- 1Guangdong Key Laboratory of Fermentation and Enzyme Engineering, School of Biology and Biological Engineering, South China University of Technology, Guangzhou, China
- 2School of Light Industry and Engineering, South China University of Technology, Guangzhou, China
The model of intracellular metabolic network based on enzyme kinetics parameters plays an important role in understanding the intracellular metabolic process of Corynebacterium glutamicum, and constructing such a model requires a large number of enzymological parameters. In this work, the genes encoding the relevant enzymes of the EMP and HMP metabolic pathways from Corynebacterium glutamicum ATCC 13032 were cloned, and engineered strains for protein expression with E.coli BL21 and P.pastoris X33 as hosts were constructed. The twelve enzymes (GLK, GPI, TPI, GAPDH, PGK, PMGA, ENO, ZWF, RPI, RPE, TKT, and TAL) were successfully expressed and purified by Ni2+ chelate affinity chromatography in their active forms. In addition, the kinetic parameters (Vmax, Km, and Kcat) of these enzymes were measured and calculated at the same pH and temperature. The kinetic parameters of enzymes associated with EMP and the HMP pathway were determined systematically and completely for the first time in C.glutamicum. These kinetic parameters enable the prediction of key enzymes and rate-limiting steps within the metabolic pathway, and support the construction of a metabolic network model for important metabolic pathways in C.glutamicum. Such analyses and models aid in understanding the metabolic behavior of the organism and can guide the efficient production of high-value chemicals using C.glutamicum as a host.
1 Introduction
Cellular metabolism involves the conversion of one substance into another through a series of enzymatic reactions (Feierabend et al., 2021). By collecting and summarizing data on these enzymes and simulating metabolic networks involved, we can achieve a better understanding of dynamic behavior of cellular system, elucidate metabolic pathways flux distribution, and guide metabolic engineering design in the yield of potential targets or metabolites (Albert, 2007; Covert et al., 2001; Gombert and Nielsen, 2000). Altintas MM (Altintas et al., 2006) has used the published values for kinetic parameters of individual enzymatic reactions in the pentose phosphate pathway (HMP pathway) and the Entner-Doudoroff (ED) pathway to develop an intracellular metabolic network model in Aspergillus oryzae. They conducted successfully in silico simulations to enhance xylose utilization and increase the yield of desired metabolites.
However, it is worth noting that even for networks databases as extensive as KEGG or MetaCyc, the available parameters often prove insufficient to construct precise metabolic model and describe metabolite dynamics. An important reason for this limitation is that these parameters are typically obtained or estimated from measurements reported by different laboratories, each employing various in vitro models and conditions (Tohsato et al., 2013). Bertilsson et al. (2008) compiled kinetic parameters of enzymes from various sources, constructed a kinetic model for glucose and xylose co-substrates uptake in Saccharomyces cerevisiae, and proposed strategies to improve co-substrate utilization based on transport systems modifications. However, the kinetic parameters for Hxt3p with respect to xylose were unavailable in the literature, requiring researchers to relay on parameters from another homologous enzyme, potentially affecting the accuracy of the model. Dräger used multiple kinetic equations and presented a comprehensive mathematical model of valine and leucine biosynthesis in C. glutamicum (Drager et al., 2009). Nevertheless, kinetic modeling or metabolic network reconstitution necessitates an extensive amount of information regarding the kinetics of each enzyme, including reaction rates, Michaelis constants, and so on (Andersen and Nielsen, 2009; Zupanic et al., 2020).
Corynebacterium glutamicum has been engineered as an important platform organism which far outweighed amino acid production during the last decades. Notably, it has not only excelled in producing organic acids such as pyruvic acid and succinic acid, but also demonstrated the capability to synthesize high-value compounds like putrescine (1,4-diaminobutane) and resveratrol (Kallscheuer et al., 2019; Kogure et al., 2016; Zha et al., 2023). In silico, well-characterized metabolic modeling is expected to enable the design of artificial metabolic networks for desired product synthesis in C.glutamicum. Hence, it is essential to have accurate data regarding some central metabolism or related pathways, including glycolysis (EMP pathway) and the pentose phosphate pathway (HMP pathway) since they provide ATP as well as offer reducing power (NADPH) in cells (Aziz and Mohiuddin, 2022; Tan et al., 2016). The acquisition of comprehensive and systematic kinetic parameters for enzymes involved in these pathways will undoubtedly facilitate the construction of intracellular metabolic networks and enhance the accuracy of mathematical models.
In this study, the EMP and HMP pathway-related enzyme genes were cloned from the model strain C.glutamicum ATCC13032. Subsequently, these genes were expressed exogenously in Escherichia coli BL21 (DE3) or Pichia pastoris X33, and the corresponding enzymes were purified and prepared as far as possible. By determining and calculating the kinetic parameters of these enzymes, valuable data for the construction of a metabolic network model encompassing the central metabolic pathways in C. glutamicum were obtained. In order to provide a more comprehensive reference for researchers, we also collected and summarized the enzymatic properties of reported enzymes related to the above two pathways from different cells. These works contribute significantly to a thorough understanding of the dynamic behavior of cellular systems and flux control distribution in C. glutamicum. As a result, they greatly benefit the design of new metabolic pathway in C. glutamicum, positioning it as a promising cell factory for potential application.
2 Material and methods
2.1 Strains and culture conditions
The plasmid of pET-28a (+): Bacterial vector for the expression of N-terminal 6x His-tagged proteins. E.coli TOP10F′ cells (Invitrogen, Carlsbad, CA, United States) were cultured at 37°C in Luria-Bertani medium (LB) (1% w/v tryptone, 0.5% w/v yeast extract, 1% w/v sodium chloride) containing 50 mg/L kanamycin to select and obtain the recombinant plasmid. E.coli BL21 (DE3) served as host for heterologous gene expression and was grown in LB medium at 37°C and 200 rpm. P.pastoris X33 was used as host for heterologous gene expression and grown in BMMY/BMGY medium at 30°C and 250 rpm. These cells were used for DNA manipulations. Buffered methanol complex and glycerol complex medium (BMMY/BMGY) was prepared according to yeast fermentation guidelines.
