- 1Department of Radiology, Ningbo No. 2 Hospital, Ningbo, China
- 2Auckland Bioengineering Institute, The University of Auckland, Auckland, New Zealand
- 3Faculty of Engineering, University of Pannonia, Veszprém, Hungary
- 4Center for Medical Imaging, Faculty of Medical and Health Sciences, The University of Auckland, Auckland, New Zealand
- 5Vehicle Industry Research Center, Széchenyi István University, Győr, Hungary
- 6Faculty of Sports Science, Ningbo University, Ningbo, China
- 7Department of Engineering Science, The University of Auckland, Auckland, New Zealand
This study presents a comprehensive review of the correlation between tibial acceleration (TA), ground reaction forces (GRF), and tibial bone loading, emphasizing the critical role of wearable sensor technology in accurately measuring these biomechanical forces in the context of running. This systematic review and meta-analysis searched various electronic databases (PubMed, SPORTDiscus, Scopus, IEEE Xplore, and ScienceDirect) to identify relevant studies. It critically evaluates existing research on GRF and tibial acceleration (TA) as indicators of running-related injuries, revealing mixed findings. Intriguingly, recent empirical data indicate only a marginal link between GRF, TA, and tibial bone stress, thus challenging the conventional understanding in this field. The study also highlights the limitations of current biomechanical models and methodologies, proposing a paradigm shift towards more holistic and integrated approaches. The study underscores wearable sensors’ potential, enhanced by machine learning, in transforming the monitoring, prevention, and rehabilitation of running-related injuries.
1 Introduction
The external loading generated during locomotion is essential for generating momentum necessary for movements such as propelling, braking, and changing direction. Metrics of ground reaction forces (GRF) are crucial in understanding the biomechanical mechanisms during running (Johnson C. D. et al., 2020). This understanding plays a pivotal role in preventing musculoskeletal injuries and in evaluating rehabilitation processes (Van der Worp et al., 2016; Willwacher et al., 2022; Pan et al., 2023; Yang et al., 2023). Proper analysis and interpretation of these reaction forces can provide invaluable insights into the efficiency and safety of movement, thus informing strategies for injury prevention and the effectiveness of rehabilitation techniques (Zadpoor and Nikooyan, 2011; Johnson C. D. et al., 2020).
The piezoelectric force plate is a widely recognized and direct method for assessing external loading in biomechanical contexts (Novacheck, 1998). This technology operates on the principle that an applied force results in sensor distortion on the plate, leading to measurable voltage changes proportional to the force’s intensity (Bobbert and Schamhardt, 1990). These force plates are instrumental in capturing three-dimensional force and moment data, which are essential for conducting inverse dynamics analyses (Delp et al., 2007). Inverse dynamics is a standard process in motion analysis where the net moment at body joints is calculated based on their acceleration and velocity. This approach is crucial for understanding the mechanics of movement and the forces acting upon the body’s joints (Delp et al., 2007). In addition, the assessment of static loads is also considered a non-negligible issue in postural control rehabilitation and athletic training. A previous study (Martelli et al., 2011) underscores the critical influence of sub-optimal neuromotor control strategies on the internal load dynamics of the hip joint during regular walking activities, suggesting a potential for significantly elevated fracture risks beyond what is estimable through external loading measurements alone.
Gait lab-based kinetic measurements have been used as indictors to assess tibial acceleration (TA), which is utilized for quantifying shock attenuation (Hennig and Lafortune, 1991; Lafortune et al., 1995; Xiang et al., 2022c). The impact shock has been discussed linked with the incidence of chronic overuse injuries (Hennig et al., 1993). Given the advances of wearable technology in the past twenty decades, trial-axis acceleration and angular velocity could be measured from accelerometer and gyroscope in a single inertial sensor (Afaq et al., 2020; Xiang et al., 2022d; Xiang et al., 2022e; Mason et al., 2023; Xiang et al., 2024; Yamane et al., 2024). This made segment acceleration measurements easier and more convenient, shifting the question to: Can we use portable and affordable inertial sensors to evaluate external loading rather than the force plate, which is conventionally embedded in the floor in a gait lab and is cost-prohibitive (Sheerin et al., 2019; Hutabarat et al., 2021; Xiang et al., 2022e)?
Many studies have been conducted attempting to address this question. Johnson et al. (2023) demonstrated a moderate correlation between vertical loading rates and peak vertical TA during walking with load carriage. Tenforde et al. (2020) found that vertical TA could seers as a reliable indicator of load rates in runners with injuries, regardless of their varying foot strike patterns, based on the correlation of coefficient. The findings from Johnson et al. (2021) showed a strong correlation between TA and instantaneous loading rates in the medal-lateral axis while running on a treadmill with rearfoot strike style. Van den Berghe et al. (2019) demonstrated axial and resultant peak TA are highly correlated to peak vertical impact loading rate across different overground running speeds.
Contrarily, recent empirical studies, such as the one by Zandbergen et al. (2023), show no correlation between peak TA and tibial compressive forces. Similarly, Matijevich et al. (2019) demonstrated that metrics of GRF are not strongly correlated with tibial bone load. Therefore, linking GRF metrics with tibial forces or the risk of overuse injuries during running may be misleading (Matijevich et al., 2019).
This leads to a paradox: if TA is an index of running injuries, associated with impact loading rate, then why is there no correlation between TA and tibial bone loading, which is a crucial parameter for tibial stress fractures during running? In other words, while external acceleration is associated with generated external force, it does not correlate with internal force on tibial bone loading (Matijevich et al., 2019; Sheerin et al., 2019; Zandbergen et al., 2023). Therefore, the biomechanics or sports medicine community may need to reconsider whether external acceleration should be an indicator for running injuries, or if internal adaptation is more significant in causing injuries (Matijevich et al., 2019) (Figure 1).
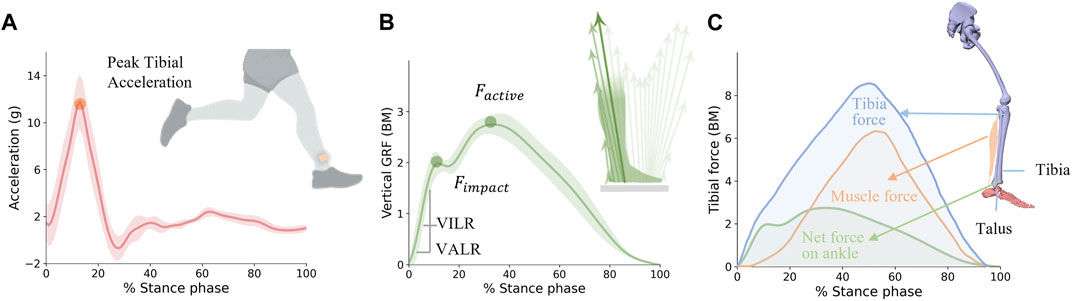
Figure 1. An illustration depicting (A) vertical tibial acceleration, (B) vertical ground reaction force, and (C) tibial force during running.
One of the most significant advancements in biomechanics facilitated by wearable sensors is their capability to enable data-driven approaches, offering portable and innovative solution (Halilaj et al., 2018; Gholami et al., 2020; Hernandez et al., 2021; Xiang et al., 2022e; Mason et al., 2023; Xiang et al., 2023). Notably, the prediction of GRF metrics from inertial sensors using deep learning algorithms has shown high accuracy, as evidenced in studies (Ngoh et al., 2018; Johnson W. R. et al., 2020; Tan et al., 2020). Similarly, projections of inner tibial bone load have been successfully explored through machine learning (Matijevich et al., 2020). Understanding the role of external TA in both external impact loading and internal tibial bone loading, therefore, becomes crucial (Matijevich et al., 2019). Enhancing the evaluation of these factors through machine learning not only presents an intriguing area of research but also holds substantial potential implications for future applications in sports medicine, injury prevention, and rehabilitation strategies (Zadpoor and Nikooyan, 2011; Johnson C. D. et al., 2020; Xiang et al., 2022a; Xiang et al., 2022b; Gao et al., 2023; Lloyd et al., 2023; Uhlrich et al., 2023; Xiang et al., 2023).
