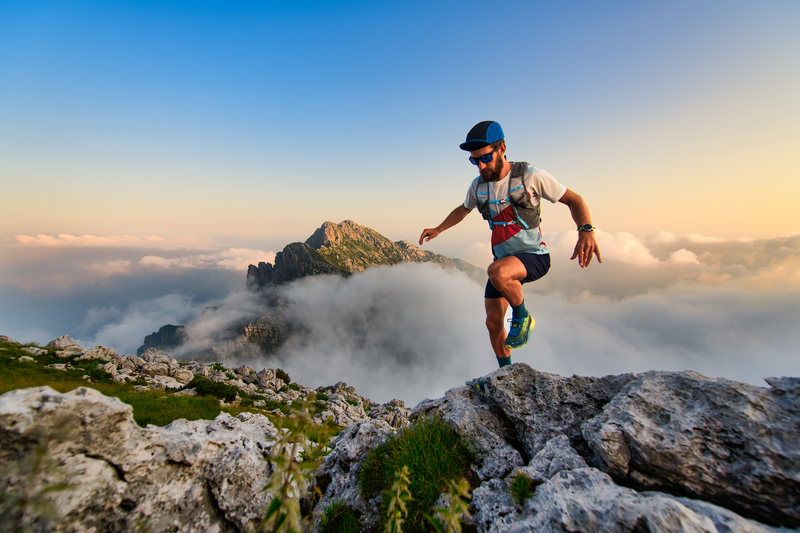
94% of researchers rate our articles as excellent or good
Learn more about the work of our research integrity team to safeguard the quality of each article we publish.
Find out more
REVIEW article
Front. Bioeng. Biotechnol. , 27 March 2024
Sec. Bioprocess Engineering
Volume 12 - 2024 | https://doi.org/10.3389/fbioe.2024.1347138
Background: Investigating the metabolic behaviour of different cellular phenotypes, i.e., good/bad grower and/or producer, in production culture is important to identify the key metabolite(s)/pathway(s) that regulate cell growth and/or recombinant protein production to improve the overall yield. Currently, LC-MS, GC-MS and NMR are the most used and advanced technologies for investigating the metabolome. Although contributed significantly in the domain, each technique has its own biasness towards specific metabolites or class of metabolites due to various reasons including variability in the concept of working, sample preparation, metabolite-extraction methods, metabolite identification tools, and databases. As a result, the application of appropriate analytical technique(s) is very critical.
Purpose and scope: This review provides a state-of-the-art technological insights and overview of metabolic mechanisms involved in regulation of cell growth and/or recombinant protein production for improving yield from CHO cultures.
Summary and conclusion: In this review, the advancements in CHO metabolomics over the last 10 years are traced based on a bibliometric analysis of previous publications and discussed. With the technical advancement in the domain of LC-MS, GC-MS and NMR, metabolites of glycolytic and nucleotide biosynthesis pathway (glucose, fructose, pyruvate and phenylalanine, threonine, tryptophan, arginine, valine, asparagine, and serine, etc.) were observed to be upregulated in exponential-phase thereby potentially associated with cell growth regulation, whereas metabolites/intermediates of TCA, oxidative phosphorylation (aspartate, glutamate, succinate, malate, fumarate and citrate), intracellular NAD+/NADH ratio, and glutathione metabolic pathways were observed to be upregulated in stationary-phase and hence potentially associated with increased cell-specific productivity in CHO bioprocess. Moreover, each of technique has its own bias towards metabolite identification, indicating their complementarity, along with a number of critical gaps in the CHO metabolomics pipeline and hence first time discussed here to identify their potential remedies. This knowledge may help in future study designs to improve the metabolomic coverage facilitating identification of the metabolites/pathways which might get missed otherwise and explore the full potential of metabolomics for improving the CHO bioprocess performances.
Chinese Hamster ovary (CHO) cells are the expression system of choice for production of over 70% of all recombinant biopharmaceutical proteins including monoclonal antibodies (like adalimumab, bezlotoxumab, brodalumab, etc.) and complex human proteins (i.e., erythropoietin and clotting factors, etc.) due to their ability to grow fast to achieve high-density in protein-free and chemically defined culture media, perform human-like post-translational modifications, appropriate protein folding, high productivity and low susceptibility to human viral infections (Wurm, 2004; Lalonde and Durocher, 2017; Singh et al., 2023). However, the cost of such products is still high and requires significant improvements in the overall yield. To date, strategies like cell engineering, enriched culture media development and process optimization (biphasic culture approaches such as temperature shift and/or use of chemicals affecting cell cycle) (Trummer et al., 2006; Bollati-Fogolı, 2008; Kaisermayer et al., 2016; Ritacco et al., 2018; Huang et al., 2020; McHugh et al., 2020; Weng et al., 2020; Donaldson et al., 2022) have been employed to meet the increasing global demand at an affordable cost. As a result, CHO production cultures are currently able to achieve up to 10 g/L, which is 100-fold higher since 1980s. However, more improvement in the yield and quality are required to further reduce their cost and this can only be achieved by improving our understanding of CHO cell biology and their behaviour in bioprocess, thereby demanding greater efforts in this direction.
Multidimensional “omics” approaches like genomics, proteomics, transcriptomics and metabolomics have been proven to be powerful and complementary tools for exploratory research and improving the current knowledge (Jendoubi, 2021). To date, the potentials of genomics, transcriptomics and proteomics in CHO biology is being explored extensively (Jendoubi, 2021; Menyhárt and Győrffy, 2021). However, limited efforts have been made to understand the CHO cell metabolism in bioprocess (Lewis et al., 2016) and hence being targeted in this review.
Metabolomics identifies and quantifies small molecules (<1,500 Da), called metabolites, which vary in concentration based on cellular response to environmental changes and hence better reflects the performance of biological pathways and the physiologic status of a cell in certain condition/environment (Mashabela et al., 2022). Metabolomics provides unique insights into cellular metabolism and complement other “omics” sciences. The first step of metabolomics analysis is the preparation of samples (metabolite extraction from debris-free culture supernatants, washed cells or headspace of the culture vessel) followed by resolution and identification of metabolites by Liquid Chromatography (LC) or Gas Chromatography (GC) coupled with mass spectrometry (MS) or identification with nuclear magnetic resonance (NMR) (Kapoore et al., 2017) (Figure 1). Bioinformatics tools are thereafter used to connect the detected peaks (metabolites) to their identity, metabolic pathways and quantifies metabolic fluxes. The metabolomics investigations are typically of two types, untargeted and targeted. Untargeted metabolomics is an unbiased analysis measuring all detectable metabolites present in the sample (global metabolic profiling) and facilitates the discovery of new molecules impacting cell metabolism (Qiu et al., 2023). In contrast, the targeted metabolomics is a quantitative approach where a single or group of known and chemically defined metabolites (often identified using untargeted approach) are quantitated with/without 15N or 13C compounds known as labelling isotopes.
Figure 1. (Abstract Image)- A metabolomics workflow strategy to measure the differences in intracellular/extracellular metabolites of CHO cells. Sample preparation: culture cells in appropriate medium, collect the spent media and centrifuge to remove the cells/debris from spent media (if analyzing the metabolites in media) or collect cells (wash them twice), quench and extract the metabolites using analytical technique-compatible method. Discovery and validation: lyophilize the metabolites, reconstitute them before the run, and analyse using analytical techniques (LC-MS/GC-MS/NMR or in combination). Implementation: perform data overlap analysis, identify the differentially expressed metabolites/pathways and implement the knowledge to modify the process for achieving improved cellular performance in the bioreactor for higher yield.
Until the last decades, our understanding of CHO cellular metabolism and metabolomics was limited to only measurement of certain nutrient metabolites (i.e., glucose and glutamine) and establish their utilization to generation and accumulation of waste metabolites (i.e., lactate and ammonia) in culture (Zhang et al., 2016). With the early success in exploring various aspects of host-pathogen interactions and disease biology, the currently available metabolomics techniques, LC-MS, GC-MS and NMR, have now started trending to elucidate cellular metabolism(s) and mechanism(s) regulating growth and productivity with a focus. However, the primary focus of studies was to identify the optimal culture media and feed components for rational media designing; but now slowly percolating towards the intercellular metabolic profiles of requisite cellular phenotypes (fast-grower and/or high-producer, clonal stability over long term culture, etc. (Coulet et al., 2022; Torres M. et al., 2023). Therefore, in this review, we have focussed on the potential of LC-MS, GC-MS and NMR for investigating the CHO metabolome during bioprocess. We have also tried to collate and discuss the published metabolite profiles of the CHO cells at different stages of bioprocess with the desired cellular phenotypes, i.e., cell growth and recombinant protein production, along with the analytical technique to detect these metabolites. We believe this knowledge may enable the development of strategies for achieving improved metabolomic coverage and generate knowledge to increase yield from the production cultures.
LC-MS, GC-MS and NMR are the most commonly used techniques for CHO metabolomics. LC-MS provides highest metabolomic coverage with a wide range of metabolite classes and hence offers great potential to investigate CHO cells in culture (Figure 2; Table 1). Briefly, the liquid chromatography (LC) resolves metabolites from the mixture and mass spectrometry (MS) provides spectral information that identify (or confirm the suspected identity of) each separated component (Markley et al., 2017). MS is not only sensitive, but also provides selective detection, relieving the need for complete chromatographic separation. GC-MS can be used to study liquid, gaseous or solid samples and hence is of great importance to investigate CHO cells. Briefly, the compounds are propelled by an inert carrier gas such as helium, hydrogen or nitrogen in GC and metabolites are detected using MS (Danzi et al., 2023). On the other hand, NMR spectroscopy allows analysis of live cells, including intracellular pH and levels of phosphorylated intermediates, along with detection of novel compounds, monitor nutrient consumption and metabolite accumulation in mammalian cell cultures (Moco, 2022). Each of these techniques has its own bias towards a specific metabolite or class and technical advantages/disadvantages (Tables 1, 2). For example, out of 474 metabolites detected, 148 were uniquely detected by LC-MS, 80 were unique for GC-MS and 36 for NMR in CHO based intracellular and extracellular metabolomics studies (Figure 2). As expected, majority of the metabolites identified by LC-MS were polar, metabolites identified by GC-MS were small, volatile and non-polar molecules due to generation of robust and reproducible mass spectra from electron ionisation and metabolites identified by NMR were inorganic molecules (Supplementary Table S1). This indicates that all these techniques are complimentary to each other and hence, utilisation of multiple analytical techniques can increase the overall metabolomic coverage.
