling cascades through the mechano-transduction mechanism (Knapik et al., 2014), which creates uncertainty about the source of inflammatory signalling in these biomechanical OA models. Currently, a key challenge needing to be resolved in PTOA models is how to develop a purely biomechanical model excluding tissue injury and inflammation following the surgery. Since current surgical OA models can potentially cause acute inflammation due to the surgery, it is not clear the extent to which the invasive surgery caused the inflammatory response or the mechanical stress initiates the OA (Deschner et al., 2003). This could result in misleading conclusions on the possible effects of mechanical factors on local and systemic inflammation, and OA progression. Interestingly, one study observed surgical inflammation in the synovium in the first week in the MMT rat model, but this resolved by week three (Salazar-Noratto et al., 2019), suggesting that the inflammatory effect might reduce to zero if the study were to progress to later time points. A non-invasive biomechanical model would be a suitable method to avoid the risk of unnatural internal joint biomechanics and localised tissue damage-induced inflammation. Such models usually initiate OA through causing a closed insult without disrupting joint function or breaking the skin and joint capsule (Christiansen et al., 2015). The earliest non-invasive model used an indenter to apply a single impact to the knee, leading to an intra-articular fracture of the tibial plateau (Furman et al., 2007). Another traumatic, but non-invasive method is the compression model, in which the limb is positioned with upper and lower loading cups fixed to the calf, and a compressive axial load (12 N peak force) is applied to cause the anterior displacement of tibia to cause ACL overload and rupture. This model has a similar mechanism as a sports injury and can result in rapid OA development (Christiansen et al., 2012). A comparative study showed the surgical (invasive) ACLT model increased anteroposterior laxity, while biomechanical (non-invasive) ACL rupture failed to modify this parameter, supporting the view that the non-invasive model represents the OA process well. However, both models produce direct intra-articular tissue damage (ACL rupture, tibial plateau fracture).
To avoid uncertainty, the molecular markers of OA could be measured from different fluids by choosing specific biomarkers of degeneration and metabolism. The local response can be studied by obtaining biomarkers from the joint synovial fluid (SF), while systemic inflammatory states representing total body level are usually collected from serum and urine (Kyostio-Moore et al., 2011). The biomarkers analysed from SF can sensitively reflect changes related to early PTOA, while urine and serum levels can be influenced by different systemic diseases or metabolic conditions (Teeple et al., 2013). Several studies have shown the differential cytokine levels in circulation (serum) and SF (Frisbie et al., 2008; Kyostio-Moore et al., 2011). Some biomarkers are less detectable in serum than in SF, or showing no clear differentiation between healthy and diseased states, as these cytokines are primarily released and consumed locally in the environment after binding to specific receptors on local cells and triggering intracellular signals (De Groote et al., 1992; Burska et al., 2014). However, in rodent studies, blood sampling is more commonly used than SF as it is easily accessible and would not affect OA progression (Burska et al., 2014). Serum change may thus only partly reflect pathology but would be a good diagnostic biomarker and disease early screening test. Serum biomarker sensitivity may be increased by adding a mechanical stimulus (such as moderate exercise) to the basic OA model; this may correspond to a later disease stage. Research has shown that exercise-induced increases in serum COMP, such as from a 30-min walk, can predict 5-year OA disease progression (Erhart-Hledik et al., 2012; Chu et al., 2018).
4.2 Developing less-invasive biomechanical models to better present the chronic overload phenotype to understand the early stages of non-traumatic OA
Due to the lack of suitable models, little is known about the pathophysiology of the chronic progression of biomechanically induced OA, especially in the early stage. This is potentially distinct from current PTOA models as their rapid OA induction is too severe to replicate human OA and the speed of OA progression means that it is hard to detect OA onset. Also, subchondral bone microfractures that are markers of early-stage OA, are hard to observe in current animal models (Ramme et al., 2016). Patients with chronic overloading OA without known major injuries, and PTOA patients also differ in other ways such as muscle activation, gait pattern, knee mechanics (Robbins et al., 2019) and radiological characteristics (Swärd et al., 2010). There are therefore two points which need to be further studied in order to address these two mechanically-induced forms of OA, (PTOA and chronic overloading OA): 1. lessening the invasiveness/trauma for biomechanical models to replicate the early stage of PTOA, and 2. producing a non-traumatic method to study the chronic overloading OA phenotype.
Recent studies have characterised less invasive models with lower controlled single impact to better study the early stages of PTOA. One study investigated a dose-response relationship by arthroscopy to directly load the canine medial femoral condyle to create a controlled acute injury at multiple different loading levels; magnetic resonance imaging (MRI) and symptomatic assessments were used as outcome measures (Brimmo et al., 2016). It was found that an 18 MPa impact replicated a ‘physiological’ impact equivalent to daily activities, which is near the threshold (20 MPa) for chondrocyte apoptosis; 40 MPa caused an ‘athletic trauma’, which led to degenerative changes in animal models; and a 60 MPa impact led to a ‘severe trauma’, which is associated with PTOA changes. The lowest level successfully generated subchondral bone microfractures that closely mimic those found in patients’ knees (Brimmo et al., 2016). More severe soft tissue damage models have nuanced different severity levels: e.g., DMM is moderately severe, but less so than ACLT (Glasson et al., 2007). The DMM model sections the medial meniscotibial ligament to offload the medial meniscus; this produces mid-to moderate human OA with subchondral bone defects within 4 weeks (Glasson et al., 2007; Iijima et al., 2014; Culley et al., 2015).
Studies on the chronic overloading OA phenotype focus on developing a less-invasive model by the application of controlled, long-term slowly increased loads on the knee. These studies have utilised methods such as cyclic overloading (Ko et al., 2013), running exercise (Lapvetelainen et al., 1995; Lapvetelainen et al., 2002) and creating different angles of TO (Britzman et al., 2018). These are described, in turn, below.
The cyclic compression loading model is an advanced non-invasive method of OA induction that uses loading at a lower compressive force (below ACL-rupture threshold) with a longer duration. It is the only model that imposes long-term repetitive small adjustable elevated loads (Christiansen et al., 2015). The joint failure mechanism is the direct overload on cartilage and progresses over the time, which is distinct from the single loading model where the cartilage lesion is a secondary consequence of joint destabilization and did not worsen with time alone (Poulet, 2016). The method produces cartilage and subchondral bone lesions (indicators of early-stage OA) even with very low loads (4.5 N to 9N in rodent model; (Ko et al., 2013). This non-invasive cyclic compression model is a most promising model of the chronic OA phenotype induced through mechanical overuse and also used as an adjunct (1.0N∼2.0N) to DMM which induced a positive anabolic response after DMM injury (Holyoak et al., 2019).
Another method for non-invasively applying mechanical cyclic joint loads is through elevated exercise; this has been developed with treadmill or wheel running (Lapveteläinen et al., 1995). The level of exercise would need to be sufficient to induce or accelerate OA and has been found to increase the incidence and severity of knee cartilage damage in specific mouse strains with OA susceptibility (Lapvetelainen et al., 1995; Lapvetelainen et al., 2002; Baur et al., 2011). These exercise models could enhance spontaneous models by reducing the time taken to induce OA. In addition, as the exercise model directly represents the specific type of OA observed in patients with frequent occupational and sports activities, this model could be further developed to study the effects of activity factors on OA development. There is also a view that exercise stimulates circulating levels of anti-inflammatory cytokines to counteract the low-grade systemic inflammation in patients (Warnberg et al., 2010). Therefore, exercise is not commonly used to induce OA in isolation, but it is considered a confounder and so is assessed in order to differentiate the effects of exercise from exercise plus concurrent OA disease (Frisbie et al., 2008). Systemic effects are likely complicating factors here, as in other models.
TO is another promising biomechanical method to model chronic overload-induced OA. At present it is the only way to use extraarticular surgery to create OA in an animal model. TO has the advantage of a surgically induced method that precisely mimics the OA pathogenic mechanisms of abnormal joint load distribution and constantly small overloading. TO also provides more anatomical overloading direction compared to a cyclic loading machine, and avoids the joint damage of traditional intraarticular surgery. Clinically, osteotomy has been reported for over 2000 years (Smith et al., 2013) and is used for treating knee OA to improve pain and function (Brouwer et al., 2014) by unloading the affected knee compartment. The animal osteotomy models initiate cartilage overload by removing a wedge of bone in the proximal third of the tibia, distal to the tibial tubercle (Coventry, 1985). The transtibial valgus osteotomy model is most generally used, causing a shift in mechanical axis to the lateral knee compartment (Panula et al., 1997). This method has been used to induce OA in dogs (Johnson and Poole, 1988; Panula et al., 1997), rabbits (Reimann, 1973; Mankin et al., 1981) and guinea pigs (Wei et al., 1998). These studies have revealed various levels of degenerative change in either the overloaded compartment alone (Mankin et al., 1981) or both compartments (Reimann, 1973). However, the model is not popular and has not been well developed. The reasons for this may be the high level of surgical skill required, the cost, and the technical difficulties in keeping a stable osteotomy position during post-operative rehabilitation (Panula et al., 1997). A more recent study (Britzman et al., 2018) has created a 30° varus proximal TO model in healthy rats, which successfully induced OA as measured using biomechanical evaluations, histology and circulating-telopeptides of type II collagen (CTX-II, cartilage degradation biomarker). The optimal angles to investigate biomechanical factors in the natural course of OA are not known. Typically 30° malalignment is used in animal models as this causes OA changes within 3 months, yet clinical malalignment in patients is much smaller (Cooke et al., 2007). Animal biomechanics are significantly different from human due to quadrupedalism and anatomical differences, so it is hard to compare clinical and animal malalignment angles. Larger malalignments and incisions closer to the joint induce OA more quickly (Schipplein and Andriacchi, 1991) and even larger angles produce other non-physiological effects such as subluxation (Shoji and Insall, 1973; Wei et al., 1998).
5 Diagnostic tools used to identify biomechanically induced OA
There are three key diagnostic tools for biomechanically induced OA: biochemical, biomechanical and imaging markers to measure the biological, functional and structural OA changes (Andriacchi, 2012). Typically, microscopic tools (biochemical) are usually used in small animals, and macroscopic tools (biomechanical and imaging) are used in large animals and humans (Kuyinu et al., 2016). Characterizing biomechanical models and outcomes relies on measuring biomechanical factors, but biomechanical changes are very subtle in animal models, in particular in rodents due to their size and quadrupedalism, and a single mechanical factor might respond to both mechanical and inflammatory stimuli and cause multiple marker changes. This subtlety hinders early detection and characterization of this mechanically driven disease in animal models. Therefore, advanced diagnostic tools are needed for animal models in order to improve our understanding of this disease.
5.1 Standard measurement tools
The divergent in vivo OA models make it difficult to establish standard measurements. Studies that correlate the cartilage degradation, bone remodelling and biomechanics with OA development, progression and compare the outcome measures usually need a healthy group to provide baseline data, or an accepted scoring reference to compare with the diseased group. To standardise the measures and reporting techniques used in OA research, the Histological-Histochemical Grading System (HHGS) is the earliest and most often used cartilage scoring system for OA assessment in both human and animal models (Rutgers et al., 2010). The Osteoarthritis Research Society International (OARSI) scoring system was then established and has become a widely used alternative as it covers a broad range from the earlier mild OA to advanced OA; it has greater inter-rater reliability (Rutgers et al., 2010). OARSI has developed a species-specific histopathology grading system for OA animal models, mainly involving the changes in cartilage, synovial membrane and subchondral bone, based on an extensive literature review (Aigner et al., 2010). This enables comparison between different animal studies of OA, yet as the induction method between these models varies, so the robustness of the OARSI comparison is not clear.
Histopathology currently remains the gold standard for cartilage evaluation and OA in animal models. Safranin-O staining of its proteoglycan content is the classic way to visualise cartilage in order to assess any loss (Rutgers et al., 2010). For imaging markers, radiography is the classic gold standard and the most widely used way of OA imaging in clinic and research (Roemer et al., 2014; Kuyinu et al., 2016). Key structural radiographic hallmarks are joint space narrowing, osteophyte formation and subchondral sclerosis (Roos et al., 2011). Although there is a lack of sensitivity and not all joint structures can be identified, joint space narrowing detected in X-ray is still the standard in the assessment of OA progression and the efficacy of therapies (Roemer et al., 2014).Biochemical markers from joint tissue or blood samples, or elsewhere are important tools for evaluating joint tissue degeneration and remodeling in OA (Rousseau and Delmas, 2007). Specifically, markers of cartilage degradation focus on the metabolic balance between the synthesis and degradation of primary cartilage components like Type II collagen (Murphy and Lee, 2005). Key markers for increased collagen synthesis in early OA stages include Procollagen II N-terminal propeptide (PIIANP) (Shinmei et al., 1993), Cartilage Oligomeric Matrix Protein (COMP), and latexin, with Type II collagen and COMP being prominent early indicators in animal studies (Appleton et al., 2007; Legrand et al., 2017; Orhan et al., 2021). For collagen degradation, Coll2-1 and C-terminal telopeptide of type II collagen (CTX-II) are widely recognized, often serving as substitutes for histological assessments in both human and animal studies (Meulenbelt et al., 2006; Duclos et al., 2010; Legrand et al., 2017). The assessment of degradative enzymes such as matrix metalloproteinases (MMP-13 and -3) and aggrecanases (ADAMTS-4 and -5) provides insights into the cartilage tissue’s catabolic state. Inflammatory markers like interleukin-6 (IL-6), interleukin-1β (IL-1β), tumor necrosis factor-alpha (TNF-α), and Coll2-1NO2, which indicate oxidative stress, are critical for understanding inflammation and mechanical stress impacts on chondrocytes (Appleton et al., 2007; Legrand et al., 2017; Orhan et al., 2021). However, the same pattern of cytokines activity (high IL-6, TNF-α, MMP-3 expression) has also been observed in inflammatory (e.g., rheumatoid) arthritis, suggesting that these markers are an atypical presentation and may reflect inflammation rather than being disease specific (Tchetverikov et al., 2005; Burska et al., 2014).
