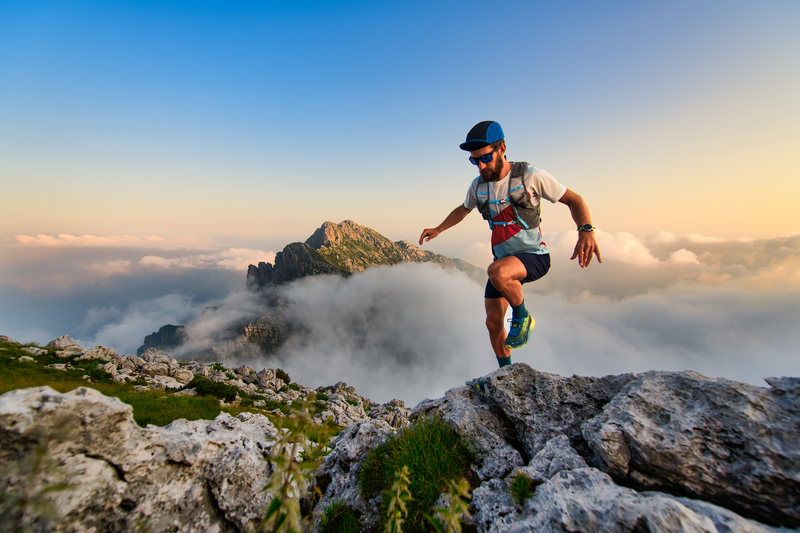
95% of researchers rate our articles as excellent or good
Learn more about the work of our research integrity team to safeguard the quality of each article we publish.
Find out more
REVIEW article
Front. Bioeng. Biotechnol. , 06 November 2023
Sec. Biomaterials
Volume 11 - 2023 | https://doi.org/10.3389/fbioe.2023.1260397
This article is part of the Research Topic Organ Mimicking Technologies and Their Applications in Drug Discovery View all 7 articles
Many neurodegenerative diseases are identified but their causes and cure are far from being well-known. The problem resides in the complexity of the neural tissue and its location which hinders its easy evaluation. Although necessary in the drug discovery process, in vivo animal models need to be reduced and show relevant differences with the human tissues that guide scientists to inquire about other possible options which lead to in vitro models being explored. From organoids to organ-on-a-chips, 3D models are considered the cutting-edge technology in cell culture. Cell choice is a big parameter to take into consideration when planning an in vitro model and cells capable of mimicking both healthy and diseased tissue, such as induced pluripotent stem cells (iPSC), are recognized as good candidates. Hence, we present a critical review of the latest models used to study neurodegenerative disease, how these models have evolved introducing microfluidics platforms, 3D cell cultures, and the use of induced pluripotent cells to better mimic the neural tissue environment in pathological conditions.
Neurodegenerative diseases are defined as the progressive loss of neuronal cells and the synapses formed between them, mainly due to the accumulation of proteins such as amyloid beta, tau, or alpha-synuclein (Kovacs, 2019). One percent of global mortality is ascribed to neurodegenerative diseases. Projections indicate an upward trend in these numbers with each passing year, primarily due to the extended lifespan of the global population. (Badhe et al., 2018). The number of people with dementia in 2018 was 50 million., two-thirds of these patients go for Alzheimer’s while Parkinson’s disease, epilepsy, Huntington’s, and motor neuron diseases like amyotrophic lateral disease (ALS) are the other one-third (Patterson, 2018). As neurodegenerative diseases do not provoke immediate death, patients’ care treatment tends to last a long period, in which their quality of life decreases with a great economic and emotional price. Consequently, patients and their families are under a big financial burden mainly because they not only need medication but also special care provided only by health professionals and external institutions, worldwide estimated cost of 1 trillion US dollars (Patterson, 2018; Cimler et al., 2019). Nonetheless, the numbers for this disease are predicted to triplicate until 2050 and the costs to double by 2030 due to the aging of the population and the increase in the lifespan. (Patterson, 2018).
Even though the discovery of these diseases goes back 100 years, they are still difficult to diagnose and treat (Möller and Graeber, 1998). This is also reflected in the drug development for their treatment. Drug discovery and validation are lengthy and costly processes, mainly due to the strict control necessary to avoid side effects, reducing the number of efficient trials. The billions of dollars invested in drug development are not translated into a high number of drugs approved and the main reason for this is that many preclinical models are not physiologically relevant, failing frequently in the prediction of drug effectiveness in humans (Ahadian et al., 2017). Animal models have been the gold standard in drug development as they allow for testing of drug effectiveness and selectivity in a living organism. However, these models are expensive, time-consuming, ethically questionable, and most of the time unpredictable as clinical trials fail due to the physiological differences between humans and animals (Zhuang et al., 2017). Ineffective drugs must be uncovered in the earlier stages of the process to reduce these costs and the lives of the animals used during the drug development. More efficient drug development can be accomplished through the use of in vitro models capable of recreating the physiological characteristics necessary to test the efficacy and safety of a new drug (Breslin and O’Driscoll, 2013). Understanding the architecture of the tissue, the different cells involved and how they interact is key when these in vitro models are developed to mimic a biologically healthy/diseased tissue or organ. In this critical review we want to cover the main features involved when developing an in vitro model for neurodegenerative diseases, aiming attention to the models that use induced pluripotent stem cells (iPSC) and microfluidic as the most versatile engineering process to mimic human tissue (Garcia-Leon et al., 2019).
Recreating a reliable in vitro model of the brain is a hard task because it is complex to assemble different types of cells, considering cell-cell interactions and the extracellular matrix (ECM). Neurons, despite being the more popular cells, are not the most abundant in the brain, a position occupied by the glia cells family (Barres, 2008). (Fang et al., 2019)This group of cell types encompasses astrocytes and oligodendrocytes, which play roles in supporting and nourishing neurons (Fang et al., 2019). Additionally, microglia protect the neurons and their synapsis, contributing constantly to homeostasis. Increasing evidence suggests that in the case of neuroinflammation, these cells support neuronal destruction and can be active players in the course of neurodegenerative disease (Tejera and Heneka, 2019). Other types of cells found in the brain are the neural stem cells (located mainly in the ventral-hypocavitary zone and the hippocampus) and the endothelial cells. The first can differentiate in any neuronal cell type to replace injured or lost ones and the second ones are the main component of the brain vasculature, being essential for the survival of this organ (Pamies et al., 2014). In the case of the functional units of the Blood-Brain Barrier (BBB), pericytes are also present as they are essential for the maintenance of the barrier functions (van der Helm et al., 2016). The constant communication between these cell types is fundamental for the development and the proper function of the brain but they are not easy to mimic in vitro. The ECM is valuable for its specific characteristics, formed by lecticans, proteoglycans with a lectin domain, and a hyaluronic acid-binding domain (Oohashi et al., 2015). The native composition of this matrix is characterized by bioactive cues in components such as laminin that influence neural processes as well as particular mechanical characteristics, such as its stiffness that affect the cells. A particular paragraph about this matrix will follow in the next section.
Animal cells are the source most used for neurodegenerative disease models and drug development due to their availability and extended knowledge of their phenotypes, but they do not fully predict the complexity of human cells neither in healthy or diseased tissues (Oddo et al., 2019). Primary cells are usually harvested from human or animal tissue (although there are some commercially available options), proving a high functional output and reflecting what happens in physiological conditions (Stacey, 2006). However, these cells are difficult to purify, they grow at a slower rate, and they can lose their phenotype in culture (Potjewyd et al., 2018). As an alternative to them, Immortalized cell lines, defined as primary cells modified to proliferate indefinitely, are widely used for their ability to grow fast, for maintaining their robustness through a high number of passages, and for their low cost in acquisition and maintenance. Even though these types of cells are very useful, the obtained results can be misleading as they do not render a fair replica of all the cells present in the organ, specifically for transporters, tight junctions, and barrier functions which sometimes can present different values depending on the cell line (Stacey, 2006; Stacey, 2006; Stacey, 2006; Rahman et al., 2016; Potjewyd et al., 2018; Potjewyd et al., 2018; Potjewyd et al., 2018)Besides immortalized cell lines and primary cultures, we can find stem cell cultures but their use is often coupled with ethical problems (Bordoni et al., 2018). Adult stem cell extraction is difficult or even not feasible (Zimmermann and Schaffer, 2019).
Then, human induced pluripotent stem cells (iPSC) arise as a promising cell type to develop more reliable neurological in vitro models. iPSCs can self-renew and differentiate into any cell type of the three germ layers (Takahashi and Yamanaka, 2006). In this particular case, iPSCs are obtained from somatic cells, healthy humans or patients, that go through a reprogramming process when exposed to a specific cocktail of factors, Oct3/4, SOX2, c-Myc, and Klf4 (Takahashi et al., 2007). This not only allows the construction of a more accurate disease model with cells from diseased patients but, also the evaluation of the response to different treatments for personalized medicine as they contain the genetic information of the patient, which immortalized or primary cells might lack (Poon et al., 2017; Vadodaria et al., 2018).
Cultivating diverse cell types not only enhances the intricacy of the model but also fosters the proliferation of these cell types, as their behavior is influenced by interactions with other cell types reminiscent of those found in native tissues. (Jeon et al., 2016; Haenseler et al., 2017). There are several available protocols for iPSC differentiation, but they face problems regarding a high variability in the differentiation (Nierode et al., 2019). The enhancement of purity and specificity can be achieved through the application of CRISPR-Cas9 technology, which enables genetic manipulation for the creation or correction of disease-associated mutations, as discussed by Kelava et al. (Kelava and Lancaster, 2016b). However, it is essential to acknowledge that this process is resource-intensive and time-consuming, involving the reversion of cells to a stem-like state and subsequent differentiation into neural cells. Moreover, its complexity and resource demands can further escalate when replicating age-related diseases, impacting the model and potentially leading to hypermethylation (Ghaffari et al., 2018). Figure 1 gives an overview of the iPSCs for different types of models. They differ in terms of (a) their architecture and dimension, (2D or 3D); (b) the absence of scaffolds for spheroids and organoids; (c) the presence of biomaterials representative for scaffold-based cultures; (d) the compartmentalization of the cells culture and (e) presence of physical and chemical cues such as fluid flow offered by microfluidic devices.
FIGURE 1. The use of human induced pluripotent stem cells (hiPSC) in different types of neuronal cell culture. Somatic cells are harvested from patients and reprogrammed using the cocktail of factors Oct4, Sox2, Klf4, and c-Myc until the pluripotent state. From that state, iPSC can be differentiated into the main cell types present in the brain. 2D culture plates, spheroids, organoids, scaffold-based culture, and organ-on-a-chip are the main examples of hiPSC culture approached in this review for neurodegenerative diseases (Illustrations obtained from Smart Servier Clinical Art).
ECM is another key component when building an in-vitro model for brain and neurodegenerative diseases because it includes mechanic and chemical cues that influence cell behavior. ECM takes around 20% of the space in the brain. (Rauch, 2004) It is responsible for the low Young’s modulus (around 1 kPa) of this tissue. The glycosaminoglycans (proteoglycans and HA) contribute to the gel-like consistency and viscoelastic properties of this matrix while hydrating it. The low content of elastic fibers and collagen, when compared to other tissues of the body, also contributes to the low stiffness of this tissue (Engler et al., 2006; Shin et al., 2017; Wu et al., 2017)). When building the in-vitro model, it is important to replicate the low stiffness of the matrix since neurons tend to prefer softer substrates, but the glia cells prefer stiffer substrates (Her et al., 2013). This divergence is related to the difference of young moduli between the white and grey matter regions of the brain. On the white matter region, the majority of cells are glia and neurons’ axons, being the stiffer region, with an elastic modulus around 1.9 kPa. On the other side, the grey matter region has a lower elastic modulus (around 1.4 kPa) and it is composed of neuronal cell bodies (Budday et al., 2015).
Bioactive cues, such as growth factors or peptides naturally present in the brain ECM, are necessary to mimic the real environment (Gumera et al., 2011). Accordingly, it is common to introduce molecules such as laminin, collagen, or fibronectin in the cell culture as they stimulate cell growth, migration, adhesion, and differentiation. Laminin is one of the most used proteins in brain models since they present specific domains that promote neuronal survival and axonal growth while other domains benefit adhesion and not growth (Edgar et al., 1984; Gumera et al., 2011). Fibronectin and HA are two other examples of bioactive molecules used in neural cultures for their influence on neuron adhesion, migration, guidance, and extension (Tate et al., 2009; Khaing and Seidlits, 2015). The use of small peptides facilitates the integration of the cells while not taking too much space or unnecessary binding sites, as well as avoids disease transmission or batch variation that can appear while isolating the whole protein (Rao and Winter, 2009; Gumera et al., 2011).
In addition to ECM composition, brain tissue architecture, and mechanical factors are also a great influence on neuronal culture. Architecture in a 3D model is documented as extremely valuable for cell-cell interactions along with cell-matrix interactions (Nguyen-Vu et al., 2007; Sun et al., 2007; Kunze et al., 2011; Duval et al., 2017). Related to this architecture, the space and the shape of the compartment where neurons are cultured affect the axon growth since these cells prefer narrow spaces. In addition to topographical cues, the influence of mechanical factors such as stretch and strain plays a significant role in promoting the alignment of neuronal cells and axon growth. The application of fluid flow and shear stress is equally noteworthy in affecting these cultures, especially in modulating their differentiation process. These mechanical factors offer a more realistic simulation of the interactions observed with cerebrospinal fluid in in vivo conditions (Pedersen et al., 2007; Rambani et al., 2009; Shin et al., 2009; Peyrin et al., 2011; Choi et al., 2013; Jenkins et al., 2015; Tsao et al., 2015; Mei et al., 2022).
When cultured in 3D models, cells present biological processes such as differentiation, proliferation, or migration more similarly to what is observed in vivo (Baharvand et al., 2004; Liu et al., 2006; Soares et al., 2012). Zhang et al. compared the culture of hIPSC-derived neuroepithelial stem cells culture in a 2D well plate versus a 3D self-assembly peptide matrix when studying Alzheimer’s disease and the results prove that the 3D model was more capable of mimicking the pathological side originated by Aβ oligomers (Zhang et al., 2013). We can find different 3D cell culture models in literature for resemble brain and neuropathological diseases (Murphy et al., 2017) and we can classify them according to their fabrication technology, if they use scaffolds as support where cells are seeded on top or embedded in the biomaterial or if the three-dimensionality comes from a cell aggregate (scaffold-free). In the scaffold-based models, the biomaterial is fundamental to introduce mechanical and biochemical cues to the cell culture while in cell aggregates the close interaction of the cells and their self-organization during the differentiation process allows the development of a neural model.