Figure 2. Venn diagram showing the overlap of intracellular (A) and extracellular (B) metabolites detected using LC-MS, GC–MS and NMR in different published studies. For this, the published and publicly available data sets for CHO cells were fetched and analysed.
Table 1. Generic comparison of commonly used analytical techniques for investigating CHO metabolome.
Table 2. Overlapping of metabolites detected in CHO-based bioprocess using LC-MS, GC-MSand NMR for intracellular and extracellular metabolites.
As of now, GC-MS has been utilized significantly in CHO bioprocessing for understanding the cellular behaviour in the production culture and to identify key metabolites and/or pathways that regulate high-growth, -survival and/or -productivity phenotypes. Whereas the other tools like LC-MS and NMR have been limitedly utilised in CHO bioprocess, thus its potential needs to be fully explored in order to get a better coverage of metabolites in CHO cells.
One of the most important interests of the biopharma industry is high yield at lower cost (Chusainow et al., 2009; Lai et al., 2013; Tihanyi and Nyitray, 2020). Some of the key characteristics of production cell lines to protect biopharma’s interest are: monoclonality, rapid growth, roughness, stability, higher production rate and consistency in product quality (Barnes et al., 2003; O'Flaherty et al., 2020). CHO metabolism plays key role in achieving high cell-specific productivity and is typically defined as the uptake of substrates from the culture media and feeds to utilize it as carbon and nitrogen sources (Selvarasu et al., 2012; Templeton et al., 2013; Saldanha et al., 2023). However, CHO metabolism is generally believed to be inefficient and suboptimal. The nutrients at certain concentrations lead to the intra/extra-cellular accumulation of metabolites, their intermediates, and by-products as a metabolic bottleneck in key pathways and inefficient flux distribution which may increase or decrease the cell growth, productivity and protein quality (Sellick et al., 2015; Kirsch et al., 2022). A number of studies has been done on understanding CHO bioprocess using metabolomics (Table 3) with majority of them focussing on understanding the cell growth and productivity with some on cell line development, bioprocess optimisation as discussed below.
Table 3. Representative list of key metabolites/pathways identified in CHO using metabolomics-based approaches from literature.
The glycolytic pathway and its associated pathways upregulates in the exponential phase of culture to potentially meet the increased demand of energy during cell proliferation (Sengupta et al., 2011; Templeton et al., 2013; Zhu et al., 2022; Naik et al., 2023) (Table 3; Figure 3). For example, glucose, glucose-6-phosphate, pyruvate, phosphoenolpyruvate, fructose 1,6-bisphosphate, and fructose 6-phosphate increases in the exponentially growing cells (Buchsteiner et al., 2018; Zhang et al., 2021; Coulet et al., 2022). Majority of these metabolites can be detected using GC-MS, except glucose which can be universally identified using any of the three techniques (LC-MS, GC-MS, NMR) (Supplementary Table S1). These metabolites are highly utilised in the exponential phase of cells thereby increasing the cell proliferation (Hsu et al., 2017). The metabolites of gluconeogenesis pathway (L-glutamine, UMP, deoxycytidine, orotate, acetate, glycerone phosphate) also upregulate to support the increasing demand of energy during this growth phase (Supplementary Table S1). Besides the intracellular level of UDP-glucose, detected using LC-MS, was also reported increased in growing cultures with low level of UDP-glcA due to reduced activity of UDP-glucose 6-dehydrogenase, however both are crucial for cell growth. Therefore, improving the levels of UDP-glucose 6-dehydrogenase by improved media or process design and cell engineering approaches may overcome the bottleneck of cellular metabolism for growth (Dietmair et al., 2012).
Figure 3. Metabolic overview of CHO culture representing key metabolites and pathways expressed over different phases of cell culture. Red represents downregulated metabolites/pathways, and green represents upregulated metabolites/pathways. Abbreviations: TCA- Tricarboxylic acid cycle, PPP- Pentose Phosphate Pathway, dNTPs-deoxy Nucleoside Triphosphates, Qp-cell specific productivity, AMP- Adenosine monophosphate, GMP- Guanosine monophosphate, ADP- Adenosine diphosphate, GDP- Guanosine diphosphate, dCTP- Deoxycytidine triphosphate, CTP- Cytidine triphosphate, GTP- Guanosine triphosphate, ATP- Adenosine triphosphate, NAD-Nicotinamide adenine dinucleotide, NADH- nicotinamide adenine dinucleotide (NAD) + hydrogen, GSH- Glutathione, FAD-flavin adenine dinucleotide.
The TCA cycle metabolic fluxes (succinate; oxalosuccinate, (S)-malate, citrate; pyruvate, succinic acid) remains upregulated at the stationary phase as compared to the exponential phase (Bai et al., 2011; Sengupta et al., 2011; Dietmair et al., 2012; Templeton et al., 2013; Duarte et al., 2014). Most of these metabolites were detected by both LC-MS and NMR, except fumarate and succinate which were reported to be only detected using GC-MS (Supplementary Table S1). This indicates that the cells at stationary phase mostly rely on oxidative phosphorylation pathway for ATP generation, whereas the cells at exponential phase primarily depends on glycolysis for ATP production via substrate level phosphorylation. As the growth rate decreases (stationary-phase), the metabolic-shift to utilize glucose in the TCA cycle increases to achieve high viable cell mass and antibody titres (Dean and Reddy, 2013). A significant portion of the pyruvate formed from the glucose is converted into lactate, which is secreted and acidifies the medium, with the rest being used to supply the TCA cycle thus channelising the cells into the stationary phase indicating the shift from lactate producers (glucose consumers) to lactate consumer cells (Selvarasu et al., 2012; Wijaya et al., 2021). However, contrary to glucose consumption, glutamine is also reported to be utilized more efficiently than glucose for anaplerotic replenishment of TCA intermediates and contributed more significantly to lactate production during the exponential phase (Templeton et al., 2013). Therefore, optimizing the concentration of glycolytic and TCA intermediates, (ornithine, pyridoxine and pyruvic acid in the growth medium, and citric acid and succinic acid in the feed medium) can help to regulate cell growth in culture (Saldanha et al., 2023). The significant flux of oxidative pentose phosphate pathway (oxPPP) has also been reported to be upregulated indicating additional requirement of NADPH and glutathione, which contributes in fighting oxidative stress during the stationary phase (Ahn and Antoniewicz, 2011; Sengupta et al., 2011). PPP metabolites were mostly detected using LC and GC-MS only (Supplementary Table S1). Therefore, stationary phase may be characterized by a reduced glycolysis flux, lactate uptake, low consumption of carbon and nitrogen sources, increased oxPPP flux, and reduced rate of anaplerosis.
All the 20 amino acids can be detected using all three techniques (LC, GC, NMR) in different phases of CHO cell culture; however, most of them are uniformly detected by GC-MS, followed by NMR (Supplementary Table S1). Intracellular level of a number of amino acids (glycine, serine, threonine, arginine, tyrosine, valine, aspartate and phenylalanine) are significantly lower in the stationary phase compared to the exponential phase (Lu et al., 2005; Kirsch et al., 2022). Depletion of specific amino acids, including arginine, cysteine, histidine, phenylalanine, tryptophan and pyruvate, leads to the initiation of the decline phase in the CHO cultures (Sellick et al., 2015). The depletion of eight metabolites, including glucose, glutamine, proline, serine, cystine, asparagine, choline, and hypoxanthine, from the production media of stably antibody-expressing CHO cells exerts cellular stress limiting the cell growth and supplementation of these metabolites as a nutrient cocktail result in improved peak cell density by ∼ 75% (Sellick et al., 2015). These amino acids are known to enter into catabolic processes thereby leading to their high consumption in culture, to complement the energy gain from glucose (Fan et al., 2015). Thus, decreased level of many of these amino acids in stationary phase of culture may potentially be indicating the exhaustion of other possible substrates and their utilisation for energy generation, instead of being used as building blocks in protein synthesis. However, contradictorily cysteine is reported to induce senescence and deaccelerate cell growth (Chu and Liu, 2015). Moreover, 5-hydroxy indole acetaldehyde (5-HIAAld), a tryptophan metabolite, has a strong negative correlation with peak viable cell density and hence tryptophan supplementation is also observed to have growth-inhibitory effects potentially leading to excessive accumulation of 5-HIAAld in the culture medium (Alden et al., 2020).
Nucleotides (dCTP, CTP, GTP, ATP, and NAD) also play an important role in cellular metabolism in the growth phase, acting as building blocks for the synthesis of RNA or DNA and/or cofactors in a large number of reactions (Dietmair et al., 2012). These nucleotides can be mostly detected by both LC and GC-MS (Supplementary Table S1). Contrastly, few nucleotides (ATP, AMP, GMP, ADP, GDP, adenosine) are predicted to induce growth arrest of CHO cells in the G1 phase thereby channelising them from exponential to stationary growth phase (Pereira et al., 2018). Additionally, various vitamins (choline chloride, i-inositol, niacinamide, folic acid, thiamine, pyridoxine) and hormones (choline chloride, triiodothyronine (T3) and human insulin like growth factor-I (IGF-I) enhances the culture performances through increased growth and productivity (Kim et al., 2005; Selvarasu et al., 2012).