In parallel, research on bone markers helps assess bone metabolic and turnover activities, essential for understanding subchondral bone dynamics in OA (Chapurlat and Confavreux, 2016). Markers linked to osteoblast differentiation and bone formation include bone morphogenetic proteins (BMP-2, BMP-7) (Ma et al., 2020), and elements of the receptor activator of nuclear factor kappa-Β ligand (RANKL)/RANK/osteoprotegerin (OPG) system (Walsh and Choi, 2014), along with periostin (POSTN) (Chapurlat et al., 2000), fibulin-3, and fibronectin (FN1). Markers of osteoclastogenesis and bone resorption, such as type I procollagen (PINP), Sclerostin, and WNT1 (Krishnan et al., 2006; Chapurlat and Confavreux, 2016), as well as Cathepsin K, a key catalytic enzyme involved in bone extracellular matrix degradation (Chapurlat, 2014), are also pivotal. These bone markers are emerging as potential new OA markers, offering insights into the relative differentiation of the metabolic activity of subchondral and trabecular bone compared to traditional joint structural changes from imaging (Chapurlat and Confavreux, 2016).
Despite the extensive range of markers recorded, obtaining joint tissue/fluid samples is challenging, and blood samples often lack sensitivity and specificity. As a result, no universally accepted gold standard biochemical marker exists in OA research and clinical practice. The search for specific biomarkers of biomechanically induced OA is ongoing, supported by recent advances in high-throughput methods such as transcriptomics, proteomics, and metabolomics that facilitate new discoveries in both human and animal studies. (Parra-Torres et al., 2014; de Sousa et al., 2017; Sieker et al., 2018; Zhai et al., 2018; Soul et al., 2021; Hahn et al., 2022).
5.2 Quantitative biomechanical markers and gait analysis
Apart from the standard tools described above, mechanical factors in biomechanically induced OA are receiving more attention because of their significant influence on joint loading with both experimental and computational tools being used. Mechanical factors are experimentally quantified by gait analysis technology, which uses motion analysis, force analysis and musculoskeletal dynamics to derive internal loading on tissues (Howard et al., 2000; Cleather and Bull, 2011; Eftaxiopoulou et al., 2014). Although gait analysis and biomechanical markers have been widely used in human studies (Hamai et al., 2009; Heiden et al., 2009; Yakhdani et al., 2010; Whittle, 2014), characterising the animal mechanical behaviour is challenging (Carmo et al., 2012; Sander et al., 2012; Sagawa et al., 2013; Olive et al., 2017). Despite these challenges, rodent gait models (Table 2) have been developed and used for rheumatoid arthritis (Berryman et al., 2009) and neuropathic pain behaviour research. Although these tools have not been widely applied in OA, they can provide a rich suite of biomechanical biomarkers (Table 2).
Knee flexion moment (KFM) and knee adduction moment (KAM) have been shown in clinical OA studies as surrogate measures of joint loading that are associated with cartilage thickness (Erhart-Hledik et al., 2015; Maly et al., 2015; Agrawal et al., 2019). KAM is more significantly correlated with severe OA, while peak KFM has a greater effect in the early-stages of OA (Erhart-Hledik et al., 2015; Zeng et al., 2017). Also, it is observed that OA knees have significantly reduced joint range of motion (ROM) (Zeng et al., 2017). Other derived measures such as KAM impulse and coupled vector angle have been shown to be associated with joint loading and structural severity of OA (Kean et al., 2011; Agrawal et al., 2019). These mechanics markers have been used in human OA gait analysis and are often applied in preclinical animal studies. For example, dynamic changes in ground reaction force (GFR) and gait asymmetry can be observed as early as 1 week post-surgery in the rat MMT model (Jacobs et al., 2017). Higher peak KAM and medial knee joint contact force and decreased peak flexion and extension velocities in the swing phase of gait have also been observed (Vilensky et al., 1994; Britzman et al., 2018). Gait compensation and gait asymmetry has been found to be a biomechanical marker correlated to the severity of cartilage lesions in a rat OA model (Allen et al., 2012), but this does not have a strong correlation with SF cytokines in the spontaneous dog OA model (Allen et al., 2019). Combining spatiotemporal, kinematic and dynamic gait analysis can measure more subtle gait changes (Eftaxiopoulou et al., 2014) and, when combined with musculoskeletal modelling (Johnson et al., 2008; Britzman et al., 2018) and computational finite element modelling, this can lead to tissue-specific analysis of local biomechanical overload. This has been applied extensively in humans (Zanjani-Pour et al., 2020) and presents potential in preclinical rodent models of chronic overload OA.
5.3 Advanced imaging methods and markers
Advanced non-invasive 3D imaging methods accelerate OA research as they non-destructively enable the assessment of the early state of OA to directly image cartilage and subchondral microstructure changes and synovitis. MRI is applicable for early diagnosis and prediction of OA because of its ability to visualise cartilage, subchondral bone marrow lesions (BMLs), synovium, menisci, ligament and hypertrophic chondrocytes, as well as to quantify cartilage glycosaminoglycan content (Brimmo et al., 2016; Link et al., 2017; Wang et al., 2018; Accart et al., 2022). MRI techniques have been used experimentally in large animals (swine (Unger et al., 2018)) to small rodents (McErlain et al., 2012) to characterise OA pathophysiology (Głodek et al., 2016), including the ability to obtain markers from machine learning approaches to predict cartilage lesion progression (Pedoia et al., 2017). Microscopic computed tomography (MicroCT) is another powerful 3D imaging technique for non-invasive joint structural evaluation, such as BMLs, healing and remodelling and has been used for early stage subchondral change quantification in rodents (Botter et al., 2006). Enhancements include the use of contrast agents (Stewart et al., 2013), and novel segmentation to assess mineralised joint microstructural changes as a result of OA in rats (Ramme et al., 2017). Recent research has highlighted that changes in subchondral tissues–including subchondral trabecular bone deterioration, subchondral microdamage, and subchondral plate thickness–may serve as new structural markers in the early stages of knee OA. These subchondral changes, observed with advanced MRI and CT scans, seem to come prior to histological lesions in the cartilage layer (Day et al., 2001; Hayami et al., 2006; Li et al., 2013). MRI’s capability to detect BMLs has been associated with subchondral microdamage, as evidenced by microCT scans (Felson et al., 2001), and is supported by findings in a canine model, which suggested that subchondral bone marrow oedema could be one of the earliest signs of OA (Libicher et al., 2005). This was partially validated by human studies showing that subchondral bone damage might be the most predictive of radiographic OA, compared to other early-stage features such as cartilage or meniscal damage (Roemer et al., 2015; Singh et al., 2019; Preiswerk et al., 2022).
Beyond the structural imaging techniques like microCT and MRI, molecular imaging techniques are crucial for detecting selective molecular activities that serve as imaging markers over the course of OA disease. Techniques such as PET (Positron Emission Tomography) and optical imaging offer significant insights (Lim et al., 2020). PET uses radiolabeled tracers, such as the commonly used 18F-Fludeoxyglucose, to detect regions of heightened metabolic activity. This can reveal regional blood flow and bone remodeling conditions in human OA (Beckers et al., 2006). In a rat ACLT OA model, the PET tracer 18F-fluoride showed increased uptake in the subchondral bone of weight-bearing areas of the medial femur and tibia (Umemoto et al., 2010).
Optical imaging methods like bioluminescence and fluorescence imaging utilize light-emitting probes to visualize target gene expression within tissues in live animals. For instance, the transgenic murine line (Acan-CreER-Ires-Luc) with an aggrecan (Acan) gene enhancer allows indirect monitoring of the transcriptional activity of the aggrecan gene in cartilage, as reported in a post-DMM surgery model (Cascio et al., 2014). In fluorescence optical imaging, the intra-articular injection of the far-red probe Cy5.5 conjugated to an antibody selective for reactive-oxygen-damaged type II collagen has been used in vivo in DMM mice models, serving as an imaging marker for monitoring cartilage degradation (Lim et al., 2015).
Photoacoustic (PA) imaging is a hybrid imaging modality based on optical and ultrasound imaging. Quantitative ultrasound imaging relies on echogenicity, where strong echoes are observed at the surfaces of the cartilage and sub-cartilage bone (Saïed et al., 2009). PA imaging uses a nano-second pulsed laser to illuminate biological tissue. The absorption coefficient of the tissues, which depends on their heat and elastic characteristics, generates different pressure waves (PA signals). These signals are then detected by an ultrasonic transducer, with stronger signals in tissues with higher vascular distribution (Hagiwara et al., 2015). Ultrasound and photoacoustic imaging can use a combined US/PA transducer to visualize the OA knee, providing complementary imaging information (Izumi et al., 2013). Additionally, cartilage-targeted biochemical PA contrast agents have been developed with sufficient PA intensity and targeted interaction with GAGs in cartilage. These agents sensitively reflect the reduced content of GAGs in the DMM mice model (Xiao et al., 2020).
6 Discussion
Knee OA is a multifactorial complex disease which is not yet fully understood. As a mechanically-driven or mediated disease, biomechanical factors are involved in all OA phenotypes. The aim of this review was to give a narrative description of preclinical in vivo models and advanced measurement tools to address these biomechanical factors in the context of clinical phenotypes. We hypothesised that there are two key considerations when studying mechanically driven OA, which are: 1) how to develop a mechanical model without inflammatory sequelae and 2) how to induce OA without significant experimental trauma and so enable the detection of changes indicative of early-stage OA in the absence of such sequelae.
We first summarised the evidence for the association between biomechanical factors with knee OA, including anatomical factors (joint morphology and limb alignment) and functional factors (knee injury, poor muscle function, reduced proprioceptive acuity, activities, and joint laxity). KOA has been categorised into two phenotypes (primary and secondary OA) and several subgroups based on aetiology and risk factors, yet they are frequently overlapping and OA in many individuals likely reflects a combination of many risk factors. Cohort case studies and meta-analyses have helped to narrow these down into six phenotypes based on the clinical characteristics (DellIsola et al., 2016). As abnormal intra-articular stress is thought to be a major determinant and key feature of OA pathology in all clinical phenotypes initiated by multiple factors, the mechanical mechanism has an overwhelming importance in OA pathophysiology and treatment (Brandt et al., 2009), suggesting that all OA might share a common final pathway linking the mechanical and biological process to cause joint lesions. Treating this single causative factor (mechanical stress) might prevent the disease process in all OA phenotypes. Thus, the focus is to identify broad biomechanical principles, but more work needs to be done to characterize the effect of single biomechanical factors on OA progression when considering the complex interaction between mechanical and biological factors.
Animal models may play a critical role in identifying biomechanical factors in OA which currently still rely on results from retrospective cohort studies or theoretical biomechanical studies. A major limitation for OA model research is that the OA resulting from different aetiologies are often lumped together within the same model despite the significant heterogeneity. As the disease characteristics of OA differ between phenotypes and the phenotype-specific treatment is needed, so animal models must take these into account.
In this review we build on recent work that classifies OA into six phenotypes, of which the mechanical overloading phenotype accounts for the highest proportion of OA incidences (DellIsola et al., 2016). We propose in this article that this overloading phenotype include distinct subcategories of the PTOA subtype that occurs after trauma and, separately, a new ‘primary’ chronic subtype that represents mechanical overloading phenotype after long-term knee overuse without known major injuries. Recent studies that compare post-traumatic OA and non-traumatic OA would seem to support this proposal. Some cohort studies have indeed found that radiological structural changes in the medial and lateral compartments are equally distributed in PTOA patients but are primarily in the medial compartment in non-traumatic patient (Swärd et al., 2010). Also, non-traumatic OA patients have higher quadriceps and lateral hamstring electromyography, and higher knee adduction angles and moments compared to PTOA (Robbins et al., 2019).
Accordingly, in vivo OA models need to be subcategorised and established to explicitly represent certain clinical phenotypes. Our review classifies the main models in the literature in the context of the clinical phenotypes. The comparative analysis of OA models in Table 1 underscores differences in utility for disease studies and the fidelity with which they replicate the OA process. We found that there are advances in modelling the PTOA phenotype through, for example, low impact arthroscopic insults, non-invasive ACL rupture or DMM. These have proven successful in reproducing subchondral bone changes that indicate the early stage of OA. Although there are models with external cyclic compression, extra-articular osteotomy and running exercise, a primary chronic overloading phenotype is, however, less well-modelled. Whilst invasive models are intuitively closer to PTOA and non-invasive models may be closer to the primary chronic overloading phenotype, this direct correspondence should not be assumed. Full analysis, including measures of inflammatory and biomechanical markers needs to be conducted to test these potential assumptions. If models could be developed that minimised or even stopped an acute inflammation stage and so slowed down the OA progression, then this would enable early-stage OA to be detected and analysed. Moreover, ensuring repeatability and reliability to minimize variability for these biomechanical models (both invasive and non-invasive) remains a challenge. Although these models more accurately replicate clinical conditions and thereby guide more relevant therapeutic developments, they often lack the rigorous standardization seen in chemically-induced models, which are preferred for their speed and cost-effectiveness in preliminary drug tests but fail to accurately reflect the disease’s true pathogenic processes or the underlying joint damage. Consequently, standardization is particularly vital for the widespread adoption of biomechanical models. There is a critical need for standardized protocols to enhance reproducibility across studies and boost the translational potential of research findings from biomechanical preclinical models into different subtypes of human OA.