2.2 Cloning of EMP and HMP pathway related enzyme genes from C. glutamicum ATCC 13032
Seventeen enzyme genes which related to EMP and HMP pathway from the genomic DNA of C.glutamicum ATCC 13032 (GeneBank: GCA_000011325.1) were clonded. The primers used for amplification were designed based on the nucleotide sequences of these genes (Supplementary Tables S1, S2). The coding sequence of the related protein genes were inserted into the expression plasmid of pET28a (+) by using homologous recombination technology, and their sequences were verified by DNA sequencing. The plasmids are then transformed into E.coli BL21 (DE3) for expression (Figure 1).
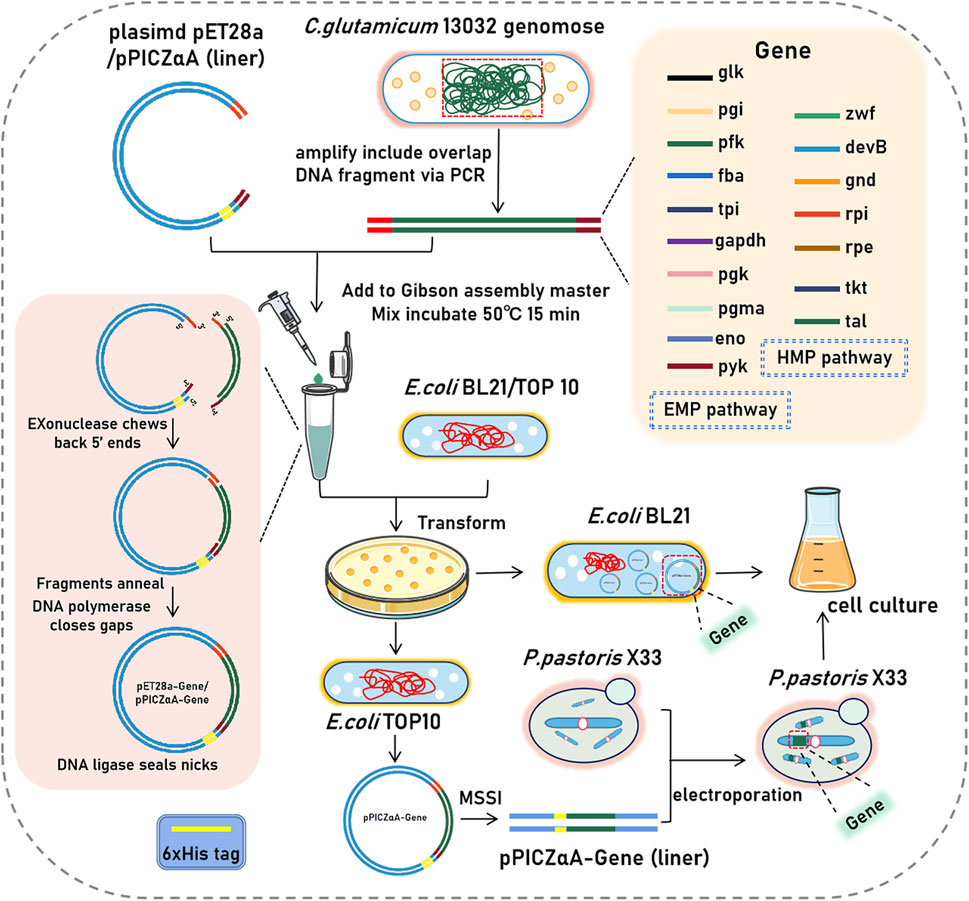
FIGURE 1. Schematic diagram of plasmid and expression strains construction. (glk, glucokinase; gpi, glucose-6-phosphate isomerase; pfk, 6-phosphofructokinase; fba, fructose-bisphosphate aldolase; tpi, triosephosphate isomerase; gapdh, glyceraldehyde 3-phosphate dehydrogenase; pgk, phosphoglycerate kinase; pgma, phosphoglycerate mutase; eno, enolase; pyk, pyruvate kinase; zwf, glucose-6-phosphate dehydrogenas; gnd, gluconate 6-phosphate dehydrogenase; devB, 6-phosphogluconolactonase; rpi, ribose-5-phosphate isomeras; rpe, ribulose-5-phosphate 3-epimerase; tkt, transketolase; tal, transaldolase).
In addition, five enzyme genes (6-phosphofructokinase, fructose-bisphosphate aldolase, enolase, pyruvate kinase, and gluconate-6-phosphate dehydrogenase) were inserted into the expression plasmids of pPICZαA. Similar to pET28a (+) plasmids, the constructed pPICZαA plasmids was also verified by DNA sequencing. To enable expression in P.pastoris X33, the pPICZαA plasmids were linearized using a restriction enzyme digestion site MSSI on the plasmid. The linearized plasmids were then introduced into P.pastoris X33 cells via electroporation (Feng et al., 2017).
2.3 Enzyme expression in E.coli BL21 and P.pastoris X33
To prepare the inoculum, 100 μL of the cell suspension stored at −80°C was transferred to a 50 mL Erlenmeyer flask containing 10 mL of LB medium with 50 mg/L kanamycin, incubated at 37°C and 200 rpm for 10–12 h, and inoculated at a ratio of 1:100 (v/v) in 100 mL shake flasks at 37°C and 200 rpm until the OD600 nm values in the LB medium reached 0.6–0.8 (Carmignotto and Azzoni, 2019). Isopropyl-β-D-thiogalactoside (final IPTG concentration 0.4 mM) was then added to the culture medium and incubation continued for another approximately 16 h at 16°C. Cells were collected at the end of induction by centrifugation at 6,000 rpm, 15 min, 4°C.
Inoculums were prepared by transferring 100 μL of the cell suspension stored at −80°C to a 50 mL erlenmeyer flask containing 5 mL of BMGY medium with 100 μg/mL zeocin and supplemented with 1% (v/v) glycerol as the sole carbon source. Culture was performed overnight at 30°C and 250 rpm. The inoculum was then inoculated into 250 mL shake flasks containing 50 mL of BMMY medium until the OD600 nm values in the BMMY medium reached 1.0, and supplemented with 1% (v/v) methanol as the sole carbon source, incubation was continued for another 3–5 days at 30°C. The supernatant was obtained at the end of cultivation by centrifugation at 6,000 rpm, 10 min, 4°C.