Besides, the waste products accumulated in production culture due to inefficient metabolism also impacts the growth and productivity. Consumption of glutamine and asparagine leads to generation and accumulation of ammonia in culture which are well known to negatively affect the cell-growth, product quality and productivity (Selvarasu et al., 2012). Reduced accumulation of other growth-limiting waste metabolites (lactate and alanine) is also associated with increased culture longevity and protein productivity in hypothermia-based biphasic cultures (Wagstaff et al., 2013). Similarly, indole 3-carboxylate, 4-hydroxyphenylpyruvate, homocysteine, 2-hydroxybutyrate, lactate, Phenyl lactate, Indole 3-lactate, Formate, Isovalerate (intermediates or byproducts) of amino acid catabolism also inhibits the cell growth in fed-batch cultures of Chinese hamster ovary cells (Mulukutla et al., 2017). Controlled supplementation of some of these amino acids during the growth phase can reduce the rate of their production and accumulation in later-stage culture, improving peak-cell density and productivity from culture (Mulukutla et al., 2017). For example, reduced generation and accumulation of three branched-chain amino acids (isovalerate, isobutyrate and 2-methylbutyrate) by knocking-out the gene branched-chain amino acid transaminase 1 (BCAT1) coding an enzyme required for their production helped in retaining high culture viability (≥95%) even on the 20th day of fed-batch culture (Mulukutla et al., 2019). Minimizing the accumulation of intermediates and byproducts of the phenylalanine-tyrosine pathway by over-expressing the genes involved in their effective conversion enabled the culture to achieve higher peak-cell density and productivity (Coulet et al., 2022). Recently, the extracellular detection of L-glutamine, pyroglutamic acid, 4-hydroxyproline, choline, glucose, lactate, alanine, and proline were proposed to be the biochemical markers of the logarithmic growth phase whereas isoleucine, leucine, valine, acetate, and alanine for the stationary growth phase and acetate, glycine, glycerine, and gluconic acid for the cell decline phase.
Therefore, metabolic engineering for efficient characterization of nutrients for growth and protein production and to minimize generation and accumulation of growth limiting metabolites in culture could help to improve the cell growth and productivity (Zhao et al., 2023).
Metabolites in the TCA cycle and its intermediate/precursors (alanine, aspartate, and glutamate metabolism pathway), PPP, glutathione synthesis pathway and polyol pathway have the strongest association with qP (Dietmair et al., 2012) (Table 3; Figure 3). Significantly upregulated TCA cycle (including citric acid, isocitric acid, α-ketoglutarate and glutamate) and downregulated intracellular glycolytic pathway (i.e., 3-phosphoglycerate) with a metabolic-shift from lactate accumulation to consumption and lipid metabolic pathways (i.e., choline and phosphoryl-choline that is required for membrane phospholipids) are associated with reduced growth and increased cell-specific and overall productivity (Zhu et al., 2022).
Increased intracellular levels of three activated sugar precursors (GDP-fucose, UDP-Gal/UDP-Glc, and UDP-GlcNAc) potentially associated with protein glycosylation, have been reported in CHO cells with increased qP for mAbs (Chong et al., 2012). These sugar precursors can be majorly detected using GC-MS except few (UDP-Gal, UDP-galactose, UDP-glucose) which were uniquely identified in studies performed using GC-MS, whereas NMR was unable to detect these polar metabolites (Supplementary Table S1). An efficient glycometabolism for protein production allows less glucose uptake by cells (Zhang et al., 2021). Besides, reduction of lactate levels in CHO cell cultures improves the product titre by 11%–32% without having significant impact on cell growth suggesting that reduced level of lactate in culture potentially either due to its consumption or inhibited generation may be the primary cause of its beneficial effects (Ahn and Antoniewicz, 2011; Naik et al., 2023).
Enhanced TCA activity is the predominant distinguishing feature between high and low-producer CHO cell lines (Saldanha et al., 2023). Supplementation of TCA intermediates, or their precursors (aspartate, glutamate, succinic acid, malic acid, fumaric acid) in the stationary phase of culture increases cell growth and mAb production by > 50% without affecting its quality (Saldanha et al., 2023). These TCA cycle intermediates can be mostly detected using LC-MS except fumaric acid which is observed to be uniquely detected by GC-MS (Supplementary Table S1). Moreover, supplementation of TCA intermediate, citrate, alone was observed to increase the qP by up to 490% and more than doubled the titre (Yao et al., 2021); this might be because of the amount of substrate available for the TCA cycle is increased and further, citrate also promote iron chelation that ultimately led to increased qP (Bai et al., 2011; Zhang et al., 2020). Similarly, aspartate was also found to increase the recombinant protein production (Yao et al., 2021). Besides, intracellular and extracellular levels of mitochondrial metabolites (sorbitol, glycerol, threitol) and polyol pathway (high glucose, fructose, sorbitol) are increased in cells with higher cell-specific productivity indicating the cellular need for increased citric acid cycle function and mitochondrial oxidative capacity to achieve higher productivity and is a characteristic for high-producing CHO cell lines (Templeton et al., 2013; Sellick et al., 2015; Templeton et al., 2017).
Addition of a few amino acids (glycine, methionine, phenylalanine, threonine, tyrosine) also increases the productivity of CHO cells by inhibiting their growth. Their accumulation in the culture medium during the growth phase mediates the transition of exponential to stationary phase. These metabolites inhibit the pyruvate kinase which produces ammonia through pyruvate transamination during late stages of culture (Wijaya et al., 2021; Pereira et al., 2018). The amino acids, glutamine and asparagine present inside the cell milieu has often been correlated with the productivity and healthiness of the cells in culture (Wishart et al., 2009; Mohmad-Saberi et al., 2013). However, increased glutamine supplementation also elevates lactate, alanine, and ammonia fluxes during the early exponential phase, which is known to impact growth and productivity in cultures through TCA cycle (Kirsch et al., 2022). Therefore, a balanced supplementation of glutamine in the media is recommended to support the elevated level of glutathione, nucleotides and nucleotide sugars (such as UDP-GlcNAc) that has been associated with increased productivity. Depletion of histidine, which is involved in folding and assembly of newly synthesised proteins, from the culture media was observed to significantly decrease the production of recombinant antibody without significantly impacting the CHO cell growth and hence increased availability of histidine in the culture medium may improve the productivity (Lu et al., 2006; Ladiwala et al., 2023). Contrarily reduction of growth and productivity inhibitory metabolites (Lysine, Isoleucine, Tryptophan, Leucine and Arginine) in medium decreased the accumulation of inhibitory metabolites and improved growth and IgG production in the batch and fed-batch processes (Ladiwala et al., 2023). Similarly, production and accumulation of eight metabolic derivatives including aconitic acid, 2-hydroxyisocaproic acid, methyl succinic acid, cytidine monophosphate, trigonelline, and n-acetyl putrescine, in culture media due to inefficient cell metabolism were reported to reduce cell growth (∼27%) and productivity (∼40%) besides inhibiting the formation of mono-galactosylated biantennary (G1F) and biantennary galactosylated (G2F) N-glycans of the antibody (Kuang et al., 2021). Further, these accumulating metabolites are product associated with branched-chain amino acids, tryptophan, nicotinamide and polyamine pathways (Harrington et al., 2021).
Intracellular NAD+/NADH ratio, intracellular NADPH, FAD and glutathione metabolic pathways are also upregulated which might be reasoned to the fact that NADPH is a vital cofactor and crucial redox partner in various cellular reactions and contribute to anabolic reactions including pathways of citric acid cycle, oxidative phosphorylation, glutathione metabolism, and protein glycosylation (Chong et al., 2012; Hosios and Vander Heiden, 2018) affecting the overall qP. Besides, the pentose phosphate pathway is also upregulated, potentially fulfilling the increased demand of energy (NADPH) of cells with qP (Buchsteiner et al., 2018).
Taken together from LC-MS, GC-MS and NMR-based metabolomics, the higher availability of metabolites involved in TCA cycle and amino acids are vital to support the cell growth while the upregulation of metabolites of the TCA cycle, polyol pathway, mitochondrial oxidative capacity and glutathione metabolism during the stationary phase of culture are important for both, cell growth and productivity, in CHO cell culture.
Recently, distinct metabolomic signatures (increased consumption of glucose, amino acids, accumulation of greater amounts of lactate and TCA cycle intermediates) were identified for long-term cultured (long-passage) cells which typically adapt metabolome towards cell proliferation and survival instead for productivity (product yield and quality) compared early-passaged cells (Torres and Dickson, 2022; Kaur et al., 2023). Metabolic characterization of CHO cells also led to identification of an additional phase called the cell size increase (SI) phase that occurs between the exponential proliferation phase (also called number increase (NI) phase) and the stationary phase, during which the cell division comes to a halt but the cell growth continues in the form of an increase in cell size, thereby increasing average volume and dry weight per cell by threefold with time (Pan et al., 2017). The average mAb specific productivity per cell increases linearly with the cell volume curating two times higher in the SI phase than NI phase. Accumulation of fatty acids and formation of lipid droplets in the cells are observed during the SI phase, indicating that the fatty acids synthesis rate exceeds the demand for the synthesis of membrane lipids (Pan et al., 2017). The CHO cells showed increased under hydrostatic pressure (≥60 mmHg) cell-specific glucose consumption rate, cell-specific lactate production rate and cell-specific ammonium production rate under hydrostatic pressure (≥60 mmHg) compared to 0 mmHg. However, an increase cell proliferation and productivity observed under ∼30 mmHg (Shang et al., 2021). Besides, metabolomics of different CHO cell line (CHO-K1, CHO-GS, CHO-S and CHO-DG44) reflect a clear heterogeneity among them, with each having some unique metabolites being detected (Supplementary Table S1), which may be either due to the difference in their culture media used during investigation, expressing different product and/or process design. More interestingly, no intracellular metabolite has been observed to be significantly differentially expressed to connect the scale-up effect among 10L, 100L and 1,000L bioreactor cultures (Vodopivec et al., 2019).