This systematic review aims to bridge a critical gap in our understanding of the relationship among GRF, TA, tibial bone loading, and running-related injuries, a topic of significant importance to both athletes and recreational runners. By focusing on the burgeoning role of wearable technology in this domain, we seek to analyze and synthesize recent advancements in this field, considering their increased accessibility and application in both research and practical settings. Our review will methodically examine existing literature, employing rigorous criteria to evaluate the validity and reliability of various measurement techniques. Ultimately, this review endeavors to provide valuable insights into running mechanics and injury prevention, potentially informing future research directions, training methodologies, and rehabilitative practices, thereby leveraging the latest advancements in technology and data analysis.
2 Methods
The protocol of this systematic review was designed in alignment with the Preferred Reporting Items for Systematic Reviews and Meta-Analysis (PRISMA) guidelines (Moher et al., 2010). Additionally, the protocol was officially registered with PROSPERO (Registration Number: CRD42023483210).
2.1 Search strategy
PubMed, Scopus, SPORTDiscus, and IEEE Xplore electronic databases were searched for the period from 2000 to November 2023, using the specified terms combined with the Boolean operators outlined in Table 1. Additionally, relevant studies were identified by reviewing bibliographies in academic articles. The titles, abstracts, and full texts of the retrieved documents were meticulously evaluated to determine their relevance. Only papers published in English that specifically measured TA/tibial loading and GRF in the context of running were considered. Exclusion criteria included papers that exclusively assessed GRF signals, those with sensor placements other than the tibial region, and studies involving participants using any form of aid or equipment during running.
2.2 Eligibility criteria
In accordance with the Participants, Intervention, Comparisons, and Outcomes (PICO) criteria, information was extracted from thirteen included studies. This extraction focused on participant details, correlation variables, and data-driven approaches. The participant information encompassed the number of participants, their gender, age, height, weight, and running speed during data collection. The Pearson correlation coefficient was used for the correlation evaluation in included studies. The correlation variable included data calculated by the acceleration sensor and/or the force plate, as well as running conditions (speeds and surfaces) for data collection. Machine learning including deep learning were extracted from the included studies. The calculation of the Vertical Average Loading Rate (VALR) is based on the gradient of the initial impact transient, specifically over its linear section, typically spanning from 20% to 80% of the vertical impact peak. In contrast, the Vertical Instantaneous Loading Rate (VILR) is determined by identifying the maximum slope between any two consecutive data points within the same region of interest (Davis et al., 2015).
Two independent reviewers (Z.G. and L.X.) conducted the selection process. Disagreements between these authors regarding article inclusion were resolved through further discussion. In cases where consensus was unattainable, a third reviewer (J.F.) was consulted for resolution. Studies were excluded if they met the following criteria: 1) Participants exhibiting physical injuries during testing; 2) TA measured from the proximal tibia or medial aspect of the distal tibia; 3) Absence of correlation or data-driven approaches; 4) Studies that scored below 75% in the quality assessment. The collation of articles and the removal of duplicates were carried out using EndNote X9 (Thomson Reuters, Carlsbad, California, United States).
2.3 Quality assessment
The assessment protocol for appraising the quality of the included articles was based on a modified version of established scales in the fields of sports science, healthcare, and rehabilitation. This approach, commonly used in analyzing studies in an exercise-based training context, adopted the study quality scoring system developed by Black et al. (2016). Two assessors, Z.G. and L.X., independently employed this scoring system to evaluate the quality of the graded articles. The results were then reviewed and confirmed by a third reviewer (J.F.). The evaluation included nine distinct criteria, each contributing to a cumulative score (range: 0–18). The criteria were as follows: (1) inclusion criteria stated (score: 0–2); (2) appropriate assignment of subjects (random/equal baseline); (3) description of intervention; (4) definition of dependent variables; (5) practicality of assessments; (6) practicality of training duration (acute vs. long term); (7) appropriateness of statistics (variability, repeated measures); (8) detailed results (mean, standard deviation, percent change, effect size); (9) insightful conclusions (clear, concise, future directions), with each criterion graded from 0 (no) to 1 (maybe) or 2 (yes). To maintain impartiality in the quality assessment of the included studies, the scores were converted to a percentage scale, ranging from 0% to 100%.
2.4 Data synthesis
2.4.1 Data processing and subgroup analysis
Fisher’s Z transformation is utilized in meta-analysis to synthesize correlation coefficients from diverse studies. This transformation stabilizes the variance of the correlation coefficients, effectively converting them to a scale where they approximate a normal distribution. Consequently, this method facilitates a more precise and dependable estimation of the overall correlation across the compiled studies. In meta-analysis, moderator analysis was performed to analyze the factors of running surface (overground and treadmill running) and foot strike patterns (RFS: rearfoot strike pattern, MFS: midfoot strike pattern, and FFS: forefoot strike pattern). That might influence the size or direction of the effect between vertical peak TA and GRF, i.e., VALR and VILR.
The I2 statistic quantifies the percentage of total variation across studies attributable to heterogeneity rather than random chance. Conventionally, I2 values of 25%, 50%, and 75% are interpreted as indicative of low, moderate, and high heterogeneity, respectively (Higgins et al., 2003). Tau-squared (τ2) serves as an estimate of the variance between studies within the framework of a random-effects model, with larger τ2 values signaling increased heterogeneity. For all tests conducted, an alpha level of 0.05 was established to determine statistical significance. The meta-analysis was conducted using the Meta statistical analysis package in R (version 4.3.2, R Foundation for Statistical Computing, Vienna, Austria).
2.4.2 Sensitivity analysis
Sensitivity analyses were performed to identify potential factors contributing to the observed high heterogeneity and to assess the robustness of the synthesized results. This involved conducting the analysis multiple times, each time sequentially excluding the study with the lowest weight, and then the two studies with the lowest weights, and so on, until the n-1 studies with the lowest weights were excluded (where n equals the total number of included studies). Considering the diversity in the studies included in this review and the variation in effect sizes from one study to another, random effects models were employed in the meta-analysis to accommodate these discrepancies.
3 Results
3.1 Search results
A total of 503 articles were identified via electronic databases retrieve (PubMed = 81, SPORTDiscus = 149, Scopus = 120, IEEE Xplore = 2, ScienceDirect = 151). Of these, 182 duplicate records were removed, and a further 294 articles were excluded based on the title and the abstract screening. Twenty-seven full-text articles were then evaluated, with seven being excluded. Reasons for exclusion included four articles not applying a correlation or data-driven approach, two focusing on jumping and walking studies, and one not addressing vertical direction. Five articles were not included in the quantitative synthesis due to data ineligibility for meta-analysis. The details of the search strategy are presented in Figure 2.
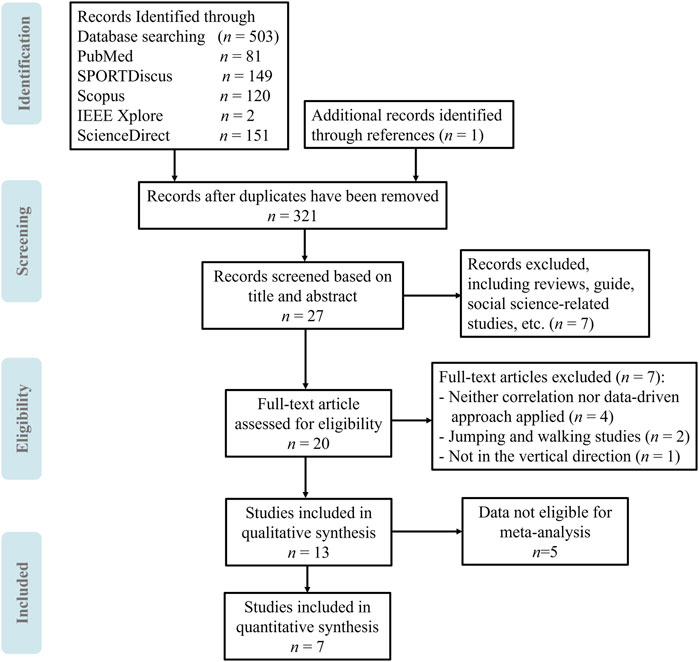
Figure 2. The Preferred Reporting Items for Systematic Reviews and Meta-Analysis (PRISMA) flow diagram illustrating the search strategy used in this review.