2.4 Enzyme purification and analysis of protein concentration
The collected cells were resuspended in 10 mL of buffer A (20 mM Tris-HCl, pH 7.0, and 500 mM NaCl). This was followed by comminution by ultrasonication in an ice-water bath with 300 cycles of 3 s of sonication followed by a 3-s pause between cycles. The crude extract (supernatant) was obtained by centrifugation (10,000 rpm, 20 min, 4°C), and enzymes associated with the EMP and the HMP pathway were purified by a nickel ion affinity chromatography column and analyzed by SDS-PAGE. For analysis SDS-PAGE samples were taken before induction, after induction, the crushed supernatant and the precipitate to analyse the solubility and molecular weights of the protein. Gels were stained with Komas Brilliant Blue R-250 and decolorized by washing with 10% acetic acid. Enzyme concentrations were determined by the Bradford method using bovine serum albumin as a concentration standard (Xue-ling et al., 2010).
The supernatant was filtered through a 0.22 µm membrane and purified with a nickel-affinity chromatography column (TIANGEN Biotech (Beijing) Co.). The column was equilibrated with buffer A and the purification pathway was rinsed. The heteroproteins were first eluted with buffer B (0.5 M imidazole, 20 mM Tris-HCl pH 7.0, and 500 mM NaCl) containing 0.5 M imidazole, and then each enzyme was eluted in turn with buffer B containing 0.2 M imidazole, 0.3 M imidazole and 0.5 M imidazole. The purified samples were desalted in a desalting column with buffer A.
2.5 Determination of enzymatic kinetic parameters
Enzyme kinetic parameters were measured in the 100 mM PBS buffer (137 mM NaCl, 2.7 mM KCl, 10 mM Na2HPO4, 2 mM KH2PO4, 0.5 mM MgCl2, pH7.4) and at 37°C (Supplementary Material S2). One unit (U) of enzyme activity was defined as the amount of enzyme that catalyzes the conversion of 1 µmol of substrate per minute into specific products (Tan et al., 2016). With substrate concentration as the horizontal coordinate and enzyme reaction rate as the vertical coordinate, the curve of the Mee equation was fitted using origin software. From the fitted cureve, the values of Km (Michaelis constant) and Vmax (maximum reaction rate) were calculated. Furthermore, the turnover number, Kcat, was calculated based on Kcat = Vmax/enzyme concentration.
3 Results
3.1 Expression of EMP and HMP pathway related enzymes
Six enzymes (GLK, GPI, TPI, GAPDH, PGMA, and PYK) from the EMP metabolic pathway of C.glutamicum ATCC 13032 were successfully expressed exogenously in E.coli BL21. SDS-PAGE analysis confirmed that all six enzymes were soluble, and their molecular weights are found to be consistent with the reported or predicted values in the literature (Mathur et al., 2005; Mathur et al., 2006; Sangolgi et al., 2016a). The theoretical molecular weights of these enzymes are estimated to be 35.37 kDa (GLK), 60.35 kDa (GPI), 28.39 kDa (TPI), 37.23 kDa (GAPDH), 28.43 kDa (PGMA), and 52.81 kDa (PYK), separately (Figure 2). However, two enzymes (PFK and FBA) were expressed as inactive inclusion bodies form. The other two enzymes (PGK and ENO) that could not expressed in E.coli BL21 were successfully expressed exogenously in P.pastoris X33. The molecular weight of the expressed PGK and ENO enzymes in P. pastoris X33 are closed to the reported or predicted values in the literature (Cayir et al., 2014a; Shang et al., 2021), which are predicted to be 43.88 kDa (PGK-Y) and 46.10 kDa (ENO-Y), respectively (Figure 2).
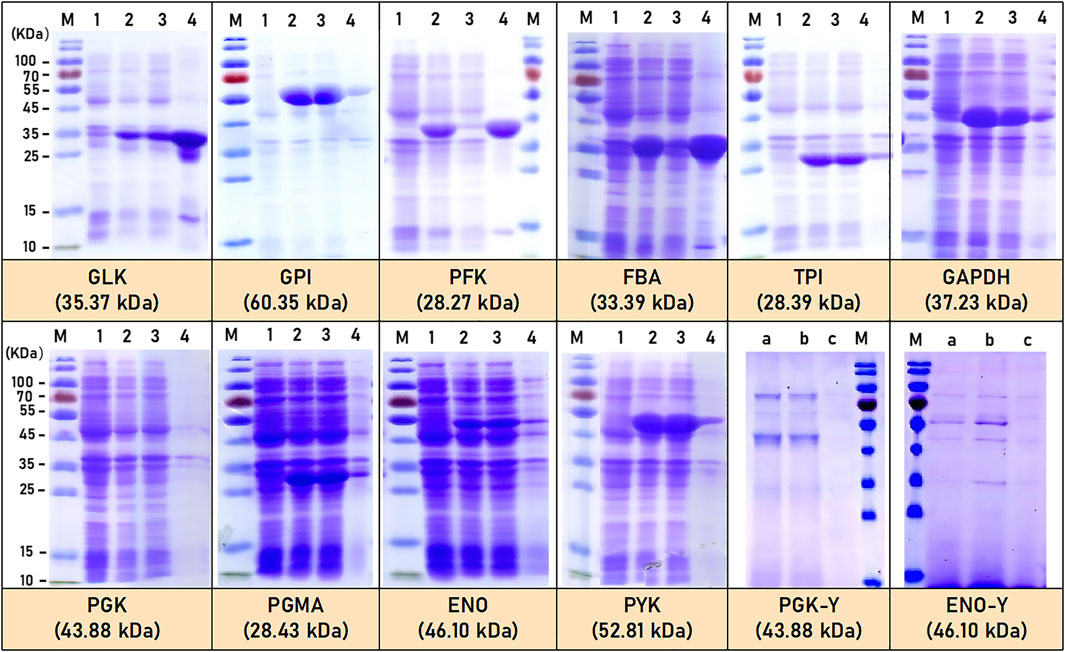
FIGURE 2. SDS-PAGE of EMP pathway related enzymes expression by E.coli and P.pastoris. M: marker; 1: before induction; 2: after induction; 3: crushed supernatant; 4: precipitate; a: yeast expression for 3 days; b: yeast expression for 5 days; c: control.