Metabolomics, as of now, has primarily been utilized to evaluate the effects of growth medium composition on cell growth and productivity and hence, facilitated rational medium design leading to significant improvements in CHO-based bioprocessing and thereof yield. However, there is much more to be explored by incorporating the recent advancement in current metabolomic tools for extracellular as well as intracellular metabolite analysis which may not be feasible without fixing the currently existing technical gaps in the metabolomics pipeline as detailed below-
Each metabolomic technique has its own biasness towards specific metabolites or class of metabolites depending upon their physicochemical properties (polar, lipids, organic acid, etc.) (Table 2). For instance, LC-MS is more suited for the identification of large, polar, ionic, or non-volatile, thermally unstable metabolites (e.g., most amino acids, NAD, NAG, FAD, etc.), GC-MS is more suited to volatile organic compounds, short-chain fatty acids, sugars and hydroxyl acids (e.g., putrescine, tetradecane, 2-hydroxycaproic acid, etc.) (Supplementary Table S1). And, NMR is preferred when sample treatment or chemical derivatization is not desired and to detect specific inorganic metabolites/ions and protein-bound metabolites which are not detected or distinguishable by MS techniques (e.g., leucine, isoleucine, etc (Behera et al., 2020).
Although, LC-MS-based approaches detect the highest number of metabolites (>4000 features) in a cell and have been proven the most feasible approach for omics-based studies, but its spectral libraries are smaller in size (8,171 compounds in NIST14 library, 12,099 compounds in Metlin library, lacking retention information). Whereas, the NIST14 library for GC-MS comprises mass spectra for 242,477 unique compounds, of which roughly one-third have records for standardized retention times. An ultra-high performance metabolomics platform can detect up to 7,000 metabolic features in a typical application (Dunn et al., 2011). However, the majority of the studies as of now have been only able to identify a lesser number (∼350 metabolites), suggesting that the analytical coverage of a metabolome is far from achieving the expected range and thereby might miss the critical metabolites that actually regulate cell growth and productivity in culture.
Different metabolite quenching and extraction methods have biased towards different classes of compounds (amino acids, carbohydrates, nucleotides, sugars, etc.). Hence, cataloguing methods with respect to their biasness towards metabolite extraction for general use can facilitate the detection of a larger range of metabolites or help in the quantification of a specific/targeted metabolite(s) (Kumar et al., 2022; Singh et al., 2023). For example, the extraction of metabolites using 100% methanol extraction followed by water was found to be most effective for the recovery of the largest range of metabolites in CHO cells by GC-MS (Sellick et al., 2010). Derivatisation with propyl chloroformate/propanol obtains excellent extraction of amino acid analytes (Batista et al., 2023). The recovery of fatty acids (e.g., stearic acid and palmitic acid) was maximized by hot ethanol extraction, while the recovery of glycerol-1-phosphate was significantly greater when cellular metabolites were extracted with KOH (Park et al., 2021). On the other hand, polar metabolites are extracted with cold methanol + MSTFA (N-Methyl-N-trifluoroacetamide) + 1% TMCS (trimethylchlorosilane); organic acids, ketoacids by BSTFA (N,O-Bis(trimethylsilyl)trifluoroacetamide) and bile acids by methanol +2% sulfuric acid + MSTFA +1% TMCS for NMR (Bouatra et al., 2013).
Several studies have used metabolomics to compare the metabolic characteristics of high-growth and/or high-productivity cell lines to identify metabolites that accumulate in the cell or culture and inhibit growth (Figures 4A, D) and/or productivity. However, these studies vary in terms of CHO cell hosts (and clone) (Figures 4B, E), medium (and feed) (Figures 4C, F), type of recombinant protein being expressed and culture conditions limiting its application in other systems. As a result, it is unclear whether the metabolites identified in these studies are only relevant to a particular culture media, product and/or cell line, as each cell line may have different host cell proteins and metabolome under different media. Whereas, the need of the hour is the identification of more general/universal growth and/or productivity indicators that can be applied to multiple hosts and culture media for improved clone selection and/or process designing.
Figure 4. Venn diagrams showing overlap of intracellular and extracellular metabolites in CHO cultures identified using LC-MS, GC-MS and NMR. (A, D) represents data of intracellular and extracellular metabolites for different growth phases, (B, E) represents data of intracellular and extracellular metabolites for cell lines, (C, F) represents data of intracellular and extracellular metabolites for culture media and (G) represents data of intracellular and extracellular metabolites for Venn diagram is presented with the overlapping regions corresponding to the number of expressed metabolites present in more than one parameter type. The central region corresponds to the expressed metabolites present in all conditions of the same parameter.
There have been a few significant technical advancements in the domain of metabolomics and in the understanding of the metabolomics pipeline that may facilitate better designing of future studies to investigate CHO cell behaviour in culture and get requisite knowledge to better regulate mammalian bioprocess for improved protein productivity.
High-resolution mass spectrometry (HRMS) is an evolving method in CHO metabolomics that offers high accuracy and sensitivity in the detection of isobaric metabolite detection (Castelli et al., 2022). Isobaric metabolites represent the same molecular weight but different structures. This is a significant advantage over traditional MS methods, which cannot differentiate between isobaric compounds. Using HRMS, the glucose and galactose were reported to have a significant impact on CHO cell metabolism, while other sugars (such as GDP-Fucose, UDP-Glc/UDP-Gal, UDP-GlcNAc/UDP-GalNAc) had no or minor effect (Durrant et al., 2020). HRMS can also be utilized to analyse glycosaminoglycans (GAGs), which are complex carbohydrates that play a critical role in various biological processes (Torres CL. et al., 2023). Isotope labelling is another evolving method in CHO metabolomics which involves the incorporation of stable isotopes such as 13C and 15N into carbohydrates, for tracking the in vivo fate of carbohydrates in various metabolic diseases such as diabetes and obesity (Marin et al., 2004). This method shall be applied to study carbohydrate/amino acid’s metabolism in CHO-based bioprocessing also. Advancements in NMR techniques like 2D NMR also presents a chance to uncover signals that are overlapping in 1D NMR spectroscopy due to similar resonant frequencies and hence discover metabolites that were otherwise not resolved by 1D NMR (Mahrous and Farag, 2015). Besides, an emerging technique, Mass Spectrometry Imaging (MSI), is also a highly futuristic tool which enables untargeted investigations of a variety of samples sectioned into different spatial distributions (Liao et al., 2023). It has a capability to image thousands of molecules, such as metabolites, lipids, peptides, proteins, and glycans, in a single experiment without labelling. The information gained from MS and MSI can also be combinedly used for analysis and characterisation of biological samples.
An approach of combining multiple analytical platforms (NMR and MS) can be advantageous in order to improve the metabolome coverage. Integration of GC–MS, LC-MS and NMR has been shown to increase the metabolomics coverage of cells in culture (Bhinderwala et al., 2018; Fei et al., 2019; Zeki et al., 2020). Combining NMR and MS for metabolomics by using small chemical compound-treatments of Chlamydomonas reinhardtii, identified a total of 122 metabolites were detected (82 by GC-MS, 20 by NMR, and 20 by both); more than any single technique identified (Bhinderwala et al., 2018). Metabolomics of human urine samples using analytical platforms (NMR, GC-MS, LC-MS, ICP-MS and HPLC) identified a total of 445 metabolites consisting 209 by NMR, 179 by GC-MS, 12 by DFI/LC-MS/MS, 40 by ICP-MS and 10 by HPLC (Bouatra et al., 2013). Combining NMR with MS was reported to identify 21 altered metabolites between cancer and healthy controls, of which 13 were first time reported (Zhong et al., 2022). Combined NMR and MS also have advantages for isotope tracing and metabolic flux analysis (Shi et al., 2020). MS generally quantifies isotopic labelling distributions; even with MS/MS, it often does not give the specific labelling position, which can be detected using NMR. Besides, utilizing different methods of sample preparation/metabolite extraction has also been reported to improve metabolomic coverage. For example, in LC-MS of Klebsiella pneumoniae, 151 metabolites were identified in sample with metabolite extracted using Freeze thaw cycle (FTC), 103 by sonication cycle (SC) method and 132 metabolites by both (FTC + SC), enabling cumulative identification of 199 unique metabolites; more than any of the single method identified (Kumar et al., 2022). Similarly, in LC-MS of Staphylococcus aureus, a total of 116, 119, and 99 metabolites were identified in samples with metabolite extracted using the FTC, SC, and FTC + SC methods, respectively, leading to the identification of 163 metabolites cumulatively (Singh et al., 2023). This indicates that each method of metabolite extraction also has its own biasness (Sowa et al., 2020; Singh et al., 2023). Therefore, utilizing multiple methods of metabolite extraction with multiple analytical methods (LC-MS/GC-MS and NMR) will present a clearer and more accurate picture of the metabolic profile due to increased metabolomic coverage and help to overcome the limitations of individual techniques/methods.