3.2 Quality assessment
The quality appraisal ratings for each article are presented in Table 2. Overall, the risk of bias was moderate. Methodological quality scores ranged from 14 to 17 out of a possible 18. The average quality assessment rate for the selected articles in this systematic review was 86.75%. The highest average quality assessment among the quality scores was 1.92 (Q2, Q4, and Q9), and the lowest was 1.38 (Q7). Additionally, seven articles were included in the meta-analysis (Laughton et al., 2003; Greenhalgh et al., 2012; Zhang et al., 2016; Cheung et al., 2019; Van den Berghe et al., 2019; Tenforde et al., 2020; Bradach et al., 2023).
3.3 Study characteristics of data synthesis
As indicated in Table 3, seven studies included in this review assessed the relationship between TA and GRF metrics (Laughton et al., 2003; Greenhalgh et al., 2012; Zhang et al., 2016; Cheung et al., 2019; Van den Berghe et al., 2019; Tenforde et al., 2020; Bradach et al., 2023). Four studies (Zhang et al., 2016; Cheung et al., 2019; Tenforde et al., 2020; Bradach et al., 2023) were conducted on a treadmill, while three studies (Laughton et al., 2003; Greenhalgh et al., 2012; Van den Berghe et al., 2019) involved overground running. Two studies employed tri-axial accelerometers (Greenhalgh et al., 2012; Van den Berghe et al., 2019), one used a bi-axial accelerometer (Cheung et al., 2019), and one used a uniaxial accelerometer (Laughton et al., 2003), while two other studies utilized IMU sensors (Tenforde et al., 2020; Bradach et al., 2023). The frequency of IMU sensors was 1000 Hz in four studies (Greenhalgh et al., 2012; Cheung et al., 2019; Tenforde et al., 2020; Bradach et al., 2023), followed by 960 Hz in one (Laughton et al., 2003), 400 Hz in one (Zhang et al., 2016), and 100 Hz in another (Van den Berghe et al., 2019). Furthermore, the variable from IMU sensors was peak TA (in 7 studies), and the most common GRF variables were VILR (in 6 studies) (Greenhalgh et al., 2012; Zhang et al., 2016; Cheung et al., 2019; Van den Berghe et al., 2019; Tenforde et al., 2020; Bradach et al., 2023) and VALR (in 4 studies) (Laughton et al., 2003; Zhang et al., 2016; Cheung et al., 2019; Tenforde et al., 2020). Extremely strong (3 occurrences), strong (3 occurrences), medium (4 occurrences), weak (1 occurrence), and extremely weak (1 occurrence) correlations between peak TA and GRF metrics were reported in the seven collected literatures.
3.4 Meta-analysis
3.4.1 The correlation between overground and treadmill running
Figure 3 presents a forest plot comparing the Pearson correlation coefficients between peak vertical TA and GRF, specifically VALR and VILR. The sensitivity analysis results were shown in Supplementary Material A (Supplementary Table SA1). For subgroup analysis, the moderating variable of running surfaces was considered, with the overground group comprising 3 studies (5 items) and the treadmill group consisting of 4 studies (7 items). In the overground and treadmill groups, the correlations were 0.62 and 0.73, respectively, with 95% confidence intervals (CI) of 0.42–0.76 for the overground group and 0.68 to 0.77 for the treadmill group. The I2 values were 0% for the overground group (p = 0.69) and 30% for the treadmill group (p = 0.3), indicating heterogeneity levels. The overall correlation between peak vertical acceleration and both VALR and VILR is 0.72, with a 95% CI of 0.67–0.76, and an I2 heterogeneity of 15% (p = 0.3).
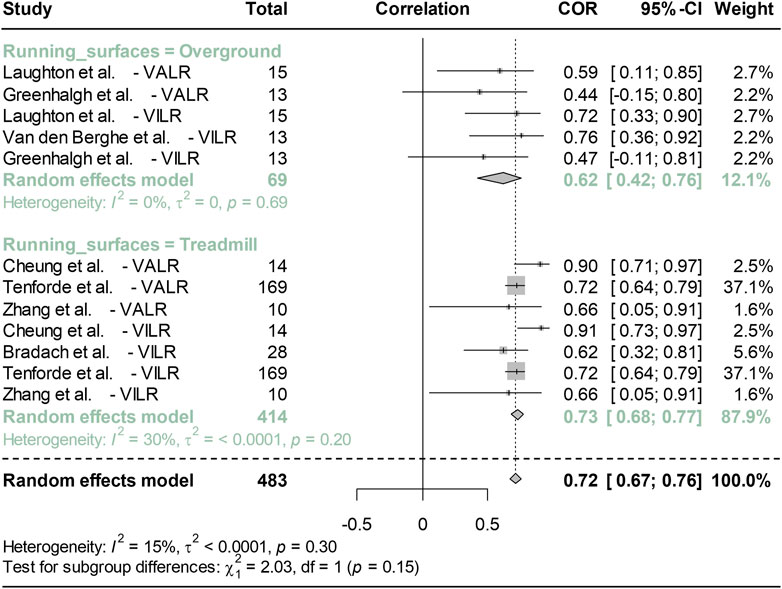
Figure 3. Meta-analysis compares the Pearson correlation coefficient between peak vertical acceleration and both VALR and VILR between overground and treadmill running. Note: VALR represents vertical average load rate, and VILR denotes for vertical instantaneous load rate.
3.4.2 The correlation among different foot strike patterns
Figure 4 displays a forest plot comparing the Pearson correlation coefficients between peak vertical TA and both VALR and VILR across various foot strike patterns. The sensitivity analysis results were shown in Supplementary Materia1 A (Supplementary Table SA2). For the subgroup analysis, the foot strike pattern was used as a moderating variable. The RFS group included 4 studies (comprising 7 items), the FFS group encompassed 2 studies (4 items), and the MFS group consisted of 1 study (2 items). The correlations in the RFS, FFS, and MFS groups were 0.73, 0.75, and 0.74, respectively, with 95% confidence intervals (CI) of 0.61–0.82 for RFS, 0.62–0.83 for FFS, and 0.51–0.86 for MFS. The I2 values indicated heterogeneity levels of 49% for the RFS group, and 0% for both the FFS and MFS groups. Collectively, the correlation coefficient across all groups was 0.71 with a 95% CI of 0.65–0.76, and an I2 value of 14% (p = 0.3).
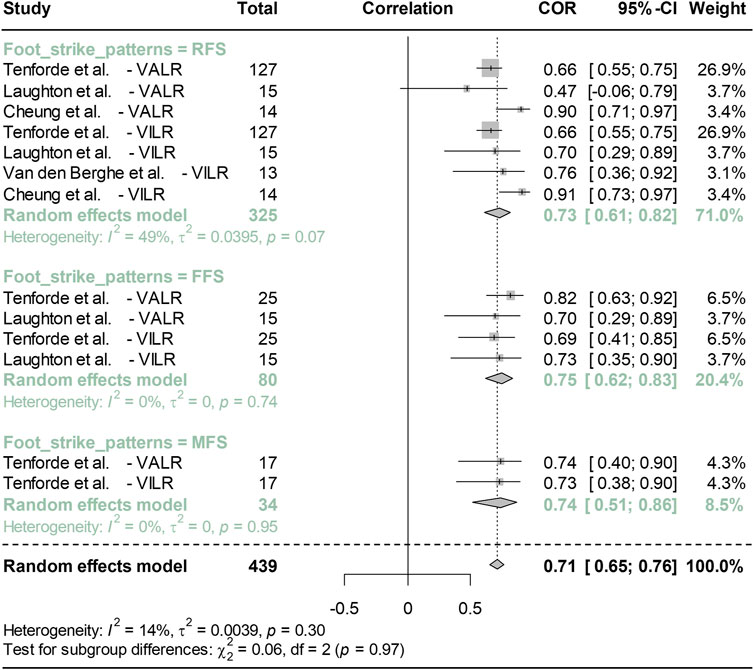
Figure 4. Meta-analysis compares the Pearson correlation coefficient between peak vertical acceleration and both VALR and VILR among different strike patterns. Note: VALR represents vertical average load rate, VILR denotes for vertical instantaneous load rate, RFS is rearfoot strike pattern, MFS is midfoot strike pattern, and FFS is forefoot strike pattern.