An ideal scaffold should keep its integrity for enough time to allow cells to self-organize, communicate, and recruit other cells, neurons, or glia. The perfect scenario would be the one presenting a scaffold that stays the time needed for the cells to produce their own ECM to replace it (Knowlton et al., 2018). Several models possess fibers, pores, or channels to stimulate communication within the cells.
The optimal bioaterials for neural culture should be biocompatible, easy to manipulate, capable of chemical or physical modification; allow the permeation of oxygen, nutrients, and electrical conductance; reproducible, and not too expensive (Potter et al., 2008). Taking into consideration the mechanical stiffness and structure of the brain ECM, softer materials are preferred to mimic the consistency of this tissue (Wen et al., 2018). Besides the mechanical strength to support the cells, the scaffold should also have a porosity appropriated for cell migration without compromising its mechanical integrity (Huang et al., 2011).
Hydrogels are one of the most used biomaterials in neural culture. and are described as polymeric networks created through chemical and physical crosslinking with high water content and permeability (Kajtez et al., 2021; Hull et al., 2022). They are biocompatible and flexible in their physical characteristics and composition which allows them to be used in several applications such as cell culture, cell therapy, and drug delivery (Jose et al., 2019; Neves et al., 2020; Poorna et al., 2021). Hydrogels can be tuned to encompass biochemical and biophysical cues present in the brain to promote dynamic interaction between cells and their environment They can be classified as natural, synthetic, or hybrid based on their origin. In Table 1 we show some examples of different types of materials used for the fabrication of scaffolds in neuronal stem cell culture. They are classified by their origin, their production technique, and the cell type used. We also pay attention to their application.
TABLE 1. Examples illustrate the use of various polymers in induced pluripotent stem cell models for neuronal culture, each chosen based on its specific application.
Natural polymers are obtained from plants or animals an incorporate the molecules present in the native tissue ECM. Some of the most used natural polymers in neural models are gelatine (Baiguera et al., 2014), alginate (Mosahebi et al., 2001), collagen (Sundararaghavan et al., 2009; Cho et al., 2018), hyaluronic acid (Liang et al., 2013; Wu et al., 2017), Matrigel® (Choi et al., 2014) silk (Hopkins et al., 2013), cellulose (Pértile et al., 2012). However, these materials are difficult to purify and sterilize, processes that can even denature the proteins present in the tissue (Jorfi et al., 2018a). In consequence, there is less control over their physical and chemical properties, as well as their degradation rates, when compared with synthetic biomaterials, presenting differences from batch to batch. Nonetheless, the incorporation of a variety of materials can be advantageous in attaining the intended scaffold structure. (Kuo and Wang, 2013).
Führmann et al. used a collagen type-I scaffold for a nerve regeneration model where 2D and 3D architecture were tested for axon growth, thus promoting the effects of human neural progenitor-derived astrocytes found in dorsal root ganglion axon regeneration. The results showed greater axon regeneration on 3D culture when compared to laminin-coated subtract that acted as a positive control. The 3D scaffolds where hNP-astrocytes were seeded with dorsal root ganglion explants showed greater axon regeneration than those without astrocytes. The porosity and the topography of the scaffold also contributed to better communication with the astrocytes, and the migration of Schawn cells and the fibroblast (Führmann et al., 2010). Moxon et al. also used collagen in combination with alginate to create a tuneable biomaterial for 3D neuronal culture. The results showed successful incorporation of the collagen fibrils on the scaffold structure as well and adherent human iPSC-derived neurons were capable of creating complex neural networks. The gene expression of the cells was also influenced by the mechanical tuning of the hydrogel stiffness (Moxon et al., 2019).
Inspired by a marine mussel, Hong et al. developed a hyaluronic acid catechol biopolymer stable and compatible with neural stem cell culture and with adhesive and cohesive properties depending on the pH. These make this biopolymer interesting for 2D cell culture as well as for 3D when incorporated into gels (Hong et al., 2013). Madl et al. produced hydrogels composed of elastin-like proteins with RGD bioactive domains with different stiffnesses and degradability. The influence of these characteristics on neuronal progenitor cells (NPC) stemness was assessed. Remarkably, it was dependent on the degradability and not the stiffness of the substrate (Madl et al., 2017). Later, they also reported NPC cultures in proteolytically degradable hydrogels that express neuronal and astrocytic markers, maturating to neurotransmitter-responsive neurons only when there is degradation of the matrix occurred before the differentiation (Madl et al., 2019).
One of the most natural materials used as a coating in iPSC culture is Matrigel® where human neural progenitor cells have a higher survival rate when differentiating into neurons (Kaiser et al., 2019). Kim et al. utilized Matrigel® as a 3D scaffold for the culture of human-derived neurons expressing familial AD mutations. This model could reproduce not only the aggregation of amyloid-β but also the accumulation of hyperphosphorylated tau characteristic of the disease (Choi et al., 2014). Miguel et al. also used Matrigel® in the form of capsules coated with alginate for modeling tauopathies. The results identified the switch in Tau splicing along the differentiation of iPSC-derived neurons as well as proved the iPSC-induced neurons were able to differentiate into cortical neurons within the capsules (Miguel et al., 2019).
On the other hand, synthetic materials are more easily tuneable when it comes to their physical and chemical properties as their exact composition is known and can be adjusted. The mechanical properties and degradation rate can be controlled by changing parameters such as molecular weight or crosslinking level. This ability makes them much more reproducible when compared to natural polymers (Zimmermann and Schaffer, 2019). Polyethylene glycol (PEG) (Namba et al., 2009), poly 2-hydroxyethyl methacrylate (Plant et al., 1995), and RADA-16 (Zhang et al., 2013)are some examples. are some examples.
Cerrone et al. used electrospinning as a technique to create polyhydroxyphenylvalerate (PHPV) with polycaprolactone (PCL) nanofibers and evaluate their influence on neurite outgrowth of human iPSC. The results showed that this conjugation of synthetic materials increased the cells’ lifespan up to 2.3-fold and the neurite elongation by 3.8-fold. The migration of the cells was also increased when compared to PCL alone (Cerrone et al., 2020). Crowe et al. used polyethylene glycol diacrylate with Irgacure 2,959 (PEG2959) and Dental LT Clear (DClear) as suitable biomaterials using a two-photon polymerization technique to create a scaffold for neural culture. This scaffold was biocompatible with the network development of these cells, facilitating observation using imaging techniques. The results showed a scaffold that supported the growth, differentiation, and alignment of the cells while being capable of assessing the individual cells optically (Crowe et al., 2020). Ranjan et al. developed a 3D microfibrous scaffold where AD patient iPSC derived NPC was encapsulated in poly (lactic-co-glycolic acid) (PLGA). Results showed reduced cell proliferation and significant acceleration in neuronal differentiation when compared to 2D cultures. In addition, the 3D culture demonstrated higher levels of pathogenic amyloid-beta 42 and phospho-tau in cultures where neurons carried familial AD mutations when compared to heathy neurons (Ranjan et al., 2020).
However, synthetic materials do not possess biomolecules such as adhesion ligands or proliferation and differentiation promotors, limiting the interaction between neural cells and scaffolds. An easy solution for this downside is to undergo biochemical modifications in the materials or combine them with natural polymers to insert biomolecules such as growth factors or adhesion molecules to create a scaffold more similar to the ECM (Aurand et al., 2014). Cunha et al. combined RADA 16-I based self-assembly peptides with RGD and laminin-derived motifs, BMHP1 and BMHP2, to create a 3D scaffold for the culture of neuro stem cells. The cells were found to be viable, and proliferative and they differentiated on this scaffold (Cunha et al., 2011). However we need to be aware that the products of synthetic material degradation can become toxic for the cell culture as they do not have a natural origin (Zimmermann and Schaffer, 2019).
The methacrylation of natural polymers, such as gelatin, serves as a facilitating mechanism for the covalent linkage of multiple polymers, whether they are of natural or synthetic origin. (Yue et al., 2015). Hybrid scaffolds combine the best properties of natural and synthetic polymers, being reproducible, easy manipulation of their physical and chemical properties, and high affinity for the cells.
Cantley et al. showed the combination of different materials to create a 3D neural model. The scaffold was composed of a sponge of fibrin coated with polyornithine and laminin, natural polymers known to influence neural cell culture, to which collagen was added to support the cell culture. This model permitted the culture of healthy and patient-derived iPSC providing a functional network of interconnected neurons and astrocytes after 5 days of culture. Stem cells derived from Alzheimer’s and Parkinson’s disease patients expressed growth and gene expression similar to the native disease tissue, proving that this scaffold was suitable for neurodegenerative disease modeling (Cantley et al., 2018). Zhang et al. developed a model using methacrylate-modified hyaluronic acid to compare the maturation and differentiation of human iPSC-derived neural progenitors from both healthy donors and Rett syndrome patients. The results demonstrated accelerated maturation and differentiation of the healthy cells while MeCP2-mutant cells were defective in migration, having a slower differentiation rate with defective neurite outgrowth and synaptogenesis (Zhang et al., 2016). Karvinen et al. created a scaffold adequate for neural cell culture composed of hyaluronan-polyvinyl alcohol (HA-PVA) and alginate-polyvinyl alcohol (AL-PVA). The results proved that the scaffolds were tunable, with a similar stiffness to brain tissue. The presence of HA enhanced the neuronal growth of iPSC-derived neurons (Karvinen et al., 2018).
Another option for scaffold fabrication in cell culture is decellularized ECM. This type of matrix is characterized by the maintenance of the intact native ECM molecules of the tissue without the cellular components where other cell types, including from other species, can be cultured. Removing the original cells present in the tissue allows the escape from the immune and inflammatory responses a scaffold could have when new cells are added to the system while mimicking better the cell environment by maintaining the architecture and biomolecules present in the ECM (Kabirian and Mozafari, 2019). Decellularized ECM can be used the moment after extracting the cells present or lyophilized and pulverized during the purification process and later solubilized to be used as scaffold material (Jang et al., 2017; Santschi et al., 2019). Sood et al. studied the difference between using fetal and adult decellularized brains on the differentiation and functional maturation of hiPSC. Fetal ECM brain showed the best results for the maintenance of differentiated neurons with better activity. in long-term culture Astrocytes were also present during the second month of differentiation, proving to be less toxic for the culture. The biochemical cues of both fetal and adult decellularized brains were assessed, showing properties similar to native tissue (Sood et al., 2019).
Obtaining intact decellularized ECM scaffolds can be challenging due to the potential damage inflicted on native components or the alteration of mechanical properties during the decellularization process. DeQuach et al. developed a process to decellularize the porcine brain using detergents capable of keeping several isoforms of collagen, glycosaminoglycans, perlecan, and laminin and be used later in a neural cell culture. This material was proven to be appropriate as a coating of iPSC-derived neurons which expressed normal neuronal markers as well as morphology (DeQuach et al., 2011; Sood et al., 2019) Decellularized ECM can also be coupled with other materials to enhance their performance. Barroca et al. combined decellularized ECM from the adipose tissue with reduced graphene oxide through thermally induced phase separation aided by carbodiimide crosslinking to study the effect of on neural culture since this particular source of ECM had not been exploited for this cell culture. The scaffold proved to benefit from the protein-rich ECM and structural support provided by the reduced graphene oxide where neural stem cells adhered and grown. The concentration of reduced graphene oxide had a direct impact on the cell fate of these stem cells, with higher concentrations inducing neuron differentiation (Barroca et al., 2023).
On the other hand, scaffold-free models use cell aggregates, tissue strands, or cell sheets as building blocks for seeding the cells in 3D environment. As this model does not use any material to build its architecture, the organogenesis/embryogenesis process can produce heterogeneous tissues including complicated architectural structures (Jeong et al., 2020).
Spheroids for neuronal models are characterized by an agglomeration of cells, formed spontaneously or by force when they are cultured in a way that they are unable to attach to a substrate material, like the process that naturally happens in embryonic development where cells self-assembled to form more complex tissues (Laschke and Menger, 2017). One of the most interesting features of spheroids is that they together can produce their own ECM as the cells are forced to interact with each other (Dingle et al., 2015). Besides producing ECM, spheroids recapitulate cell-cell interactions and features of natural tissue such as mechanical stiffness and electrophysiology (Laschke and Menger, 2017). These characteristics make spheroids good candidates to model the brain, for performing drug screening, or for modeling a disease. Sloan et al. generated and combined neural spheroids to form forebrain assembloids to evaluate the interactions of glutamatergic and GABAergic neurons in vivo (Sloan et al., 2018). However, Leite et al. used brain spheroids to assess the neurotoxicity of nanoparticles (Leite et al., 2019). Regarding studies of neurodegenerative diseases, Lee et al. generated 3D neurospheroids from iPSC of Alzheimer patients to characterize the Aβ generation and drug inhibition (Lee et al., 2016).
The cell organization in spheroids promotes the formation of cell-cell junctions which are crucial to keep the structural integrity of the spheroid while facilitating barrier functions. However, these junctions differ from synaptic junctions which are essential for neural communication and signaling. It is then important to assess the formation of tight junctions and synaptic activity for a complete characterization of the brain model (Wilson et al., 2020; Jurek et al., 2022).
Spheroids can also be used as building blocks, coupling them with scaffolds such as hydrogels, matrigel, or collagen in models for brain disease (Tang-Schomer et al., 2018). Xiao et al. used a modified alginate hydrogel to support the culture and differentiation of spheroids generated from neural pluripotent cells derived from mouse iPSC (Xiao et al., 2019). Jorfi et al. used iPSC neurospheroids derived from a familiar mutation of Alzheimer’s disease. Spheroids were cultured in Matrigel for 8 weeks and results showed an accumulation of amyloid-β and phosphorylated tau (Jorfi et al., 2018b).
Similar to them, organoids are also formed of cell aggregates, but the cells are capable of self-organizing and differentiating within the aggregate, creating an organ-like structure and mimicking some of its functions. These body structures are more stable, and they can survive for longer periods when compared to spheroids (Brawner et al., 2017). There are two different ways to produce a brain organoid, the undirected method where the stem cells self-organize, or the directed method where the differentiation is externally conditioned by the addition of molecules and factors. The first method originates greater cell diversity within the aggregate while the last allows the regional development of specific organoids (Hartlaub et al., 2019). Then these brain organoids can be fused to study their interactions.