There is an urgent need for improved and user-friendly bioinformatics tools to analyse metabolomic data and integrate this knowledge with other ‘omic’ approaches. As of now, data driven mathematical modelling has proven beneficial for optimizing media composition, culture parameters, metabolites, growth and productivity from the CHO cell lines (Galleguillos et al., 2017). Commonly used modelling approaches performs non-steady-state kinetics based metabolic flux analysis (MFA) and flux balance analysis (FBA) using system biology and machine learning (Nolan and Lee, 2012). Recently, a model constructed using production rates of metabolites as a function of specific growth rates from a 2L small-scale culture as training set were observed to identify metabolic phases and predict cell metabolism and productivity of 2000L production scale (Ben Yahia et al., 2017). However, data-driven models are challenging due to data scarcity and heterogeneity and may be of limited use to extrapolate beyond the training set (Danzi et al., 2023; Gong et al., 2023). Hence, hybrid models combining fundamental kinetics with data-driven approach were developed which included impurities generated by the cell (host cell proteins and DNA content released due to cell death) during the process along with description of cell viability, mAb production, glucose, and lactate concentrations (Okamura et al., 2022). The model was observed to yield higher accuracy than both the kinetic or statistical modules alone. Genome-scale metabolic models (GeMs), which integrate the knowledge from genomics, transcriptomics, epigenomics, proteomics and metabolomics, provide detailed information about biochemical reactions networks that compose cellular metabolism and offers potential to connect molecular networks to the observed phenotype (Rejc et al., 2017). In the line, a number of metabolic pathways were reconstructed and associated with >1,700 genes in CHO genome as a resource for GeM to predict the growth and productivity characteristics of the CHO cells (Hefzi et al., 2016). A GeM analysis of a wild-type and antibody producer CHO cell line revealed extensive transcriptional re-wiring of DNA damage repair and cellular metabolism with the genomic data, supported by substantial increase in energy metabolism in the producer cells by the transcriptomic data and elevated long chain lipid species (potentially facilitating the protein transport and secretion requirements) by metabolomic data establishing that observation from different omics data sets to be significantly overlapping and complementary (Yusufi et al., 2017). GeMs constructed using unconventional uptake-rate objective functions (primarily considering the availability of CHO-specific essential nutrients in the media) instead of conventional biomass objective function have been shown to be more accurate and able to metabolically distinguish different CHO cells compared to predictions based on conventional biomass objective functions (Chen et al., 2019). Recently, CHOmpact, a reduced metabolic model, was reported to deploy robust and nonlinear optimization, compute physiologically consistent flux distributions and enhanced interpretability of simulation (Jiménez del Val et al., 2023). However, implementation of GeMs based approaches in CHO based bioprocess is still limited. In overall, as of now, no single method or approach is comprehensive, but rather, complementary.
More targeted efforts are required to investigate the intracellular metabolites of the most effective cell phenotype in the bioprocess as extracellular metabolic profiles alone typically fail to explain cellular behaviour (high-growth or -productivity) in the production culture (Dietmair et al., 2012). A total of 107 unique intracellular metabolites, 77 unique extracellular metabolites and 83 common metabolites have been reported in different CHO based metabolomics studies performed using LC-MS, GC-MS and NMR (Figure 4G). The intracellular metabolite enrichment could be due to their uptake from culture media as it is (nutrient) or as its precursor. Therefore, analysing only extracellular or intracellular metabolome may remain unable in elucidating the detailed metabolomic process suggesting their complementarity. Integrating quantitative extracellular metabolomic profiles with intracellular metabolic profiles and flux states might enable to better understand the metabolomic variations and candidate flux distributions in CHO bioprocess. Hence, intracellular and extracellular metabolic profiling shall be performed together to achieve a holistic picture of metabolomic pathways working together.
CHO cells are the most preferred cell lines for industrial production of protein biotherapeutics. Significant improvement in the yield of such products has been witnessed over time, primarily because of improved media and bioprocess design. However, these products still remain costly and hence demand further improvement in the performance of CHO cells in the bioprocess. This could only be achieved by improving our understanding of the different cellular phenotypes, good/bad-grower and/or producer CHOs, in the culture.
Metabolomics is a promising approach in the bioproduction field, as it detects the downstream products (metabolites) of the other ‘omics’ sciences - genomics, transcriptomics and proteomics and is believed to mirror the cellular phenotype more accurately. Despite the limited utilization in the domain, metabolomics has contributed significantly in designing the media and feed to achieve improved cell growth and overall productivity. Initially, increased consumption of glucose and glutamine were known to increase generation and accumulation of lactate and ammonia in culture that are well-known to inhibit the cell-growth and productivity in culture. However, overtime, with increasing efforts of investigating CHO metabolome, our knowledge of cell metabolism in bioprocess has improved and as a result, several metabolites and pathways that are associated with regulation of cell growth and productivity in culture, have been identified. For example, increased glycolytic pathway is shown to support the cell growth in exponential phase, whereas increased TCA cycle, oxidative phosphorylation and glutathione pathways have been shown to slow-down cell growth and -productivity in CHO bioprocess. The availability of amino acids, specifically aspartate, citrate and histidine, has been shown to be associated with cell-specific productivity in culture. Recent technical advancement and our experience with the technology suggest that metabolomics can help in identifying not only extracellular growth- and/or productivity-regulating metabolites to further improve culture media and feed but also intracellular metabolites and pathways that actually performs to achieve improved growth and productivity from production culture, providing the targets for rational cell engineering. However, multiple analytical and sample preparation methods must be employed to achieve higher metabolomic coverage and minimize the chances of missing out the identification of key-regulator metabolites during metabolomics-based investigations. Taken together, metabolomics-based investigations offer great potential in improving CHO-based bioprocesses’ performance and hence demand more profound investigation.
RS: Conceptualization, Data curation, Formal Analysis, Investigation, Methodology, Software, Validation, Visualization, Writing–original draft, Writing–review and editing. EF: Data curation, Writing–review and editing. LT: Writing–review and editing. SS: Writing–review and editing. CR: Writing–review and editing. NK: Conceptualization, Funding acquisition, Project administration, Resources, Supervision, Visualization, Writing–review and editing.
The author(s) declare that no financial support was received for the research, authorship, and/or publication of this article.
We are very grateful to Professor Jayanta Bhattacharya (Executive Director (Additional charge, THSTI, Faridabad) and Mr. M.V. Santo (Head Administration), Shailesh Kumar and Manoj Kumar (Lab Technicians-MCTR) for their support and Council of Scientific and Industrial Research (CSIR), Government of India for providing the fellowship to RS for persual of Doctoral degree. EF received fellowship from THSTI. LT received fellowship from Department of Biotechnology (DBT), SS received fellowship from Department of Biotechnology (DBT), CR received fellowship from THSTI, Government of India and THSTI for support.
The authors declare that the research was conducted in the absence of any commercial or financial relationships that could be construed as a potential conflict of interest.
All claims expressed in this article are solely those of the authors and do not necessarily represent those of their affiliated organizations, or those of the publisher, the editors and the reviewers. Any product that may be evaluated in this article, or claim that may be made by its manufacturer, is not guaranteed or endorsed by the publisher.
The Supplementary Material for this article can be found online at: https://www.frontiersin.org/articles/10.3389/fbioe.2024.1347138/full#supplementary-material
Ahn, W. S., and Antoniewicz, M. R. (2011). Metabolic flux analysis of CHO cells at growth and non-growth phases using isotopic tracers and mass spectrometry. Metab. Eng. 13 (5), 598–609. doi:10.1016/j.ymben.2011.07.002
Alden, N., Raju, R., McElearney, K., Lambropoulos, J., Kshirsagar, R., Gilbert, A., et al. (2020). Using metabolomics to identify cell line-independent indicators of growth inhibition for Chinese hamster ovary cell-based bioprocesses. Metabolites 10 (5), 199. doi:10.3390/metabo10050199
Aranibar, N., Borys, M., Mackin, N. A., Ly, V., Abu-Absi, N., Abu-Absi, S., et al. (2011). NMR-based metabolomics of mammalian cell and tissue cultures. J. Biomol. NMR 49 (3-4), 195–206. doi:10.1007/s10858-011-9490-8
Bai, Y., Wu, C., Zhao, J., Liu, Y. H., Ding, W., and Ling, W. L. W. (2011). Role of iron and sodium citrate in animal protein-free CHO cell culture medium on cell growth and monoclonal antibody production. Biotechnol. Prog. 27 (1), 209–219. doi:10.1002/btpr.513
Barnes, L. M., Bentley, C. M., and Dickson, A. J. (2003). Stability of protein production from recombinant mammalian cells. Biotechnol. Bioeng. 81 (6), 631–639. doi:10.1002/bit.10517
Batista, J. M., Neves, M. J., Menezes, H. C., and Cardeal, Z. L. (2023). Evaluation of amino acid profile by targeted metabolomics in the eukaryotic model under exposure of benzo[a]pyrene as the exclusive stressor. Talanta 265, 124859. doi:10.1016/j.talanta.2023.124859
Behera, S. P., Dubey, A., Chen, W. N., De Paula, V. S., Zhang, M., Sgourakis, N. G., et al. (2020). Nearest-neighbor NMR spectroscopy: categorizing spectral peaks by their adjacent nuclei. Nat. Commun. 11 (1), 5547. doi:10.1038/s41467-020-19325-4
Ben Yahia, B., Gourevitch, B., Malphettes, L., and Heinzle, E. (2017). Segmented linear modeling of CHO fed-batch culture and its application to large scale production. Biotechnol. Bioeng. 114 (4), 785–797. doi:10.1002/bit.26214
Bhinderwala, F., Wase, N., DiRusso, C., and Powers, R. (2018). Combining mass spectrometry and NMR improves metabolite detection and annotation. J. Proteome Res. 17 (11), 4017–4022. doi:10.1021/acs.jproteome.8b00567
Bollati-Fogolı, M. (2008). “Cloning and expression of heterologous proteins in animal cells,” in Animal cell technology (Online as ebook ISBN 9780203895160: Taylor and Francis), 77–112.