3.5 The relationship between TA/GRF, and tibial bone load
As shown in Table 4, two studies included in this review assessed the relationship between TA/GRF and tibial bone load (Matijevich et al., 2019; Zandbergen et al., 2023). Both studies were conducted on treadmills with participants wearing self-selected running shoes. Only one study reported the foot strike pattern as RFS (Zandbergen et al., 2023). In this study (Zandbergen et al., 2023), an IMU sensor, specifically the Xsens model with a sampling frequency of 240 Hz, was used to measure peak TA. Moreover, both studies utilized the Pearson correlation coefficient for correlation analysis. These studies explored correlations between GRF variables (weak correlations) and peak TA (extremely weak correlations) in relation to tibial load.
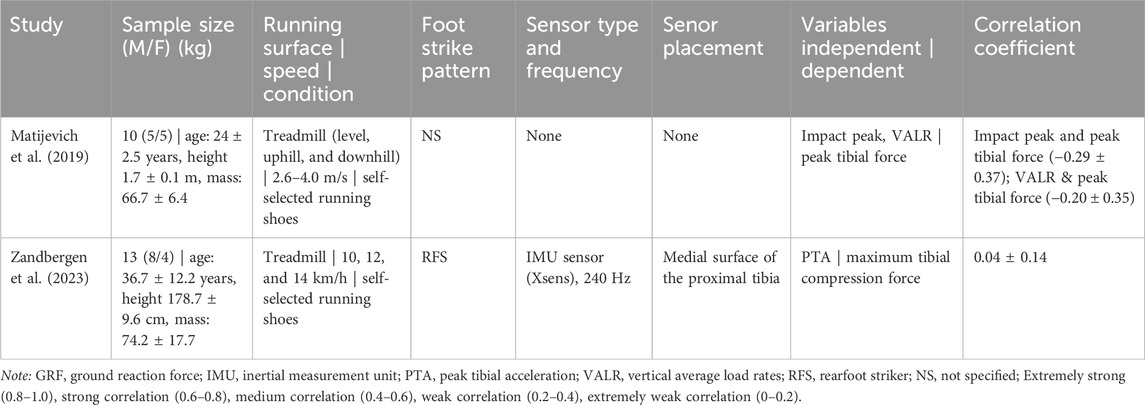
Table 4. Details of studies information of the relationship between tibial acceleration/GRF, and tibial bone load.
3.6 Data-driving approaches
As presented in Table 5, three studies employed data-driven approaches to predict GRF metrics using acceleration data (Komaris et al., 2019; Derie et al., 2020; Tan et al., 2020), and one study used this approach to predict tibial loading force using IMU signals (Komaris et al., 2019). Additionally, three studies were conducted on treadmills (Komaris et al., 2019; Matijevich et al., 2020; Tan et al., 2020), and one was conducted overground (Derie et al., 2020). One study utilized IMU sensors (Tan et al., 2020), one used tri-axial accelerometers (Komaris et al., 2019), and two used virtual accelerometers (Derie et al., 2020; Matijevich et al., 2020), where the acceleration data were derived from kinematic measurements. Various data-driven methods were applied: gradient boosted regression trees (XGB) (Derie et al., 2020), artificial neural networks (ANN) (Komaris et al., 2019), convolutional neural networks (CNN) (Tan et al., 2020), and LASSO regression (Matijevich et al., 2020). The studies consistently showed high predictive accuracy: mean absolute percentage error (MAPE) was below 10% in two studies (Derie et al., 2020; Matijevich et al., 2020), normalized root mean square error (NRMSE) was under 10% in one study (Tan et al., 2020), and RMSE remained less than 0.2 BW across all (Komaris et al., 2019).
4 Discussion
This review critically evaluates the correlation between tibial acceleration, ground reaction forces, and tibial bone loading in running. It highlights the mixed results obtained from existing research in this domain and emphasizes the marginal link found between these biomechanical factors and tibial bone stress. The discussion also underscores the pivotal role of wearable sensor technology in measuring these forces, and its potential when combined with machine learning techniques, in redefining our approach to monitoring, preventing, and rehabilitating running-related injuries.
4.1 Peak tibial acceleration and impact loading rate
The body segment acceleration is shaped by GRF and dampening from bodily shock absorbers. Capturing peak positive acceleration at distal locations allows measurement before attenuation as the shock wave propagates proximally. Notably, vertical acceleration correlates directly with vertical GRF: higher vertical GRF load rate leads to increased vertical axial acceleration prior to attenuation (Lafortune et al., 1995). This findings from the data synthesis analysis showed only moderate correlation of coefficient between peak TA and GRF loading rate, which does not support with the general hypothesis under many studies that peak TA is an indicator of impact loading rate (Bigelow et al., 2013; Lucas-Cuevas et al., 2017; Raper et al., 2018; Cheung et al., 2019; Van den Berghe et al., 2019; Johnson et al., 2021; Ryu et al., 2021; Bradach et al., 2023; Johnson et al., 2023; van Middelaar et al., 2023; Zandbergen et al., 2023). This aligns with findings from the meta-analysis in this study, particularly for overground running.
The prevailing hypothesis in gait retraining research posits a robust positive correlation between the vertical GRF load rate and TA (Cheung et al., 2019; Tirosh et al., 2019; Sheerin et al., 2020; Van den Berghe et al., 2020; Derie et al., 2022). This assumption underpins studies suggesting that mitigating peak TA could be instrumental in reducing overuse injury risks by concurrently diminishing the load rate (Milner et al., 2006; Huang et al., 2019; Tavares et al., 2020; Warden et al., 2021). However, reliance on this correlation as a foundation for gait retraining strategies may result in oversimplified approaches that overlook the complexities of individual gait patterns and the multifaceted nature of injury risk factors (Pohl et al., 2008; van Gelder et al., 2023).
4.2 The correlation between GRF or acceleration and tibial bone load
TA is often used as a proxy for impact forces during running because it's relatively easy to measure, especially with the advent of wearable technology (Ryu et al., 2021; Xiang et al., 2022c; Xiang et al., 2022d; Bradach et al., 2023; van Middelaar et al., 2023; Xiang et al., 2023). However, the relationship between external forces (such as GRF and TA) and internal stresses (such as bone loading) is not always straightforward (Matijevich et al., 2020). Several factors can influence this relationship. Individual biomechanics, such as gait patterns, muscle strength, and joint stability, can significantly alter how external forces are translated into internal stresses (Baggaley et al., 2022). Moreover, the body’s adaptive responses to running, such as increased bone density or changes in soft tissue properties, can also affect this relationship. These adaptations can provide a buffering effect, reducing the impact of external forces on internal structures. A more holistic approach that considers both external forces and individual biomechanical factors could be more effective in understanding and preventing running-related injuries.