These organoids are used in several types of studies such as fetal development, cell-cell interactions, and/or drug screening (Lancaster et al., 2013; 2017; Quadrato et al., 2017; Zhou et al., 2017; Arlotta, 2018). Bagley et al. developed ventral and dorsal forebrain cerebral organoids from hPSCs and co-cultured them to recreate the dorsal-ventral axis. Results showed the migration of GABAergic neurons from the ventral to the dorsal forebrain by using a labeling technique adequate to study neuronal migration (Bagley et al., 2017). Raja et al. developed a protocol for the culture of organoids derived from AD patients’ iPSCs which recapitulated characteristic features of the pathology, i.e., hyperphosphorylated tau protein, amyloid aggregation, and endosome abnormalities (Raja et al., 2016). Alternatively, Abud et al. used organoids to evaluate the function of microglia-like cells derived from iPSC in neurological diseases; specifically, targeting the effect of Aβ fibrils, tau oligomers, and gene expression, also related to AD (Abud et al., 2017). Wulansari et al. developed midbrain-like organoids using hESC with DNAJC6 mutation (identified in early-onset PD patients). The model was capable of replicating midbrain-type dopamine neuron degeneration, aggregation of pathologic α-synuclein, mitochondrial and lysosomal dysfunctions, and increase of intrinsic neuronal firing frequency (Wulansari et al., 2021).
One of the disadvantages of organoids and spheroids is that the center of the organoid can be absent of oxygen and nutrient transportation as the vasculature-like networks are not properly developed, limiting their culture time (Kelava and Lancaster, 2016a; Eglen and Reisine, 2019). This inability to culture organoids for long periods is also a problem for neurodegenerative disease studies. These diseases are characterized by late onset, so the culture time of these organoids might not be sufficient to grow mature neurons and glia cells to display the characteristics of diseased cells (Gopalakrishnan, 2019). To overcome this difficulty, there is a need to find a manner to perfuse the cells with a culture medium. Currently, bioreactors with agitation have been used to ensure oxygenation, allowing longer culture periods (Qian et al., 2016). To induce the vascularization of brain organoids, Pham et al. embedded endothelial cells from patients on 34-day older iPSC organoids with Matrigel®. These organoids showed robust vascularization ether during the 3–5 weeks of in vitro culture and 2 weeks after transplantation on mice (Pham et al., 2018). Another disadvantage of organoids is the lack of control of the shape and size when forming the structures since the organoid replicas must be as similar to each other as possible to validate the results observed from these experiments (Jeong et al., 2022).
Another complexity of the use of these cell-based models is the difficulty of real-time optical monitoring. Live or time-lapsing imaging is possible but to assess the center of the aggregate, researchers use histological and immunohistochemical analysis (Lancaster et al., 2017), meaning that the organoid has to be cut into slices to allow better diffusion of the staining agents and microscope visualization (Lancaster and Knoblich, 2014).
Among the fabrication techniques for developing 3D models, 3D printing arises as an innovative and useful tool to produce scaffolds. It is described as the process where thin layers are deposited in a substrate to be cured later, producing a 3D structure. When referring to bioprinting, cells and materials are printed together. One of the most interesting features of this bioprinting approach for neural models is the precise control of the deposition site of both materials and cells, allowing the recreation of the brain tissue architecture more realistically (de la Vega et al., 2019).
Several critical parameters must be taken into consideration when engaging in bioprinting for neural models, including the selection of ink with its relevant rheological properties, nozzle diameter, operational temperature, and radiation. (Ding et al., 2018; Heidarian et al., 2019). The printing process may lead to reduced cell viability, particularly when working with sensitive cell types like neural cells, especially when rigid materials are employed. To mitigate this, the use of shear-thinning bioinks, such as hydrogels, is preferable, as they offer better cell protection. Neural cells exhibit a preference for softer biomaterials as substrates or scaffolds, and hydrogels present favorable mechanical properties and a wide range of available polymers, making them suitable candidates (Cadena et al., 2021).
In addition to the choice of material, these bioinks can be enriched with bioactive cues to enhance neural cell survival, proliferation, and differentiation. Utilizing materials with a low Young’s modulus may compromise scaffold integrity, impeding the creation of layered structures or achieving substantial dimensions in 3D models (Her et al., 2013). The recurrent issue of structural collapse presents a challenge, particularly when working with soft materials, thereby complicating the construction of scaffolds. (Hospodiuk et al., 2017; Oliveira et al., 2019). The nozzle diameter is also important since small diameters allow a better resolution but might suffer clogging (Chang et al., 2008; Malda et al., 2013; Xu et al., 2019). Temperature and radiation also must be adequate to not harm the cells during the process. Particularly print with temperatures compatible with cell culture (close to 37°C) and a short exposure time to radiation. (Nahmias and Odde, 2006; Schiele et al., 2010). Of the several techniques that can be used in bioprinting, extrusion-based bioprinting is the most used technique (Ma et al., 2018). It is the one that applies less pressure, making it safer for the cells and avoiding the dispersion of them into the edge of the construct which can lead to a non-homogeneous distribution of cells (Kabirian and Mozafari, 2019). Gu et al. successfully bioprinted human iPSCs with a polysaccharide-based bioink using an extrusion method. This allowed the proliferation and differentiation of the iPSCs into neuronal subtypes and supporting microglia (Figure 2A). This bioprinted scaffold proved to be a useful model for performing drug screening in addition to the disease models (Gu et al., 2017). For neurodegenerative disease modeling, Zhang et al. used extrusion bioprinting to develop a 3D core-shell model to simulate AD neural tissue. They utilized NSC with FAD mutation embedded in Matrigel as the core bioink and alginate as the shell bioink. These structures demonstrated tendencies to self-cluster, maintaining high viability over a long-term period while displaying enhanced differentiation in comparison to 2D models. The engineered construct significantly affected the NSC growth, leading to heightened aggregation of Aβ as well as its expression together with tau isoform genes, when compared to 2D models (Zhang et al., 2022).
FIGURE 2. Examples of bioprinting for neural models. (A) Bioprinting of iPSC cells in agglomerated to form embryonic bodies and later differentiated in several neural cell types (Gu et al., 2017). (B) Microfluidic extrusion used to quickly print iPSC-derived neuronal progenitors (de la Vega et al., 2018) (C) Different cell types and hydrogels used to replicate a spinal cord injury (Joung et al., 2018); (D) Laser bioprinting of iPSC-derived NSC and neurons (Koch et al., 2023b). Images were reprinted with permission from references.
The microfluidic extrusion is a similar technique where the bioink and the crosslinking agent meet in a microfluidic chamber before extrusion, polymerizing before the deposition which allows an easy flow when passing through the nozzle, printing more defined structures (Thomas and Willerth, 2017). De la Vega et al. used extrusion to print hiPSC-derived neural progenitor cells in under 5 min while maintaining the viability and differentiation capacity of the cells to generate spinal cord motor neurons (Figure 2B). This bioprinter can process the materials before printing, allowing the programming of the cell-laden bioinks patterns which is an advantageous tool to control the deposition of the cells in an architecture close to the brain tissue. Cells were cultured for a month and exposed to small molecules (SB431542 (SB), CHIR99021 (CHIR), LDN-193189 (LDN), retinoic acid (RA), and purmorphamine (Puro)) to evaluate their effect during the differentiation process (de la Vega et al., 2018).
One of the advantages of using bioprinting for the development of neural models is that it allows printing multiple cell types as you can load different bioinks in independent print heads. (Knowlton et al., 2018). Culturing multiple cell types is of great interest in brain models. Printing neurons together with glia cells (astrocytes and pericytes) would produce a more complex model where the interaction between the different cells can be studied, including the influence of glia cells in the development and survival of neurons. Joung et al. were able to create a 3D bioprinted platform that incorporates iPSC-derived spinal neuronal progenitor cells and oligodendrocytes progenitor cells capable of a later differentiation to spinal neurons and oligodendrocytes (Figure 2C). iPSC-derived spinal neural progenitor cells and oligodendrocytes progenitor cells clusters were bioprinted through extrusion-based multimaterial 3D bioprinting, allowing precise control over the position of these cell agglomerates and the use of different bioinks in different print heads. These oligodendrocytes were capable of myelinating the axons and providing a model for damaged central nervous system tissue (Joung et al., 2018). Inkjet bio printing is another technique that can be used to print neural cultures, including multiple cell types. It uses forces such as thermal, acoustic, piezoelectric, or electro to eject the drops of the bioink into the substrate (Gudapati et al., 2016). Its main advantages are the lower cost when compared with other techniques, high printing speeds, and high spatial resolution. However, it is limited to printing low-viscosity bioinks and low cell density as the clogging of the nozzle is common, being suitable for low thermal bioinks as well (Heidarian et al., 2019). Sullivan et al. used a commercial bioprinter to deposit nanoliter droplets of a PEG-based matrix, supplemented with RGD and Tyr-Ile-Gly-Ser-Arg peptide motifs and collagen IV to create a 3D model using iPSC-derived cells for neural culture. IPSC-derived astrocytes, neural progenitors. Brain endothelial-like cells and neurons were found viable when cultured in this matrix and the system allowed the endothelial-like vasculogenesis and improved neural differentiation and spontaneous activity (Sullivan et al., 2023).
Other works used laser-based bioprinting for neural cell culture. This technique is based on a laser beam focused on an absorbing layer that propels the bioink toward the collector. It maintains a high resolution with a medium/high speed of printing; however, it is expensive when compared to other bioprinting techniques. In addition, both, the laser and the dropping force, can cause cell death (Sarker et al., 2019). Koch et al. used laser-based bioprinting to compare parameters such as viability, differentiation potential, and functionality of both iPSC-derived neural stem cells and neural differentiated NSCs (Figure 2D). Results showed a higher viability after printing of NSC in comparison with printed differentiated neurons. The frequency and intensity of the activity were also superior in NSC culture. The co-culture with astrocytes was also evaluated and proven to further support the network formation and collective activity in NSC culture (Koch et al., 2023a).
Another great advantage of printing different cell types is the possibility of printing endothelial cells in the same construct as neural cells (Sullivan et al., 2023). The vasculature is essential for tissue survival and the including of it in an in vitro model contributes to the addition of more physiological relevant features while improving the culture of neural cells. Another important advantage is the improve of the culture time of these 3D constructs. Since large bioprinted structures can have their diffusion of media limited, the creation of vasculature within the model would allow an extension of the time of culture. This extension of culture protocols is advantageous not only for the completion of the long-lasting differentiation protocols of iPSC but also to better mimic late-set diseases such as neurodegenerative diseases (Cadena et al., 2021). Skylar-Scott et al. bioprinted iPSC cells to create a layered structure with NSC, endothelium, and neurons, resulting in vascularized and patterned cortical organoids within days (Skylar-Scott et al., 2022).
The brain models discussed above are stationary and have certain constraints. To introduce dynamic features to neural models, among other characteristics, microfluidic platforms are an interesting alternative. By using these small sized platforms, it is possible to create models with a tunable framework and fluid flow withing micro-compartmentalized devices (Bhatia and Ingber, 2014). Culturing different types of cells together in other types of in vitro models can be a challenge because one type of media cannot be suitable for all cells. However, microfluidic devices can assist in this matter as their structure allows the introduction of different fluids, minimizing the mixing effect, and allowing each cell type to receive its corresponding medium (Achyuta et al., 2013). Thus, enabling the culture of different cell types as they are seeded in different compartments that are interconnected and allowing the study of their interaction without risking cell viability. Another advantage of this type of cell culture is the compatibility with inspection techniques such as optical or high-resolution video microscopy allowing easier monitoring of the culture over time due to the transparency of the materials chosen for the fabrication of this microfluidic device (Wang Z. et al., 2018; Moutaux et al., 2018).
Cells can be easily cultured inside the device and form a simple monolayer on the base of a microchannel (Taylor et al., 2005). These 2D microfluidic devices still present an increased complexity of the system, compared to a regular 2D culture dish, because or their architecture and ability to include co-culture, fluid flow, and cell response to shear stress. Other times cells are embedded in hydrogel to recreate a more 3D dimensional model, where the advantages of organ-on-a-chip and 3D models can be combined (Koo et al., 2018a).
The ability to modulate the architecture of the channels in a chip allows some control of the cell evolution, such as in the direction of growth or migration (Oddo et al., 2019). Within the various channel distributions that this type of device can take, the most popular used architectures are stack and parallel formation (Amirifar et al., 2022). The stack is when two channels are vertically aligned and separated by a porous membrane. It is a very common architecture for replicating the blood-brain barrier (BBB) and studying drug permeation, cell migration, and/or invasion, among others. (Kilic et al., 2016; Motallebnejad et al., 2019a; Staicu et al., 2021; Fanizza et al., 2022). The parallel structure is when the channels are aligned parallelly to each other and separated by small pillars. This architecture is often used with hydrogels and cells embedded in the central microchannel (Adriani et al., 2017; Osaki et al., 2018a; Campisi et al., 2018).
In neuronal culture, it is common the presence of narrow microchannels between two cell culture chambers. These microchannels are usually designed with 5–10 µm of width to allow only the axons to pass since the cell body usually has bigger dimensions (around 20 µm), enabling the study of the axonal growth, signal propagation, and cell-cell interaction (Taylor et al., 2005; Coquinco et al., 2014). These types of devices can be used for the study of only 1 cell type and the axon’s behavior when exposed to certain substances or they can be used to study the interaction of two or more cell types by seeding the cells in interconnected chambers (Roach et al., 2019). Virlogeux et al. used a three-compartment device connected by microchannels to recapitulate both the healthy and Huntington’s disease (HD) corticostriatal network. They use the chambers and channels to create a presynaptic, synaptic, and postsynaptic region. Results showed dysfunctions and hypersynchrony on HD devices (Virlogeux et al., 2018). Li et al. also used a three-compartment chip but this time to study the neurotoxicity of Aβ peptides, very characteristic of Alzheimer’s disease (Figure 3A). The authors integrated two microgrooves connected to the compartments to create a gradient of chemotactic factors. These devices allowed the demonstration of a localized mechanism for the neurotoxicity induced by Aβ peptides, useful for the development of Alzheimer’s disease treatments (Li et al., 2017). Roach et al. developed a microfluidic device to mimic the complex brain circuitry with 5 chambers where different sub-types of neurons were culture, and connected by microchannels (Figure 3B). The chip proved to recapitulate the formation of an active neural network with normal cell morphology (Roach et al., 2019). Sala-Jarque et al. developed a microfluidic device composed of two chambers separated by microchannels to mimic the neuromuscular junction on peripheral nerve injuries (Figure 3C). Motor neurons were cultured on one side while muscle cells were on the other. A perpendicular channel between chambers and passing through all microchannels was used to vacuum-induce axotomy through air bubbles. The regenerative abilities of the neurons were assessed after optogenetic stimulation both on neurons and muscle cells. Results showed an increased axon regeneration after increasing neuronal activity as well as a release of paracrine factors by the muscle cells after stimulation, also triggering the regrowth of axons on the lesion area (Sala-Jarque et al., 2020). Another model of the neuromuscular junction used a similar microfluidic platform to differentiate healthy and amyotrophic lateral sclerosis hNSC into proprioceptive sensory neurons, co-cultured with motor neurons. Results demonstrated that the cells were able to interact by the formation of annulospiral wrapping-like structures. The genetic profile comparison between healthy and ALS samples pointed to lower values of ETV1 (important for motor feedback) which highlights the involvement of proprioceptive sensory neurons in this pathology (Badiola-Mateos et al., 2022). Haberts et al. also developed a brain-on-a-chip where microchannels connected different compartments (Figure 3D). In this design, elevated cavities were produced through 3D nanoprinting and interconnected by microchannels to form a complex structure. Human iPSC-derived neurons were cultured in this model and the neural outgrowth, network size, and branching behavior were evaluated. Results showed a developed neural network with functional activity after only a few days in culture, validating the device design (Harberts et al., 2020).