Bouatra, S., Aziat, F., Mandal, R., Guo, A. C., Wilson, M. R., Knox, C., et al. (2013). The human urine metabolome. PLOS ONE 8 (9), e73076. doi:10.1371/journal.pone.0073076
Bradley, S. A., Ouyang, A., Purdie, J., Smitka, T. A., Wang, T., and Kaerner, A. J. J. A. C. S. (2010). Fermentanomics: monitoring mammalian cell cultures with NMR spectroscopy. J. Am. Chem. Soc. 132 (28), 9531–9533. doi:10.1021/ja101962c
Buchsteiner, M., Quek, L. E., Gray, P., and Nielsen, L. K. (2018). Improving culture performance and antibody production in CHO cell culture processes by reducing the Warburg effect. Biotechnol. Bioeng. 115 (9), 2315–2327. doi:10.1002/bit.26724
Castelli, F. A., Rosati, G., Moguet, C., Fuentes, C., Marrugo-Ramírez, J., Lefebvre, T., et al. (2022). Metabolomics for personalized medicine: the input of analytical chemistry from biomarker discovery to point-of-care tests. Anal. Bioanal. Chem. 414 (2), 759–789. doi:10.1007/s00216-021-03586-z
Chen, Y., McConnell, B. O., Gayatri Dhara, V., Mukesh Naik, H., Li, C. T., Antoniewicz, M. R., et al. (2019). An unconventional uptake rate objective function approach enhances applicability of genome-scale models for mammalian cells. NPJ Syst. Biol. Appl. 5, 25. doi:10.1038/s41540-019-0103-6
Chong, W. P. K., Goh, L. T., Reddy, S. G., Yusufi, F. N. K., Lee, D. Y., Wong, N. S. C., et al. (2009). Metabolomics profiling of extracellular metabolites in recombinant Chinese Hamster Ovary fed-batch culture. Rapid Commun. Mass Spectrom. 23 (23), 3763–3771. doi:10.1002/rcm.4328
Chong, W. P., Reddy, S. G., Yusufi, F. N., Lee, D. Y., Wong, N. S., Heng, C. K., et al. (2010). Metabolomics-driven approach for the improvement of Chinese hamster ovary cell growth: overexpression of malate dehydrogenase II. J. Biotechnol. 147 (2), 116–121. doi:10.1016/j.jbiotec.2010.03.018
Chong, W. P., Yusufi, F. N., Lee, D. Y., Reddy, S. G., Wong, N. S., Heng, C. K., et al. (2011). Metabolomics-based identification of apoptosis-inducing metabolites in recombinant fed-batch CHO culture media. J. Biotechnol. 151 (2), 218–224. doi:10.1016/j.jbiotec.2010.12.010
Chong, W. P., Thng, S. H., Hiu, A. P., Lee, D. Y., Chan, E. C., and Ho, Y. S. (2012). LC-MS-based metabolic characterization of high monoclonal antibody-producing Chinese hamster ovary cells. Biotechnol. Bioeng. 109 (12), 3103–3111. doi:10.1002/bit.24580
Chu, P.-Y., and Liu, M.-Y. (2015). Amino acid cysteine induces senescence and decelerates cell growth in melanoma. J. Funct. Foods 18, 455–462. doi:10.1016/j.jff.2015.08.010
Chusainow, J., Yang, Y. S., Yeo, J. H., Toh, P. C., Asvadi, P., Wong, N. S., et al. (2009). A study of monoclonal antibody-producing CHO cell lines: what makes a stable high producer? Biotechnol. Bioeng. 102 (4), 1182–1196. doi:10.1002/bit.22158
Coulet, M., Kepp, O., Kroemer, G., and Basmaciogullari, S. (2022). Metabolic profiling of CHO cells during the production of biotherapeutics. Cells 11 (12), 1929. doi:10.3390/cells11121929
Danzi, F., Pacchiana, R., Mafficini, A., Scupoli, M. T., Scarpa, A., Donadelli, M., et al. (2023). To metabolomics and beyond: a technological portfolio to investigate cancer metabolism. Signal Transduct. Target Ther. 8 (1), 137. doi:10.1038/s41392-023-01380-0
Dean, J., and Reddy, P. (2013). Metabolic analysis of antibody producing CHO cells in fed-batch production. Biotechnol. Bioeng. 110 (6), 1735–1747. doi:10.1002/bit.24826
Dietmair, S., Hodson, M. P., Quek, L.-E., Timmins, N. E., Chrysanthopoulos, P., Jacob, S. S., et al. (2012). Metabolite profiling of CHO cells with different growth characteristics. Biotechnol. Bioeng. 109 (6), 1404–1414. doi:10.1002/bit.24496
Donaldson, J., Kleinjan, D.-J., and Sjcoib, R. (2022). Synthetic biology approaches for dynamic CHO cell engineering. Curr. Opin. Biotechnol. 78, 102806. doi:10.1016/j.copbio.2022.102806
Duarte, T. M., Carinhas, N., Barreiro, L. C., Carrondo, M., Alves, P. M., and Teixeira, A. P. (2014). Metabolic responses of CHO cells to limitation of key amino acids. Biotechnol. Bioeng. 111 (10), 2095–2106. doi:10.1002/bit.25266
Dunn, W. B., Broadhurst, D., Begley, P., Zelena, E., Francis-McIntyre, S., Anderson, N., et al. (2011). Procedures for large-scale metabolic profiling of serum and plasma using gas chromatography and liquid chromatography coupled to mass spectrometry. Nat. Protoc. 6 (7), 1060–1083. doi:10.1038/nprot.2011.335
Durrant, C., Fuehring, J. I., Willemetz, A., Chrétien, D., Sala, G., Ghidoni, R., et al. (2020). Defects in galactose metabolism and glycoconjugate biosynthesis in a UDP-glucose pyrophosphorylase-deficient cell line are reversed by adding galactose to the growth medium. Int. J. Mol. Sci. 21 (6), 2028. doi:10.3390/ijms21062028
Fan, Y., Jimenez Del Val, I., Müller, C., Wagtberg Sen, J., Rasmussen, S. K., Kontoravdi, C., et al. (2015). Amino acid and glucose metabolism in fed-batch CHO cell culture affects antibody production and glycosylation. Biotechnol. Bioeng. 112 (3), 521–535. doi:10.1002/bit.25450
Fei, Q., Wang, D., Jasbi, P., Zhang, P., Nagana Gowda, G., Raftery, D., et al. (2019). Combining NMR and MS with chemical derivatization for absolute quantification with reduced matrix effects. Anal. Chem. 91 (6), 4055–4062. doi:10.1021/acs.analchem.8b05611
Floris, P., McGillicuddy, N., Morrissey, B., Albrecht, S., Kaisermayer, C., Hawe, D., et al. (2019). A LC–MS/MS platform for the identification of productivity markers in industrial mammalian cell culture media. Process Biochem. 86, 136–143. doi:10.1016/j.procbio.2019.08.014
Galleguillos, S. N., Ruckerbauer, D., Gerstl, M. P., Borth, N., Hanscho, M., and Zanghellini, J. (2017). What can mathematical modelling say about CHO metabolism and protein glycosylation? Comput. Struct. Biotechnol. J. 15, 212–221. doi:10.1016/j.csbj.2017.01.005
Gong, Y., Ding, W., Wang, P., Wu, Q., Yao, X., and Yang, Q. (2023). Evaluating machine learning methods of analyzing multiclass metabolomics. J. Chem. Inf. Model. 63 (24), 7628–7641. doi:10.1021/acs.jcim.3c01525
Harrington, C., Jacobs, M., Bethune, Q., Kalomeris, T., Hiller, G. W., and Mulukutla, B. C. (2021). Production of butyrate and branched-chain amino acid catabolic byproducts by CHO cells in fed-batch culture enhances their specific productivity. Biotechnol. Bioeng. 118 (12), 4786–4799. doi:10.1002/bit.27942
Hefzi, H., Ang, K. S., Hanscho, M., Bordbar, A., Ruckerbauer, D., Lakshmanan, M., et al. (2016). A consensus genome-scale reconstruction of Chinese hamster ovary cell metabolism. Cell Syst. 3 (5), 434–443.e8. doi:10.1016/j.cels.2016.10.020
Hosios, A. M., and Vander Heiden, M. G. (2018). The redox requirements of proliferating mammalian cells. J. Biol. Chem. 293 (20), 7490–7498. doi:10.1074/jbc.tm117.000239
Hsu, H.-H., Araki, M., Mochizuki, M., Hori, Y., Murata, M., Kahar, P., et al. (2017). A systematic approach to time-series metabolite profiling and RNA-seq analysis of Chinese hamster ovary cell culture. Sci. Rep. 7 (1), 43518. doi:10.1038/srep43518
Huang, Z., Xu, J., Yongky, A., Morris, C. S., Polanco, A. L., Reily, M., et al. (2020). CHO cell productivity improvement by genome-scale modeling and pathway analysis: application to feed supplements. Biochem. Eng. J. 160, 107638. doi:10.1016/j.bej.2020.107638
Jendoubi, T. (2021). Approaches to integrating metabolomics and multi-omics data: a primer. J. Metab. 11 (3), 184. doi:10.3390/metabo11030184
Jiménez del Val, I., Kyriakopoulos, S., Albrecht, S., Stockmann, H., Rudd, P. M., Polizzi, K. M., et al. (2023). CHOmpact: a reduced metabolic model of Chinese hamster ovary cells with enhanced interpretability. Biotechnol. Bioeng. 120 (9), 2479–2493. doi:10.1002/bit.28459
Kaisermayer, C., Reinhart, D., Gili, A., Chang, M., Aberg, P.-M., Castan, A., et al. (2016). Biphasic cultivation strategy to avoid Epo-Fc aggregation and optimize protein expression. J. Biotechnol. 227, 3–9. doi:10.1016/j.jbiotec.2016.03.054
Kang, S., Zhang, Z., Richardson, J., Shah, B., Gupta, S., Huang, C.-J., et al. (2015). Metabolic markers associated with high mannose glycan levels of therapeutic recombinant monoclonal antibodies. J. Biotechnol. 203, 22–31. doi:10.1016/j.jbiotec.2015.03.002
Kapoore, R. V., Coyle, R., Staton, C. A., Brown, N. J., and Vaidyanathan, S. (2017). Influence of washing and quenching in profiling the metabolome of adherent mammalian cells: a case study with the metastatic breast cancer cell line MDA-MB-231. J. Anal. 142 (11), 2038–2049. doi:10.1039/c7an00207f