Concerning the relationship between GRF and internal bone loads, it is pertinent to note that recent studies, including those by Zandbergen et al. (2023); Matijevich et al. (2019), have provided compelling evidence challenging the traditionally assumed strong correlation. Zandbergen et al. (2023) found no significant correlation between acceleration and internal bone loads in the tibia, nor between GRF features and tibial bone load during running. Consistent with these findings, our meta-analysis demonstrates that peak TA does not directly correlate with the external loading rate. Further, Matijevich et al. (2019) substantiated that GRF metrics are not consistently correlated with tibial bone load across varied running speeds and slopes, thereby questioning the reliability of GRF as a predictor of internal bone stress in different running conditions. Considering that tibial compression forces encompass both external and internal forces, internal biomechanical adaptations may impact internal forces, even in the presence of external overload, thus influencing the prevention of related injuries (Baggaley et al., 2022). This is supported by recent studies (Milner et al., 2006; Van der Worp et al., 2016; Milner et al., 2023). These insights necessitate a reconsideration of existing biomechanical models and wearable technology applications in running injury prevention. It also highlights that the strategy of reducing peak TA or GRF to mitigate tibial stress fracture risk may be misleading (Van der Worp et al., 2016; Zandbergen et al., 2023).
In the realm of running biomechanics, the interplay between neuromotor control and muscle co-contraction presents a critical avenue for understanding the complex dynamics of tibial acceleration, GRF, and tibial bone loading. The coordinated muscle actions, steered by sophisticated neuromotor control, significantly dictate the force distribution and magnitudes transmitted through the musculoskeletal system during running (Kellis et al., 2011; Di Nardo et al., 2015). Insights from Martelli et al. (2011) shed light on how sub-optimal neuromotor strategies can amplify joint loads, potentially leading to increased tibial bone stress in runners. Furthermore, while muscle co-contraction is crucial for joint stabilization, it's important to note that excessive co-contraction might paradoxically decrease stability by increasing the mechanical loads on the tibia, without proportionally enhancing stability (Benjuya et al., 2004; Cenciarini et al., 2009; Tassani et al., 2019). This highlights the importance of identifying an optimal level of muscle co-contraction that ensures joint stability without contributing to unnecessary stress, aligning with the perspectives offered by Martelli et al. (2011).
The advent of wearable sensor technology, capable of capturing these complex neuromotor and muscle dynamics in real-time, opens up new vistas. By amalgamating this data with traditional measures such as GRF and TA, wearable sensors can offer a more nuanced understanding of running biomechanics. This comprehensive approach not only challenges traditional paradigms but also heralds a new era of integrated strategies in monitoring, preventing, and rehabilitating running-related injuries, emphasizing the shift towards more holistic models in running biomechanics studies.
4.3 Data-driven approach to external and internal predictions
The ongoing progression in machine learning and wearable technology has facilitated the innovative use of data from inertial sensors, particularly in the prediction of GRF metrics (Higgins et al., 2003; Cheung et al., 2019; Hernandez et al., 2021). This advancement is notable in its potential to offer a more dependable methodology compared to approaches reliant on the correlation between peak TA and impact loading rate. The latter method’s assumption of a strong correlation may not always hold true (Laughton et al., 2003; Greenhalgh et al., 2012; Zhang et al., 2016), underscoring the significance of this novel application of inertial sensor data in biomechanics studies.
Nevertheless, caution is warranted when asserting that reducing the impact loading rate could effectively mitigate musculoskeletal injuries in running, such as tibial stress fractures (Milner et al., 2006; Milner et al., 2007; Matijevich et al., 2019; Milner et al., 2023). The data-driven approach has also yielded favorable outcomes in projecting tibial bone force using wearable sensor data (Matijevich et al., 2020; Elstub et al., 2022). This approach incorporates the muscular forces acting on the tibia, potentially offering a more comprehensive understanding of musculoskeletal injuries (Matijevich et al., 2019). By integrating this data with external impact loading rates, a more holistic view of the biomechanical factors contributing to injury risk can be achieved, enhancing the precision and effectiveness of injury prediction and prevention strategies. Although data-driven approaches using wearable sensors show promise for predicting external loading (Derie et al., 2020; Tan et al., 2020) and internal muscular force (Matijevich et al., 2019; Matijevich et al., 2020), their opaque “black-box” nature presents a challenge in terms of data interpretability or explainable artificial intelligence (XAI) (Halilaj et al., 2018; Uhlrich et al., 2023). This area warrants further investigation to understand how wearable sensor signals correlate with biomechanical forces (Kaji et al., 2019; Schlegel et al., 2019; Jeyakumar et al., 2020; Gandin et al., 2021; Xiang et al., 2024). Therefore, personalized biomechanical adaptation strategies, tailored for precise injury prevention and rehabilitation monitoring, can be more effectively applied once a deeper understanding of these correlations is achieved.
4.4 Implications for future studies
➢ The utility of peak TA as an indicator of GRF, particularly VALR and VILR during running, is subject to skepticism in the context of current literature, especially with respect to overground running.
➢ A moderate to strong correlation exists between peak TA and vertical loading rate, irrespective of the foot strike patterns. However, it is important to note that the sample sizes for RFS and MFS are relatively limited, warranting caution in generalization of these findings.
➢ Strategies for gait retraining that focus on diminishing loading rates through the reduction of peak TA may not be adequately supported by empirical evidence.
➢ While a correlation between peak TA and impact loading is observed, this does not necessarily imply a direct linear relationship between either GRF or TA and the internal forces exerted on the tibial bone.
➢ Data-driven models, which utilize acceleration data from inertial wearable sensors, exhibit a proficient capability in accurately predicting both external impact loading and internal tibial bone loading.
➢ Future studies should focus on enhancing XAI to augment interpretability of data-driven biomechanical models. This advancement is crucial for effectively correlating wearable sensor data with biomechanical forces.
➢ Embracing multifactorial methodologies that integrate insights from biomechanics, data science, kinesiology, and clinical practice not only minimizes confounding factors but also enriches the interpretation and applicability of research outcomes in real-world settings.
5 Conclusion
In conclusion, this study critically assesses the relationship between TA, GRF, and tibial bone loading in the context of running. It highlights the limited correlation between these biomechanical factors and tibial bone stress, challenging traditional beliefs. The research underscores the significant potential of wearable sensors and machine learning in advancing our understanding of running biomechanics. These technologies offer promising avenues for injury monitoring, prevention, and rehabilitation, suggesting a need for a shift towards more integrated and holistic approaches in running biomechanics.
Author contributions
LX: Conceptualization, Data curation, Investigation, Methodology, Visualization, Software, Writing–original draft. ZG: Conceptualization, Formal Analysis, Investigation, Methodology, Software, Writing–original draft. AW: Methodology, Data curation, Writing–review and editing. VS: Formal Analysis, Investigation, Validation, Writing–review and editing. GF: Data curation, Investigation, Validation, Writing–review and editing. YG: Conceptualization, Funding acquisition, Investigation, Writing–review and editing. JF: Methodology, Project administration, Writing–review and editing.
Funding
The author(s) declare that financial support was received for the research, authorship, and/or publication of this article. This research was supported by the Zhejiang Provincial Natural Science Foundation of China for Distinguished Young Scholars (LR22A020002), Zhejiang Provincial Key Research and Development Program of China (2021C03130), Zhejiang Provincial Natural Science Foundation (LTGY23H040003), Ningbo key R&D Program (2022Z196), Research Academy of Medicine Combining Sports, Ningbo (No. 2023001), the Project of NINGBO Leading Medical and Health Discipline (No. 2022-F15 and 2022-F22), Ningbo Natural Science Foundation (20221JCGY010532, 20221JCGY010607), Public Welfare Science and Technology Project of Ningbo, China (2021S134), Zhejiang Rehabilitation Medical Association Scientific Research Special Fund (ZKKY2023001), and K. C. Wong Magna Fund in Ningbo University. LX and ZG are being sponsored by the China Scholarship Council (CSC).
Conflict of interest
The authors declare that the research was conducted in the absence of any commercial or financial relationships that could be construed as a potential conflict of interest.