FIGURE 3. Examples of microfluidic device designs for brain modeling. (A) A device with two cell chambers divided with microchannels with a synaptic chamber in between for Alzheimer’s disease (Li et al., 2017). (B) Multichambered device for culture of different types of neurons connected through microchannels (Roach et al., 2019). (C) Microfluidic-assisted axotomy device to study the regenerative ability of motor neurons after lesion (Sala-Jarque et al., 2020); (D) Brain-on-a-chip composed of microcompartments where hiPSC-derived neurons were cultured and formed networks (Harberts et al., 2020). (E) 3D BBB model with human endothelial cells embedded in Matrigel®(Chung et al., 2020); (F) Sandwich-type microfluidic device with an endothelial layer of cells cultured on the top channel and separated with a porous membrane from a bottom layer where astrocytes were seeded in a 3D matrix (Motallebnejad et al., 2019b). (G) BBB-model where endothelial cells were seeded in close contact with neurons, astrocytes, and microglia, creating a 3D tetra-culture brain model (Koo et al., 2018c). Images were reprinted with permission from references.
The separation between the axons and the cell body not only can be done using the microchannels mentioned above but also through pillars with embedded hydrogel (Adriani et al., 2017; Wang Y. et al., 2018; Kamande et al., 2019; Klim et al., 2019). Chung et al. used 3 channels where brain endothelial cells were cultured on the middle one for blood-brain barrier modeling (Figure 3E). The receptor-mediated transcytosis, as well as brain endothelial-specific penetrating abilities of different peptides, were evaluated and the models were proven to be used for drug development (Chung et al., 2020). Osaki et al. used a compartmentalized model to study amyotrophic lateral sclerosis by culturing iPSC-derived motor neurons from patients in the form of spheroids together with skeletal muscle bundles. The results showed the neuromuscular junctions between the axons and the muscle fibers. It also demonstrated fewer muscle contractions, motor neuron degradation, and increased apoptosis of the muscle on the ALS-motor unit, compared to non-ALS devices (Osaki et al., 2018b).
Static microfluidic platform culture models present interesting features for modeling healthy and diseased tissue since their architecture is still innovative. An example is the model developed by Park et al. that incorporates three different cell types in a 3D architecture for Alzheimer’s disease modeling in a no-flow experiment (Park et al., 2018). Neurons, astrocytes, and microglia cells derived from iPSC were chosen to recapitulate representative features of Alzheimer’s disease such as beta-amyloid aggregation, neuroinflammatory activity, and phosphorylated tau accumulation. However, the inclusion of perfusion in a cell culture model is, as mentioned above, a great advantage of these platforms. The presence of fluid flow improves the diffusion of media through the culture as well as exposes the cells to shear stress which influences cell morphology, behavior, and gene and protein expression (Huang et al., 2022).
Uzel et al. designed two microfluidic devices capable of generating orthogonal gradients within a gel region and studied the ability of two different molecules to induce the cellular response of mouse embryonic stem cells (Uzel et al., 2016). The presence of a gradient of molecule concentrations in a cell culture model is important because it influences cell behaviors such as differentiation and maturation (Kilic et al., 2016). In static conditions, the design of the device allows the insertion of gradients that can influence the proliferation and differentiation of cells (Park et al., 2009). However, by taking advantage of the perfusion that can be used in a microfluidic device, the exposure of cells to different gradient concentrations can be easily performed (Demers et al., 2016; Uzel et al., 2016).
Looking at the bibliography, we can find a superior number of publications on static devices than on dynamic ones. This is mainly due to the difficulty of introducing fluid flow in neuronal culture and the physiology of the brain tissue itself. Cells in the brain are extremely sensitive, especially primary cultures or induced stem cells. A harsh small movement in the seeding or maintenance of the culture can lead to cell detachment and subsequent loss of the cells in the culture. Inclusive, high-shear stress can be used to model traumatic brain injury, for example, by including a channel that crosses the microchannels where the axons are located where a high fluid flow is applied, damaging the cultured axons and thus simulating the injury (Cullen and LaPlaca, 2006)). A possible solution to minimize this effect is to combine the organoid culture with the microfluidic one. iPSC in organoids presents higher resistance to shear stress and fluid flow can be easily incorporated at the embryonic body stage of differentiation, which would also be advantageous to the perfusion problem that organoid culture faces (Park et al., 2015; Wang Y. et al., 2018).
Regarding the tissue, neuronal cells are fed by the brain vasculature. This vasculature is different from the other vasculature in the body due to the BBB present in every blood vessel in the brain. This tight unit is built not only by endothelial but also by neural cells such as astrocytes and pericytes (Chow and Gu, 2015). When planning the design of a microfluidic model, the most physiological way to mimic the fluid that nourishes the neurons is to mimic the BBB. So, there are several models of BBB-on-a-chip that besides culturing the different cell types present in the NVU, also introduce fluid flow in the channels with endothelial cell culture (Achyuta et al., 2013; Deosarkar et al., 2015; Adriani et al., 2017; Bang et al., 2017; Sances et al., 2018a; Campisi et al., 2018; Vatine et al., 2019a; Chung et al., 2020) Sances et al. developed a spinal cord-chip system with iPSC-derived spinal neural progenitor cells cultured with brain microvascular endothelial cells (Sances et al., 2018b). This co-culture model had increased neuronal activity in vascular-neural interaction genes, showing a developmental gene expression closer to what happens in vivo. Vatine et al. used a BBB-on-a-chip with co-culture of neural and endothelial cells, and perfusion which enhanced the performance of the barrier, exhibiting physiologically relevant TEER values (Vatine et al., 2019b). The model was able to replicate the inflammatory response and transport of biomarkers. When using cells from patients, the model could detect functional differences when compared to healthy donors. Motallebnejad et al. developed a BBB model where hiPSC-derived brain microvascular endothelial cells were cultured in a top channel, connected through a porous membrane to a lower channel where astrocytes embedded in a hydrogel were seeded (Figure 3F). The presence of tight junction proteins and permeability studies confirmed the integrity of the BBB. Moreover, by adding TGF-β1 the integrity of the BBB was disrupted, contributing to the versatility of this model (Motallebnejad et al., 2019a). Koo et al. created a 3D BBB-on-a-chip using the commercially available device from Mimetas (OrganoPlate) with four different cell types (Figure 3G). Endothelial cells were seeded in direct contact with neuroblastoma, microglia, and astrocytes embedded in a hydrogel. This model was used to assess the neurotoxicity of organophosphate-based compounds (OPs) and results demonstrated the permeation of OPs through the BBB and inhibited the acetylcholinesterase activity. These results correlated with in vivo data, validating the potential of this device (Koo et al., 2018b).
Despite the many advantages of microfluidic devices, they still present some limitations. The handling of these devices is not easy as they can face throughput and reproducibility issues. In addition, it is difficult to have control over the properties and microstructure of the devices in every manual replica. Clogging of the channels with cells, debris, or bubbles influences their functioning and the shear stress must be applied carefully to not harm the cells in culture (Bhatia and Ingber, 2014). Even though, parallel formations with transparent materials such as PDMS facilitate the visualization of the cells in culture and the incorporation of sensors in the design of the device facilitates the analysis during culture time, (Kuswandi et al., 2007).
Recent models indicate that the future of 3D culture will be related to the integration of microfluidic devices, hydrogels, and/or spheroids/organoids to evolve these devices into better mimicking models (Liu et al., 2019). As mentioned before, organoids are widely used as 3D brain models to study neurodevelopment or brain diseases. One of the biggest disadvantages of this culture is the necrotic core that happens when the size of the organoid increases and the diffusion of nutrients and oxygen becomes difficult. The combination of microfluidic devices in organoid culture is a possible solution to allow the continuous exchange of cell culture medium without the need for big reactors. Furthermore, the ability to control the organoid culture with microfluidic devices allows a less heterogeneous production of these agglomerates, another common downside. Wang et al. used a microfluidic platform for brain organoid culture to evaluate the effects of nicotine exposure on prenatal brain development. The device was composed of five channels, two with the iPSC-derived organoids embedded in Matrigel® and the other three for fluid flow (Wang Y. et al., 2018). The dimensionality of the organoids and fluid flow increased the cell viability and marker expression in comparison with the 2D culture.
Taking advantage of the possibility of compartmentalization and fluid flow, these platforms incorporate one or several channels surrounded by fluid channels to allow the exchange of nutrients and oxygen. Adriani et al. built a 3D neurovascular microfluidic model with 4 channels separated by pillars to mimic the neurovascular unit (Adriani et al., 2017). The channels included medium, primary rat astrocytes embedded in the hydrogel, primary rat neurons embedded in the hydrogel, and human endothelial cells plus medium. The cells presented type-specific morphology and functional properties similar to other existing models. Lee et al. developed a microfluidic device of 3 channels separated with pillars where hiPSC-derived endothelial cells, primary brain pericytes, and astrocytes were cultured with a fibrin hydrogel to quantify nanoparticle permeability. Surface-functionalized particles showed a higher permeability than non-functionalized ones. This model enabled rapid analysis of the permeability compared to transwells models and with a more physiological relevance (Lee et al., 2020).
Novak et al. developed a robotic platform that links 8 different types of organs-on-chips, using what they called the Interrogator (Novak et al., 2019), to create a complex model. This completely automatic platform allows continuous liquid transfer from one organ-on-a-chip to the other with in situ imaging. Relating this type of model with a disease can be of extreme value in evaluating not only the side effects of a drug for its treatment but also the influence of a diseased organ on the other healthy organs.
Characterization of 2D models by immunochemical staining, electrophysical measurements or biochemical analysis is well described but characterizing 3D microenvironments is still challenging. There is a limitation for the detection and monitorization of 3D features such as the synaptic activity of brain models. The possibility of using microfluidic devices with electrophysiological sensors is another promising integration when performing neuronal culture (Habibey et al., 2017). Honrado et al. combined neuronal networks with a microfluidic device for impedance cytometry capable of predicting cell size, cross-sectional position, and velocity (Honrado et al., 2020). This is an example of how microfluidic devices can be used in high-throughput measurements useful for drug testing, diagnosis, and personalized medicine. Grist et al. created a microfluidic platform that combined spatiotemporal oxygen control and long-term microscopy to assess tumor spheroid response to hypoxia (Grist et al., 2023). Results showed the accumulation of doxorubicin in tumor spheroids is cycling hypoxia dependent as well as the size of the tumor during treatment due to the oxygen control the microfluidic platform allowed as well as the long-term two-photon microscopy monitoring. The conjugation of electrodes is very useful to monitor if the network is functional and acting according to healthy or diseased circumstances. Palma-Florez et al. used a microfluidic platform with a trans-endothelial electrical resistance measurement system to evaluate the permeability of a therapeutic nanosystem for AD treatment. Human astrocytes and pericytes were cultured in the central channel while endothelial cells were placed in the lateral channel. The nanoparticles were able to permeate through the barrier, particularly the ones containing angiopep-2 peptide, a molecule that has been shown to help in the disaggregation of the amyloid. This platform was functional for the study of nanotherapeutics’ effects on brain permeability (Palma-Florez et al., 2023).
A feature of 3D printing available to incorporate into microfluidic devices is bioprinting. A way to do this is using different print heads, one for the material of the device and the other(s) for bioink(s) with different types of cells. The structural material of the device can be cured in advance, so cells are not harmed during the curing process. A more accurate and controlled positioning of the cells within the model would be beneficial, especially when taking into consideration the different placement of neural cells within the brain. Considering the brain has zones with different percentages of various cell types and that most models do not use multi-cell type culture and the spatial distribution is aleatory, bioprinting arises as a promising tool to help build an accurate brain model, with co-culture but also with the disposition of cells corresponding to the different brain regions. Johnson et al. used an approach based on addictive manufacture to produce a 3D printed nervous system on a chip. Through micro-extrusion, microchannels and chambers were printed, mimicking the communication between the glial cells and axons in the nervous system to test nervous system infections. This platform was a successful multiscale and biomimetic model (Johnson et al., 2016). Yi et al. created a microfluidic device to study glioblastoma using bioprinting which resulted in a model composed of patient-derived glioblastoma, vascular endothelial cells and decellularized ECM from porcine brain capable of recreating features of this tumor to be used as drug testing platform (Yi et al., 2019). Kajtez et al. developed a 3D printing soft-lithography technique to print a device for neuronal culture. The printed platform stands for the long-term culture of human stem cell-derived neurons and astrocytes. The authors also used this platform to modulate the nigrostriatal pathway in Parkinson’s disease and were proven successful in maximizing the unidirectional growth of the dopaminergic projections (Kajtez et al., 2020).
We can find some examples of organoids’ growth inside microfluidic devices to improve their perfusion and vascularization procedures. The incorporation of better characterization techniques or sensors is also being studied and integrated into recent models. Combining bioprinting with the microfabrication process of organs-on-chips can be a way to improve the mechanical properties as well as the porosity, microstructure, and even the polymerization mechanisms of the material chosen, for example, a hydrogel. This combination allows control over the composition of the device to better mimic the tissue. It is also possible to introduce growth factors, cytokines, or other molecules in the bioink and place them directly where it is desired. Printing cells into these devices would decrease the time and complexity of cell seeding in a chip. The possibility of creating a vascular network is another attractive option (Yu and Choudhury, 2019).