On the other hand, all seven enzymes (ZWF, DEVB, GND, RPI, RPE, TKT, and TAL) of the HMP pathway in C.glutamicum ATCC13032 were successfully expressed exogenously in E.coli BL21. SDS-PAGE analysis confirmed that all seven enzymes are soluble proteins, with only a small amount observed as inclusion bodies (Figure 3). Importantly, the molecular weight positions of the expressed enzymes closely matched the predicted values. Specifically, the molecular weights of seven enzymes were predicted as follows: ZWF (58.66 kDa), DEVB (25.66 kDa), GND (53.76 kDa), RPI (18.17 kDa), RPE (25.05 kDa), TKT (72.26 kDa), and TAL (39.51 kDa) (Figure 3).
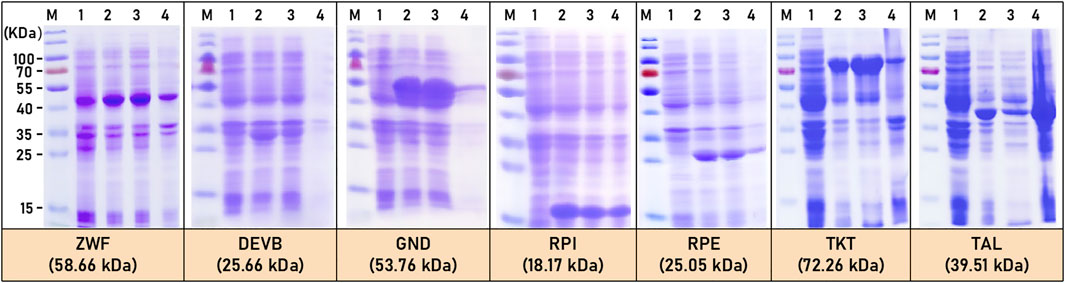
FIGURE 3. SDS-PAGE of HMP pathway related enzymes expression. M: marker; 1: before induction; 2: after induction; 3: precipitate; 4: crushed supernatant.
3.2 Purification of enzymes associated with the EMP and HMP pathway
The fifteen successfully expressed enzymes from crude cell extracts were purified using Ni ion-affinity chromatography columns, which utilized a His tag at either the C-terminus or N-terminus of the recombinant enzymes. Validation of the purified samples by SDS-PAGE showed that GLK, GPI, TPI, GAPDH, PGMA, ENO, PYK, PGK-Y, and ENO-Y were eluted with buffer B containing 0.5 M, 0.2 M, 0.2 M, 0.3 M, 0.3 M, 0.3 M, 0.3 M, 0.2 M, and 0.2 M (elution concentration of protein) imidazole, respectively. ZWF, GND, RPI, RPE, TKT and TAL were eluted with buffer B containing 0.5 M, 0.5 M, 0.5 M, 0.3 M, 0.3 M and 0.2 M imidazole, respectively (Figure 4).
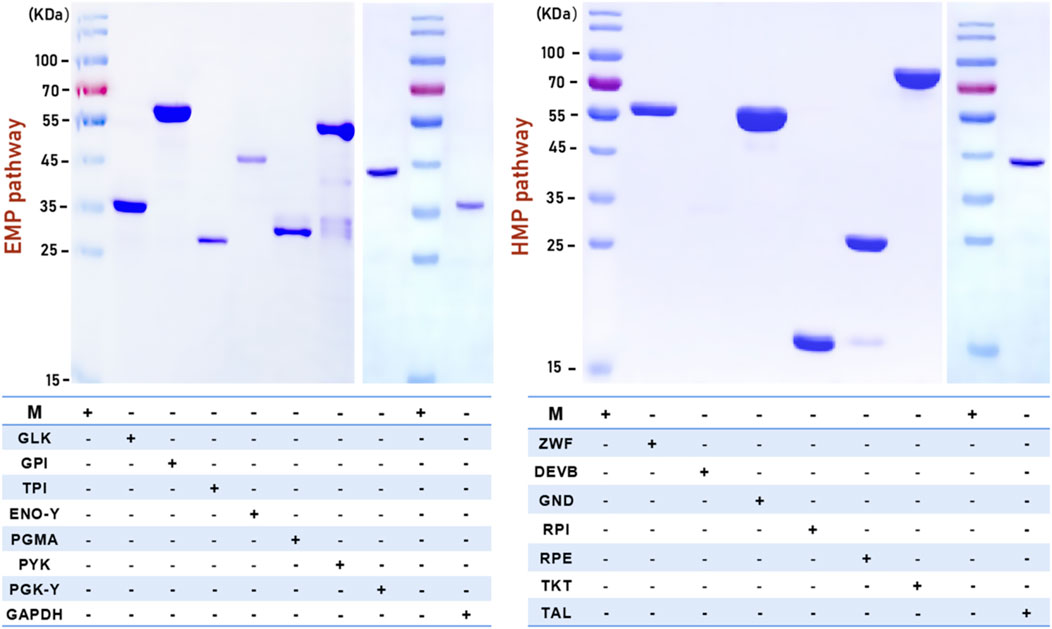
FIGURE 4. SDS-PAGE of EMP and HMP pathway related pure enzymes. “+“: contain related enzyme in this swimming lane; “-“: excluding related enzyme in this swimming lane.
3.3 Determination of kinetic parameters of enzymes associated with the EMP and HMP pathways
Enzyme kinetic curves were obtained by conducting experiments (Figures 5, 6), and enzyme kinetic parameters were calculated for fourteen purified enzymes from the EMP and HMP pathways (Table 1). The kinetic measurements were carried out under consistent conditions, ensuring the temperature and pH remained constant. However, it was observed that the purified enzymes PYK and GND did not show any activity with their respective substrates, namely phosphoenolpyruvate and glucose-6-phosphate. Additionally, the substrate for DEVB could not be determined as it was not available or provided in the previous studies and literature.