Kaur, R., Jain, R., Budholiya, N., and Rathore, A. S. (2023). Long term culturing of CHO cells: phenotypic drift and quality attributes of the expressed monoclonal antibody. J. Biotechnol. Lett. 45 (3), 357–370. doi:10.1007/s10529-023-03346-2
Kim, D. Y., Lee, J. C., Chang, H. N., and Oh, D. J. (2005). Effects of supplementation of various medium components on Chinese hamster ovary cell cultures producing recombinant antibody. Cytotechnology 47 (1), 37–49. doi:10.1007/s10616-005-3775-2
Kirsch, B. J., Bennun, S. V., Mendez, A., Johnson, A. S., Wang, H., Qiu, H., et al. (2022). Metabolic analysis of the asparagine and glutamine dynamics in an industrial Chinese hamster ovary fed-batch process. Biotechnol. Bioeng. 119 (3), 807–819. doi:10.1002/bit.27993
Kuang, B., Dhara, V. G., Hoang, D., Jenkins, J., Ladiwala, P., Tan, Y., et al. (2021). Identification of novel inhibitory metabolites and impact verification on growth and protein synthesis in mammalian cells. Metab. Eng. Commun. 13, e00182. doi:10.1016/j.mec.2021.e00182
Kumar, A., Singh, S., Gupta, S. K., Kumar, S., Kumar, S., Singh, R., et al. (2022). Identification of metabolite extraction method for targeted exploration of antimicrobial resistance associated metabolites of Klebsiella pneumoniae. Sci. Rep. 12 (1), 8939. doi:10.1038/s41598-022-12153-0
Ladiwala, P., Dhara, V. G., Jenkins, J., Kuang, B., Hoang, D., Yoon, S., et al. (2023). Addressing amino acid-derived inhibitory metabolites and enhancing CHO cell culture performance through DOE-guided media modifications. Biotechnol. Bioeng. 120, 2542–2558. doi:10.1002/bit.28403
Lai, T., Yang, Y., and Ng, S. K. (2013). Advances in mammalian cell line development technologies for recombinant protein production. J. Pharm. 6 (5), 579–603. doi:10.3390/ph6050579
Lalonde, M.-E., and Durocher, Y. (2017). Therapeutic glycoprotein production in mammalian cells. J. Biotechnol. 251, 128–140. doi:10.1016/j.jbiotec.2017.04.028
Lewis, A. M., Abu-Absi, N. R., Borys, M. C., and Li, Z. J. (2016). The use of ‘Omics technology to rationally improve industrial mammalian cell line performance. Biotechnol. Bioeng. 113 (1), 26–38. doi:10.1002/bit.25673
Liao, T., Ren, Z., Chai, Z., Yuan, M., Miao, C., Li, J., et al. (2023). A super-resolution strategy for mass spectrometry imaging via transfer learning. Nat. Mach. Intell. 5 (6), 656–668. doi:10.1038/s42256-023-00677-7
Lu, S., Sun, X., and Zhang, Y. (2005). Insight into metabolism of CHO cells at low glucose concentration on the basis of the determination of intracellular metabolites. Process Biochem. 40 (5), 1917–1921. doi:10.1016/j.procbio.2004.07.004
Lu, Y., Hu, Q., Yang, C., and Gao, F. (2006). Histidine 89 is an essential residue for Hsp70 in the phosphate transfer reaction. Cell stress and chaperones 11 (2), 148–153. doi:10.1379/csc-152r.1
Mahrous, E. A., and Farag, M. A. (2015). Two dimensional NMR spectroscopic approaches for exploring plant metabolome: a review. J. Adv. Res. 6 (1), 3–15. doi:10.1016/j.jare.2014.10.003
Marin, S., Lee, W. N., Bassilian, S., Lim, S., Boros, L. G., Centelles, J. J., et al. (2004). Dynamic profiling of the glucose metabolic network in fasted rat hepatocytes using [1,2-13C2]glucose. Biochem. J. 381 (1), 287–294. doi:10.1042/bj20031737
Markley, J. L., Brüschweiler, R., Edison, A. S., Eghbalnia, H. R., Powers, R., Raftery, D., et al. (2017). The future of NMR-based metabolomics. Curr. Opin. Biotechnol. 43, 34–40. doi:10.1016/j.copbio.2016.08.001
Mashabela, M. D., Masamba, P., and Kappo, A. P. (2022). Metabolomics and chemoinformatics in agricultural biotechnology research: complementary probes in unravelling new metabolites for crop improvement. J. Biol. 11 (8), 1156. doi:10.3390/biology11081156
McHugh, K. P., Xu, J., Aron, K. L., Borys, M. C., and Li, Z. J. (2020). Effective temperature shift strategy development and scale confirmation for simultaneous optimization of protein productivity and quality in Chinese hamster ovary cells. Biotechnol. Prog. 36 (3), e2959. doi:10.1002/btpr.2959
Menyhárt, O., and Győrffy, B. (2021). Multi-omics approaches in cancer research with applications in tumor subtyping, prognosis, and diagnosis. Comput. Struct. Biotechnol. J. 19, 949–960. doi:10.1016/j.csbj.2021.01.009
Moco, S. (2022). Studying metabolism by NMR-based metabolomics. Front. Mol. Biosci. 9, 882487. doi:10.3389/fmolb.2022.882487
Mohmad-Saberi, S. E., Hashim, Y. Z., Mel, M., Amid, A., Ahmad-Raus, R., and Packeer-Mohamed, V. (2013). Metabolomics profiling of extracellular metabolites in CHO-K1 cells cultured in different types of growth media. Cytotechnology 65 (4), 577–586. doi:10.1007/s10616-012-9508-4
Mulukutla, B. C., Kale, J., Kalomeris, T., Jacobs, M., and Hiller, G. W. (2017). Identification and control of novel growth inhibitors in fed-batch cultures of Chinese hamster ovary cells. Biotechnol. Bioeng. 114 (8), 1779–1790. doi:10.1002/bit.26313
Mulukutla, B. C., Mitchell, J., Geoffroy, P., Harrington, C., Krishnan, M., Kalomeris, T., et al. (2019). Metabolic engineering of Chinese hamster ovary cells towards reduced biosynthesis and accumulation of novel growth inhibitors in fed-batch cultures. Metab. Eng. 54, 54–68. doi:10.1016/j.ymben.2019.03.001
Naik, H. M., Kumar, S., Reddy, J. V., Gonzalez, J. E., McConnell, B. O., Dhara, V. G., et al. (2023). Chemical inhibitors of hexokinase-2 enzyme reduce lactate accumulation, alter glycosylation processing, and produce altered glycoforms in CHO cell cultures. Biotechnol. Bioeng. 120 (9), 2559–2577. doi:10.1002/bit.28417
Nolan, R. P., and Lee, K. (2012). Dynamic model for CHO cell engineering. J. Biotech. 158 (1), 24–33. doi:10.1016/j.jbiotec.2012.01.009
O'Flaherty, R., Bergin, A., Flampouri, E., Mota, L. M., Obaidi, I., Quigley, A., et al. (2020). Mammalian cell culture for production of recombinant proteins: a review of the critical steps in their biomanufacturing. Biotechnol. Adv. 43, 107552. doi:10.1016/j.biotechadv.2020.107552
Okamura, K., Badr, S., Murakami, S., and Sugiyama, H. (2022). Hybrid modeling of CHO cell cultivation in monoclonal antibody production with an impurity generation module. Ind. Eng. Chem. Res. 61 (40), 14898–14909. doi:10.1021/acs.iecr.2c00736
Pan, X., Dalm, C., Wijffels, R. H., and Martens, D. E. (2017). Metabolic characterization of a CHO cell size increase phase in fed-batch cultures. Appl. Microbiol. Biotechnol. 101 (22), 8101–8113. doi:10.1007/s00253-017-8531-y
Park, H., Song, W.-Y., Cha, H., and Kim, T.-Y. (2021). Development of an optimized sample preparation method for quantification of free fatty acids in food using liquid chromatography-mass spectrometry. Sci. Rep. 11 (1), 5947. doi:10.1038/s41598-021-85288-1
Pereira, S., Kildegaard, H. F., and Andersen, M. R. (2018). Impact of CHO metabolism on cell growth and protein production: an overview of toxic and inhibiting metabolites and nutrients. J. Biotechnol. 13 (3), 1700499. doi:10.1002/biot.201700499
Qiu, S., Cai, Y., Yao, H., Lin, C., Xie, Y., Tang, S., et al. (2023). Small molecule metabolites: discovery of biomarkers and therapeutic targets. J. Signal Transduct. 8 (1), 132. doi:10.1038/s41392-023-01399-3
Rejc, Ž., Magdevska, L., Tršelič, T., Osolin, T., Vodopivec, R., Mraz, J., et al. (2017). Computational modelling of genome-scale metabolic networks and its application to CHO cell cultures. Comput. Biol. Med. 88, 150–160. doi:10.1016/j.compbiomed.2017.07.005
Ritacco, F. V., Wu, Y., and Khetan, A. (2018). Cell culture media for recombinant protein expression in Chinese hamster ovary (CHO) cells: history, key components, and optimization strategies. Biotechnol. Prog. 34 (6), 1407–1426. doi:10.1002/btpr.2706
Saldanha, M., Padhye, K., Warke, V. G., Dandekar, P., and Jain, R. (2023). A feed enrichment strategy targeting the tricarboxylic acid cycle for increasing monoclonal antibody production and alleviating ammonia accumulation in Chinese hamster ovary cell culture. Biochem. Eng. J. 192, 108836. doi:10.1016/j.bej.2023.108836
Sellick, C. A., Knight, D., Croxford, A. S., Maqsood, A. R., Stephens, G. M., Goodacre, R., et al. (2010). Evaluation of extraction processes for intracellular metabolite profiling of mammalian cells: matching extraction approaches to cell type and metabolite targets. Metabolomics 6, 427–438. doi:10.1007/s11306-010-0216-9
Sellick, C. A., Croxford, A. S., Maqsood, A. R., Stephens, G. M., Westerhoff, H. V., Goodacre, R., et al. (2015). Metabolite profiling of CHO cells: molecular reflections of bioprocessing effectiveness. Biotechnol. J. 10 (9), 1434–1445. doi:10.1002/biot.201400664