Neurodegenerative diseases affect a high percentage of elderly people around the world and due to the increasing in the age of the population, the number is estimated to advance rapidly. When animal models and traditional 2D culture are not enough to answer how to treat these conditions, more elaborate models are needed, recognizing the importance of tissue architecture and the use of more specific cells. It is critical to correctly mimic the communication that undergoes between all the different cell types present in the brain, not only in the case of a healthy situation but also in the case of a disease. To do so, induced pluripotent stem cells arise as an accurate choice since they are obtained from the patient and can create all the cell types from the three germ layers. This type of cell is then considered the future of in vitro modeling and their combination with 3D models is on the edge of the research.
However, neurodegenerative cell culture models still face some challenges. The time of culture is one of them when considering iPSC. The model must guarantee cell survival/viability and function for as long as the experiment is intended. Most times the model looks very promising but then the cells are only alive for a few hours or days which does not allow the mimicking of long-term diseases or their effects (Kelava and Lancaster, 2016b). Other challenges in 3D are the compatibility with live imaging techniques or the difficulty in the perfusion of nutrients and oxygen. Recently has been proposed 4D bioprinting as a cutting-edge additive manufacturing technology that has an intrinsic capability to fabricate de novo living tissue constructs which can be made to change in various mechanical or physio-spatial aspects when subjected to predetermined stimuli or trigger sources. 4D bioprinting techniques can be used to place both living cells and growth factors in highly ordered, biomimetic motifs which can undergo physiologically relevant transformations to accurately simulate developmental processes, such as tissue stretching, compression, or shifting of the biomaterial’s modulus. The fourth dimension in “4D bioprinting” refers to the time element on which one or more of a 3D printed object’s physical attributes are functionally dependent (Esworthy et al., 2019). New techniques developed recently show the feasibility of manufacturing cerebral organoids and assembloids by mimicking human organogenesis. However, the bottom-up technology to produce organoids still requires further work to advance functional maturation and assemble neural circuits. Multilineage assembloids could provide a more complex system including blood vessels although the process is very difficult to standardize. In this sense, some future improvements would include: i) the development of techniques to bioprint hNPCs that will go for terminal differentiation in the 3D structure. ii) Demonstration of the selective effect of bio-inks with natural ECM on neuronal differentiation. iii) Development of the techniques to bioprint blood vessels with artificial- or natural-derived bio-inks and iv) Improve 4D bioprinting techniques to bioprint all components of the brain organoids.
The perfect in vitro model for neurodegenerative disease needs still to be found but combined efforts of all these years have shown that we are going in the right direction and that there is still hope to find a more accurate model to better understand these diseases, how and when they appear, how they evolve and maybe, help in finding a better drug to treat them. Learning from every downside of the existing models is crucial to tune the right direction. The perfect scenario is one where we can combine all their advantages to include the self-organization of the cells occurring in organoids with the precise control over cell seeding and scaffolds that bioprinting allows or the compartmentalization combined with the possibility of recreating mechanical forces that microfluidic devices possess.
IP: Conceptualization, Writing–original draft, Writing–review and editing. ML: Conceptualization, Supervision, Writing–original draft, Writing–review and editing. JS: Conceptualization, Supervision, Writing–original draft, Writing–review and editing.
The author(s) declare financial support was received for the research, authorship, and/or publication of this article. This work was supported by the Networking Biomedical Research Center (CIBER), in Spain. CIBER is an initiative funded by the VI National R&D&i Plan 2008–2011, Iniciativa Ingenio 2010, Consolider Program, CIBER Actions, and the Instituto de Salud Carlos III (RD16/0006/0012), with the support of the European Regional Development Fund (ERDF). This work was funded by the C CERCA Program, and by the Commission for Universities and Research of the Department of Innovation, Universities, and Enterprise of the Generalitat de Catalunya (2017 SGR 1079), and the Spanish Ministry of Economy and Competitiveness (MINECO) through the project NEUR-ON-A-CHIP (RTI2018-097038-B-C21 and RTI2018-097038-B-C22). This study was developed in the context of AdvanceCat and Base3D with the support of ACCIÓ (Catalonia Trade and Investment; Generalitat de Catalunya) under the Catalonian ERDF operational program (European Regional Development Fund) 2014–2020. IP is a fellow of the European Union’s Horizon 2020 Research and Innovation Program under the Marie Skłodowska-Curie Innovative Training Networks (ASCTN Training Network, grant agreement No. 813851).
We would like to acknowledge the funding by the research and innovation project Training on Advanced Stem Cell Technologies in Neurology Marie Sklodowska-Curie European Training Network (MSCA-ITN-2018–813851).
The authors declare that the research was conducted in the absence of any commercial or financial relationships that could be construed as a potential conflict of interest.
The author(s) declared that they were an editorial board member of Frontiers, at the time of submission. This had no impact on the peer review process and the final decision.
All claims expressed in this article are solely those of the authors and do not necessarily represent those of their affiliated organizations, or those of the publisher, the editors and the reviewers. Any product that may be evaluated in this article, or claim that may be made by its manufacturer, is not guaranteed or endorsed by the publisher.
Abud, E. M., Ramirez, R. N., Martinez, E. S., Healy, L. M., Nguyen, C. H. H., Newman, S. A., et al. (2017). iPSC-derived human microglia-like cells to study neurological diseases. Neuron 94, 278–293.e9. doi:10.1016/J.NEURON.2017.03.042
Achyuta, A. K. H., Conway, A. J., Crouse, R. B., Bannister, E. C., Lee, R. N., Katnik, C. P., et al. (2013). A modular approach to create a neurovascular unit-on-a-chip. Lab. Chip 13, 542–553. doi:10.1039/c2lc41033h
Adriani, G., Ma, D., Pavesi, A., Kamm, R. D., and Goh, E. L. K. (2017). A 3D neurovascular microfluidic model consisting of neurons, astrocytes and cerebral endothelial cells as a blood-brain barrier. Lab. Chip 17, 448–459. doi:10.1039/c6lc00638h
Ahadian, S., Bannerman, D., Korolj, A., Lai, B., Wang, E., Davenport-Huyer, L., et al. (2017). Organ-on-A-chip platforms: a convergence of advanced materials, cells, and microscale technologies. Adv. Healthc. Mater 7, 1700506. doi:10.1002/adhm.201800734
Amirifar, L., Shamloo, A., Nasiri, R., de Barros, N. R., Wang, Z. Z., Unluturk, B. D., et al. (2022). Brain-on-a-chip: recent advances in design and techniques for microfluidic models of the brain in health and disease. Biomaterials 285, 121531. doi:10.1016/J.BIOMATERIALS.2022.121531
Arlotta, P. (2018). Organoids required! A new path to understanding human brain development and disease. Nat. Methods 15, 27–29. doi:10.1038/nmeth.4557
Aurand, E. R., Wagner, J. L., Shandas, R., and Bjugstad, K. B. (2014). Hydrogel formulation determines cell fate of fetal and adult neural progenitor cells. Stem Cell. Res. 12, 11–23. doi:10.1016/j.scr.2013.09.013
Badhe, R. V., Chejara, D. R., Kumar, P., Choonara, Y. E., and Pillay, V. (2018). “Neurodegenerative disease conditions and genomic treatment for better health,” in Genomics-driven healthcare (Springer), 281–310.
Badiola-Mateos, M., Osaki, T., Kamm, R. D., and Samitier, J. (2022). In vitro modelling of human proprioceptive sensory neurons in the neuromuscular system. Sci. Rep. 12, 1–19. doi:10.1038/s41598-022-23565-3
Bagley, J. A., Reumann, D., Bian, S., Lévi-Strauss, J., and Knoblich, J. A. (2017). Fused cerebral organoids model interactions between brain regions. Nat. Methods 14, 743–751. doi:10.1038/nmeth.4304
Baharvand, H., Hashemi, S. M., Ashtiani, S. K., and Farrokhi, A. (2004). Differentiation of human embryonic stem cells into hepatocytes in 2D and 3D culture systems in vitro. Int. J. Dev. Biol. 50, 645–652. doi:10.1387/ijdb.052072hb
Baiguera, S., Del Gaudio, C., Lucatelli, E., Kuevda, E., Boieri, M., Mazzanti, B., et al. (2014). Electrospun gelatin scaffolds incorporating rat decellularized brain extracellular matrix for neural tissue engineering. Biomaterials 35, 1205–1214. doi:10.1016/j.biomaterials.2013.10.060
Bang, S., Lee, S.-R., Ko, J., Son, K., Tahk, D., Ahn, J., et al. (2017). A low permeability microfluidic blood-brain barrier platform with direct contact between perfusable vascular network and astrocytes. Sci. Rep. 7, 8083. doi:10.1038/s41598-017-07416-0
Barres, B. A. (2008). The mystery and magic of glia: a perspective on their roles in health and disease. Neuron 60, 430–440. doi:10.1016/J.NEURON.2008.10.013
Barroca, N., da Silva, D. M., Pinto, S. C., Sousa, J. P. M., Verstappen, K., Klymov, A., et al. (2023). Interfacing reduced graphene oxide with an adipose-derived extracellular matrix as a regulating milieu for neural tissue engineering. Biomater. Adv. 148, 213351. doi:10.1016/J.BIOADV.2023.213351
Bhatia, S. N., and Ingber, D. E. (2014). Microfluidic organs-on-chips. Nat. Biotechnol. 32, 760–772. doi:10.1038/nbt.2989
Bordoni, M., Rey, F., Fantini, V., Pansarasa, O., Di Giulio, A., Carelli, S., et al. (2018). From neuronal differentiation of iPSCs to 3D neuro-organoids: modelling and therapy of neurodegenerative diseases. Int. J. Mol. Sci. 19, 3972. doi:10.3390/ijms19123972
Brawner, A. T., Xu, R., Liu, D., and Jiang, P. (2017). Generating CNS organoids from human induced pluripotent stem cells for modeling neurological disorders. Int. J. Physiol. Pathophysiol. Pharmacol. 9, 101–111.
Breslin, S., and O’Driscoll, L. (2013). Three-dimensional cell culture: the missing link in drug discovery. Drug Discov. Today 18, 240–249. doi:10.1016/j.drudis.2012.10.003
Budday, S., Nay, R., de Rooij, R., Steinmann, P., Wyrobek, T., Ovaert, T. C., et al. (2015). Mechanical properties of gray and white matter brain tissue by indentation. J. Mech. Behav. Biomed. Mater 46, 318–330. doi:10.1016/j.jmbbm.2015.02.024
Cadena, M., Ning, L., King, A., Hwang, B., Jin, L., Serpooshan, V., et al. (2021). 3D bioprinting of neural tissues. Adv. Healthc. Mater 10, 2001600. doi:10.1002/ADHM.202001600
Campisi, M., Shin, Y., Osaki, T., Hajal, C., Chiono, V., and Kamm, R. D. (2018). 3D self-organized microvascular model of the human blood-brain barrier with endothelial cells, pericytes and astrocytes. Biomaterials 180, 117–129. doi:10.1016/J.BIOMATERIALS.2018.07.014
Cantley, W. L., Du, C., Lomoio, S., Depalma, T., Peirent, E., Kleinknecht, D., et al. (2018). Functional and sustainable 3D human neural network models from pluripotent stem cells. ACS Biomater. Sci. Eng. 4, 4278–4288. doi:10.1021/acsbiomaterials.8b00622
Cerrone, F., Pozner, T., Siddiqui, A., Ceppi, P., Winner, B., Rajendiran, M., et al. (2020). Polyhydroxyphenylvalerate/polycaprolactone nanofibers improve the life-span and mechanoresponse of human IPSC-derived cortical neuronal cells. Mater. Sci. Eng. C 111, 110832. doi:10.1016/j.msec.2020.110832
Chang, R., Nam, J., and Sun, W. (2008). Effects of dispensing pressure and nozzle diameter on cell survival from solid freeform fabrication–based direct cell writing. Tissue Eng. Part A 14, 41–48. doi:10.1089/ten.2007.0004
Cho, H. J., Verbridge, S. S., Davalos, R. V., and Lee, Y. W. (2018). Development of an in vitro 3D brain tissue model mimicking in vivo-Like pro-inflammatory and pro-oxidative responses. Ann. Biomed. Eng. 46, 877–887. doi:10.1007/s10439-018-2004-z
Choi, S. H., Kim, Y. H., Hebisch, M., Sliwinski, C., Lee, S., D’Avanzo, C., et al. (2014). A three-dimensional human neural cell culture model of Alzheimer’s disease. Nature 515, 274–278. doi:10.1038/nature13800
Choi, Y. J., Chae, S., Kim, J. H., Barald, K. F., Park, J. Y., and Lee, S.-H. (2013). Neurotoxic amyloid beta oligomeric assemblies recreated in microfluidic platform with interstitial level of slow flow. Sci. Rep. 3, 1921. doi:10.1038/srep01921