The enzymes GLK, GPI, TPI, GAPDH, PGK, PMGA and ENO correspond to the substrates glucose/ATP, D-fructose-6-disodium phosphate hydrate, dihydroxyacetone phosphate, dihydroxyacetone phosphate, glyceraldehyde triphosphate, disodium phosphoglycerate, 3-phosphoglycerate, and 2-phosphoglycerate with Km values of 4.6 ± 0.18 mM/0.81 ± 0.08 mM, 0.57 ± 0.13 mM, 5.43 ± 0.16 mM, 1.47 ± 0.11 mM, 0.94 ± 0.15 mM, 4.44 ± 0.32 mM, and 0.06 ± 0.01 mM, respectively.
The enzymes ZWF, GND, RPI, RPE, TKT, and TAL correspond to the substrates NADP/glucose-6-phosphate, D-ribulose-5-phosphate, D-xylulose-5-phosphat/D-ribulose-5-phosphate and D-sedoheptulose-7-phosphate/glyceraldehyde-3-phosphate, respectively, with Km values of 0.46 ± 0.04 mM/1.61 ± 0.15 mM, 0.42 ± 0.06 mM, 2.81 ± 1.25 mM, 0.47 ± 0.04 mM/1.13 ± 0.29 mM, 0.82 ± 0.02 mM/0.29 ± 0.08 mM, respectively (Table 1).
Compared with previous studies, more kinetic data of enzymes associated with EMP and HMP pathways were obtained under the same conditions. This is crucial because previous research reports indicate that powerful and potential metabolic network modeling requires accurate quantitative information about enzymatic reaction rates obtained under consistent experimenting methods or conditions (Tohsato et al., 2013). In this research, twelve enzymes kinetics parameters were achieved about EMP and HMP pathway from C.glutamicum, which provides a significant meaningful data basis for modeling the central metabolic pathway based on enzyme kinetics. At the same time, the measurement conditions for these parameters are essentially the same, which reduces the error caused by data obtained from different laboratories and measurement conditions in the process of the metabolic modeling in previous studies.
4 Discussion and conclusion
4.1 Analysis and prediction of rate limiting enzymes based on Km value
Vmax represents the maximum rate of the reaction under the given condition, while Km is the substrate concentration at half the maximum reaction rate (Barenholz et al., 2017). The Km value is often used to determine the affinity of an enzyme for its substrate and can indicate the rate-limiting step in a metabolic pathway. Enzymes with higher Km values may have a lower affinity for their substrates, and therefore, in a series of enzyme chain reactions, the Km value of the enzyme will help to identify the rate limiting step in this reaction. In this study, the higher Km values of GLK, GAPDH, TPI, ZWF, and RPE enzymes compared to other enzymes in the EMP and HMP metabolic pathway suggest that these enzymes may have a lower affinity for their substrates and be rate-limiting in their respective pathway.
GLK, as the first enzyme in both the EMP and HMP pathway, catalyzes the conversion of glucose to glucose-6-phosphate. It is essential for glucose metabolism and considered as the rate-limiting enzyme in the procession of glucose metabolism (Milanes et al., 2019; Sternisha and Miller, 2019). TPI, located at the junction of the EMP and HMP pathways, is crucial for converting dihydroxyacetone phosphate (DHAP) and glycerol-3-phosphate (G3P) in the glycolytic pathway. It plays an essential role in the net production of ATP in glucose metabolism (Myers and Palladino, 2023; Schachner et al., 2022). ZWF is primarily responsible for converting glucose-6-phosphate (G6P) to 6-phosphate glucolactone, producing NADPH or NADH. This enzyme is critical for maintaining the redox balance within cells by generating NADPH, which is essential for various cellular processes (Gomez-Manzo et al., 2016). As a result, ZWF is recognized as a rate-limiting enzyme in the HMP metabolic pathway. Notably, RPI has a lower Km value than RPE when using D-ribose 5-phosphate as a substrate, indicating that RPI is more effective than RPE in isomerizing ribose 5-phosphate. RPI and RPE involves the interconversion of ribose-5-phosphate and xylose-5-phosphate in the EMP and HMP pathways, which are important intermediates in nucleotide and cofactor biosynthesis. Therefore, there are also considered as key rate limiting enzyme on the HMP pathway. In summary, the enzymes identified by the Km comparison in this study, which represent the rate-limiting step, are consistent with existing research reports, demonstrating the data availability of this study. It also provides valuable insights into the role and importance of GLK, TPI, ZWF, RPI and RPE enzymes in the EMP and HMP metabolic pathways (Figure 7).
4.2 Analysis of metabolic regulation based on enzyme activity
Kcat is the turnover number of enzyme, representing the number of substrate molecules converted to product per enzyme molecule per unit time. The Kcat/Km ratio, also known as the specificity constant, is a measure of enzyme efficiency. A higher Kcat/Km values indicates a higher catalytic efficiency and enzyme activity (Davidi et al., 2016; Niu et al., 2022). In this study, the Kcat/Km values of GPI and RPE were relatively high, indicating higher catalytic efficiency. On the other hand, GLK, ZWF, and TKT had relatively low Kcat/Km values, suggesting that they are less efficient in catalyzing reactions and have a lower catalytic activity. These enzymes should be regulated during the metabolic process to maintain cellular metabolic balance.
Genome-scale metabolic modeling studies show that upregulating ZWF, which is contributes to NADPH production, to increase isobutanol production by C.glutamicum. Conversely, downregulating enzymes like PGK was identified as a strategy for overproduction of 1,2-propylene glycol in (Zhang et al., 2017). On the other hand, overexpression of GAPDH can significantly improve glucose utilization and enhance shikimic acid production (Kogure et al., 2016). Mutation in enzymes, such as ZWF and GND, increase the supply of NADPH from the HMP pathway, resulting in improved methionine yield (Li et al., 2016). Indeed, in metabolic networks, overexpression of rate-limiting enzymes can be a valuable strategy to enhance the flux through specific metabolic pathways, which plays a crucial role in maintaining stable metabolic flux and minimizing the consumption of intermediate metabolites.