Furthermore, for an animal model to succeed, well-defined variables and outcomes are crucial. These could include biochemical and imaging markers, mechanical measures and histological data. Advanced genetic and proteomic data can also be used for more precise phenotyping (endotypes) of these models. Lack of reliable diagnostic tools poses another concern in OA model development. This might partly explain the current poor translation from preclinical animal studies to human practice where diagnostics are entirely reliant on the presence of symptom and radiology evidence, and regulators follow this approach. Our review found that detailed quantitative biomechanical and imaging tools are not routinely used and no studies have used all of the most advanced tools simultaneously. There use in combination with other bio(chemical) markers would enable a full understanding of OA instigation and progression. Such an integrative multiscale experimental and computational framework has been applied in some large animal and human studies, and the application of this approach to small animals would facilitate many benefits due to cost, availability and genetic manipulation.
7 Conclusion
This review has identified a gap in the description of clinical OA phenotypes when applied to in vivo studies, particularly with reference to the non-traumatic chronic overloading phenotype and so we propose that the overloading phenotype include distinct subcategories of the PTOA subtype that occurs after trauma and, separately, a new ‘primary’ chronic overloading subtype that represents mechanical overloading OA phenotype after long-term knee overuse without known major injuries. This new ‘primary’ chronic overloading subtype is less well-modelled in the literature and we recommend enhanced efforts to address this. However, although invasive models are intuitively closer to PTOA and non-invasive models may be closer to the primary chronic overloading phenotype, this direct correspondence should not be assumed. Furthermore, alignment between OA onset and progression mechanisms in this ‘primary’ chronic overloading subtype and those contributing to the OA that arises in spontaneous animal models will better define their utility in translational studies.
Advances in biochemical, biomechanical and imaging biomarkers, combined with the opportunities that such new small animal models provide would enable the better development of early diagnosis of the most prevalent form of knee OA.
Author contributions
LX: Writing–original draft, Writing–review and editing. ZK: Writing–review and editing. AP: Writing–review and editing. AB: Supervision, Writing–review and editing, Funding acquisition.
Funding
The author(s) declare that financial support was received for the research, authorship, and/or publication of this article. This work was conducted under the auspices of the Royal British Legion Centre for Blast Injury Studies at Imperial College London. The authors would like to acknowledge the financial support of the Royal British Legion.
Conflict of interest
The authors declare that the research was conducted in the absence of any commercial or financial relationships that could be construed as a potential conflict of interest.
Publisher’s note
All claims expressed in this article are solely those of the authors and do not necessarily represent those of their affiliated organizations, or those of the publisher, the editors and the reviewers. Any product that may be evaluated in this article, or claim that may be made by its manufacturer, is not guaranteed or endorsed by the publisher.
References
Aaboe, J., Bliddal, H., Messier, S. P., Alkjaer, T., and Henriksen, M. (2011). Effects of an intensive weight loss program on knee joint loading in obese adults with knee osteoarthritis. Osteoarthr. Cartil. 19 (7), 822–828. doi:10.1016/j.joca.2011.03.006
Accart, N., Dawson, J., Obrecht, M., Lambert, C., Flueckiger, M., Kreider, J., et al. (2022). Degenerative joint disease induced by repeated intra-articular injections of monosodium urate crystals in rats as investigated by translational imaging. Sci. Rep. 12 (1), 157. doi:10.1038/s41598-021-04125-7
Adeyemi, W. J., and Olayaki, L. A. (2017). Effects of single or combined administration of salmon calcitonin and omega-3 fatty acids vs. diclofenac sodium in sodium monoiodoacetate-induced knee osteoarthritis in male Wistar rats. J. Basic Clin. Physiol. Pharmacol. 28 (6), 573–582. doi:10.1515/jbcpp-2017-0032
Agrawal, V., Oh, J., Milian, E., Kim, K., Eltoukhy, M., and Best, T. (2019). A novel biomechanical marker for determining the severity of knee osteoarthritis by quantifying shock absorption at the knee. Osteoarthr. Cartil. 27, S67–S67. doi:10.1016/j.joca.2019.02.094
Aigner, T., Cook, J. L., Gerwin, N., Glasson, S. S., Laverty, S., Little, C. B., et al. (2010). Histopathology atlas of animal model systems - overview of guiding principles. Osteoarthr. Cartil. 18 (Suppl. 3), S2–S6. doi:10.1016/j.joca.2010.07.013
Ali, T. S., Prasadam, I., Xiao, Y., and Momot, K. I. (2018). Progression of Post-Traumatic Osteoarthritis in rat meniscectomy models: comprehensive monitoring using MRI. Sci. Rep. 8, 6861. doi:10.1038/s41598-018-25186-1
Allen, K., Thoma, L., and Golightly, Y. (2022). Epidemiology of osteoarthritis. Osteoarthr. Cartil. 30 (2), 184–195. doi:10.1016/j.joca.2021.04.020
Allen, K. D., Chan, K. M., Yarmola, E. G., Shah, Y. Y., and Partain, B. D. (2020). The effects of age on the severity of joint damage and intra-articular inflammation following a simulated medial meniscus injury in 3, 6, and 9 month old male rats. Connect. Tissue Res. 61 (1), 82–94. doi:10.1080/03008207.2019.1641495
Allen, K. D., Mata, B. A., Gabr, M. A., Huebner, J. L., Adams, S. B., Kraus, V. B., et al. (2012). Kinematic and dynamic gait compensations resulting from knee instability in a rat model of osteoarthritis. Arthritis Res. Ther. 14 (2), R78. doi:10.1186/ar3801
Allen, P. I., Conzemius, M. G., Evans, R. B., and Kiefer, K. (2019). Correlation between synovial fluid cytokine concentrations and limb function in normal dogs and in dogs with lameness from spontaneous osteoarthritis. Vet. Surg. 48 (5), 770–779. doi:10.1111/vsu.13212
Altman, R., Asch, E., Bloch, D., Bole, G., Borenstein, D., Brandt, K., et al. (1986). Development of criteria for the classification and reporting of osteoarthritis: classification of osteoarthritis of the knee. Arthritis Rheum. 29 (8), 1039–1049. doi:10.1002/art.1780290816
Anderson, G. P. (2008). Endotyping asthma: new insights into key pathogenic mechanisms in a complex, heterogeneous disease. Lancet 372 (9643), 1107–1119. doi:10.1016/s0140-6736(08)61452-x
Andriacchi, T. P. (2012). Osteoarthritis: probing knee OA as a system responding to a stimulus. Nat. Rev. Rheumatol. 8 (7), 371–372. doi:10.1038/nrrheum.2012.59
Appleton, C. T., Pitelka, V., Henry, J., and Beier, F. (2007). Global analyses of gene expression in early experimental osteoarthritis. Arthritis Rheum. 56 (6), 1854–1868. doi:10.1002/art.22711
Arden, N., and Nevitt, M. C. (2006). Osteoarthritis: epidemiology. Best. Pract. Res. Clin. Rheumatol. 20 (1), 3–25. doi:10.1016/j.berh.2005.09.007
Arzi, B., Wisner, E. R., Huey, D. J., Kass, P. H., Hu, J., and Athanasiou, K. A. (2012). A proposed model of naturally occurring osteoarthritis in the domestic rabbit. Lab. Anim. 41 (1), 20–25. doi:10.1038/laban0112-20
Baur, A., Henkel, J., Bloch, W., Treiber, N., Scharffetter-Kochanek, K., Brüggemann, G.-P., et al. (2011). Effect of exercise on bone and articular cartilage in heterozygous manganese superoxide dismutase (SOD2) deficient mice. Free Radic. Res. 45 (5), 550–558. doi:10.3109/10715762.2011.555483
Beckers, C., Jeukens, X., Ribbens, C., André, B., Marcelis, S., Leclercq, P., et al. (2006). 18 F-FDG PET imaging of rheumatoid knee synovitis correlates with dynamic magnetic resonance and sonographic assessments as well as with the serum level of metalloproteinase-3. Eur. J. Nucl. Med. Mol. imaging 33, 275–280. doi:10.1007/s00259-005-1952-3
Bendele, A. M. (2001). Animal models of osteoarthritis. J. Musculoskelet. Neuronal Interact. 1 (4), 363–376.
Bennell, K., Hunter, D. J., and Vicenzino, B. (2012). Long-term effects of sport: preventing and managing OA in the athlete. Nat. Rev. Rheumatol. 8 (12), 747–752. doi:10.1038/nrrheum.2012.119
Berenbaum, F. (2013). Osteoarthritis as an inflammatory disease (osteoarthritis is not osteoarthrosis!). Osteoarthr. Cartil. 21 (1), 16–21. doi:10.1016/j.joca.2012.11.012
Berenbaum, F., Eymard, F., and Houard, X. (2013). Osteoarthritis, inflammation and obesity. Curr. Opin. Rheumatol. 25 (1), 114–118. doi:10.1097/BOR.0b013e32835a9414
Berryman, E. R., Harris, R. L., Moalli, M., and Bagi, C. M. (2009). Digigait quantitation of gait dynamics in rat rheumatoid arthritis model. J. Musculoskelet. Neuronal Interact. 9 (2), 89–98.
Bierma-Zeinstra, S. M., and van Middelkoop, M. (2017). Osteoarthritis: in search of phenotypes. Nat. Rev. Rheumatol. 13 (12), 705–706. doi:10.1038/nrrheum.2017.181
Botter, S. M., van Osch, G. J., Waarsing, J. H., Day, J. S., Verhaar, J. A., Pols, H. A., et al. (2006). Quantification of subchondral bone changes in a murine osteoarthritis model using micro-CT. Biorheology 43 (3), 379–388.
Brandt, K. D., Dieppe, P., and Radin, E. L. (2009). Commentary: is it useful to subset "primary" osteoarthritis? A critique based on evidence regarding the etiopathogenesis of osteoarthritis. Semin. Arthritis Rheum. 39 (2), 81–95. doi:10.1016/j.semarthrit.2009.06.001
Brederson, J. D., Chu, K. L., Xu, J., Nikkel, A. L., Markosyan, S., Jarvis, M. F., et al. (2018). Characterization and comparison of rat monosodium iodoacetate and medial meniscal tear models of osteoarthritic pain. J. Orthop. Res. 36 (8), 2109–2117. doi:10.1002/jor.23869
Brimmo, O. A., Pfeiffer, F., Bozynski, C. C., Kuroki, K., Cook, C., Stoker, A., et al. (2016). Development of a novel canine model for posttraumatic osteoarthritis of the knee. J. Knee Surg. 29 (3), 235–241. doi:10.1055/s-0035-1549026
Brioschi, F. A., Di Cesare, F., Gioeni, D., Rabbogliatti, V., Ferrari, F., D’Urso, E. S., et al. (2020). Oral transmucosal cannabidiol oil formulation as part of a multimodal analgesic regimen: effects on pain relief and quality of life improvement in dogs affected by spontaneous osteoarthritis. Animals 10 (9), 1505. doi:10.3390/ani10091505
Britzman, D., Igah, I., Eftaxiopoulou, T., Macdonald, W., and Bull, A. M. J. (2018). Tibial osteotomy as a mechanical model of primary osteoarthritis in rats. Sci. Rep. 8, 5132. doi:10.1038/s41598-018-23405-3
Brouwer, G. M., van Tol, A. W., Bergink, A. P., Belo, J. N., Bernsen, R. M., Reijman, M., et al. (2007). Association between valgus and varus alignment and the development and progression of radiographic osteoarthritis of the knee. Arthritis Rheum. 56 (4), 1204–1211. doi:10.1002/art.22515
Brouwer, R. W., Huizinga, M. R., Duivenvoorden, T., van Raaij, T. M., Verhagen, A. P., Bierma-Zeinstra, S. M., et al. (2014). Osteotomy for treating knee osteoarthritis. Cochrane Database Syst. Rev. 12, CD004019. doi:10.1002/14651858.CD004019.pub4
Brown, S. B., Hornyak, J. A., Jungels, R. R., Shah, Y. Y., Yarmola, E. G., Allen, K. D., et al. (2020). Characterization of post-traumatic osteoarthritis in rats following anterior cruciate ligament rupture by non-invasive knee injury (NIKI). J. Orthop. Res. 38 (2), 356–367. doi:10.1002/jor.24470
Brown, T. D., Johnston, R. C., Saltzman, C. L., Marsh, J. L., and Buckwalter, J. A. (2006). Posttraumatic osteoarthritis: a first estimate of incidence, prevalence, and burden of disease. J. Orthop. Trauma 20 (10), 739–744. doi:10.1097/01.bot.0000246468.80635.ef
Burska, A., Boissinot, M., and Ponchel, F. (2014). Cytokines as biomarkers in rheumatoid arthritis. Mediat. Inflamm. 2014, 1–24. doi:10.1155/2014/545493
Cake, M. A., Appleyard, R. C., Read, R. A., Smith, M. M., Murrell, G. A., and Ghosh, P. (2005). Ovariectomy alters the structural and biomechanical properties of ovine femoro-tibial articular cartilage and increases cartilage iNOS. Osteoarthr. Cartil. 13 (12), 1066–1075. doi:10.1016/j.joca.2005.07.001
Carmo, A. A., Kleiner, A. F. R., da Costa, P. H. L., and Barros, R. M. L. (2012). Three-dimensional kinematic analysis of upper and lower limb motion during gait of post-stroke patients. Braz. J. Med. Biol. Res. 45 (6), 537–545. doi:10.1590/S0100-879x2012007500051
Cascio, L. L., Liu, K., Nakamura, H., Chu, G., Lim, N. H., Chanalaris, A., et al. (2014). Generation of a mouse line harboring a bi-transgene expressing luciferase and tamoxifen-activatable creERT2 recombinase in cartilage. genesis 52 (2), 110–119. doi:10.1002/dvg.22734
Castaneda, S., Calvo, E., Largo, R., Gonzalez-Gonzalez, R., de la Piedra, C., Diaz-Curiel, M., et al. (2008). Characterization of a new experimental model of osteoporosis in rabbits. J. Bone Min. Metab. 26 (1), 53–59. doi:10.1007/s00774-007-0797-1
Castaneda, S., Largo, R., Calvo, E., Bellido, M., Gomez-Vaquero, C., and Herrero-Beaumont, G. (2010). Effects of estrogen deficiency and low bone mineral density on healthy knee cartilage in rabbits. J. Orthop. Res. 28 (6), 812–818. doi:10.1002/jor.21054
Chapurlat, R., Garnero, P., Sornay-Rendu, E., Arlot, M., Claustrat, B., and Delmas, P. (2000). Longitudinal study of bone loss in pre-and perimenopausal women: evidence for bone loss in perimenopausal women. Osteoporos. Int. 11, 493–498. doi:10.1007/s001980070091
Chapurlat, R. D. (2014). Treatment of postmenopausal osteoporosis with odanacatib. Expert Opin. Pharmacother. 15 (4), 559–564. doi:10.1517/14656566.2014.881470
Chapurlat, R. D., and Confavreux, C. B. (2016). Novel biological markers of bone: from bone metabolism to bone physiology. Rheumatology 55 (10), 1714–1725. doi:10.1093/rheumatology/kev410
Chen, J. H., Liu, C., You, L., and Simmons, C. A. (2010). Boning up on Wolff’s Law: mechanical regulation of the cells that make and maintain bone. J. Biomech. 43 (1), 108–118. doi:10.1016/j.jbiomech.2009.09.016
Cheng, F., Yan, F. F., Liu, Y. P., Cong, Y., Sun, K. F., and He, X. M. (2019). Dexmedetomidine inhibits the NF-κB pathway and NLRP3 inflammasome to attenuate papain-induced osteoarthritis in rats. Pharm. Biol. 57 (1), 649–659. doi:10.1080/13880209.2019.1651874
Chow, Y. Y., and Chin, K. Y. (2020). The role of inflammation in the pathogenesis of osteoarthritis. Mediat. Inflamm. 2020, 8293921. doi:10.1155/2020/8293921
Christiansen, B. A., Anderson, M. J., Lee, C. A., Williams, J. C., Yik, J. H. N., and Haudenschild, D. R. (2012). Musculoskeletal changes following non-invasive knee injury using a novel mouse model of post-traumatic osteoarthritis. Osteoarthr. Cartil. 20 (7), 773–782. doi:10.1016/j.joca.2012.04.014
Christiansen, B. A., Guilak, F., Lockwood, K. A., Olson, S. A., Pitsillides, A. A., Sandell, L. J., et al. (2015). Non-invasive mouse models of post-traumatic osteoarthritis. Osteoarthr. Cartil. 23 (10), 1627–1638. doi:10.1016/j.joca.2015.05.009
Chu, C. R., Sheth, S., Erhart-Hledik, J. C., Do, B., Titchenal, M. R., and Andriacchi, T. P. (2018). Mechanically stimulated biomarkers signal cartilage changes over 5 years consistent with disease progression in medial knee osteoarthritis patients. J. Orthop. Res. 36 (3), 891–897. doi:10.1002/jor.23720
Cicuttini, F. M., and Wluka, A. E. (2014). Is OA a mechanical or systemic disease? Nat. Rev. Rheumatol. 10 (9), 515–516. doi:10.1038/nrrheum.2014.114
Cleather, D. J., and Bull, A. M. J. (2011). An optimization-based simultaneous approach to the determination of muscular, ligamentous, and joint contact forces provides insight into musculoligamentous interaction. Ann. Biomed. Eng. 39 (7), 1925–1934. doi:10.1007/s10439-011-0303-8
Cooke, T. D., Sled, E. A., and Scudamore, R. A. (2007). Frontal plane knee alignment: a call for standardized measurement. J. Rheumatol. 34 (9), 1796–1801.