Selvarasu, S., Ho, Y. S., Chong, W. P., Wong, N. S., Yusufi, F. N., Lee, Y. Y., et al. (2012). Combined in silico modeling and metabolomics analysis to characterize fed-batch CHO cell culture. Biotechnol. Bioeng. 109 (6), 1415–1429. doi:10.1002/bit.24445
Sengupta, N., Rose, S. T., and Morgan, J. A. (2011). Metabolic flux analysis of CHO cell metabolism in the late non-growth phase. J. Biotechnol. Lett. 108 (1), 82–92. doi:10.1002/bit.22890
Shang, M., Kwon, T., Hamel, J.-F. P., Lim, C. T., Khoo, B. L., and Han, J. (2021). Investigating the influence of physiologically relevant hydrostatic pressure on CHO cell batch culture. Sci. Rep. 11 (1), 162. doi:10.1038/s41598-020-80576-8
Shi, X., Xi, B., Jasbi, P., Turner, C., Jin, Y., and Gu, H. (2020). Comprehensive isotopic targeted mass spectrometry: reliable metabolic flux analysis with broad coverage. Anal. Chem. 92 (17), 11728–11738. doi:10.1021/acs.analchem.0c01767
Singh, R., Thakur, L., Kumar, A., Singh, S., Kumar, S., Kumar, M., et al. (2023). Comparison of freeze-thaw and sonication cycle-based methods for extracting AMR-associated metabolites from Staphylococcus aureus. Front. Microbiol. 14, 1152162. doi:10.3389/fmicb.2023.1152162
Sowa, S. W., Qian, Y., Aron, K. L., Xu, P., Langsdorf, E., Warrack, B., et al. (2020). Metabolomic and quality data for early and late passages of an antibody-producing industrial CHO cell line. Data brief 33, 106591. doi:10.1016/j.dib.2020.106591
Templeton, N., Dean, J., Reddy, P., and Young, J. D. (2013). Peak antibody production is associated with increased oxidative metabolism in an industrially relevant fed-batch CHO cell culture. Biotechnol. Bioeng. 110 (7), 2013–2024. doi:10.1002/bit.24858
Templeton, N., Smith, K. D., McAtee-Pereira, A. G., Dorai, H., Betenbaugh, M. J., Lang, S. E., et al. (2017). Application of 13C flux analysis to identify high-productivity CHO metabolic phenotypes. Metab. Eng. 43, 218–225. doi:10.1016/j.ymben.2017.01.008
Tihanyi, B., and Nyitray, L. (2020). Recent advances in CHO cell line development for recombinant protein production. Drug Discov. today Technol. 38, 25–34. doi:10.1016/j.ddtec.2021.02.003
Torres, M., and Dickson, A. J. (2022). Reprogramming of Chinese hamster ovary cells towards enhanced protein secretion. Metab. Eng. 69, 249–261. doi:10.1016/j.ymben.2021.12.004
Torres, M., Betts, Z., Scholey, R., Elvin, M., Place, S., Hayes, A., et al. (2023a). Long term culture promotes changes to growth, gene expression, and metabolism in CHO cells that are independent of production stability. Biotechnol. Bioeng. 120 (9), 2389–2402. doi:10.1002/bit.28399
Torres, C. L., Scalco, F. B., de Oliveira, M. L. C., Peake, R. W. A., and Garrett, R. (2023b). Untargeted LC-HRMS metabolomics reveals candidate biomarkers for mucopolysaccharidoses. Clin. chimica acta; Int. J. Clin. Chem. 541, 117250. doi:10.1016/j.cca.2023.117250
Trummer, E., Fauland, K., Seidinger, S., Schriebl, K., Lattenmayer, C., Kunert, R., et al. (2006). Process parameter shifting: Part I. Effect of DOT, pH, and temperature on the performance of Epo-Fc expressing CHO cells cultivated in controlled batch bioreactors. Biotechnol. Bioeng. 94 (6), 1033–1044. doi:10.1002/bit.21013
Vodopivec, M., Lah, L., Narat, M., and Curk, T. (2019). Metabolomic profiling of CHO fed-batch growth phases at 10, 100 and 1000 L. Biotechnol. Bioeng. 116 (10), 2720–2729. doi:10.1002/bit.27087
Wagstaff, J. L., Masterton, R. J., Povey, J. F., Smales, C. M., and Howard, M. J. (2013). 1H NMR spectroscopy profiling of metabolic reprogramming of Chinese hamster ovary cells upon a temperature shift during culture. PLOS ONE 8 (10), e77195. doi:10.1371/journal.pone.0077195
Weng, Z., Jin, J., Shao, C., and Li, H. J. C. (2020). Reduction of charge variants by CHO cell culture process optimization. Cytotechnology 72 (2), 259–269. doi:10.1007/s10616-020-00375-x
Wijaya, A. W., and Takors, R. (2021). A transhydrogenase-like mechanism in CHO cells comprising concerted cytosolic reaction and mitochondrial shuttling activities. Biochem. Eng. J. 170, 107986. doi:10.1016/j.bej.2021.107986
Wijaya, A. W., Ulmer, A., Hundsdorfer, L., Verhagen, N., Teleki, A., and Takors, R. (2021). Compartment-specific metabolome labeling enables the identification of subcellular fluxes that may serve as promising metabolic engineering targets in CHO cells. J. Bioprocess 44, 2567–2578. doi:10.1007/s00449-021-02628-1
Wishart, D. S., Knox, C., Guo, A. C., Eisner, R., Young, N., Gautam, B., et al. (2009). HMDB: a knowledgebase for the human metabolome. Nucleic acids Res. 37, D603–D610. doi:10.1093/nar/gkn810
Wurm, F. M. (2004). Production of recombinant protein therapeutics in cultivated mammalian cells. J. Nat. Biotechnol. 22 (11), 1393–1398. doi:10.1038/nbt1026
Yao, G., Aron, K., Borys, M., Li, Z., Pendse, G., and Lee, K. (2021). A metabolomics approach to increasing Chinese hamster ovary (CHO) cell productivity. Metabolites 11 (12), 823. doi:10.3390/metabo11120823
Yusufi, F. N. K., Lakshmanan, M., Ho, Y. S., Loo, B. L. W., Ariyaratne, P., Yang, Y., et al. (2017). Mammalian systems biotechnology reveals global cellular adaptations in a recombinant CHO cell line. Cell Syst. 4 (5), 530–542.e6. doi:10.1016/j.cels.2017.04.009
Zeki, Ö. C., Eylem, C. C., Reçber, T., Kır, S., and Nemutlu, E. (2020). Integration of GC–MS and LC–MS for untargeted metabolomics profiling. J. Pharm. 190, 113509. doi:10.1016/j.jpba.2020.113509
Zhang, L., Zhang, W., Wang, C., Liu, J., Deng, X., Liu, X., et al. (2016). Responses of CHO-DHFR cells to ratio of asparagine to glutamine in feed media: cell growth, antibody production, metabolic waste, glutamate, and energy metabolism. J. Bioresour. 3, 5–12. doi:10.1186/s40643-015-0072-6
Zhang, X., Jiang, R., Lin, H., and Xu, S. (2020). Feeding tricarboxylic acid cycle intermediates improves lactate consumption and antibody production in Chinese hamster ovary cell cultures. Biotechnol. Prog. 36 (4), e2975. doi:10.1002/btpr.2975
Zhang, H.-Y., Fan, Z.-L., and Wang, T.-Y. (2021). Advances of glycometabolism engineering in Chinese hamster ovary cells. Front. Bioeng. Biotechnol. 9, 774175. doi:10.3389/fbioe.2021.774175
Zhao, F., Wan, Y., Nie, L., Jiao, J., Gao, D., Sun, Y., et al. (2023). 1H NMR-based process understanding and biochemical marker identification methodology for monitoring CHO cell culture process during commercial-scale manufacturing. Biotechnol. J. 18 (7), e2200616. doi:10.1002/biot.202200616
Zhong, A. B., Muti, I. H., Eyles, S. J., Vachet, R. W., Sikora, K. N., Bobst, C. E., et al. (2022). Multiplatform metabolomics studies of human cancers with NMR and mass spectrometry imaging. Front. Mol. Biosci. 9, 785232. doi:10.3389/fmolb.2022.785232
Keywords: Chinese hamster ovary cells, metabolomics, bioprocess, cell engineering, growth, productivity, extraction, process design
Citation: Singh R, Fatima E, Thakur L, Singh S, Ratan C and Kumar N (2024) Advancements in CHO metabolomics: techniques, current state and evolving methodologies. Front. Bioeng. Biotechnol. 12:1347138. doi: 10.3389/fbioe.2024.1347138
Received: 30 November 2023; Accepted: 28 February 2024;
Published: 27 March 2024.
Edited by:
Simon Fischer, Boehringer Ingelheim Pharma GmbH & Co. KG, GermanyReviewed by:
Thomas Wucherpfennig, Boehringer Ingelheim, GermanyCopyright © 2024 Singh, Fatima, Thakur, Singh, Ratan and Kumar. This is an open-access article distributed under the terms of the Creative Commons Attribution License (CC BY). The use, distribution or reproduction in other forums is permitted, provided the original author(s) and the copyright owner(s) are credited and that the original publication in this journal is cited, in accordance with accepted academic practice. No use, distribution or reproduction is permitted which does not comply with these terms.
*Correspondence: Niraj Kumar, bmt1bWFyQHRoc3RpLnJlcy5pbg==
Disclaimer: All claims expressed in this article are solely those of the authors and do not necessarily represent those of their affiliated organizations, or those of the publisher, the editors and the reviewers. Any product that may be evaluated in this article or claim that may be made by its manufacturer is not guaranteed or endorsed by the publisher.
Research integrity at Frontiers
Learn more about the work of our research integrity team to safeguard the quality of each article we publish.