The use of enzyme kinetics parameters for intracellular metabolism flow analysis (MFC) and metabolic network modeling is very effective for analyzing microbial metabolic pathways and formulating optimal design and operation strategies for fermentation (Oshiro et al., 2009). Moreno-Sánchez R. established the first kinetic model of glycolytic metabolism through determined kinetic parameters for five enzymes and their reversal reactions, and predicted flux control by 3-phosphoglycerate mutase and hexokinase under conditions of low substrate and product concentrations (Moreno-Sanchez et al., 2008). By combining experimental enzyme characterizations with mathematical modeling approaches, Kuschmierz L. were able to design and optimize enzyme cascades and whole-cell biocatalysts for efficient d-xylose metabolism (Kuschmierz et al., 2022). Oshiro M. created a kinetic simulation model for acetone-butanol-ethanol fermentation and uncovered several important metabolic pathways that contribute to higher butanol yield (Oshiro et al., 2009). Apparently, cellular metabolic model construction, along with techniques like MFA, provides valuable insights into the balance of metabolic pathway fluxes and aids in the identification of rate-limiting steps. However, one of the challenges in developing kinetic model is the lack of comprehensive experimental data sets that can be used for parameterization, and gathering a complete set of kinetic parameters for all the enzymes and reactions in a metabolic network is a complex task.
In this study, 17 enzymes associated with the EMP and the HMP pathway were cloned from C.glutamicum ATCC 13032 and expressed in E.coli BL21 and P.pastoris X33 strains. By determining and calculating kinetic parameters such as Km, Kcat, and Vmax values of 12 enzymes, a systematic and complete characterization of their enzymes activities was achieved for the first time. These kinetic parameters of enzymes enable the prediction of key enzymes and rate-limiting steps within the metabolic pathway, as well as support the construction of a metabolic network model for important metabolic pathways in C.glutamicum. Such analyses and models aid in understanding the metabolic behavior of the organism and can guide the efficient production of high-value chemicals using C.glutamicum as a host.
Data availability statement
The datasets presented in this study can be found in online repositories. The names of the repository/repositories and accession number(s) can be found below: https://www.ncbi.nlm.nih.gov/, GCF_000011325.1.
Author contributions
LY: Formal analysis, Data curation, Writing–original draft. JL: Methodology, Visualization. YZ: Methodology, Visualization; LC: Methodology, Visualization; ZO: Conceptualization, Methodology; DL: Conceptualization, Methodology; FZ: Conceived and designed the analysis, Writing–review & editing; SH: Conceptualization, Supervision, Writing–review and editing, Funding acquisition.
Funding
The author(s) declare financial support was received for the research, authorship, and/or publication of this article. This work was supported by the Tianjin Synthetic Biotechnology Innovation Capacity Improvement Project (TSBICIP-KJGG-005).
Acknowledgments
Thanks for the support of Tianjin Synthetic Biotechnology Innovation Capacity Improvement Project.
Conflict of interest
The authors declare that the research was conducted in the absence of any commercial or financial relationships that could be construed as a potential conflict of interest.
Publisher’s note
All claims expressed in this article are solely those of the authors and do not necessarily represent those of their affiliated organizations, or those of the publisher, the editors and the reviewers. Any product that may be evaluated in this article, or claim that may be made by its manufacturer, is not guaranteed or endorsed by the publisher.
Supplementary material
The Supplementary Material for this article can be found online at: https://www.frontiersin.org/articles/10.3389/fbioe.2023.1296880/full#supplementary-material
References
Albert, R. (2007). Network inference, analysis, and modeling in systems biology. Plant. Cell. 19 (11), 3327–3338. doi:10.1105/tpc.107.054700
Altintas, M. M., Eddy, C. K., Zhang, M., McMillan, J. D., and Kompala, D. S. (2006). Kinetic modeling to optimize pentose fermentation in zymomonas mobilis. Biotechnol. Bioeng. 94 (2), 273–295. doi:10.1002/bit.20843
Andersen, M. R., and Nielsen, J. (2009). Current status of systems biology in aspergilli. Fungal Genet. Biol. 46 (Suppl. 1), S180–S190. doi:10.1016/j.fgb.2008.07.006
Aziz, H., and Mohiuddin, S. S. (2022). Biochemistry, hexose monophosphate pathway. Treasure Island (FL: StatPearls.