Cope, P. J., Ourradi, K., Li, Y., and Sharif, M. (2019). Models of osteoarthritis: the good, the bad and the promising. Osteoarthr. Cartil. 27 (2), 230–239. doi:10.1016/j.joca.2018.09.016
Coventry, M. B. (1985). Upper tibial osteotomy for osteoarthritis. J. Bone Jt. Surg. Am. 67 (7), 1136–1140. doi:10.2106/00004623-198567070-00025
Culley, K. L., Dragomir, C. L., Chang, J., Wondimu, E. B., Coico, J., Plumb, D. A., et al. (2015). Mouse models of osteoarthritis: surgical model of posttraumatic osteoarthritis induced by destabilization of the medial meniscus. Methods Mol. Biol. 1226, 143–173. doi:10.1007/978-1-4939-1619-1_12
Dai, G., Wang, S., Li, J., Liu, C., and Liu, Q. (2006). The validity of osteoarthritis model induced by bilateral ovariectomy in Guinea pig. J. Huazhong Univ. Sci. Technol. Med. Sci. 26 (6), 716–719. doi:10.1007/s11596-006-0624-2
Das Neves Borges, P., Forte, A. E., Vincent, T. L., Dini, D., and Marenzana, M. (2014). Rapid, automated imaging of mouse articular cartilage by microCT for early detection of osteoarthritis and finite element modelling of joint mechanics. Osteoarthr. Cartil. 22 (10), 1419–1428. doi:10.1016/j.joca.2014.07.014
Day, J. S., Ding, M., van der Linden, J. C., Hvid, I., Sumner, D. R., and Weinans, H. (2001). A decreased subchondral trabecular bone tissue elastic modulus is associated with pre-arthritic cartilage damage. J. Orthop. Res. 19 (5), 914–918. doi:10.1016/s0736-0266(01)00012-2
De Groote, D., Zangerle, P. F., Gevaert, Y., Fassotte, M. F., Beguin, Y., Noizat-Pirenne, F., et al. (1992). Direct stimulation of cytokines (IL-1β, TNF-α, IL-6, IL-2, IFN-γ and GM-CSF) in whole blood. I. Comparison with isolated PBMC stimulation. Cytokine 4 (3), 239–248. doi:10.1016/1043-4666(92)90062-v
DellIsola, A., Allan, R., Smith, S. L., Marreiros, S. S., and Steultjens, M. (2016). Identification of clinical phenotypes in knee osteoarthritis: a systematic review of the literature. BMC Musculoskelet. Disord. 17 (1), 425. doi:10.1186/s12891-016-1286-2
Delp, S. L., Anderson, F. C., Arnold, A. S., Loan, P., Habib, A., John, C. T., et al. (2007). OpenSim: open-source software to create and analyze dynamic simulations of movement. IEEE Trans. Biomed. Eng. 54 (11), 1940–1950. doi:10.1109/TBME.2007.901024
Deschner, J., Hofman, C. R., Piesco, N. P., and Agarwal, S. (2003). Signal transduction by mechanical strain in chondrocytes. Curr. Opin. Clin. Nutr. Metab. Care 6 (3), 289–293. doi:10.1097/01.mco.0000068964.34812.2b
de Sousa, E. B., dos Santos, G. C., Duarte, M. E. L., Neto, V. M., and Aguiar, D. P. (2017). Metabolomics as a promising tool for early osteoarthritis diagnosis. Braz. J. Med. Biol. Res. 50 (11), e6485. doi:10.1590/1414-431X20176485
Deveza, L. A., and Loeser, R. F. (2018). Is osteoarthritis one disease or a collection of many? Rheumatol. Oxf. 57 (Suppl. l_4), iv34–iv42. doi:10.1093/rheumatology/kex417
Deveza, L. A., Nelson, A. E., and Loeser, R. F. (2019). Phenotypes of osteoarthritis: current state and future implications. Clin. Exp. Rheumatol. 37 (5), 64–72.
Duclos, M. E., Roualdes, O., Cararo, R., Rousseau, J. C., Roger, T., and Hartmann, D. J. (2010). Significance of the serum CTX-II level in an osteoarthritis animal model: a 5-month longitudinal study. Osteoarthr. Cartil. 18 (11), 1467–1476. doi:10.1016/j.joca.2010.07.007
Dugdale, T. W., Noyes, F. R., and Styer, D. (1992). Preoperative planning for high tibial osteotomy. The effect of lateral tibiofemoral separation and tibiofemoral length. Clin. Orthop. Relat. Res. 274, 248–264. doi:10.1097/00003086-199201000-00025
Eftaxiopoulou, T., Macdonald, W., Britzman, D., and Bull, A. M. J. (2014). Gait compensations in rats after a temporary nerve palsy quantified using temporo-spatial and kinematic parameters. J. Neurosci. Methods 232, 16–23. doi:10.1016/j.jneumeth.2014.04.011
Erhart-Hledik, J. C., Favre, J., and Andriacchi, T. P. (2015). New insight in the relationship between regional patterns of knee cartilage thickness, osteoarthritis disease severity, and gait mechanics. J. Biomechanics 48 (14), 3868–3875. doi:10.1016/j.jbiomech.2015.09.033
Erhart-Hledik, J. C., Favre, J., Asay, J. L., Smith, R. L., Giori, N. J., Mündermann, A., et al. (2012). A relationship between mechanically-induced changes in serum cartilage oligomeric matrix protein (COMP) and changes in cartilage thickness after 5 years. Osteoarthr. Cartil. 20 (11), 1309–1315. doi:10.1016/j.joca.2012.07.018
Esdaille, C. J., Ude, C. C., and Laurencin, C. T. (2022). Regenerative engineering animal models for knee osteoarthritis. Regen. Eng. Transl. Med. 8 (2), 284–297. doi:10.1007/s40883-021-00225-y
Felson, D. T., Chaisson, C. E., Hill, C. L., Totterman, S. M. S., Gale, M. E., Skinner, K. M., et al. (2001). The association of bone marrow lesions with pain in knee osteoarthritis. Ann. Intern. Med. 134 (7), 541–549. doi:10.7326/0003-4819-134-7-200104030-00007
Figueroa, D., Espinosa, M., Calvo, R., Scheu, M., Valderrama, J. J., Gallegos, M., et al. (2014). Treatment of acute full-thickness chondral defects with high molecular weight hyaluronic acid; an experimental model. Rev. Esp. Cir. Ortop. Traumatol. 58 (5), 261–266. doi:10.1016/j.recot.2014.03.001
Flanigan, D. C., Harris, J. D., Brockmeier, P. M., and Siston, R. A. (2010). The effects of lesion size and location on subchondral bone contact in experimental knee articular cartilage defects in a bovine model. Arthroscopy-the J. Arthrosc. Relat. Surg. 26 (12), 1655–1661. doi:10.1016/j.arthro.2010.05.017
Frisbie, D. D., Al-Sobayil, F., Billinghurst, R. C., Kawcak, C. E., and McIlwraith, C. W. (2008). Changes in synovial fluid and serum biomarkers with exercise and early osteoarthritis in horses. Osteoarthr. Cartil. 16 (10), 1196–1204. doi:10.1016/j.joca.2008.03.008
Furman, B. D., Strand, L., Hembree, W. C., Ward, B. D., Guilak, F., and Olson, S. A. (2007). Joint degeneration following closed Intraarticular fracture in the mouse knee: a model of posttraumatic arthritis. J. Orthop. Res. 25 (5), 578–592. doi:10.1002/jor.20331
Gardiner, B. S., Woodhouse, F. G., Besier, T. F., Grodzinsky, A. J., Lloyd, D. G., Zhang, L., et al. (2016). Predicting knee osteoarthritis. Ann. Biomed. Eng. 44 (1), 222–233. doi:10.1007/s10439-015-1393-5
GBD 2017 Disease and Injury Incidence and Prevalence Collaborators, Abate, D., Abate, K. H., Abay, S. M., Abbafati, C., Abbasi, N., et al. (2018). Global, regional, and national incidence, prevalence, and years lived with disability for 354 diseases and injuries for 195 countries and territories, 1990-2017: a systematic analysis for the Global Burden of Disease Study 2017. Lancet 392 (10159), 1789–1858. doi:10.1016/S0140-6736(18)32279-7
Gilbert, S. J., and Blain, E. J. (2018). “Chapter 4 - cartilage mechanobiology: how chondrocytes respond to mechanical load,” in Mechanobiology in health and disease. Editor S. W. Verbruggen (Academic Press), 99–126.
Gilbertson, E. M. (1975). Development of periarticular osteophytes in experimentally induced osteoarthritis in the dog. A study using microradiographic, microangiographic, and fluorescent bone-labelling techniques. Ann. Rheum. Dis. 34 (1), 12–25. doi:10.1136/ard.34.1.12
Glasson, S. S., Blanchet, T. J., and Morris, E. A. (2007). The surgical destabilization of the medial meniscus (DMM) model of osteoarthritis in the 129/SvEv mouse. Osteoarthr. Cartil. 15 (9), 1061–1069. doi:10.1016/j.joca.2007.03.006
Głodek, J., Adamiak, Z., and Przeworski, A. (2016). Magnetic resonance imaging of reptiles, rodents, and lagomorphs for clinical diagnosis and animal research. Comp. Med. 66 (3), 216–219.