Publisher’s note
All claims expressed in this article are solely those of the authors and do not necessarily represent those of their affiliated organizations, or those of the publisher, the editors and the reviewers. Any product that may be evaluated in this article, or claim that may be made by its manufacturer, is not guaranteed or endorsed by the publisher.
Supplementary material
The Supplementary Material for this article can be found online at: https://www.frontiersin.org/articles/10.3389/fbioe.2024.1377383/full#supplementary-material
References
Afaq, S., Loh, M., Kooner, J., and Chambers, J. (2020). Evaluation of three accelerometer devices for physical activity measurement amongst South Asians and Europeans. Phys. Activity Health 4 (1), 1–10. doi:10.5334/paah.46
Baggaley, M., Derrick, T. R., Vernillo, G., Millet, G. Y., and Edwards, W. B. (2022). Internal tibial forces and moments during graded running. J. biomechanical Eng. 144 (1), 011009. doi:10.1115/1.4051924
Benjuya, N., Melzer, I., and Kaplanski, J. (2004). Aging-induced shifts from a reliance on sensory input to muscle cocontraction during balanced standing. Journals Gerontology Ser. A Biol. Sci. Med. Sci. 59 (2), 166–171. doi:10.1093/gerona/59.2.M166
Bigelow, E. M., Elvin, N. G., Elvin, A. A., and Arnoczky, S. P. (2013). Peak impact accelerations during track and treadmill running. J. Appl. biomechanics 29 (5), 639–644. doi:10.1123/jab.29.5.639
Black, G. M., Gabbett, T. J., Cole, M. H., and Naughton, G. (2016). Monitoring workload in throwing-dominant sports: a systematic review. Sports Med. 46, 1503–1516. doi:10.1007/s40279-016-0529-6
Bobbert, M. F., and Schamhardt, H. C. (1990). Accuracy of determining the point of force application with piezoelectric force plates. J. biomechanics 23 (7), 705–710. doi:10.1016/0021-9290(90)90169-4
Bradach, M. M., Gaudette, L. W., Tenforde, A. S., Outerleys, J., de Souza Júnior, J. R., and Johnson, C. D. (2023). The effects of a simple sensor reorientation procedure on peak tibial accelerations during running and correlations with ground reaction forces. Sensors 23 (13), 6048. doi:10.3390/s23136048
Cenciarini, M., Loughlin, P. J., Sparto, P. J., and Redfern, M. S. (2009). Stiffness and damping in postural control increase with age. IEEE Trans. Biomed. Eng. 57 (2), 267–275. doi:10.1109/TBME.2009.2031874
Cheung, R. T., Zhang, J. H., Chan, Z. Y., An, W. W., Au, I. P., MacPhail, A., et al. (2019). Shoe-mounted accelerometers should be used with caution in gait retraining. Scand. J. Med. Sci. sports 29 (6), 835–842. doi:10.1111/sms.13396
Davis, I. S., Bowser, B. J., and Mullineaux, D. R. (2015). Greater vertical impact loading in female runners with medically diagnosed injuries: a prospective investigation. Br. J. sports Med. 50, 887–892. doi:10.1136/bjsports-2015-094579
Delp, S. L., Anderson, F. C., Arnold, A. S., Loan, P., Habib, A., John, C. T., et al. (2007). OpenSim: open-source software to create and analyze dynamic simulations of movement. IEEE Trans. Biomed. Eng. 54 (11), 1940–1950. doi:10.1109/TBME.2007.901024
Derie, R., Robberechts, P., Van den Berghe, P., Gerlo, J., De Clercq, D., Segers, V., et al. (2020). Tibial acceleration-based prediction of maximal vertical loading rate during overground running: a machine learning approach. Front. Bioeng. Biotechnol. 8, 33. doi:10.3389/fbioe.2020.00033
Derie, R., Van den Berghe, P., Gerlo, J., Bonnaerens, S., Caekenberghe, I. V., Fiers, P., et al. (2022). Biomechanical adaptations following a music-based biofeedback gait retraining program to reduce peak tibial accelerations. Scand. J. Med. Sci. Sports 32 (7), 1142–1152. doi:10.1111/sms.14162
Di Nardo, F., Mengarelli, A., Maranesi, E., Burattini, L., and Fioretti, S. (2015). Assessment of the ankle muscle co-contraction during normal gait: a surface electromyography study. J. Electromyogr. Kinesiol. 25 (2), 347–354. doi:10.1016/j.jelekin.2014.10.016
Elstub, L., Nurse, C., Grohowski, L., Volgyesi, P., Wolf, D., and Zelik, K. (2022). Tibial bone forces can be monitored using shoe-worn wearable sensors during running. J. sports Sci. 40 (15), 1741–1749. doi:10.1080/02640414.2022.2107816
Gandin, I., Scagnetto, A., Romani, S., and Barbati, G. (2021). Interpretability of time-series deep learning models: a study in cardiovascular patients admitted to Intensive care unit. J. Biomed. Inf. 121, 103876. doi:10.1016/j.jbi.2021.103876
Gao, Z., Xiang, L., Fekete, G., Baker, J. S., Mao, Z., and Gu, Y. (2023). A data-driven approach for fatigue detection during running using pedobarographic measurements. Appl. Bionics Biomechanics 2023, 1–11. doi:10.1155/2023/7022513
Gholami, M., Napier, C., and Menon, C. (2020). Estimating lower extremity running gait kinematics with a single accelerometer: a deep learning approach. Sensors 20 (10), 2939. doi:10.3390/s20102939
Greenhalgh, A., Sinclair, J., Protheroe, L., and Chockalingam, N. (2012). Predicting impact shock magnitude: which ground reaction force variable should we use. Int. J. Sports Sci. Eng. 6 (4), 225–231.
Halilaj, E., Rajagopal, A., Fiterau, M., Hicks, J. L., Hastie, T. J., and Delp, S. L. (2018). Machine learning in human movement biomechanics: best practices, common pitfalls, and new opportunities. J. biomechanics 81, 1–11. doi:10.1016/j.jbiomech.2018.09.009
Hennig, E. M., and Lafortune, M. A. (1991). Relationships between ground reaction force and tibial bone acceleration parameters. J. Appl. Biomechanics 7 (3), 303–309. doi:10.1123/ijsb.7.3.303
Hennig, E. M., Milani, T. L., and Lafortune, M. A. (1993). Use of ground reaction force parameters in predicting peak tibial accelerations in running. J. Appl. biomechanics 9 (4), 306–314. doi:10.1123/jab.9.4.306
Hernandez, V., Dadkhah, D., Babakeshizadeh, V., and Kulić, D. (2021). Lower body kinematics estimation from wearable sensors for walking and running: a deep learning approach. Gait posture 83, 185–193. doi:10.1016/j.gaitpost.2020.10.026
Higgins, J. P., Thompson, S. G., Deeks, J. J., and Altman, D. G. (2003). Measuring inconsistency in meta-analyses. Bmj 327 (7414), 557–560. doi:10.1136/bmj.327.7414.557
Huang, Y., Xia, H., Chen, G., Cheng, S., Cheung, R. T., and Shull, P. B. (2019). Foot strike pattern, step rate, and trunk posture combined gait modifications to reduce impact loading during running. J. Biomechanics 86, 102–109. doi:10.1016/j.jbiomech.2019.01.058
Hutabarat, Y., Owaki, D., and Hayashibe, M. (2021). Recent advances in quantitative gait analysis using wearable sensors: a review. IEEE Sensors J. 21 (23), 26470–26487. doi:10.1109/JSEN.2021.3119658
Jeyakumar, J. V., Noor, J., Cheng, Y.-H., Garcia, L., and Srivastava, M. (2020). How can i explain this to you? an empirical study of deep neural network explanation methods. Adv. Neural Inf. Process. Syst. 33, 4211–4222.