Chow, B. W., and Gu, C. (2015). The molecular constituents of the blood–brain barrier. Trends Neurosci. 38, 598–608. doi:10.1016/J.TINS.2015.08.003
Chung, B., Kim, J., Nam, J., Kim, H., Jeong, Y., Liu, H., et al. (2020). Evaluation of cell-penetrating peptides using microfluidic in vitro 3D brain endothelial barrier. Macromol Biosci.1900425
Cimler, R., Maresova, P., Kuhnova, J., and Kuca, K. (2019). Predictions of Alzheimer’s disease treatment and care costs in European countries. PLoS One 14, e0210958. doi:10.1371/journal.pone.0210958
Coquinco, A., Kojic, L., Wen, W., Wang, Y. T., Jeon, N. L., Milnerwood, A. J., et al. (2014). A microfluidic based in vitro model of synaptic competition. Mol. Cell. Neurosci. 60, 43–52. doi:10.1016/j.mcn.2014.03.001
Crowe, J. A., El-Tamer, A., Nagel, D., Koroleva, A. V., Madrid-Wolff, J., Olarte, O. E., et al. (2020). Development of two-photon polymerised scaffolds for optical interrogation and neurite guidance of human iPSC-derived cortical neuronal networks. Lab. Chip 20, 1792–1806. doi:10.1039/c9lc01209e
Cullen, D. K., and LaPlaca, M. C. (2006). Neuronal response to high rate shear deformation depends on heterogeneity of the local strain field. J. Neurotrauma 23, 1304–1319. doi:10.1089/neu.2006.23.1304
Cunha, C., Panseri, S., Villa, O., Silva, D., and Gelain, F. (2011). 3D culture of adult mouse neural stem cells within functionalized self-assembling peptide scaffolds. Int. J. Nanomedicine 6, 943–955. doi:10.2147/IJN.S17292
de la Vega, L., Lee, C., Sharma, R., Amereh, M., and Willerth, S. M. (2019). 3D bioprinting models of neural tissues: the current state of the field and future directions. Brain Res. Bull. 150, 240–249. doi:10.1016/J.BRAINRESBULL.2019.06.007
de la Vega, L., Rosas Gómez, A. D., Abelseth, E., Abelseth, L., Allisson da Silva, V., Willerth, S., et al. (2018). 3D bioprinting human induced pluripotent stem cell-derived neural tissues using a novel lab-on-a-printer technology. Appl. Sci. 8, 2414. doi:10.3390/app8122414
Demers, C. J., Soundararajan, P., Chennampally, P., Cox, G. A., Briscoe, J., Collins, S. D., et al. (2016). Development-on-chip: in vitro neural tube patterning with a microfluidic device. Development 143, 1884–1892. doi:10.1242/dev.126847
Deosarkar, S. P., Prabhakarpandian, B., Wang, B., Sheffield, J. B., Krynska, B., and Kiani, M. F. (2015). A novel dynamic neonatal blood-brain barrier on a chip. PLoS One 10, e142821. doi:10.1371/journal.pone.0142725
DeQuach, J. A., Yuan, S. H., Goldstein, L. S. B., and Christman, K. L. (2011). Decellularized porcine brain matrix for cell culture and tissue engineering scaffolds. Tissue Eng. Part A 17, 2583–2592. doi:10.1089/ten.tea.2010.0724
Ding, S., Feng, L., Wu, J., Zhu, F., Tan, Z., and Yao, R. (2018). Bioprinting of stem cells: interplay of bioprinting process, bioinks, and stem cell properties. ACS Biomater. Sci. Eng. 4, 3108–3124. doi:10.1021/acsbiomaterials.8b00399
Dingle, Y.-T. L., Boutin, M. E., Chirila, A. M., Livi, L. L., Labriola, N. R., Jakubek, L. M., et al. (2015). Three-dimensional neural spheroid culture: an in vitro model for cortical studies. Tissue Eng. Part C Methods 21, 1274–1283. doi:10.1089/ten.tec.2015.0135
Duval, K., Grover, H., Han, L.-H., Mou, Y., Pegoraro, A. F., Fredberg, J., et al. (2017). Modeling physiological events in 2D vs. 3D cell culture. Physiology 32, 266–277. doi:10.1152/physiol.00036.2016
Edgar, D., Timpl, R., and Thoenen, H. (1984). The heparin-binding domain of laminin is responsible for its effects on neurite outgrowth and neuronal survival. EMBO J. 3, 1463–1468. doi:10.1002/j.1460-2075.1984.tb01997.x
Eglen, R. M., and Reisine, T. (2019). Human iPS cell-derived patient tissues and 3D cell culture Part 2: spheroids, organoids, and disease modeling. SLAS Technol. Transl. Life Sci. Innov. 24, 18–27. doi:10.1177/2472630318803275
Engler, A. J., Sen, S., Sweeney, H. L., and Discher, D. E. (2006). Matrix elasticity directs stem cell lineage specification. Cell. 126, 677–689. doi:10.1016/J.CELL.2006.06.044
Esworthy, T. J., Miao, S., Lee, S. J., Zhou, X., Cui, H., Zuo, Y. Y., et al. (2019). Advanced 4D-bioprinting technologies for brain tissue modeling and study. Int. J. Smart Nano Mater 10, 177–204. doi:10.1080/19475411.2019.1631899
Fanizza, F., Campanile, M., Forloni, G., Giordano, C., and Albani, D. (2022). Induced pluripotent stem cell-based organ-on-a-chip as personalized drug screening tools: a focus on neurodegenerative disorders. J. Tissue Eng. 13, 204173142210953. doi:10.1177/20417314221095339
Führmann, T., Hillen, L. M., Montzka, K., Wöltje, M., and Brook, G. A. (2010). Cell–Cell interactions of human neural progenitor-derived astrocytes within a microstructured 3D-scaffold. Biomaterials 31, 7705–7715. doi:10.1016/j.biomaterials.2010.06.060
Garcia-Leon, J. A., Vitorica, J., and Gutierrez, A. (2019). Use of human pluripotent stem cell-derived cells for neurodegenerative disease modeling and drug screening platform. Future Med. Chem. 11, 1305–1322. doi:10.4155/fmc-2018-0520
Ghaffari, L. T., Starr, A., Nelson, A. T., and Sattler, R. (2018). Representing diversity in the dish: using patient-derived in vitro models to recreate the heterogeneity of neurological disease. Front. Neurosci. 12, 56–18. doi:10.3389/fnins.2018.00056
Gopalakrishnan, J. (2019). The emergence of stem cell-based brain organoids: trends and challenges. BioEssays 41, 1900011. doi:10.1002/bies.201900011
Grist, S. M., Soroush Nasseri, S., Laplatine, L., Schmok, J. C., Yao, D., Hua, J., et al. (2023). Long-term monitoring in a microfluidic system to study tumour spheroid response to chronic and cycling hypoxia. Sci Rep. 9(1), 17782. doi:10.1038/s41598-019-54001-8
Gu, Q., Tomaskovic-Crook, E., Wallace, G. G., and Crook, J. M. (2017). 3D bioprinting human induced pluripotent stem cell constructs for in situ cell proliferation and successive multilineage differentiation. Adv. Healthc. Mater 6, 1700175. doi:10.1002/adhm.201700175
Gudapati, H., Dey, M., and Ozbolat, I. (2016). A comprehensive review on droplet-based bioprinting: past, present and future. Biomaterials 102, 20–42. doi:10.1016/J.BIOMATERIALS.2016.06.012
Gumera, C., Rauck, B., and Wang, Y. (2011). Materials for central nervous system regeneration: bioactive cues. J. Mater Chem. 21, 7033–7051. doi:10.1039/c0jm04335d
Habibey, R., Latifi, S., Mousavi, H., Pesce, M., Arab-Tehrany, E., and Blau, A. (2017). A multielectrode array microchannel platform reveals both transient and slow changes in axonal conduction velocity. Sci. Rep. 7, 8558. doi:10.1038/s41598-017-09033-3
Haenseler, W., Sansom, S. N., Buchrieser, J., Newey, S. E., Moore, C. S., Nicholls, F. J., et al. (2017). A highly efficient human pluripotent stem cell microglia model displays a neuronal-co-culture-specific expression profile and inflammatory response. Stem Cell. Rep. 8, 1727–1742. doi:10.1016/j.stemcr.2017.05.017
Harberts, J., Fendler, C., Teuber, J., Siegmund, M., Silva, A., Rieck, N., et al. (2020). Toward brain-on-a-chip: human induced pluripotent stem cell-derived guided neuronal networks in tailor-made 3D nanoprinted microscaffolds. ACS Nano 14, 13091–13102. doi:10.1021/acsnano.0c04640
Hartlaub, A. M., McElroy, C. A., Maitre, N. L., and Hester, M. E. (2019). Modeling human brain circuitry using pluripotent stem cell platforms. Front. Pediatr. 7, 57–58. doi:10.3389/fped.2019.00057
Heidarian, P., Kouzani, A., Kaynak, A., Paulino, M., and Nasri-Nasrabadi, B. (2019). Dynamic hydrogels and polymers as inks for three-dimensional printing. ACS Biomater. Sci. Eng. Acsbiomaterials. 5, 2688–2707. doi:10.1021/acsbiomaterials.9b00047
Her, G. J., Wu, H. C., Chen, M. H., Chen, M. Y., Chang, S. C., and Wang, T. W. (2013). Control of three-dimensional substrate stiffness to manipulate mesenchymal stem cell fate toward neuronal or glial lineages. Acta Biomater. 9, 5170–5180. doi:10.1016/j.actbio.2012.10.012
Hong, S., Yang, K., Kang, B., Lee, C., Song, I. T., Byun, E., et al. (2013). Hyaluronic acid catechol: a biopolymer exhibiting a pH-dependent adhesive or cohesive property for human neural stem cell engineering. Adv. Funct. Mater 23, 1774–1780. doi:10.1002/adfm.201202365
Honrado, C., McGrath, J. S., Reale, R., Bisegna, P., Swami, N. S., and Caselli, F. (2020). A neural network approach for real-time particle/cell characterization in microfluidic impedance cytometry. Anal. Bioanal. Chem. 412, 3835–3845. doi:10.1007/s00216-020-02497-9
Hopkins, A. M., De Laporte, L., Tortelli, F., Spedden, E., Staii, C., Atherton, T. J., et al. (2013). Silk hydrogels as soft substrates for neural tissue engineering. Adv. Funct. Mater 23, 5140–5149. doi:10.1002/adfm.201300435
Hospodiuk, M., Dey, M., Sosnoski, D., and Ozbolat, I. T. (2017). The bioink: a comprehensive review on bioprintable materials. Biotechnol. Adv. 35, 217–239. doi:10.1016/j.biotechadv.2016.12.006
Huang, G. Y., Zhou, L. H., Zhang, Q. C., Chen, Y. M., Sun, W., Xu, F., et al. (2011). Microfluidic hydrogels for tissue engineering. Biofabrication 3, 012001. doi:10.1088/1758-5082/3/1/012001
Huang, X., Huang, Z., Gao, W., Gao, W., He, R., Li, Y., et al. (2022). Current advances in 3D dynamic cell culture systems. Gels 8, 829. doi:10.3390/GELS8120829
Hull, S. M., Brunel, L. G., and Heilshorn, S. C. (2022). 3D bioprinting of cell-laden hydrogels for improved biological functionality. Adv. Mater. 34, 2103691. doi:10.1002/ADMA.202103691
Jang, J., Park, H.-J., Kim, S.-W., Kim, H., Park, J. Y., Na, S. J., et al. (2017). 3D printed complex tissue construct using stem cell-laden decellularized extracellular matrix bioinks for cardiac repair. Biomaterials 112, 264–274. doi:10.1016/j.biomaterials.2016.10.026
Jenkins, P. M., Laughter, M. R., Lee, D. J., Lee, Y. M., Freed, C. R., and Park, D. (2015). A nerve guidance conduit with topographical and biochemical cues: potential application using human neural stem cells. Nanoscale Res. Lett. 10, 264–267. doi:10.1186/s11671-015-0972-6
Jeon, O. H., Panicker, L. M., Lu, Q., Chae, J. J., Feldman, R. A., and Elisseeff, J. H. (2016). Human iPSC-derived osteoblasts and osteoclasts together promote bone regeneration in 3D biomaterials. Sci. Rep. 6, 26761. doi:10.1038/srep26761
Jeong, E., Choi, S., and Cho, S. W. (2022). Recent advances in brain organoid technology for human brain research. ACS Appl. Mater Interfaces 15, 200–219. doi:10.1021/acsami.2c17467
Jeong, H.-J., Jimenez, Z., Mukhambetiyar, K., Seo, M., Choi, J.-W., and Park, T.-E. (2020). Engineering human brain organoids: from basic research to tissue regeneration. Tissue Eng. Regen. Med. 17, 747–757. doi:10.1007/s13770-020-00250-y
Johnson, B. N., Lancaster, K. Z., Hogue, I. B., Meng, F., Kong, Y. L., Enquist, L. W., et al. (2016). 3D printed nervous system on a chip. Lab. Chip 16, 1393–1400. doi:10.1039/c5lc01270h
Jorfi, M., D’Avanzo, C., Kim, D. Y., and Irimia, D. (2018a). Three-dimensional models of the human brain development and diseases. Adv. Healthc. Mater 7, 1700723. doi:10.1002/adhm.201700723
Jorfi, M., D’Avanzo, C., Tanzi, R. E., Kim, D. Y., and Irimia, D. (2018b). Human neurospheroid arrays for in vitro studies of Alzheimer’s disease. Sci. Rep. 8, 2450. doi:10.1038/s41598-018-20436-8
Jose, G., Shalumon, K. T., and Chen, J.-P. (2019). Natural polymers based hydrogels for cell culture applications. Curr. Med. Chem. 27, 2734–2776. doi:10.2174/0929867326666190903113004
Joung, D., Truong, V., Neitzke, C. C., Guo, S. Z., Walsh, P. J., Monat, J. R., et al. (2018). 3D printed stem-cell derived neural progenitors generate spinal cord scaffolds. Adv. Funct. Mater 28, 1801850–1801860. doi:10.1002/adfm.201801850
Jurek, B., Denk, L., Schäfer, N., Salehi, M. S., Pandamooz, S., and Haerteis, S. (2022). Oxytocin accelerates tight junction formation and impairs cellular migration in 3D spheroids: evidence from Gapmer-induced exon skipping. Front. Cell. Neurosci. 16, 1000538. doi:10.3389/fncel.2022.1000538
Kabirian, F., and Mozafari, M. (2019). Decellularized ECM-derived bioinks: prospects for the future. Methods 171, 108–118. doi:10.1016/J.YMETH.2019.04.019
Kaiser, A., Kale, A., Novozhilova, E., and Olivius, P. (2019). The effects of Matrigel® on the survival and differentiation of a human neural progenitor dissociated sphere culture. Anat. Rec. 303, 441–450. doi:10.1002/ar.24131
Kajtez, J., Buchmann, S., Vasudevan, S., Birtele, M., Rocchetti, S., Pless, C. J., et al. (2020). 3D-Printed soft lithography for complex compartmentalized microfluidic neural devices. Adv. Sci. (Weinh) 2001150, 2001150–2001164. doi:10.1002/advs.202001150
Kajtez, J., Nilsson, F., Fiorenzano, A., Parmar, M., and Emnéus, J. (2021). 3D biomaterial models of human brain disease. Neurochem. Int. 147, 105043. doi:10.1016/J.NEUINT.2021.105043