Glyn-Jones, S., Palmer, A. J., Agricola, R., Price, A. J., Vincent, T. L., Weinans, H., et al. (2015). Osteoarthr. Lancet 386 (9991), 376–387. doi:10.1016/S0140-6736(14)60802-3
Goetz, J. E., Coleman, M. C., Fredericks, D. C., Petersen, E., Martin, J. A., McKinley, T. O., et al. (2017). Time-dependent loss of mitochondrial function precedes progressive histologic cartilage degeneration in a rabbit meniscal destabilization model. J. Orthop. Res. 35 (3), 590–599. doi:10.1002/jor.23327
Griffin, T. M., and Guilak, F. (2005). The role of mechanical loading in the onset and progression of osteoarthritis. Exerc Sport Sci. Rev. 33 (4), 195–200. doi:10.1097/00003677-200510000-00008
Guilak, F. (2011). Biomechanical factors in osteoarthritis. Best Pract. Res. Clin. Rheumatology 25 (6), 815–823. doi:10.1016/j.berh.2011.11.013
Hagiwara, Y., Izumi, T., Yabe, Y., Sato, M., Sonofuchi, K., Kanazawa, K., et al. (2015). Simultaneous evaluation of articular cartilage and subchondral bone from immobilized knee in rats by photoacoustic imaging system. J. Orthop. Sci. 20 (2), 397–402. doi:10.1007/s00776-014-0692-2
Hahn, A. K., Rawle, R. A., Bothner, B., Lopes, E. B. P., Griffin, T. M., and June, R. K. (2022). In vivo mechanotransduction: effect of acute exercise on the metabolomic profiles of mouse synovial fluid. Osteoarthr. Cartil. Open 4 (1), 100228. doi:10.1016/j.ocarto.2021.100228
Halder, A., Kutzner, I., Graichen, F., Heinlein, B., Beier, A., and Bergmann, G. (2012). Influence of limb alignment on mediolateral loading in total knee replacement: in vivo measurements in five patients. J. Bone Jt. Surg. Am. 94 (11), 1023–1029. doi:10.2106/jbjs.K.00927
Hamai, S., Moro-oka, T. A., Miura, H., Shimoto, T., Higaki, H., Fregly, B. J., et al. (2009). Knee kinematics in medial osteoarthritis during in vivo weight-bearing activities. J. Orthop. Res. 27 (12), 1555–1561. doi:10.1002/jor.20928
Hayami, T., Pickarski, M., Zhuo, Y., Wesolowski, G. A., Rodan, G. A., and Duong, L. T. (2006). Characterization of articular cartilage and subchondral bone changes in the rat anterior cruciate ligament transection and meniscectomized models of osteoarthritis. Bone 38 (2), 234–243. doi:10.1016/j.bone.2005.08.007
Heiden, T. L., Lloyd, D. G., and Ackland, T. R. (2009). Knee joint kinematics, kinetics and muscle co-contraction in knee osteoarthritis patient gait. Clin. Biomech. (Bristol, Avon) 24 (10), 833–841. doi:10.1016/j.clinbiomech.2009.08.005
Herrero-Beaumont, G., Roman-Blas, J. A., Castañeda, S., and Jimenez, S. A. (2009). Primary osteoarthritis no longer primary: three subsets with distinct etiological, clinical, and therapeutic characteristics. Semin. Arthritis Rheum. 39 (2), 71–80. doi:10.1016/j.semarthrit.2009.03.006
Hoegh-Andersen, P., Tanko, L. B., Andersen, T. L., Lundberg, C. V., Mo, J. A., Heegaard, A. M., et al. (2004). Ovariectomized rats as a model of postmenopausal osteoarthritis: validation and application. Arthritis Res. Ther. 6 (2), R169–R180. doi:10.1186/ar1152
Holyoak, D. T., Chlebek, C., Kim, M. J., Wright, T. M., Otero, M., and van der Meulen, M. C. H. (2019). Low-level cyclic tibial compression attenuates early osteoarthritis progression after joint injury in mice. Osteoarthr. Cartil. 27 (10), 1526–1536. doi:10.1016/j.joca.2019.06.005
Howard, C. S., Blakeney, D. C., Medige, J., Moy, O. J., and Peimer, C. A. (2000). Functional assessment in the rat by ground reaction forces. J. Biomechanics 33(6), 751–757. doi:10.1016/S0021-9290(00)00023-3
Hunt, M. A., Charlton, J. M., and Esculier, J. F. (2020). Osteoarthritis year in review 2019: mechanics. Osteoarthr. Cartil. 28 (3), 267–274. doi:10.1016/j.joca.2019.12.003
Hunter, D. J., and Bierma-Zeinstra, S. (2019). Osteoarthr. Lancet 393 (10182), 1745–1759. doi:10.1016/S0140-6736(19)30417-9
Iijima, H., Aoyama, T., Ito, A., Tajino, J., Nagai, M., Zhang, X., et al. (2014). Destabilization of the medial meniscus leads to subchondral bone defects and site-specific cartilage degeneration in an experimental rat model. Osteoarthr. Cartil. 22 (7), 1036–1043. doi:10.1016/j.joca.2014.05.009
Issa, R. I., and Griffin, T. M. (2012). Pathobiology of obesity and osteoarthritis: integrating biomechanics and inflammation. Pathobiol. Aging Age Relat. Dis. 2 (2012), 17470. doi:10.3402/pba.v2i0.17470
Izumi, T., Sato, M., Yabe, Y., Hagiwara, Y., and Saijo, Y. (2013). “Ultrasonic and photoacoustic imaging of knee joints in normal and osteoarthritis rats,” in 2013 35th annual international conference of the IEEE engineering in medicine and biology society (Osaka, Japan: EMBC), 1116–1119.
Jackson, D. W., Grood, E. S., Goldstein, J. D., Rosen, M. A., Kurzweil, P. R., Cummings, J. F., et al. (1993). A comparison of patellar tendon autograft and allograft used for anterior cruciate ligament reconstruction in the goat model. Am. J. Sports Med. 21(2), 176–185. doi:10.1177/036354659302100203
Jacobs, B. Y., Dunnigan, K., Pires-Fernandes, M., and Allen, K. D. (2017). Unique spatiotemporal and dynamic gait compensations in the rat monoiodoacetate injection and medial meniscus transection models of knee osteoarthritis. Osteoarthr. Cartil. 25 (5), 750–758. doi:10.1016/j.joca.2016.12.012
Jacobs, B. Y., Kloefkorn, H. E., and Allen, K. D. (2014). Gait analysis methods for rodent models of osteoarthritis. Curr. Pain Headache Rep. 18 (10), 456. doi:10.1007/s11916-014-0456-x
Jimenez, P. A., Glasson, S. S., Trubetskoy, O. V., and Haimes, H. B. (1997). Spontaneous osteoarthritis in Dunkin Hartley Guinea pigs: histologic, radiologic, and biochemical changes. Lab. Anim. Sci. 47 (6), 598–601.
Johnson, R. G., and Poole, A. R. (1988). Degenerative changes in dog articular cartilage induced by a unilateral tibial valgus osteotomy. Exp. Pathol. 33 (3), 145–164. doi:10.1016/s0232-1513(88)80061-6
Johnson, W. L., Jindrich, D. L., Roy, R. R., and Reggie Edgerton, V. (2008). A three-dimensional model of the rat hindlimb: musculoskeletal geometry and muscle moment arms. J. Biomech. 41 (3), 610–619. doi:10.1016/j.jbiomech.2007.10.004
Kahn, D., Mittelstaedt, D., Matyas, J., Qu, X., Lee, J. H., Badar, F., et al. (2016). Meniscus induced cartilaginous damage and non-linear gross anatomical progression of early-stage osteoarthritis in a canine model. Open Orthop. J. 10, 690–705. doi:10.2174/1874325001610010690
Kamekura, S., Hoshi, K., Shimoaka, T., Chung, U., Chikuda, H., Yamada, T., et al. (2005). Osteoarthritis development in novel experimental mouse models induced by knee joint instability. Osteoarthr. Cartil. 13 (7), 632–641. doi:10.1016/j.joca.2005.03.004
Kean, C., Hinman, R., Bowles, K., and Bennell, K. (2011). “22 ABILITY OF PEAK KNEE ADDUCTION MOMENT AND KNEE ADDUCTION MOMENT IMPULSE TO DISTINGUISH BETWEEN CLINICAL AND STRUCTURAL DISEASE SEVERITY,” in Osteoarthritis and cartilage. (UK).
Kernozek, T., Torry, M., Shelburne, K., Durall, C. J., and Willson, J. (2013). From the gait laboratory to the rehabilitation clinic: translation of motion analysis and modeling data to interventions that impact anterior cruciate ligament loads in gait and drop landing. Crit. Rev. Biomed. Eng. 41 (3), 243–258. doi:10.1615/critrevbiomedeng.2014010676
Khorasani, M. S., Diko, S., Hsia, A. W., Anderson, M. J., Genetos, D. C., Haudenschild, D. R., et al. (2015). Effect of alendronate on post-traumatic osteoarthritis induced by anterior cruciate ligament rupture in mice. Arthritis Res. Ther. 17, 30. doi:10.1186/s13075-015-0546-0
Kimmerling, K. A., Furman, B. D., Mangiapani, D. S., Moverman, M. A., Sinclair, S. M., Huebner, J. L., et al. (2015). Sustained intra-articular delivery of il-1ra from a thermally-responsive elastin-like polypeptide as a therapy for post-traumatic arthritis. Eur. Cells Mater. 29, 124–140. doi:10.22203/eCM.v029a10
Kloppenburg, M., and Berenbaum, F. (2020). Osteoarthritis year in review 2019: epidemiology and therapy. Osteoarthr. Cartil. 28 (3), 242–248. doi:10.1016/j.joca.2020.01.002
Knapik, D. M., Perera, P., Nam, J., Blazek, A. D., Rath, B., Leblebicioglu, B., et al. (2014). Mechanosignaling in bone health, trauma and inflammation. Antioxidants redox Signal. 20 (6), 970–985. doi:10.1089/ars.2013.5467
Knights, C. B., Gentry, C., and Bevan, S. (2012). Partial medial meniscectomy produces osteoarthritis pain-related behaviour in female C57BL/6 mice. Pain 153 (2), 281–292. doi:10.1016/j.pain.2011.09.007
Knoop, J., Steultjens, M. P., van der Leeden, M., van der Esch, M., Thorstensson, C. A., Roorda, L. D., et al. (2011). Proprioception in knee osteoarthritis: a narrative review. Osteoarthr. Cartil. 19 (4), 381–388. doi:10.1016/j.joca.2011.01.003
Ko, F. C., Dragomir, C., Plumb, D. A., Goldring, S. R., Wright, T. M., Goldring, M. B., et al. (2013). In vivo cyclic compression causes cartilage degeneration and subchondral bone changes in mouse tibiae. Arthritis Rheum. 65 (6), 1569–1578. doi:10.1002/art.37906
Korotkyi, O., Vovk, A., Blokhina, O., Dvorshchenko, K., Falalyeyeva, T., Abenavoli, L., et al. (2019). Effect of chondroitin sulfate on blood serum cytokine profile during carrageenan-induced edema and monoiodoacetate-induced osteoarthritis in rats. Rev. Recent Clin. Trials 14 (1), 50–55. doi:10.2174/1574887113666181102111247
Kraus, V. B., Blanco, F. J., Englund, M., Karsdal, M. A., and Lohmander, L. S. (2015). Call for standardized definitions of osteoarthritis and risk stratification for clinical trials and clinical use. Osteoarthr. Cartil. 23 (8), 1233–1241. doi:10.1016/j.joca.2015.03.036
Kraus, V. B., Huebner, J. L., DeGroot, J., and Bendele, A. (2010). The OARSI histopathology initiative - recommendations for histological assessments of osteoarthritis in the Guinea pig. Osteoarthr. Cartil. 18 (Suppl. 3), S35–S52. doi:10.1016/j.joca.2010.04.015
Kreipke, T. C., Rivera, N. C., Garrison, J. G., Easley, J. T., Turner, A. S., and Niebur, G. L. (2014). Alterations in trabecular bone microarchitecture in the ovine spine and distal femur following ovariectomy. J. Biomech. 47 (8), 1918–1921. doi:10.1016/j.jbiomech.2014.03.025
Krishnan, V., Bryant, H. U., and MacDougald, O. A. (2006). Regulation of bone mass by Wnt signaling. J. Clin. investigation 116 (5), 1202–1209. doi:10.1172/jci28551
Kujala, U. M., Kaprio, J., and Sarna, S. (1994). Osteoarthritis of weight bearing joints of lower limbs in former elite male athletes. BMJ 308 (6923), 231–234. doi:10.1136/bmj.308.6923.231
Kuyinu, E. L., Narayanan, G., Nair, L. S., and Laurencin, C. T. (2016). Animal models of osteoarthritis: classification, update, and measurement of outcomes. J. Orthop. Surg. Res. 11, 19. doi:10.1186/s13018-016-0346-5
Kyostio-Moore, S., Nambiar, B., Hutto, E., Ewing, P. J., Piraino, S., Berthelette, P., et al. (2011). STR/ort mice, a model for spontaneous osteoarthritis, exhibit elevated levels of both local and systemic inflammatory markers. Comp. Med. 61 (4), 346–355.