Barenholz, U., Davidi, D., Reznik, E., Bar-On, Y., Antonovsky, N., Noor, E., et al. (2017). Design principles of autocatalytic cycles constrain enzyme kinetics and force low substrate saturation at flux branch points. Elife 6, e20667. doi:10.7554/eLife.20667
Bertilsson, M., Andersson, J., and Liden, G. (2008). Modeling simultaneous glucose and xylose uptake in saccharomyces cerevisiae from kinetics and gene expression of sugar transporters. Bioprocess. Biosyst. Eng. 31 (4), 369–377. doi:10.1007/s00449-007-0169-1
Carmignotto, G. P., and Azzoni, A. R. (2019). On the expression of recombinant cas9 protein in e. Coli bl21(de3) and bl21(de3) rosetta strains. J. Biotechnol. 306, 62–70. doi:10.1016/j.jbiotec.2019.09.012
Cayir, E., Erdemir, A., Ozkan, E., Topuzogullari, M., Bolat, Z. B., Akat, A., et al. (2014a). Cloning of intron-removed enolase gene and expression, purification, kinetic characterization of the enzyme from theileria annulata. Mol. Biotechnol. 56 (8), 689–696. doi:10.1007/s12033-014-9747-z
Cayir, E., Erdemir, A., Ozkan, E., Topuzogullari, M., Bolat, Z. B., Akat, A., et al. (2014b). Cloning of intron-removed enolase gene and expression, purification, kinetic characterization of the enzyme from theileria annulata. Mol. Biotechnol. 56 (8), 689–696. doi:10.1007/s12033-014-9747-z
Covert, M. W., Schilling, C. H., Famili, I., Edwards, J. S., Goryanin, I. I., Selkov, E., et al. (2001). Metabolic modeling of microbial strains in silico. Trends biochem. Sci. 26 (3), 179–186. doi:10.1016/s0968-0004(00)01754-0
Davidi, D., Noor, E., Liebermeister, W., Bar-Even, A., Flamholz, A., Tummler, K., et al. (2016). Global characterization of in vivo enzyme catalytic rates and their correspondence to in vitro kcat measurements. Proc. Natl. Acad. Sci. U. S. A. 113 (12), 3401–3406. doi:10.1073/pnas.1514240113
Drager, A., Kronfeld, M., Ziller, M. J., Supper, J., Planatscher, H., Magnus, J. B., et al. (2009). Modeling metabolic networks in c. Glutamicum: a comparison of rate laws in combination with various parameter optimization strategies. BMC Syst. Biol. 3, 5. doi:10.1186/1752-0509-3-5
Feierabend, M., Renz, A., Zelle, E., Noh, K., Wiechert, W., and Drager, A. (2021). High-quality genome-scale reconstruction of corynebacterium glutamicum atcc 13032. Front. Microbiol. 12, 750206. doi:10.3389/fmicb.2021.750206
Feng, S., Han, S., Yalan, N., Lei, Z., and Yongfu, L. (2017). Transaminase encoded by ncgl2515 gene of corynebacterium glutamicum atcc13032 is involved in γ-aminobutyric acid decomposition. Process Biochem. 55, 55–60. doi:10.1016/j.procbio.2017.01.016
Gombert, A. K., and Nielsen, J. (2000). Mathematical modelling of metabolism. Curr. Opin. Biotechnol. 11 (2), 180–186. doi:10.1016/s0958-1669(00)00079-3
Gomez-Manzo, S., Marcial-Quino, J., Vanoye-Carlo, A., Serrano-Posada, H., Ortega-Cuellar, D., Gonzalez-Valdez, A., et al. (2016). Glucose-6-phosphate dehydrogenase: update and analysis of new mutations around the world. Int. J. Mol. Sci. 17 (12), 2069. doi:10.3390/ijms17122069
Guo, F. (2010). Studies on sjpgam of schistosoma japonicum. master. [Beijing: Chinese Academy of Agricultural Sciences.
Jiang, F., Yan, J., Zhang, R., Ma, X., Bao, Y., Gu, Y., et al. (2021). Functional characterization of a novel heterozygous mutation in the glucokinase gene that causes mody2 in Chinese pedigrees. Front. Endocrinol. 12, 803992. doi:10.3389/fendo.2021.803992
Kallscheuer, N., Kage, H., Milke, L., Nett, M., and Marienhagen, J. (2019). Microbial synthesis of the type I polyketide 6-methylsalicylate with Corynebacterium glutamicum. Appl. Microbiol. Biotechnol. 103 (23–24), 9619–9631. doi:10.1007/s00253-019-10121-9
Kogure, T., Kubota, T., Suda, M., Hiraga, K., and Inui, M. (2016). Metabolic engineering of corynebacterium glutamicum for shikimate overproduction by growth-arrested cell reaction. Metab. Eng. 38, 204–216. doi:10.1016/j.ymben.2016.08.005
Kuschmierz, L., Shen, L., Brasen, C., Snoep, J., and Siebers, B. (2022). Workflows for optimization of enzyme cascades and whole cell catalysis based on enzyme kinetic characterization and pathway modelling. Curr. Opin. Biotechnol. 74, 55–60. doi:10.1016/j.copbio.2021.10.020
Li, Y., Cong, H., Liu, B., Song, J., Sun, X., Zhang, J., et al. (2016). Metabolic engineering of corynebacterium glutamicum for methionine production by removing feedback inhibition and increasing nadph level. Ant. Van. Leeuwenhoek. 109 (9), 1185–1197. doi:10.1007/s10482-016-0719-0
Mathur, D., Ahsan, Z., Tiwari, M., and Garg, L. C. (2005). Biochemical characterization of recombinant phosphoglucose isomerase of mycobacterium tuberculosis. Biochem. Biophys. Res. Commun. 337 (2), 626–632. doi:10.1016/j.bbrc.2005.09.092
Mathur, D., Malik, G., and Garg, L. C. (2006). Biochemical and functional characterization of triosephosphate isomerase from mycobacterium tuberculosis h37rv. FEMS Microbiol. Lett. 263 (2), 229–235. doi:10.1111/j.1574-6968.2006.00420.x
Milanes, J. E., Suryadi, J., Abendroth, J., Van Voorhis, W. C., Barrett, K. F., Dranow, D. M., et al. (2019). Enzymatic and structural characterization of the naegleria fowleri glucokinase. Antimicrob. Agents. Chemother. 63 (5), e02410-18. doi:10.1128/AAC.02410-18
Moreno-Sanchez, R., Encalada, R., Marin-Hernandez, A., and Saavedra, E. (2008). Experimental validation of metabolic pathway modeling. FEBS J. 275 (13), 3454–3469. doi:10.1111/j.1742-4658.2008.06492.x
Myers, T. D., and Palladino, M. J. (2023). Newly discovered roles of triosephosphate isomerase including functions within the nucleus. Mol. Med. 29 (1), 18. doi:10.1186/s10020-023-00612-x