Kamande, J. W., Nagendran, T., Harris, J., and Taylor, A. M. (2019). Multi-compartment microfluidic device geometry and covalently bound poly-D-lysine influence neuronal maturation. Front. Bioeng. Biotechnol. 7, 84–89. doi:10.3389/fbioe.2019.00084
Karvinen, J., Joki, T., Ylä-Outinen, L., Koivisto, J. T., Narkilahti, S., and Kellomäki, M. (2018). Soft hydrazone crosslinked hyaluronan- and alginate-based hydrogels as 3D supportive matrices for human pluripotent stem cell-derived neuronal cells. React. Funct. Polym. 124, 29–39. doi:10.1016/j.reactfunctpolym.2017.12.019
Kelava, I., and Lancaster, M. A. (2016a). Dishing out mini-brains: current progress and future prospects in brain organoid research. Dev. Biol. 420, 199–209. doi:10.1016/J.YDBIO.2016.06.037
Kelava, I., and Lancaster, M. A. (2016b). Stem cell models of human brain development. Cell. Stem Cell. 18, 736–748. doi:10.1016/j.stem.2016.05.022
Khaing, Z. Z., and Seidlits, S. K. (2015). Hyaluronic acid and neural stem cells: implications for biomaterial design. J. Mater Chem. B 3, 7850–7866. doi:10.1039/C5TB00974J
Kilic, O., Pamies, D., Lavell, E., Schiapparelli, P., Feng, Y., Hartung, T., et al. (2016). Brain-on-a-chip model enables analysis of human neuronal differentiation and chemotaxis. Lab. Chip 16, 4152–4162. doi:10.1039/c6lc00946h
Klim, J. R., Williams, L. A., Limone, F., Guerra, I., Juan, S., Gamage, K. K., et al. (2019). ALS-implicated protein TDP-43 sustains levels of STMN2, a mediator of motor neuron growth and repair. Nat. Neurosci. 22, 167–179. doi:10.1038/s41593-018-0300-4
Knowlton, S., Anand, S., Shah, T., and Tasoglu, S. (2018). Bioprinting for neural tissue engineering. Trends Neurosci. 41, 31–46. doi:10.1016/J.TINS.2017.11.001
Koch, L., Deiwick, A., Soriano, J., and Chichkov, B. (2023a). Laser bioprinting of human iPSC-derived neural stem cells and neurons: effect on cell survival, multipotency, differentiation, and neuronal activity. Int. J. Bioprinting 9, 672. doi:10.18063/IJB.V9I2.672
Koch, L., Deiwick, A., Soriano, J., and Chichkov, B. (2023b). Laser bioprinting of human iPSC-derived neural stem cells and neurons: effect on cell survival, multipotency, differentiation, and neuronal activity. Int. J. Bioprint 9, 672. doi:10.18063/ijb.v9i2.672
Koo, Y., Hawkins, B. T., and Yun, Y. (2018a). Three-dimensional (3D) tetra-culture brain on chip platform for organophosphate toxicity screening. Sci. Rep. 8, 2841–2847. doi:10.1038/s41598-018-20876-2
Koo, Y., Hawkins, B. T., and Yun, Y. (2018b). Three-dimensional (3D) tetra-culture brain on chip platform for organophosphate toxicity screening. Sci. Rep. 8, 2841. doi:10.1038/s41598-018-20876-2
Koo, Y., Hawkins, B. T., and Yun, Y. (2018c). Three-dimensional (3D) tetra-culture brain on chip platform for organophosphate toxicity screening. Sci. Rep. 8, 2841. doi:10.1038/s41598-018-20876-2
Kovacs, G. G. (2019). Molecular pathology of neurodegenerative diseases: principles and practice. J. Clin. Pathol. 72, 725–735. doi:10.1136/jclinpath-2019-205952
Kunze, A., Giugliano, M., Valero, A., and Renaud, P. (2011). Micropatterning neural cell cultures in 3D with a multi-layered scaffold. Biomaterials 32, 2088–2098. doi:10.1016/j.biomaterials.2010.11.047
Kuo, Y.-C., and Wang, C.-C. (2013). Guided differentiation of induced pluripotent stem cells into neuronal lineage in alginate–chitosan–gelatin hydrogels with surface neuron growth factor. Colloids Surf. B Biointerfaces 104, 194–199. doi:10.1016/j.colsurfb.2013.01.001
Kuswandi, B., NurimanHuskens, J., and Verboom, W. (2007). Optical sensing systems for microfluidic devices: a review. Anal. Chim. Acta 601, 141–155. doi:10.1016/J.ACA.2007.08.046
Lancaster, M. A., Corsini, N. S., Wolfinger, S., Gustafson, E. H., Phillips, A. W., Burkard, T. R., et al. (2017). Guided self-organization and cortical plate formation in human brain organoids. Nat. Biotechnol. 35, 659–666. doi:10.1038/nbt.3906
Lancaster, M. A., and Knoblich, J. A. (2014). Generation of cerebral organoids from human pluripotent stem cells. Nat. Protoc. 9, 2329–2340. doi:10.1038/nprot.2014.158
Lancaster, M. A., Renner, M., Martin, C.-A., Wenzel, D., Bicknell, L. S., Hurles, M. E., et al. (2013). Cerebral organoids model human brain development and microcephaly. Nature 501, 373–379. doi:10.1038/nature12517
Laschke, M. W., and Menger, M. D. (2017). Life is 3D: boosting spheroid function for tissue engineering. Trends Biotechnol. 35, 133–144. doi:10.1016/J.TIBTECH.2016.08.004
Lee, H. K., Velazquez Sanchez, C., Chen, M., Morin, P. J., Wells, J. M., Hanlon, E. B., et al. (2016). Three dimensional human neuro-spheroid model of Alzheimer’s disease based on differentiated induced pluripotent stem cells. PLoS One 11, e163123. doi:10.1371/journal.pone.0163072
Lee, S. W. L., Campisi, M., Osaki, T., Possenti, L., Mattu, C., Adriani, G., et al. (2020). Modeling nanocarrier transport across a 3D in vitro human blood-brain–barrier microvasculature. Adv. Healthc. Mater 9, 1901486. doi:10.1002/adhm.201901486
Leite, P. E. C., Pereira, M. R., Harris, G., Pamies, D., dos Santos, L. M. G., Granjeiro, J. M., et al. (2019). Suitability of 3D human brain spheroid models to distinguish toxic effects of gold and poly-lactic acid nanoparticles to assess biocompatibility for brain drug delivery. Part Fibre Toxicol. 16, 22. doi:10.1186/s12989-019-0307-3
Li, W., Xu, Z., Xu, B., Chan, C. Y., Lin, X., Wang, Y., et al. (2017). Investigation of the subcellular neurotoxicity of amyloid-β using a device integrating microfluidic perfusion and chemotactic guidance. Adv. Healthc. Mater 6, 1600895. doi:10.1002/adhm.201600895
Liang, Y., Walczak, P., and Bulte, J. W. M. (2013). The survival of engrafted neural stem cells within hyaluronic acid hydrogels. Biomaterials 34, 5521–5529. doi:10.1016/j.biomaterials.2013.03.095
Liu, H., Lin, J., and Roy, K. (2006). Effect of 3D scaffold and dynamic culture condition on the global gene expression profile of mouse embryonic stem cells. Biomaterials 27, 5978–5989. doi:10.1016/j.biomaterials.2006.05.053
Liu, H., Wang, Y., Cui, K., Guo, Y., Zhang, X., and Qin, J. (2019). Advances in hydrogels in organoids and organs-on-a-chip. Adv. Mater. 31, e1902128. doi:10.1002/adma.201902042
Ma, J., Wang, Y., and Liu, J. (2018). Bioprinting of 3D tissues/organs combined with microfluidics. RSC Adv. 8, 21712–21727. doi:10.1039/c8ra03022g
Madl, C. M., Lesavage, B. L., Dewi, R. E., Dinh, C. B., Stowers, R. S., Khariton, M., et al. (2017). Maintenance of neural progenitor cell stemness in 3D hydrogels requires matrix remodelling. Nat Mater. 16(12), 1233-1242. doi:10.1038/NMAT5020
Madl, C. M., LeSavage, B. L., Dewi, R. E., Lampe, K. J., Heilshorn, S. C., Madl, C. M., et al. (2019). Matrix remodeling enhances the differentiation capacity of neural progenitor cells in 3D hydrogels. Adv Sci (Weinh). 11(4), 1801716. doi:10.1002/advs.201801716
Malda, J., Visser, J., Melchels, F. P., Jüngst, T., Hennink, W. E., Dhert, W. J. A., et al. (2013). 25th anniversary article: engineering hydrogels for biofabrication. Adv. Mater. 25, 5011–5028. doi:10.1002/adma.201302042
Mei, Q., Yuen, H. Y., and Zhao, X. (2022). Mechanical stretching of 3D hydrogels for neural stem cell differentiation. Biodes Manuf. 5, 714–728. doi:10.1007/s42242-022-00209-z
Miguel, L., Rovelet-Lecrux, A., Feyeux, M., Frebourg, T., Nassoy, P., Campion, D., et al. (2019). Detection of all adult Tau isoforms in a 3D culture model of iPSC-derived neurons. Stem Cell. Res. 40, 101541. doi:10.1016/j.scr.2019.101541
Möller, H.-J., and Graeber, M. B. (1998). The case described by Alois Alzheimer in 1911. Eur. Arch. Psychiatry Clin. Neurosci. 248, 111–122. doi:10.1007/s004060050027
Mosahebi, A., Simon, M., Wiberg, M., and Terenghi, G. (2001). A novel use of alginate hydrogel as Schwann cell matrix. Tissue Eng. 7, 525–534. doi:10.1089/107632701753213156
Motallebnejad, P., Thomas, A., Swisher, S. L., and Azarin, S. M. (2019a). An isogenic HiPSC-derived BBB-on-a-Chip. Biomicrofluidics 13, 064119. doi:10.1063/1.5123476
Motallebnejad, P., Thomas, A., Swisher, S. L., and Azarin, S. M. (2019b). An isogenic hiPSC-derived BBB-on-a-chip. Biomicrofluidics 13, 064119. doi:10.1063/1.5123476
Moutaux, E., Charlot, B., Genoux, A., Saudou, F., and Cazorla, M. (2018). An integrated microfluidic/microelectrode array for the study of activity-dependent intracellular dynamics in neuronal networks. Lab. Chip 18, 3425–3435. doi:10.1039/C8LC00694F
Moxon, S. R., Corbett, N. J., Fisher, K., Potjewyd, G., Domingos, M., and Hooper, N. M. (2019). Blended alginate/collagen hydrogels promote neurogenesis and neuronal maturation. Mater. Sci. Eng. C 104, 109904. doi:10.1016/j.msec.2019.109904
Murphy, A. R., Laslett, A., O’brien, C. M., and Cameron, N. R. (2017). Scaffolds for 3D in vitro culture of neural lineage cells. Acta Biomater. 54, 1-20. doi:10.1016/j.actbio.2017.02.046
Nahmias, Y., and Odde, D. J. (2006). Micropatterning of living cells by laser-guided direct writing: application to fabrication of hepatic–endothelial sinusoid-like structures. Nat. Protoc. 1, 2288–2296. doi:10.1038/nprot.2006.386
Namba, R. M., Cole, A. A., Bjugstad, K. B., and Mahoney, M. J. (2009). Development of porous PEG hydrogels that enable efficient, uniform cell-seeding and permit early neural process extension. Acta Biomater. 5, 1884–1897. doi:10.1016/J.ACTBIO.2009.01.036
Neves, S. C., Moroni, L., Barrias, C. C., and Granja, P. L. (2020). Leveling up hydrogels: hybrid systems in tissue engineering. Trends Biotechnol. 38, 292–315. doi:10.1016/J.TIBTECH.2019.09.004
Nguyen-Vu, T. D. B., Chen, H., Cassell, A. M., Andrews, R. J., Meyyappan, M., and Li, J. (2007). Vertically aligned carbon nanofiber architecture as a multifunctional 3-D neural electrical interface. IEEE Trans. Biomed. Eng. 54, 1121–1128. doi:10.1109/tbme.2007.891169
Nierode, G. J., Gopal, S., Kwon, P., Clark, D. S., Schaffer, D. V., and Dordick, J. S. (2019). High-throughput identification of factors promoting neuronal differentiation of human neural progenitor cells in microscale 3D cell culture. Biotechnol. Bioeng. 116, 168–180. doi:10.1002/bit.26839
Novak, R., Ingram, M., Clauson, S., Das, D., Delahanty, A., Herland, A., et al. (2019). A robotic platform for fluidically-linked human body-on-chips experimentation. bioRxiv Prepr. doi:10.1101/569541
Oddo, A., Peng, B., Tong, Z., Wei, Y., Tong, W. Y., Thissen, H., et al. (2019). Advances in microfluidic blood–brain barrier (BBB) models. Trends Biotechnol. 37, 1295–1314. doi:10.1016/J.TIBTECH.2019.04.006
Oliveira, E. P., Malysz-Cymborska, I., Golubczyk, D., Kalkowski, L., Kwiatkowska, J., Reis, R. L., et al. (2019). Advances in bioinks and in vivo imaging of biomaterials for CNS applications. Acta Mater. Inc. 95, 60–72. doi:10.1016/j.actbio.2019.05.006
Oohashi, T., Edamatsu, M., Bekku, Y., and Carulli, D. (2015). The hyaluronan and proteoglycan link proteins: organizers of the brain extracellular matrix and key molecules for neuronal function and plasticity. Exp. Neurol. 274, 134–144. doi:10.1016/J.EXPNEUROL.2015.09.010
Osaki, T., Sivathanu, V., and Kamm, R. D. (2018a). Engineered 3D vascular and neuronal networks in a microfluidic platform. Sci. Rep. 8, 5168–5181. doi:10.1038/s41598-018-23512-1
Osaki, T., Uzel, S. G. M., and Kamm, R. D. (2018b). Microphysiological 3D model of amyotrophic lateral sclerosis (ALS) from human iPS-derived muscle cells and optogenetic motor neurons. Sci. Adv. 4, eaat5847. doi:10.1126/sciadv.aat5847
Palma-Florez, S., López-Canosa, A., Moralez-Zavala, F., Castaño, O., Kogan, M. J., Samitier, J., et al. (2023). BBB-on-a-chip with integrated micro-TEER for permeability evaluation of multi-functionalized gold nanorods against Alzheimer’s disease. J. Nanobiotechnology 21, 115. doi:10.1186/s12951-023-01798-2
Pamies, D., Hartung, T., and Hogberg, H. T. (2014). Biological and medical applications of a brain-on-a-chip. Exp. Biol. Med. 239, 1096–1107. doi:10.1177/1535370214537738
Park, J., Lee, B. K., Jeong, G. S., Hyun, J. K., Lee, C. J., and Lee, S. H. (2015). Three-dimensional brain-on-a-chip with an interstitial level of flow and its application as an in vitro model of Alzheimer’s disease. Lab. Chip 15, 141–150. doi:10.1039/c4lc00962b
Park, J., Wetzel, I., Marriott, I., Dréau, D., D’Avanzo, C., Kim, D. Y., et al. (2018). A 3D human triculture system modeling neurodegeneration and neuroinflammation in Alzheimer’s disease. Nat. Neurosci. 21, 941–951. doi:10.1038/s41593-018-0175-4
Park, J. Y., Kim, S. K., Woo, D. H., Lee, E. J., Kim, J. H., and Lee, S. H. (2009). Differentiation of neural progenitor cells in a microfluidic chip-generated cytokine gradient. Stem Cells 27, 2646–2654. doi:10.1002/stem.202
Patterson, C. (2018). World Alzheimer Report 2018—the state of the art of dementia research: new frontiers. London, UK: Alzheimer’s Disease International.