Lahm, A., Uhl, M., Edlich, M., Erggelet, C., Haberstroh, J., and Kreuz, P. C. (2005). An experimental canine model for subchondral lesions of the knee joint. Knee 12 (1), 51–55. doi:10.1016/j.knee.2004.01.005
Lakes, E. H., and Allen, K. D. (2016). Gait analysis methods for rodent models of arthritic disorders: reviews and recommendations. Osteoarthr. Cartil. 24 (11), 1837–1849. doi:10.1016/j.joca.2016.03.008
Lampropoulou-Adamidou, K., Lelovas, P., Karadimas, E. V., Liakou, C., Triantafillopoulos, I. K., Dontas, I., et al. (2014). Useful animal models for the research of osteoarthritis. Eur. J. Orthop. Surg. Traumatol. 24 (3), 263–271. doi:10.1007/s00590-013-1205-2
Lapvetelainen, T., Hyttinen, M. M., Saamanen, A. M., Langsjo, T., Sahlman, J., Felszeghy, S., et al. (2002). Lifelong voluntary joint loading increases osteoarthritis in mice housing a deletion mutation in type II procollagen gene, and slightly also in non-transgenic mice. Ann. Rheumatic Dis. 61(9), 810–817. doi:10.1136/ard.61.9.810
Lapveteläinen, T., Nevalainen, T., Parkkinen, J., Arokoski, J., Kiraly, K., Hyttinen, M., et al. (1995). Lifelong moderate running training increases the incidence and severity of osteoarthritis in the knee joint of C57BL mice. Anatomical Rec. 242 (2), 159–165. doi:10.1002/ar.1092420204
Lapvetelainen, T., Nevalainen, T., Parkkinen, J. J., Arokoski, J., Kiraly, K., Hyttinen, M., et al. (1995). Lifelong moderate running training increases the incidence and severity of osteoarthritis in the knee-joint of C57bl mice. Anat. Rec. 242(2), 159–165. doi:10.1002/ar.1092420204
Laurent, D., Wasvary, J., Rudin, M., O’Byrne, E., and Pellas, T. (2003). In vivo assessment of macromolecular content in articular cartilage of the goat knee. Magn. Reson Med. 49 (6), 1037–1046. doi:10.1002/mrm.10466
Lavigne, P., Benderdour, M., Lajeunesse, D., Reboul, P., Shi, Q., Pelletier, J. P., et al. (2005). Subchondral and trabecular bone metabolism regulation in canine experimental knee osteoarthritis. Osteoarthr. Cartil. 13 (4), 310–317. doi:10.1016/j.joca.2004.12.015
Lee, K. M., Prasad, V., Achuthan, A., Fleetwood, A. J., Hamilton, J. A., and Cook, A. D. (2020). Targeting GM-CSF for collagenase-induced osteoarthritis pain and disease in mice. Osteoarthr. Cartil. 28 (4), 486–491. doi:10.1016/j.joca.2020.01.012
Legrand, C. B., Lambert, C. J., Comblain, F. V., Sanchez, C., and Henrotin, Y. E. (2017). Review of soluble biomarkers of osteoarthritis: lessons from animal models. Cartilage 8 (3), 211–233. doi:10.1177/1947603516656739
Lespasio, M. J., Piuzzi, N. S., Husni, M. E., Muschler, G. F., Guarino, A., and Mont, M. A. (2017). Knee osteoarthritis: a primer. Perm. J. 21, 16–183. doi:10.7812/TPP/16-183
Lewis, J. S., Hembree, W. C., Furman, B. D., Tippets, L., Cattel, D., Huebner, J. L., et al. (2011). Acute joint pathology and synovial inflammation is associated with increased intra-articular fracture severity in the mouse knee. Osteoarthr. Cartil. 19 (7), 864–873. doi:10.1016/j.joca.2011.04.011
Li, G. Y., Yin, J. M., Gao, J. J., Cheng, T. S., Pavlos, N. J., Zhang, C. Q., et al. (2013). Subchondral bone in osteoarthritis: insight into risk factors and microstructural changes. Arthritis Res. Ther. 15 (6), 223. doi:10.1186/ar4405
Libicher, M., Ivancic, M., Hoffmann, V., and Wenz, W. (2005). Early changes in experimental osteoarthritis using the Pond-Nuki dog model: technical procedure and initial results of in vivo MR imaging. Eur. Radiol. 15 (2), 390–394. doi:10.1007/s00330-004-2486-y
Lim, N., Wen, C., and Vincent, T. (2020). Molecular and structural imaging in surgically induced murine osteoarthritis. Osteoarthr. Cartil. 28 (7), 874–884. doi:10.1016/j.joca.2020.03.016
Lim, N. H., Vincent, T. L., and Nissim, A. (2015). In vivo optical imaging of early osteoarthritis using an antibody specific to damaged arthritic cartilage. Arthritis Res. Ther. 17, 376–410. doi:10.1186/s13075-015-0898-5
Link, T. M., Neumann, J., and Li, X. J. (2017). Prestructural cartilage assessment using MRI. J. Magnetic Reson. Imaging 45 (4), 949–965. doi:10.1002/jmri.25554
Little, C. B., and Hunter, D. J. (2013). Post-traumatic osteoarthritis: from mouse models to clinical trials. Nat. Rev. Rheumatol. 9 (8), 485–497. doi:10.1038/nrrheum.2013.72
Little, C. B., and Zaki, S. (2012). What constitutes an "animal model of osteoarthritis"--the need for consensus? Osteoarthr. Cartil. 20 (4), 261–267. doi:10.1016/j.joca.2012.01.017
Lockwood, K. A., Chu, B. T., Anderson, M. J., Haudenschild, D. R., and Christiansen, B. A. (2014). Comparison of loading rate-dependent injury modes in a murine model of post-traumatic osteoarthritis. J. Orthop. Res. 32 (1), 79–88. doi:10.1002/jor.22480
Lotz, M. K., and Kraus, V. B. (2010). New developments in osteoarthritis. Posttraumatic osteoarthritis: pathogenesis and pharmacological treatment options. Arthritis Res. Ther. 12 (3), 211. doi:10.1186/ar3046
Ma, Y., Yao, Y., Zhong, N., Angwa, L. M., and Pei, J. (2020). The dose-time effects of fluoride on the expression and DNA methylation level of the promoter region of BMP-2 and BMP-7 in rats. Environ. Toxicol. Pharmacol. 75, 103331. doi:10.1016/j.etap.2020.103331
Maerz, T., Kurdziel, M. D., Davidson, A. A., Baker, K. C., Anderson, K., and Matthew, H. W. (2015). Biomechanical characterization of a model of noninvasive, traumatic anterior cruciate ligament injury in the rat. Ann. Biomed. Eng. 43 (10), 2467–2476. doi:10.1007/s10439-015-1292-9
Maly, M. R., Acker, S. M., Totterman, S., Tamez-Pena, J., Stratford, P. W., Callaghan, J. P., et al. (2015). Knee adduction moment relates to medial femoral and tibial cartilage morphology in clinical knee osteoarthritis. J. Biomech. 48 (12), 3495–3501. doi:10.1016/j.jbiomech.2015.04.039
Mankin, H. J., Johnson, M. E., and Lippiello, L. (1981). Biochemical and metabolic abnormalities in articular cartilage from osteoarthritic human hips. III. Distribution and metabolism of amino sugar-containing macromolecules. J. Bone Jt. Surg. Am. 63 (1), 131–139. doi:10.2106/00004623-198163010-00017
Mason, R. M., Chambers, M. G., Flannelly, J., Gaffen, J. D., Dudhia, J., and Bayliss, M. T. (2001). The STR/ort mouse and its use as a model of osteoarthritis. Osteoarthr. Cartil. 9(2), 85–91. doi:10.1053/joca.2000.0363
Masouros, S., McDermott, I., Amis, A., and Bull, A. (2008). Biomechanics of the meniscus-meniscal ligament construct of the knee. Knee Surg. sports Traumatol. Arthrosc. 16 (12), 1121–1132. doi:10.1007/s00167-008-0616-9
Mastbergen, S. C., Marijnissen, A. C., Vianen, M. E., van Roermund, P. M., Bijlsma, J. W., and Lafeber, F. P. (2006). The canine ’groove' model of osteoarthritis is more than simply the expression of surgically applied damage. Osteoarthr. Cartil. 14 (1), 39–46. doi:10.1016/j.joca.2004.07.009
McCoy, A. M. (2015). Animal models of osteoarthritis: comparisons and key considerations. Veterinary Pathol. 52 (5), 803–818. doi:10.1177/0300985815588611
McDougall, J. J., Schuelert, N., and Bowyer, J. (2010). Cathepsin K inhibition reduces CTXII levels and joint pain in the Guinea pig model of spontaneous osteoarthritis. Osteoarthr. Cartil. 18 (10), 1355–1357. doi:10.1016/j.joca.2010.07.014
McErlain, D. D., Milner, J. S., Ivanov, T. G., Jencikova-Celerin, L., Pollmann, S. I., and Holdsworth, D. W. (2011). Subchondral cysts create increased intra-osseous stress in early knee OA: a finite element analysis using simulated lesions. Bone 48 (3), 639–646. doi:10.1016/j.bone.2010.11.010
McErlain, D. D., Ulici, V., Darling, M., Gati, J. S., Pitelka, V., Beier, F., et al. (2012). An in vivo investigation of the initiation and progression of subchondral cysts in a rodent model of secondary osteoarthritis. Arthritis Res. Ther. 14 (1), R26. ARTN R26. doi:10.1186/ar3727
McIlwraith, C. W., Frisbie, D. D., and Kawcak, C. E. (2012). The horse as a model of naturally occurring osteoarthritis. Bone & Jt. Res. 1 (11), 297–309. doi:10.1302/2046-3758.111.2000132
McNulty, M. A., Loeser, R. F., Davey, C., Callahan, M. F., Ferguson, C. M., and Carlson, C. S. (2012). Histopathology of naturally occurring and surgically induced osteoarthritis in mice. Osteoarthr. Cartil. 20 (8), 949–956. doi:10.1016/j.joca.2012.05.001
Meeson, R. L., Todhunter, R. J., Blunn, G., Nuki, G., and Pitsillides, A. A. (2019a). Spontaneous dog osteoarthritis - a One Medicine vision. Nat. Rev. Rheumatol. 15 (5), 273–287. doi:10.1038/s41584-019-0202-1
Meeson, R. L., Todhunter, R. J., Blunn, G., Nuki, G., and Pitsillides, A. A. (2019b). Spontaneous dog osteoarthritis — a One Medicine vision. Nat. Rev. Rheumatol. 15 (5), 273–287. doi:10.1038/s41584-019-0202-1
Melville, K. M., Robling, A. G., and van der Meulen, M. C. (2015). In vivo axial loading of the mouse tibia. Methods Mol. Biol. 1226, 99–115. doi:10.1007/978-1-4939-1619-1_9
Meulenbelt, I., Kloppenburg, M., Kroon, H. M., Houwing-Duistermaat, J. J., Garnero, P., Le Graverand, M. P. H., et al. (2006). Urinary CTX-II levels are associated with radiographic subtypes of osteoarthritis in hip, knee, hand, and facet joints in subject with familial osteoarthritis at multiple sites: the GARP study. Ann. Rheumatic Dis. 65 (3), 360–365. doi:10.1136/ard.2005.040642
Miller, R. E., Lu, Y. Z., Tortorella, M. D., and Malfait, A. M. (2013). Genetically engineered mouse models reveal the importance of proteases as osteoarthritis drug targets. Curr. Rheumatol. Rep. 15 (8), 350. doi:10.1007/s11926-013-0350-2
Mobasheri, A., Saarakkala, S., Finnilä, M., Karsdal, M. A., Bay-Jensen, A.-C., and van Spil, W. E. (2019). Recent advances in understanding the phenotypes of osteoarthritis. F1000Research 8, 2091. F1000 Faculty Rev-2091. doi:10.12688/f1000research.20575.1
Murphy, G., and Lee, M. (2005). What are the roles of metalloproteinases in cartilage and bone damage? Ann. rheumatic Dis. 64 (Suppl. 4), iv44–iv47. doi:10.1136/ard.2005.042465
Neogi, T., Bowes, M. A., Niu, J., De Souza, K. M., Vincent, G. R., Goggins, J., et al. (2013). Magnetic resonance imaging-based three-dimensional bone shape of the knee predicts onset of knee osteoarthritis: data from the osteoarthritis initiative. Arthritis Rheum. 65 (8), 2048–2058. doi:10.1002/art.37987
Oestergaard, S., Sondergaard, B. C., Hoegh-Andersen, P., Henriksen, K., Qvist, P., Christiansen, C., et al. (2006). Effects of ovariectomy and estrogen therapy on type II collagen degradation and structural integrity of articular cartilage in rats - implications of the time of initiation. Arthritis Rheumatism 54 (8), 2441–2451. doi:10.1002/art.22009
Olive, L., Gad, S., Fining, M., Thomas, L., and Berteau, J. P. (2017). Tissue and movement biomechanical characterization of osteoarthritis progression in mouse knee joint. Comput. Methods Biomechanics Biomed. Eng. 20 (Suppl. 1), S153–S154. doi:10.1080/10255842.2017.1382907
Orhan, C., Juturu, V., Sahin, E., Tuzcu, M., Ozercan, I. H., Durmus, A. S., et al. (2021). Undenatured type II collagen ameliorates inflammatory responses and articular cartilage damage in the rat model of osteoarthritis. Front. Vet. Sci. 8, 617789. doi:10.3389/fvets.2021.617789
Palazzo, C., Nguyen, C., Lefevre-Colau, M. M., Rannou, F., and Poiraudeau, S. (2016). Risk factors and burden of osteoarthritis. Ann. Phys. Rehabil. Med. 59 (3), 134–138. doi:10.1016/j.rehab.2016.01.006
Palmieri-Smith, R. M., Thomas, A. C., Karvonen-Gutierrez, C., and Sowers, M. F. (2010). Isometric quadriceps strength in women with mild, moderate, and severe knee osteoarthritis. Am. J. Phys. Med. Rehabilitation 89 (7), 541–548. doi:10.1097/PHM.0b013e3181ddd5c3
Panula, H. E., Helminen, H. J., and Kiviranta, I. (1997). Slowly progressive osteoarthritis after tibial valgus osteotomy in young beagle dogs. Clin. Orthop. Relat. Res. 343, 192–202. doi:10.1097/00003086-199710000-00030
Parra-Torres, N. M., Cazares-Raga, F. E., and Kouri, J. B. (2014). Proteomic analysis of rat cartilage: the identification of differentially expressed proteins in the early stages of osteoarthritis. Proteome Sci. 12 (1), 55. doi:10.1186/s12953-014-0055-0
Pedoia, V., Haefeli, J., Morioka, K., Teng, H. L., Nardo, L., Souza, R. B., et al. (2017). TOPOLOGICAL DATA ANALYSIS TO PREDICT CARTILAGE LESION PROGRESSION IN KNEE OSTEOARTHRITIS. Osteoarthr. Cartil. 25, S240–S241. doi:10.1016/j.joca.2017.02.407
Pereira, J. E., Cabrita, A. M., Filipe, V. M., Bulas-Cruz, J., Couto, P. A., Melo-Pinto, P., et al. (2006). A comparison analysis of hindlimb kinematics during overground and treadmill locomotion in rats. Behav. Brain Res. 172 (2), 212–218. doi:10.1016/j.bbr.2006.04.027
Piskin, A., Gulbahar, M. Y., Tomak, Y., Gulman, B., Hokelek, M., Kerimoglu, S., et al. (2007). Osteoarthritis models after anterior cruciate ligament resection and medial meniscectomy in rats. A histological and immunohistochemical study. Saudi Med. J. 28 (12), 1796–1802.