Johnson, C. D., Outerleys, J., and Davis, I. S. (2021). Relationships between tibial acceleration and ground reaction force measures in the medial-lateral and anterior-posterior planes. J. biomechanics 117, 110250. doi:10.1016/j.jbiomech.2021.110250
Johnson, C. D., Sara, L. K., Bradach, M. M., Mullineaux, D. R., Foulis, S. A., Hughes, J. M., et al. (2023). Relationships between tibial accelerations and ground reaction forces during walking with load carriage. J. Biomechanics 156, 111693. doi:10.1016/j.jbiomech.2023.111693
Johnson, C. D., Tenforde, A. S., Outerleys, J., Reilly, J., and Davis, I. S. (2020a). Impact-related ground reaction forces are more strongly associated with some running injuries than others. Am. J. sports Med. 48(12), 3072–3080. doi:10.1177/0363546520950731
Johnson, W. R., Mian, A., Robinson, M. A., Verheul, J., Lloyd, D. G., and Alderson, J. A. (2020b). Multidimensional ground reaction forces and moments from wearable sensor accelerations via deep learning. IEEE Trans. Biomed. Eng. 68 (1), 289–297. doi:10.1109/TBME.2020.3006158
Kaji, D. A., Zech, J. R., Kim, J. S., Cho, S. K., Dangayach, N. S., Costa, A. B., et al. (2019). An attention based deep learning model of clinical events in the intensive care unit. PloS one 14 (2), e0211057. doi:10.1371/journal.pone.0211057
Kellis, E., Zafeiridis, A., and Amiridis, I. G. (2011). Muscle coactivation before and after the impact phase of running following isokinetic fatigue. J. Athl. Train. 46 (1), 11–19. doi:10.4085/1062-6050-46.1.11
Komaris, D.-S., Pérez-Valero, E., Jordan, L., Barton, J., Hennessy, L., O’Flynn, B., et al. (2019). Predicting three-dimensional ground reaction forces in running by using artificial neural networks and lower body kinematics. IEEE Access 7, 156779–156786. doi:10.1109/ACCESS.2019.2949699
Lafortune, M. A., Lake, M. J., and Hennig, E. (1995). Transfer function between tibial acceleration and ground reaction force. J. Biomechanics 28 (1), 113–117. doi:10.1016/0021-9290(95)80014-x
Laughton, C. A., Davis, I. M., and Hamill, J. (2003). Effect of strike pattern and orthotic intervention on tibial shock during running. J. Appl. biomechanics 19 (2), 153–168. doi:10.1123/jab.19.2.153
Lloyd, D. G., Jonkers, I., Delp, S. L., and Modenese, L. (2023). The history and future of neuromusculoskeletal biomechanics. J. Appl. Biomechanics 39 (5), 273–283. doi:10.1123/jab.2023-0165
Lucas-Cuevas, A. G., Encarnación-Martínez, A., Camacho-García, A., Llana-Belloch, S., and Pérez-Soriano, P. (2017). The location of the tibial accelerometer does influence impact acceleration parameters during running. J. sports Sci. 35 (17), 1734–1738. doi:10.1080/02640414.2016.1235792
Martelli, S., Taddei, F., Cappello, A., van Sint Jan, S., Leardini, A., and Viceconti, M. (2011). Effect of sub-optimal neuromotor control on the hip joint load during level walking. J. biomechanics 44 (9), 1716–1721. doi:10.1016/j.jbiomech.2011.03.039
Mason, R., Pearson, L. T., Barry, G., Young, F., Lennon, O., Godfrey, A., et al. (2023). Wearables for running gait analysis: a systematic review. Sports Med. 53 (1), 241–268. doi:10.1007/s40279-022-01760-6
Matijevich, E. S., Branscombe, L. M., Scott, L. R., and Zelik, K. E. (2019). Ground reaction force metrics are not strongly correlated with tibial bone load when running across speeds and slopes: implications for science, sport and wearable tech. PloS one 14 (1), e0210000. doi:10.1371/journal.pone.0210000
Matijevich, E. S., Scott, L. R., Volgyesi, P., Derry, K. H., and Zelik, K. E. (2020). Combining wearable sensor signals, machine learning and biomechanics to estimate tibial bone force and damage during running. Hum. Mov. Sci. 74, 102690. doi:10.1016/j.humov.2020.102690
Milner, C. E., Ferber, R., Pollard, C. D., Hamill, J., and Davis, I. S. (2006). Biomechanical factors associated with tibial stress fracture in female runners. Med. Sci. Sports Exerc. 38 (2), 323–328. doi:10.1249/01.mss.0000183477.75808.92
Milner, C. E., Foch, E., Gonzales, J. M., and Petersen, D. (2023). Biomechanics associated with tibial stress fracture in runners: a systematic review and meta-analysis. J. Sport Health Sci. 12 (3), 333–342. doi:10.1016/j.jshs.2022.12.002
Milner, C. E., Hamill, J., and Davis, I. (2007). Are knee mechanics during early stance related to tibial stress fracture in runners? Clin. Biomech. 22 (6), 697–703. doi:10.1016/j.clinbiomech.2007.03.003
Moher, D., Liberati, A., Tetzlaff, J., Altman, D. G., and Group, P. (2010). Preferred reporting items for systematic reviews and meta-analyses: the PRISMA statement. Int. J. Surg. 8 (5), e1000097–e1000341. doi:10.1371/journal.pmed.1000097
Ngoh, K.J.-H., Gouwanda, D., Gopalai, A. A., and Chong, Y. Z. (2018). Estimation of vertical ground reaction force during running using neural network model and uniaxial accelerometer. J. biomechanics 76, 269–273. doi:10.1016/j.jbiomech.2018.06.006
Novacheck, T. F. (1998). The biomechanics of running. Gait posture 7 (1), 77–95. doi:10.1016/s0966-6362(97)00038-6
Pan, J. W., Ho, M. Y. M., Loh, R. B. C., Iskandar, M. N. S., and Kong, P. W. (2023). Foot morphology and running gait pattern between the left and right limbs in recreational runners. Phys. Activity Health 7 (1), 43–52. doi:10.5334/paah.226
Pohl, M. B., Mullineaux, D. R., Milner, C. E., Hamill, J., and Davis, I. S. (2008). Biomechanical predictors of retrospective tibial stress fractures in runners. J. biomechanics 41 (6), 1160–1165. doi:10.1016/j.jbiomech.2008.02.001
Raper, D. P., Witchalls, J., Philips, E. J., Knight, E., Drew, M. K., and Waddington, G. (2018). Use of a tibial accelerometer to measure ground reaction force in running: a reliability and validity comparison with force plates. J. Sci. Med. sport 21 (1), 84–88. doi:10.1016/j.jsams.2017.06.010
Ryu, S., Lee, Y.-S., and Park, S.-K. (2021). Impact signal differences dependent on the position of accelerometer attachment and the correlation with the ground reaction force during running. Int. J. Precis. Eng. Manuf. 22, 1791–1798. doi:10.1007/s12541-021-00483-4
Schlegel, U., Arnout, H., El-Assady, M., Oelke, D., and Keim, D. A. (2019). “Towards a rigorous evaluation of XAI methods on time series,” in 2019 IEEE/CVF International Conference on Computer Vision Workshop (ICCVW) (IEEE), 4197–4201.