Niu, J., Mao, Z., Mao, Y., Wu, K., Shi, Z., Yuan, Q., et al. (2022). Construction and analysis of an enzyme-constrained metabolic model of corynebacterium glutamicum. Biomolecules 12 (10), 1499. doi:10.3390/biom12101499
Ortiz-Ramirez, P., Hernandez-Ochoa, B., Ortega-Cuellar, D., Gonzalez-Valdez, A., Martinez-Rosas, V., Morales-Luna, L., et al. (2022). Biochemical and kinetic characterization of the glucose-6-phosphate dehydrogenase from helicobacter pylori strain 29cap. Microorganisms 10 (7), 1359. doi:10.3390/microorganisms10071359
Oshiro, M., Shinto, H., Tashiro, Y., Miwa, N., Sekiguchi, T., Okamoto, M., et al. (2009). Kinetic modeling and sensitivity analysis of xylose metabolism in lactococcus lactis io-1. J. Biosci. Bioeng. 108 (5), 376–384. doi:10.1016/j.jbiosc.2009.05.003
Reddy, G. K., and Wendisch, V. F. (2014). Characterization of 3-phosphoglycerate kinase from corynebacterium glutamicum and its impact on amino acid production. BMC Microbiol. 14, 54. doi:10.1186/1471-2180-14-54
Rudolph, B., Hansen, T., and Schonheit, P. (2004). Glucose-6-phosphate isomerase from the hyperthermophilic archaeon methanococcus jannaschii: characterization of the first archaeal member of the phosphoglucose isomerase superfamily. Arch. Microbiol. 181 (1), 82–87. doi:10.1007/s00203-003-0626-4
Sangolgi, P. B., Balaji, C., Dutta, S., Jindal, N., and Jarori, G. K. (2016a). Cloning, expression, purification and characterization of plasmodium spp. Glyceraldehyde-3-phosphate dehydrogenase. Protein. Expr. Purif. 117, 17–25. doi:10.1016/j.pep.2015.08.028
Sangolgi, P. B., Balaji, C., Dutta, S., Jindal, N., and Jarori, G. K. (2016b). Cloning, expression, purification and characterization of plasmodium spp. Glyceraldehyde-3-phosphate dehydrogenase. Protein. Expr. Purif. 117, 17–25. doi:10.1016/j.pep.2015.08.028
Schachner, L. F., Soye, B. D., Ro, S., Kenney, G. E., Ives, A. N., Su, T., et al. (2022). Revving an engine of human metabolism: activity enhancement of triosephosphate isomerase via hemi-phosphorylation. ACS Chem. Biol. 17 (10), 2769–2780. doi:10.1021/acschembio.2c00324
Shang, Y., Qi, J., Wang, Y., Li, H., Liu, X., Wang, S., et al. (2021). Cloning, expression and enzymatic activity of mycoplasma synoviae phosphoglycerate kinase. Chin. J. Animal Infect. Dis. 29 (03), 71–79. doi:10.19958/j.cnki.cn31-2031/s.2021.03.011
Shcherbakova, T. A., Baldin, S. M., Shumkov, M. S., Gushchina, I. V., Nilov, D. K., and Svedas, V. K. (2022). Isolation and biochemical characterization of recombinant transketolase from mycobacterium tuberculosis. Acta Naturae 14 (2), 93–97. doi:10.32607/actanaturae.11713
Sprenger, G. A., Schorken, U., Sprenger, G., and Sahm, H. (1995). Transaldolase b of escherichia coli k-12: cloning of its gene, talb, and characterization of the enzyme from recombinant strains. J. Bacteriol. 177 (20), 5930–5936. doi:10.1128/jb.177.20.5930-5936.1995
Sternisha, S. M., and Miller, B. G. (2019). Molecular and cellular regulation of human glucokinase. Arch. Biochem. Biophys. 663, 199–213. doi:10.1016/j.abb.2019.01.011
Tan, Z., Chen, J., and Zhang, X. (2016). Systematic engineering of pentose phosphate pathway improves escherichia coli succinate production. Biotechnol. Biofuels. 9, 262. doi:10.1186/s13068-016-0675-y
Tohsato, Y., Ikuta, K., Shionoya, A., Mazaki, Y., and Ito, M. (2013). Parameter optimization and sensitivity analysis for large kinetic models using a real-coded genetic algorithm. Gene 518 (1), 84–90. doi:10.1016/j.gene.2012.11.080
Xue-ling, W. U., Bo, M., Jian, H., Qi, H. U., Jia, Z., Yuan-dong, L., et al. (2010). Purification and enzymatic properties of arsenic resistance protein arsh from heterogeneous expression in e.coli bl21. Trans. Nonferrous Metall. Soc. China. 20 (10), 1987–1992. doi:10.1016/S1003-6326(09)60406-4
Zha, J., Zhao, Z., Xiao, Z., Eng, T., Mukhopadhyay, A., Koffas, M. A., et al. (2023). Biosystem design of Corynebacterium glutamicum for bioproduction. Curr. Opin. Biotechnol. 79, 102870. doi:10.1016/j.copbio.2022.102870
Zhang, Y., Cai, J., Shang, X., Wang, B., Liu, S., Chai, X., et al. (2017). A new genome-scale metabolic model of corynebacterium glutamicum and its application. Biotechnol. Biofuels. 10, 169. doi:10.1186/s13068-017-0856-3
Zinsser, V. L., Hoey, E. M., Trudgett, A., and Timson, D. J. (2013). Biochemical characterisation of triose phosphate isomerase from the liver fluke fasciola hepatica. Biochimie 95 (11), 2182–2189. doi:10.1016/j.biochi.2013.08.014
Keywords: Corynebacterium glutamicum, EMP pathway, HMP pathway, enzyme kinetics parameters, metabolic network models
Citation: Yang L, Li J, Zhang Y, Chen L, Ouyang Z, Liao D, Zhao F and Han S (2023) Characterization of the enzyme kinetics of EMP and HMP pathway in Corynebacterium glutamicum: reference for modeling metabolic networks. Front. Bioeng. Biotechnol. 11:1296880. doi: 10.3389/fbioe.2023.1296880
Received: 19 September 2023; Accepted: 13 November 2023;
Published: 28 November 2023.
Edited by:
Hao Song, Tianjin University, ChinaReviewed by:
Karl Kochanowski, Centre de Recerca en Sanitat Animal (CReSA), SpainHui-Min Qin, Tianjin University of Science and Technology, China
Copyright © 2023 Yang, Li, Zhang, Chen, Ouyang, Liao, Zhao and Han. This is an open-access article distributed under the terms of the Creative Commons Attribution License (CC BY). The use, distribution or reproduction in other forums is permitted, provided the original author(s) and the copyright owner(s) are credited and that the original publication in this journal is cited, in accordance with accepted academic practice. No use, distribution or reproduction is permitted which does not comply with these terms.
*Correspondence: Fengguang Zhao, Zmd6aGFvQHNjdXQuZWR1LmNu; Shuangyan Han, c3loYW5Ac2N1dC5lZHUuY24=