Pitcher, T., Sousa-Valente, J., and Malcangio, M. (2016). The monoiodoacetate model of osteoarthritis pain in the mouse. J. Vis. Exp. 111, 53746. doi:10.3791/53746
Poulet, B. (2016). Non-invasive loading model of murine osteoarthritis. Curr. Rheumatol. Rep. 18 (7), 40. doi:10.1007/s11926-016-0590-z
Poulet, B., Hamilton, R. W., Shefelbine, S., and Pitsillides, A. A. (2011). Characterizing a novel and adjustable noninvasive murine joint loading model. Arthritis Rheum. 63 (1), 137–147. doi:10.1002/art.27765
Poulet, B., Ulici, V., Stone, T. C., Pead, M., Gburcik, V., Constantinou, E., et al. (2012). Time-series transcriptional profiling yields new perspectives on susceptibility to murine osteoarthritis. Arthritis & Rheumatism 64 (10), 3256–3266. doi:10.1002/art.34572
Poulet, B., Westerhof, T. A. T., Hamilton, R. W., Shefelbine, S. J., and Pitsillides, A. A. (2013). Spontaneous osteoarthritis in Str/ort mice is unlikely due to greater vulnerability to mechanical trauma. Osteoarthr. Cartil. 21 (5), 756–763. doi:10.1016/j.joca.2013.02.652
Preiswerk, F., Sury, M. S., Wortman, J. R., Neumann, G., Wells, W., and Duryea, J. (2022). Fast quantitative bone marrow lesion measurement on knee MRI for the assessment of osteoarthritis. Osteoarthr. Cartil. Open 4 (1), 100234. doi:10.1016/j.ocarto.2022.100234
Pucha, K. A., McKinney, J. M., Fuller, J. M., and Willett, N. J. (2020). Characterization of OA development between sexes in the rat medial meniscal transection model. Osteoarthr. Cartil. Open 2 (3), 100066. doi:10.1016/j.ocarto.2020.100066
Qin, Y., He, J., Xia, L., Guo, H., and He, C. (2013). Effects of electro-acupuncture on oestrogen levels, body weight, articular cartilage histology and MMP-13 expression in ovariectomised rabbits. Acupunct. Med. 31 (2), 214–221. doi:10.1136/acupmed-2012-010289
Radin, E. L., Burr, D. B., Caterson, B., Fyhrie, D., Brown, T. D., and Boyd, R. D. (1991). Mechanical determinants of osteoarthrosis. Semin. Arthritis Rheum. 21 (3 Suppl. 2), 12–21. doi:10.1016/0049-0172(91)90036-y
Ramme, A. J., Lendhey, M., Raya, J. G., Kirsch, T., and Kennedy, O. D. (2016). A novel rat model for subchondral microdamage in acute knee injury: a potential mechanism in post-traumatic osteoarthritis. Osteoarthr. Cartil. 24 (10), 1776–1785. doi:10.1016/j.joca.2016.05.017
Ramme, A. J., Lendhey, M. S., Strauss, E. J., and Kennedy, O. D. (2018). A biomechanical study of two distinct methods of anterior cruciate ligament rupture, and a novel surgical reconstruction technique, in a small animal model of posttraumatic osteoarthritis. J. Knee Surg. 31 (1), 043–049. doi:10.1055/s-0037-1600088
Ramme, A. J., Voss, K., Lesporis, J., Lendhey, M. S., Coughlin, T. R., Strauss, E. J., et al. (2017). Automated bone segmentation and surface evaluation of a small animal model of post-traumatic osteoarthritis. Ann. Biomed. Eng. 45 (5), 1227–1235. doi:10.1007/s10439-017-1799-3
Reimann, I. (1973). Experimental osteoarthritis of the knee in rabbits induced by alteration of the load-bearing. Acta Orthop. Scand. 44 (4), 496–504. doi:10.3109/17453677308989085
Rice, S. J., Beier, F., Young, D. A., and Loughlin, J. (2020). Interplay between genetics and epigenetics in osteoarthritis. Nat. Rev. Rheumatol. 16 (5), 268–281. doi:10.1038/s41584-020-0407-3
Richmond, S. A., Fukuchi, R. K., Ezzat, A., Schneider, K., Schneider, G., and Emery, C. A. (2013). Are joint injury, sport activity, physical activity, obesity, or occupational activities predictors for osteoarthritis? A systematic review. J. Orthop. Sports Phys. Ther. 43 (8), 515–B19. doi:10.2519/jospt.2013.4796
Robbins, S. M., Morelli, M., Martineau, P. A., St-Onge, N., Boily, M., Dimentberg, R., et al. (2019). A comparison of muscle activation and knee mechanics during gait between patients with non-traumatic and post-traumatic knee osteoarthritis. Osteoarthr. Cartil. 27 (7), 1033–1042. doi:10.1016/j.joca.2019.02.798
Robin, D. M. C. (2017). Establishment of a rat model of temporomandibular joint osteoarthritis using intraarticular injection of complete FREUND’S adjuvant. Macau, China: UNEJ e-Proceeding, 190–193.
Robinson, W. H., Lepus, C. M., Wang, Q., Raghu, H., Mao, R., Lindstrom, T. M., et al. (2016). Low-grade inflammation as a key mediator of the pathogenesis of osteoarthritis. Nat. Rev. Rheumatol. 12 (10), 580–592. doi:10.1038/nrrheum.2016.136
Roemer, F. W., Eckstein, F., Hayashi, D., and Guermazi, A. (2014). The role of imaging in osteoarthritis. Best. Pract. Res. Clin. Rheumatol. 28 (1), 31–60. doi:10.1016/j.berh.2014.02.002
Roemer, F. W., Guermazi, A., Niu, J., Zhang, Y., Mohr, A., and Felson, D. T. (2012). Prevalence of magnetic resonance imaging-defined atrophic and hypertrophic phenotypes of knee osteoarthritis in a population-based cohort. Arthritis Rheum. 64 (2), 429–437. doi:10.1002/art.33344
Roemer, F. W., Kwoh, C. K., Hannon, M. J., Hunter, D. J., Eckstein, F., Fujii, T., et al. (2015). What comes first? Multitissue involvement leading to radiographic osteoarthritis: magnetic resonance imaging-based trajectory analysis over four years in the osteoarthritis initiative. Arthritis Rheumatol. 67 (8), 2085–2096. doi:10.1002/art.39176
Roos, E. M., Herzog, W., Block, J. A., and Bennell, K. L. (2011). Muscle weakness, afferent sensory dysfunction and exercise in knee osteoarthritis. Nat. Rev. Rheumatol. 7 (1), 57–63. doi:10.1038/nrrheum.2010.195
Rousseau, J.-C., and Delmas, P. D. (2007). Biological markers in osteoarthritis. Nat. Clin. Pract. Rheumatol. 3 (6), 346–356. doi:10.1038/ncprheum0508
Rundell, S. A., Baars, D. C., Phillips, D. M., and Haut, R. C. (2005). The limitation of acute necrosis in retro-patellar cartilage after a severe blunt impact to the in vivo rabbit patello-femoral joint. J. Orthop. Res. 23 (6), 1363–1369. doi:10.1016/j.orthres.2005.06.001.1100230618
Rutgers, M., van Pelt, M. J., Dhert, W. J., Creemers, L. B., and Saris, D. B. (2010). Evaluation of histological scoring systems for tissue-engineered, repaired and osteoarthritic cartilage. Osteoarthr. Cartil. 18 (1), 12–23. doi:10.1016/j.joca.2009.08.009
Sagawa, Y., Armand, S., Lubbeke, A., Hoffmeyer, P., Fritschy, D., Suva, D., et al. (2013). Associations between gait and clinical parameters in patients with severe knee osteoarthritis: a multiple correspondence analysis. Clin. Biomech. 28 (3), 299–305. doi:10.1016/j.clinbiomech.2013.01.008
Saïed, A., Chérin, E., Gaucher, H., Laugier, P., Gillet, P., Floquet, J., et al. (2009). Assessment of articular cartilage and subchondral bone: subtle and progressive changes in experimental osteoarthritis using 50 MHz echography in vitro. J. Bone Mineral Res. 12 (9), 1378–1386. doi:10.1359/jbmr.1997.12.9.1378
Salazar-Noratto, G. E., De Nijs, N., Stevens, Y., Gibson, G., and Guldberg, R. E. (2019). Regional gene expression analysis of multiple tissues in an experimental animal model of post-traumatic osteoarthritis. Osteoarthr. Cartil. 27 (2), 294–303. doi:10.1016/j.joca.2018.10.007
Sandell, L. J., and Aigner, T. (2001). Articular cartilage and changes in Arthritis: cell biology of osteoarthritis. Arthritis Res. 3 (2), 107–113. doi:10.1186/ar148
Sander, K., Layher, F., Anders, C., Roth, A., Babisch, J., Scholle, H. C., et al. (2012). Gait analysis after minimally invasive total hip arthroplasty. Orthopade 41(5), 365–376. doi:10.1007/s00132-011-1891-6
Saxby, D. J., and Lloyd, D. G. (2017). Osteoarthritis year in review 2016: mechanics. Osteoarthr. Cartil. 25 (2), 190–198. doi:10.1016/j.joca.2016.09.023
Schenker, M. L., Mauck, R. L., Ahn, J., and Mehta, S. (2014). Pathogenesis and prevention of posttraumatic osteoarthritis after intra-articular fracture. J. Am. Acad. Orthop. Surg. 22 (1), 20–28. doi:10.5435/Jaaos-22-01-20
Schipplein, O. D., and Andriacchi, T. P. (1991). Interaction between active and passive knee stabilizers during level walking. J. Orthop. Res. 9 (1), 113–119. doi:10.1002/jor.1100090114
Serra, C. I., and Soler, C. (2019). Animal models of osteoarthritis in small mammals. Vet. Clin. North Am. Exot. Anim. Pract. 22 (2), 211–221. doi:10.1016/j.cvex.2019.01.004
Serra, C. I., Soler, C., Carrillo, J. M., Sopena, J. J., Redondo, J. I., and Cugat, R. (2014). Erratum to: effect of autologous platelet-rich plasma on the repair of full-thickness articular defects in rabbits. Knee Surg. Sports Traumatol. Arthrosc. 22 (7), 1710. doi:10.1007/s00167-013-2802-7
Sethi, G., Aljawadi, A., Elmajee, M., and Pillai, A. (2020). Determination of the postoperative limb alignment following a high tibial osteotomy in patients with uni-compartmental knee osteoarthritis, review article. J. Orthop. 18, 53–57. doi:10.1016/j.jor.2019.12.006
Sharma, L., Song, J., Felson, D. T., Cahue, S., Shamiyeh, E., and Dunlop, D. D. (2001). The role of knee alignment in disease progression and functional decline in knee osteoarthritis. JAMA 286 (2), 188–195. doi:10.1001/jama.286.2.188
Shinmei, M., Ito, K., Matsuyama, S., Yoshihara, Y., and Matsuzawa, K. (1993). Joint fluid carboxy-terminal type II procollagen peptide as a marker of cartilage collagen biosynthesis. Osteoarthr. Cartil. 1 (2), 121–128. doi:10.1016/s1063-4584(05)80027-5
Shoji, H., and Insall, J. (1973). High tibial osteotomy for osteoarthritis of the knee with valgus deformity. J. Bone Jt. Surg. Am. 55 (5), 963–973. doi:10.2106/00004623-197355050-00005
Sieker, J. T., Proffen, B. L., Waller, K. A., Chin, K. E., Karamchedu, N. P., Akelman, M. R., et al. (2018). Transcriptional profiling of articular cartilage in a porcine model of early post-traumatic osteoarthritis. J. Orthop. Research® 36 (1), 318–329. doi:10.1002/jor.23644
Singh, V., Oliashirazi, A., Tan, T., Fayyad, A., and Shahi, A. (2019). Clinical and pathophysiologic significance of MRI identified bone marrow lesions associated with knee osteoarthritis. Archives Bone Jt. Surg. 7 (3), 211–219.