Sheerin, K. R., Reid, D., and Besier, T. F. (2019). The measurement of tibial acceleration in runners—a review of the factors that can affect tibial acceleration during running and evidence-based guidelines for its use. Gait posture 67, 12–24. doi:10.1016/j.gaitpost.2018.09.017
Sheerin, K. R., Reid, D., Taylor, D., and Besier, T. F. (2020). The effectiveness of real-time haptic feedback gait retraining for reducing resultant tibial acceleration with runners. Phys. Ther. Sport 43, 173–180. doi:10.1016/j.ptsp.2020.03.001
Tan, T., Strout, Z. A., and Shull, P. B. (2020). Accurate impact loading rate estimation during running via a subject-independent convolutional neural network model and optimal IMU placement. IEEE J. Biomed. Health Inf. 25 (4), 1215–1222. doi:10.1109/jbhi.2020.3014963
Tassani, S., Font-Llagunes, J. M., González Ballester, M. A., and Noailly, J. (2019). Muscular tension significantly affects stability in standing posture. Gait Posture 68 (1), 220–226. doi:10.1016/j.gaitpost.2018.11.034
Tavares, J., Jost, T., Drewelow, G., and Rylander, J. (2020). Do maximalist shoes mitigate risk factors for tibial stress fractures better than stability or flexible (marketed as minimalist) shoes? Footwear Sci. 12 (1), 63–74. doi:10.1080/19424280.2019.1708977
Tenforde, A. S., Hayano, T., Jamison, S. T., Outerleys, J., and Davis, I. S. (2020). Tibial acceleration measured from wearable sensors is associated with loading rates in injured runners. PM&R 12 (7), 679–684. doi:10.1002/pmrj.12275
Tirosh, O., Steinberg, N., Nemet, D., Eliakim, A., and Orland, G. (2019). Visual feedback gait re-training in overweight children can reduce excessive tibial acceleration during walking and running: an experimental intervention study. Gait posture 68, 101–105. doi:10.1016/j.gaitpost.2018.11.006
Uhlrich, S. D., Uchida, T. K., Lee, M. R., and Delp, S. L. (2023). Ten steps to becoming a musculoskeletal simulation expert: a half-century of progress and outlook for the future. J. Biomechanics 154, 111623. doi:10.1016/j.jbiomech.2023.111623
Van den Berghe, P., Gosseries, M., Gerlo, J., Lenoir, M., Leman, M., and De Clercq, D. (2020). Change-point detection of peak tibial acceleration in overground running retraining. Sensors 20 (6), 1720. doi:10.3390/s20061720
Van den Berghe, P., Six, J., Gerlo, J., Leman, M., and De Clercq, D. (2019). Validity and reliability of peak tibial accelerations as real-time measure of impact loading during over-ground rearfoot running at different speeds. J. biomechanics 86, 238–242. doi:10.1016/j.jbiomech.2019.01.039
Van der Worp, H., Vrielink, J. W., and Bredeweg, S. W. (2016). Do runners who suffer injuries have higher vertical ground reaction forces than those who remain injury-free? A systematic review and meta-analysis. Br. J. sports Med. 50, 450–457. doi:10.1136/bjsports-2015-094924
van Gelder, L. M., Barnes, A., Wheat, J. S., and Heller, B. W. (2023). Runners’ responses to a biofeedback intervention aimed to reduce tibial acceleration differ within and between individuals. J. Biomechanics 157, 111686. doi:10.1016/j.jbiomech.2023.111686
van Middelaar, R. P., Zhang, J., Veltink, P. H., and Reenalda, J. (2023). 3D tibial acceleration and consideration of 3D angular motion using IMUs on peak tibial acceleration and impulse in running. Med. Sci. sports Exerc. 55 (12), 2253–2262. doi:10.1249/mss.0000000000003269
Warden, S. J., Edwards, W. B., and Willy, R. W. (2021). Preventing bone stress injuries in runners with optimal workload. Curr. Osteoporos. Rep. 19 (3), 298–307. doi:10.1007/s11914-021-00666-y
Willwacher, S., Kurz, M., Robbin, J., Thelen, M., Hamill, J., Kelly, L., et al. (2022). Running-related biomechanical risk factors for overuse injuries in distance runners: a systematic review considering injury specificity and the potentials for future research. Sports Med. 52 (8), 1863–1877. doi:10.1007/s40279-022-01666-3
Xiang, L., Deng, K., Mei, Q., Gao, Z., Yang, T., Wang, A., et al. (2022a). Population and age-based cardiorespiratory fitness level investigation and automatic prediction. Front. Cardiovasc. Med. 8, 758589. doi:10.3389/fcvm.2021.758589
Xiang, L., Gu, Y., Gao, Z., Yu, P., Shim, V., Wang, A., et al. (2024). Integrating an LSTM framework for predicting ankle joint biomechanics during gait using inertial sensors. Comput. Biol. Med. 170, 108016. doi:10.1016/j.compbiomed.2024.108016
Xiang, L., Gu, Y., Mei, Q., Wang, A., Shim, V., and Fernandez, J. (2022b). Automatic classification of barefoot and shod populations based on the foot metrics and plantar pressure patterns. Front. Bioeng. Biotechnol. 10, 843204. doi:10.3389/fbioe.2022.843204
Xiang, L., Gu, Y., Rong, M., Gao, Z., Yang, T., Wang, A., et al. (2022c). Shock acceleration and attenuation during running with minimalist and maximalist shoes: a time-and frequency-domain analysis of tibial acceleration. Bioengineering 9 (7), 322. doi:10.3390/bioengineering9070322
Xiang, L., Gu, Y., Wang, A., Mei, Q., Yu, P., Shim, V., et al. (2022d). Effect of foot pronation during distance running on the lower limb impact acceleration and dynamic stability. Acta Bioeng. Biomechanics 24 (4), 21–30. doi:10.37190/ABB-02165-2022-02
Xiang, L., Gu, Y., Wang, A., Shim, V., Gao, Z., and Fernandez, J. (2023). Foot pronation prediction with inertial sensors during running: a preliminary application of data-driven approaches. J. Hum. Kinet. 88, 29–40. doi:10.5114/jhk/163059
Xiang, L., Wang, A., Gu, Y., Zhao, L., Shim, V., and Fernandez, J. (2022e). Recent machine learning progress in lower limb running biomechanics with wearable technology: a systematic review. Front. Neurorobotics 16, 913052. doi:10.3389/fnbot.2022.913052
Yamane, T., Kimura, M., and Morita, M. (2024). Application of nine-axis accelerometer-based recognition of daily activities in clinical examination. Phys. Activity Health 8 (1), 29–46. doi:10.5334/paah.313
Yang, T., Xiang, L., Ying, S., Li, J., Fernandez, J., and Gu, Y. (2023). Effects of different cushioned insoles on ankle and knee joints biomechanics during load carriage running. Int. J. Biomed. Eng. Technol. 43 (3), 259–274. doi:10.1504/IJBET.2023.134589
Zadpoor, A. A., and Nikooyan, A. A. (2011). The relationship between lower-extremity stress fractures and the ground reaction force: a systematic review. Clin. Biomech. 26 (1), 23–28. doi:10.1016/j.clinbiomech.2010.08.005
Zandbergen, M. A., Ter Wengel, X. J., van Middelaar, R. P., Buurke, J. H., Veltink, P. H., and Reenalda, J. (2023). Peak tibial acceleration should not be used as indicator of tibial bone loading during running. Sports Biomech. 00, 1–18. doi:10.1080/14763141.2022.2164345
Keywords: impact load, tibial acceleration, inertial measurement unit (IMU) sensor, machine learning, running
Citation: Xiang L, Gao Z, Wang A, Shim V, Fekete G, Gu Y and Fernandez J (2024) Rethinking running biomechanics: a critical review of ground reaction forces, tibial bone loading, and the role of wearable sensors. Front. Bioeng. Biotechnol. 12:1377383. doi: 10.3389/fbioe.2024.1377383
Received: 27 January 2024; Accepted: 22 March 2024;
Published: 08 April 2024.
Edited by:
Elvira Padua, Università Telematica San Raffaele, ItalyReviewed by:
Ukadike Chris Ugbolue, University of the West of Scotland, United KingdomSimone Tassani, Pompeu Fabra University, Spain
Copyright © 2024 Xiang, Gao, Wang, Shim, Fekete, Gu and Fernandez. This is an open-access article distributed under the terms of the Creative Commons Attribution License (CC BY). The use, distribution or reproduction in other forums is permitted, provided the original author(s) and the copyright owner(s) are credited and that the original publication in this journal is cited, in accordance with accepted academic practice. No use, distribution or reproduction is permitted which does not comply with these terms.
*Correspondence: Yaodong Gu, Z3V5YW9kb25nQGhvdG1haWwuY29t
†These authors have contributed equally to this work