Slemenda, C., Brandt, K. D., Heilman, D. K., Mazzuca, S., Braunstein, E. M., Katz, B. P., et al. (1997). Quadriceps weakness and osteoarthritis of the knee. Ann. Intern. Med. 127(2), 97. doi:10.7326/0003-4819-127-2-199707150-00001
Smith, J. O., Wilson, A. J., and Thomas, N. P. (2013). Osteotomy around the knee: evolution, principles and results. Knee Surg. Sports Traumatol. Arthrosc. 21 (1), 3–22. doi:10.1007/s00167-012-2206-0
Sniekers, Y. H., Weinans, H., van Osch, G. J. V. M., and van Leeuwen, J. P. T. M. (2010). Oestrogen is important for maintenance of cartilage and subchondral bone in a murine model of knee osteoarthritis. Arthritis Res. Ther. 12 (5), R182. doi:10.1186/ar3148
Sokolove, J., and Lepus, C. M. (2013). Role of inflammation in the pathogenesis of osteoarthritis: latest findings and interpretations. Ther. Adv. Musculoskelet. Dis. 5 (2), 77–94. doi:10.1177/1759720X12467868
Soul, J., Barter, M. J., Little, C. B., and Young, D. A. (2020). OATargets: a knowledge base of genes associated with osteoarthritis joint damage in animals. Ann. Rheum. Dis. 80, 376–383. doi:10.1136/annrheumdis-2020-218344
Soul, J., Barter, M. J., Little, C. B., and Young, D. A. (2021). OATargets: a knowledge base of genes associated with osteoarthritis joint damage in animals. Ann. Rheum. Dis. 80 (3), 376–383. doi:10.1136/annrheumdis-2020-218344
Staines, K. A., Poulet, B., Wentworth, D. N., and Pitsillides, A. A. (2017a). The STR/ort mouse model of spontaneous osteoarthritis - an update. Osteoarthr. Cartil. 25 (6), 802–808. doi:10.1016/j.joca.2016.12.014
Staines, K. A., Poulet, B., Wentworth, D. N., and Pitsillides, A. A. (2017b). The STR/ort mouse model of spontaneous osteoarthritis - an update. Osteoarthr. Cartil. 25 (6), 802–808. doi:10.1016/j.joca.2016.12.014
Stewart, R. C., Bansal, P. N., Entezari, V., Lusic, H., Nazarian, R. M., Snyder, B. D., et al. (2013). Contrast-enhanced CT with a high-affinity cationic contrast agent for imaging ex vivo bovine, intact ex vivo rabbit, and in vivo rabbit cartilage. Radiology 266 (1), 141–150. doi:10.1148/radiol.12112246
Stürmer, T., Brenner, H., Koenig, W., and Günther, K. P. (2004). Severity and extent of osteoarthritis and low grade systemic inflammation as assessed by high sensitivity C reactive protein. Ann. Rheum. Dis. 63 (2), 200–205. doi:10.1136/ard.2003.007674
Sukur, E., Talu, C., Akman, Y. E., Circi, E., Ozturkmen, Y., and Tuzuner, T. (2016). Comparison of the chondroprotective effect of a novel hydrogel compound and traditional hyaluronate on rat cartilage in a papain-induced osteoarthritis model. Acta Orthop. Traumatol. Turc 50 (4), 458–463. doi:10.1016/j.aott.2016.07.008
Swain, S., Sarmanova, A., Mallen, C., Kuo, C. F., Coupland, C., Doherty, M., et al. (2020). Trends in incidence and prevalence of osteoarthritis in the United Kingdom: findings from the clinical practice research datalink (CPRD). Osteoarthr. Cartil. 28 (6), 792–801. doi:10.1016/j.joca.2020.03.004
Swärd, P., Kostogiannis, I., Neuman, P., Von Porat, A., Boegård, T., and Roos, H. (2010). Differences in the radiological characteristics between post-traumatic and non-traumatic knee osteoarthritis. Scand. J. Med. Sci. Sports 20 (5), 731–739. doi:10.1111/j.1600-0838.2009.01000.x
Tanamas, S., Hanna, F. S., Cicuttini, F. M., Wluka, A. E., Berry, P., and Urquhart, D. M. (2009). Does knee malalignment increase the risk of development and progression of knee osteoarthritis? A systematic review. Arthritis Rheum. 61 (4), 459–467. doi:10.1002/art.24336
Tchetverikov, I., Lohmander, L. S., Verzijl, N., Huizinga, T. W., TeKoppele, J. M., Hanemaaijer, R., et al. (2005). MMP protein and activity levels in synovial fluid from patients with joint injury, inflammatory arthritis, and osteoarthritis. Ann. Rheum. Dis. 64 (5), 694–698. doi:10.1136/ard.2004.022434
Teeple, E., Jay, G. D., Elsaid, K. A., and Fleming, B. C. (2013). Animal models of osteoarthritis: challenges of model selection and analysis. AAPS J. 15 (2), 438–446. doi:10.1208/s12248-013-9454-x
Temp, J., Labuz, D., Negrete, R., Sunkara, V., and Machelska, H. (2020). Pain and knee damage in male and female mice in the medial meniscal transection-induced osteoarthritis. Osteoarthr. Cartil. 28 (4), 475–485. doi:10.1016/j.joca.2019.11.003
Tetsworth, K., and Paley, D. (1994). Malalignment and degenerative arthropathy. Orthop. Clin. North Am. 25 (3), 367–377. doi:10.1016/s0030-5898(20)31921-0
Thelin, N., Holmberg, S., and Thelin, A. (2006). Knee injuries account for the sports-related increased risk of knee osteoarthritis. Scand. J. Med. Sci. Sports 16 (5), 329–333. doi:10.1111/j.1600-0838.2005.00497.x
Thomas, A. C., Hubbard-Turner, T., Wikstrom, E. A., and Palmieri-Smith, R. M. (2017). Epidemiology of posttraumatic osteoarthritis. J. Athl. Train. 52 (6), 491–496. doi:10.4085/1062-6050-51.5.08
Trayhurn, P., and Wood, I. S. (2004). Adipokines: inflammation and the pleiotropic role of white adipose tissue. Br. J. Nutr. 92 (3), 347–355. doi:10.1079/bjn20041213
Tsukada, S., and Wakui, M. (2017). Is overcorrection preferable for repair of degenerated articular cartilage after open-wedge high tibial osteotomy? Knee Surg. Sports Traumatol. Arthrosc. 25 (3), 785–792. doi:10.1007/s00167-015-3655-z
Tveit, M., Rosengren, B. E., Nilsson, J. A., and Karlsson, M. K. (2012). Former male elite athletes have a higher prevalence of osteoarthritis and arthroplasty in the hip and knee than expected. Am. J. Sports Med. 40 (3), 527–533. doi:10.1177/0363546511429278
Umemoto, Y., Oka, T., Inoue, T., and Saito, T. (2010). Imaging of a rat osteoarthritis model using 18 F-fluoride positron emission tomography. Ann. Nucl. Med. 24, 663–669. doi:10.1007/s12149-010-0411-9
Unger, M. D., Murthy, N. S., Kanwar, R., Strand, K. A., Maus, T. P., and Beutler, A. S. (2018). Clinical magnetic resonance-enabled characterization of mono-iodoacetate-induced osteoarthritis in a large animal species. Plos One 13 (8), e0201673. doi:10.1371/journal.pone.0201673
van Tunen, J. A., Dell’Isola, A., Juhl, C., Dekker, J., Steultjens, M., and Lund, H. (2016). Biomechanical factors associated with the development of tibiofemoral knee osteoarthritis: protocol for a systematic review and meta-analysis. BMJ Open 6 (6), e011066. doi:10.1136/bmjopen-2016-011066
van Tunen, J. A. C., Dell’Isola, A., Juhl, C., Dekker, J., Steultjens, M., Thorlund, J. B., et al. (2018). Association of malalignment, muscular dysfunction, proprioception, laxity and abnormal joint loading with tibiofemoral knee osteoarthritis - a systematic review and meta-analysis. Bmc Musculoskelet. Disord. 19, 273. doi:10.1186/s12891-018-2202-8
Versus Arthritis (2019). The state of musculoskeletal health 2019. Chesterfield, UK: Arthritis Care and Arthritis Research UK.
Videman, T. (1982). Experimental osteoarthritis in the rabbit: comparison of different periods of repeated immobilization. Acta Orthop. Scand. 53 (3), 339–347. doi:10.3109/17453678208992226
Vilensky, J. A., O’Connor, B. L., Brandt, K. D., Dunn, E. A., Rogers, P. I., and DeLong, C. A. (1994). Serial kinematic analysis of the unstable knee after transection of the anterior cruciate ligament: temporal and angular changes in a canine model of osteoarthritis. J. Orthop. Res. 12 (2), 229–237. doi:10.1002/jor.1100120212
Vina, E. R., and Kwoh, C. K. (2018). Epidemiology of osteoarthritis: literature update. Curr. Opin. Rheumatol. 30 (2), 160–167. doi:10.1097/BOR.0000000000000479
Vincent, T. L., Williams, R. O., Maciewicz, R., Silman, A., Garside, P., and group, f.t.A. R. U. a.m.w. (2012). Mapping pathogenesis of arthritis through small animal models. Rheumatology 51 (11), 1931–1941. doi:10.1093/rheumatology/kes035
Vrinten, D. H., and Hamers, F. F. T. (2003). CatWalk’ automated quantitative gait analysis as a novel method to assess mechanical allodynia in the rat; a comparison with von Frey testing. Pain 102 (1-2), 203–209. doi:10.1016/S0304-3959(02)00382-2
Wallace, I. J., Worthington, S., Felson, D. T., Jurmain, R. D., Wren, K. T., Maijanen, H., et al. (2017). Knee osteoarthritis has doubled in prevalence since the mid-20th century. Proc. Natl. Acad. Sci. U. S. A. 114 (35), 9332–9336. doi:10.1073/pnas.1703856114
Walsh, M. C., and Choi, Y. (2014). Biology of the RANKL-RANK-OPG system in immunity, bone, and beyond. Front. Immunol. 5, 511. doi:10.3389/fimmu.2014.00511
Wang, N., Badar, F., and Xia, Y. (2018). Compressed sensing in quantitative determination of GAG concentration in cartilage by microscopic MRI. Magn. Reson Med. 79 (6), 3163–3171. doi:10.1002/mrm.26973
Warnberg, J., Cunningham, K., Romeo, J., and Marcos, A. (2010). Physical activity, exercise and low-grade systemic inflammation. Proc. Nutr. Soc. 69 (3), 400–406. doi:10.1017/S0029665110001928
Wei, L., de Bri, E., Lundberg, A., and Svensson, O. (1998). Mechanical load and primary Guinea pig osteoarthrosis. Acta Orthop. Scand. 69 (4), 351–357. doi:10.3109/17453679808999046
Wei, L., Fleming, B. C., Sun, X. J., Teeple, E., Wu, W., Jay, G. D., et al. (2010). Comparison of differential biomarkers of osteoarthritis with and without posttraumatic injury in the Hartley Guinea pig model. J. Orthop. Res. 28 (7), 900–906. doi:10.1002/jor.21093
Wendler, A., and Wehling, M. (2010). The translatability of animal models for clinical development: biomarkers and disease models. Curr. Opin. Pharmacol. 10 (5), 601–606. doi:10.1016/j.coph.2010.05.009
Wolf, A., Dedman, D., Campbell, J., Booth, H., Lunn, D., Chapman, J., et al. (2019). Data resource profile: clinical practice research datalink (CPRD) aurum. Int. J. Epidemiol. 48 (6), 1740–1740g. doi:10.1093/ije/dyz034
Xiao, S., Tang, Y., Lin, Y., Lv, Z., and Chen, L. (2020). Tracking osteoarthritis progress through cationic nanoprobe-enhanced photoacoustic imaging of cartilage. Acta Biomater. 109, 153–162. doi:10.1016/j.actbio.2020.04.001
Xu, X., Li, X., Liang, Y., Ou, Y., Huang, J., Xiong, J., et al. (2019). Estrogen modulates cartilage and subchondral bone remodeling in an ovariectomized rat model of postmenopausal osteoarthritis. Med. Sci. Monit. 25, 3146–3153. doi:10.12659/MSM.916254
Yakhdani, H. R. F., Bafghi, H. A., Meijer, O. G., Bruijn, S. M., van den Dikkenberg, N., Stibbe, A. B., et al. (2010). Stability and variability of knee kinematics during gait in knee osteoarthritis before and after replacement surgery. Clin. Biomech. 25 (3), 230–236. doi:10.1016/j.clinbiomech.2009.12.003
Zanjani-Pour, S., Giorgi, M., and Dall’Ara, E. (2020). Development of subject specific finite element models of the mouse knee joint for preclinical applications. Front. Bioeng. Biotechnol. 8, 558815. doi:10.3389/fbioe.2020.558815
Zeng, X. L., Ma, L. M., Lin, Z. F., Huang, W. H., Huang, Z. Q., Zhang, Y., et al. (2017). Relationship between Kellgren-Lawrence score and 3D kinematic gait analysis of patients with medial knee osteoarthritis using a new gait system. Sci. Rep. 7, 4080. doi:10.1038/s41598-017-04390-5
Zhai, G., Randell, E. W., and Rahman, P. (2018). Metabolomics of osteoarthritis: emerging novel markers and their potential clinical utility. Rheumatology 57 (12), 2087–2095. doi:10.1093/rheumatology/kex497
Zhao, Z., Li, Y., Wang, M., Zhao, S., Zhao, Z., and Fang, J. (2020). Mechanotransduction pathways in the regulation of cartilage chondrocyte homoeostasis. J. Cell. Mol. Med. 24 (10), 5408–5419. doi:10.1111/jcmm.15204
Glossary
Keywords: osteoarthritis, preclinical models of osteoarthritis, biomechanical factors, biomechanically induced models, biomechanical measurement techniques
Citation: Xu L, Kazezian Z, Pitsillides AA and Bull AMJ (2024) A synoptic literature review of animal models for investigating the biomechanics of knee osteoarthritis. Front. Bioeng. Biotechnol. 12:1408015. doi: 10.3389/fbioe.2024.1408015
Received: 27 March 2024; Accepted: 02 July 2024;
Published: 25 July 2024.
Edited by:
Jingwei Zhang, Shanghai Jiao Tong University, ChinaReviewed by:
Yang Li, Shanghai Jiao Tong University, ChinaHuazhang Xiong, Affiliated Hospital of Zunyi Medical University, China
Copyright © 2024 Xu, Kazezian, Pitsillides and Bull. This is an open-access article distributed under the terms of the Creative Commons Attribution License (CC BY). The use, distribution or reproduction in other forums is permitted, provided the original author(s) and the copyright owner(s) are credited and that the original publication in this journal is cited, in accordance with accepted academic practice. No use, distribution or reproduction is permitted which does not comply with these terms.
*Correspondence: Luyang Xu, l.xu20@imperial.ac.uk