Pedersen, J. A., Boschetti, F., and Swartz, M. A. (2007). Effects of extracellular fiber architecture on cell membrane shear stress in a 3D fibrous matrix. J. Biomech. 40, 1484–1492. doi:10.1016/j.jbiomech.2006.06.023
Pértile, R., Moreira, S., Andrade, F., Domingues, L., and Gama, M. (2012). Bacterial cellulose modified using recombinant proteins to improve neuronal and mesenchymal cell adhesion. Biotechnol. Prog. 28, 526–532. doi:10.1002/btpr.1501
Peyrin, J. M., Deleglise, B., Saias, L., Vignes, M., Gougis, P., Magnifico, S., et al. (2011). Axon diodes for the reconstruction of oriented neuronal networks in microfluidic chambers. Lab. Chip 11, 3663–3673. doi:10.1039/c1lc20014c
Pham, M. T., Pollock, K. M., Rose, M. D., Cary, W. A., Stewart, H. R., Zhou, P., et al. (2018). Generation of human vascularized brain organoids. Neuroreport 29, 588–593. doi:10.1097/WNR.0000000000001014
Plant, G. W., Harvey, A. R., and Chirila, T. V. (1995). Axonal growth within poly (2-hydroxyethyl methacrylate) sponges infiltrated with Schwann cells and implanted into the lesioned rat optic tract. Brain Res. 671, 119–130. doi:10.1016/0006-8993(94)01312-6
Poon, A., Zhang, Y., Chandrasekaran, A., Phanthong, P., Schmid, B., Nielsen, T. T., et al. (2017). Modeling neurodegenerative diseases with patient-derived induced pluripotent cells: possibilities and challenges. N. Biotechnol. 39, 190–198. doi:10.1016/J.NBT.2017.05.009
Poorna, M. R., Jayakumar, R., Chen, J. P., and Mony, U. (2021). Hydrogels: a potential platform for induced pluripotent stem cell culture and differentiation. Colloids Surf. B Biointerfaces 207, 111991. doi:10.1016/J.COLSURFB.2021.111991
Potjewyd, G., Moxon, S., Wang, T., Domingos, M., and Hooper, N. M. (2018). Tissue engineering 3D neurovascular units: a biomaterials and bioprinting perspective. Trends Biotechnol. 36, 457–472. doi:10.1016/j.tibtech.2018.01.003
Potter, W., Kalil, R. E., and Kao, W. J. (2008). Neural stem cell engineering: directed differentiation of adult and embryonic stem cells into neurons. Front. Biosci. 13, 21. doi:10.2741/2558
Qian, X., Nguyen, H. N., Song, M. M., Hadiono, C., Ogden, S. C., Hammack, C., et al. (2016). Brain-region-specific organoids using mini-bioreactors for modeling ZIKV exposure. Cell. 165, 1238–1254. doi:10.1016/j.cell.2016.04.032
Quadrato, G., Nguyen, T., Macosko, E. Z., Sherwood, J. L., Yang, S. M., Berger, D. R., et al. (2017). Cell diversity and network dynamics in photosensitive human brain organoids. Nature 545, 48–53. doi:10.1038/nature22047
Rahman, N. A., Rasil, A. N. H. M., Meyding-Lamade, U., Craemer, E. M., Diah, S., Tuah, A. A., et al. (2016). Immortalized endothelial cell lines for in vitro blood–brain barrier models: a systematic review. Brain Res. 1642, 532–545. doi:10.1016/J.BRAINRES.2016.04.024
Raja, W. K., Mungenast, A. E., Lin, Y.-T., Ko, T., Abdurrob, F., Seo, J., et al. (2016). Self-organizing 3D human neural tissue derived from induced pluripotent stem cells recapitulate Alzheimer’s disease phenotypes. PLoS One 11, e0161969. doi:10.1371/journal.pone.0161969
Rambani, K., Vukasinovic, J., Glezer, A., and Potter, S. M. (2009). Culturing thick brain slices: an interstitial 3D microperfusion system for enhanced viability. J. Neurosci. Methods 180, 243–254. doi:10.1016/J.JNEUMETH.2009.03.016
Ranjan, V. D., Qiu, L., Lee, J. W. L., Chen, X., Jang, S. E., Chai, C., et al. (2020). A microfiber scaffold-based 3D in vitro human neuronal culture model of Alzheimer’s disease. Biomater. Sci. 8, 4861–4874. doi:10.1039/D0BM00833H
Rao, S., and Winter, J. (2009). Adhesion molecule-modified biomaterials for neural tissue engineering. Front. Neuroengineering 2, 6. doi:10.3389/neuro.16.006.2009
Roach, P., Fricker, R. A., Evans, M. G., Kamudzandu, M., and Köse-Dunn, M. J. (2019). A micro-fabricated in vitro complex neuronal circuit platform. Biomed. Phys. Eng. Express 5, 045016. doi:10.1088/2057-1976/ab2307
Sala-Jarque, J., Mesquida-Veny, F., Badiola-Mateos, M., Samitier, J., Hervera, A., and del Río, J. A. (2020). Neuromuscular activity induces paracrine signaling and triggers axonal regrowth after injury in microfluidic lab-on-chip devices. Cells 9, 302. doi:10.3390/cells9020302
Sances, S., Ho, R., Vatine, G., West, D., Laperle, A., Meyer, A., et al. (2018a). Human iPSC-derived endothelial cells and microengineered organ-chip enhance neuronal development. Stem Cell. Rep. 10, 1222–1236. doi:10.1016/j.stemcr.2018.02.012
Sances, S., Ho, R., Vatine, G., West, D., Laperle, A., Meyer, A., et al. (2018b). Human iPSC-derived endothelial cells and microengineered organ-chip enhance neuronal development. Stem Cell. Rep. 10, 1222–1236. doi:10.1016/j.stemcr.2018.02.012
Santschi, M., Vernengo, A., Eglin, D., D’Este, M., and Wuertz-Kozak, K. (2019). Decellularized matrix as a building block in bioprinting and electrospinning. Curr. Opin. Biomed. Eng. 10, 116–122. doi:10.1016/j.cobme.2019.05.003
Sarker, M. D., Naghieh, S., Sharma, N. K., Ning, L., and Chen, X. (2019). Bioprinting of vascularized tissue scaffolds: influence of biopolymer, cells, growth factors, and gene delivery. J. Healthc. Eng. 2019, 1–20. doi:10.1155/2019/9156921
Schiele, N. R., Corr, D. T., Huang, Y., Raof, N. A., Xie, Y., and Chrisey, D. B. (2010). Laser-based direct-write techniques for cell printing. Biofabrication 2, 032001. doi:10.1088/1758-5082/2/3/032001
Shin, H. S., Kim, H. J., Sim, S. J., and Jeon, N. L. (2009). Shear stress effect on transfection of neurons cultured in microfluidic devices. J. Nanosci. Nanotechnol. 9, 7330–7335. doi:10.1166/jnn.2009.1769
Shin, Y., Kamm, R. D., Osaki, T., Campisi, M., and Sivathanu, V. (2017). In vitro microfluidic models for neurodegenerative disorders. Adv. Healthc. Mater 7, 1700489. doi:10.1002/adhm.201700489
Skylar-Scott, M. A., Huang, J. Y., Lu, A., Ng, A. H. M., Duenki, T., Liu, S., et al. (2022). Orthogonally induced differentiation of stem cells for the programmatic patterning of vascularized organoids and bioprinted tissues. Nat. Biomed. Eng. 6, 449–462. doi:10.1038/s41551-022-00856-8
Sloan, S. A., Andersen, J., Pașca, A. M., Birey, F., and Pașca, S. P. (2018). Generation and assembly of human brain region–specific three-dimensional cultures. Nat. Protoc. 13, 2062–2085. doi:10.1038/s41596-018-0032-7
Soares, C. P., Midlej, V., Oliveira, M. E. W. de, Benchimol, M., Costa, M. L., and Mermelstein, C. (2012). 2D and 3D-organized cardiac cells shows differences in cellular morphology, adhesion junctions, presence of myofibrils and protein expression. PLoS One 7, e38147. doi:10.1371/journal.pone.0038147
Sood, D., Cairns, D. M., Dabbi, J. M., Ramakrishnan, C., Deisseroth, K., Black, L. D., et al. (2019). Functional maturation of human neural stem cells in a 3D bioengineered brain model enriched with fetal brain-derived matrix. Sci. Rep. 9, 17874. doi:10.1038/s41598-019-54248-1
Stacey, G. (2006). “Primary cell cultures and immortal cell lines,” in Encyclopedia of life sciences (Chichester, UK: John Wiley and Sons, Ltd). doi:10.1038/npg.els.0003960
Staicu, C. E., Jipa, F., Axente, E., Radu, M., Radu, B. M., and Sima, F. (2021). Lab-on-a-Chip platforms as tools for drug screening in neuropathologies associated with blood–brain barrier alterations. Biomolecules 11, 916. doi:10.3390/BIOM11060916
Sullivan, M. A., Lane, S., Volkerling, A., Engel, M., Werry, E. L., and Kassiou, M. (2023). Three-dimensional bioprinting of stem cell-derived central nervous system cells enables astrocyte growth, vasculogenesis, and enhances neural differentiation/function. Biotechnol. Bioeng. 120, 3079–3091. doi:10.1002/BIT.28470
Sun, T., Norton, D., McKean, R. J., Haycock, J. W., Ryan, A. J., and MacNeil, S. (2007). Development of a 3D cell culture system for investigating cell interactions with electrospun fibers. Biotechnol. Bioeng. 97, 1318–1328. doi:10.1002/bit.21309
Sundararaghavan, H. G., Monteiro, G. A., Firestein, B. L., and Shreiber, D. I. (2009). Neurite growth in 3D collagen gels with gradients of mechanical properties. Biotechnol. Bioeng. 102, 632–643. doi:10.1002/bit.22074
Takahashi, K., Tanabe, K., Ohnuki, M., Narita, M., Ichisaka, T., Tomoda, K., et al. (2007). Induction of pluripotent stem cells from adult human fibroblasts by defined factors. Cell. 131, 861–872. doi:10.1016/j.cell.2007.11.019
Takahashi, K., and Yamanaka, S. (2006). Induction of pluripotent stem cells from mouse embryonic and adult fibroblast cultures by defined factors. Cell. 126, 663–676. doi:10.1016/j.cell.2006.07.024
Tang-Schomer, M. D., Wu, W. B., Kaplan, D. L., and Bookland, M. J. (2018). In vitro 3D regeneration-like growth of human patient brain tissue. J. Tissue Eng. Regen. Med. 12, 1247–1260. doi:10.1002/term.2657
Tate, C. C., Shear, D. A., Tate, M. C., Archer, D. R., Stein, D. G., and LaPlaca, M. C. (2009). Laminin and fibronectin scaffolds enhance neural stem cell transplantation into the injured brain. J. Tissue Eng. Regen. Med. 3, 208–217. doi:10.1002/TERM.154
Taylor, A. M., Blurton-Jones, M., Rhee, S. W., Cribbs, D. H., Cotman, C. W., and Jeon, N. L. (2005). A microfluidic culture platform for CNS axonal injury, regeneration and transport. Nat. Methods 2, 599–605. doi:10.1038/nmeth777
Tejera, D., and Heneka, M. T. (2019). “Microglia in neurodegenerative disorders,” in Microglia (Springer), 57–67.
Thomas, M., and Willerth, S. M. (2017). 3-D bioprinting of neural tissue for applications in cell therapy and drug screening. Front. Bioeng. Biotechnol. 5, 69–11. doi:10.3389/fbioe.2017.00069
Tsao, C.-W., Cheng, Y.-C., Cheng, J.-H., Fan, S.-K., Yao, D.-J., and Tung, Y.-C. (2015). Fluid flow shear stress stimulation on a multiplex microfluidic device for rat bone marrow stromal cell differentiation enhancement. Micromachines 6, 1996–2009. doi:10.3390/MI6121470
Uzel, S. G. M., Amadi, O. C., Pearl, T. M., Lee, R. T., So, P. T. C., and Kamm, R. D. (2016). Simultaneous or sequential orthogonal gradient formation in a 3D cell culture microfluidic platform. Small 12, 612–622. doi:10.1002/smll.201501905
Vadodaria, K. C., Amatya, D. N., Marchetto, M. C., and Gage, F. H. (2018). Modeling psychiatric disorders using patient stem cell-derived neurons: a way forward. Genome Med. 10, 1–8. doi:10.1186/s13073-017-0512-3
van der Helm, M. W., van der Meer, A. D., Eijkel, J. C. T., van den Berg, A., and Segerink, L. I. (2016). Microfluidic organ-on-chip technology for blood-brain barrier research. Tissue Barriers 4, e1142493. doi:10.1080/21688370.2016.1142493
Vatine, G. D., Barrile, R., Workman, M. J., Sances, S., Barriga, B. K., Rahnama, M., et al. (2019a). Human iPSC-derived blood-brain barrier chips enable disease modeling and personalized medicine applications. Cell. Stem Cell. 24, 995–1005.e6. doi:10.1016/j.stem.2019.05.011
Vatine, G. D., Barrile, R., Workman, M. J., Sances, S., Barriga, B. K., Rahnama, M., et al. (2019b). Human iPSC-derived blood-brain barrier chips enable disease modeling and personalized medicine applications. Cell. Stem Cell. 24, 995–1005.e6. doi:10.1016/j.stem.2019.05.011
Virlogeux, A., Moutaux, E., Christaller, W., Genoux, A., Bruyère, J., Fino, E., et al. (2018). Reconstituting corticostriatal network on-a-Chip reveals the contribution of the presynaptic compartment to Huntington’s disease. Cell. Rep. 22, 110–122. doi:10.1016/j.celrep.2017.12.013
Wang, Y., Wang, L., Zhu, Y., and Qin, J. (2018a). Human brain organoid-on-a-chip to model prenatal nicotine exposure. Lab. Chip 18, 851–860. doi:10.1039/c7lc01084b
Wang, Z., Boddeda, A., Parker, B., Samanipour, R., Ghosh, S., Menard, F., et al. (2018b). A high-resolution minimicroscope system for wireless real-time monitoring. IEEE Trans. Biomed. Eng. 65, 1524–1531. doi:10.1109/TBME.2017.2749040
Wen, Y.-Q., Gao, X., Wang, A., Yang, Y., Liu, S., Yu, Z., et al. (2018). Substrate stiffness affects neural network activity in an extracellular matrix proteins dependent manner. Colloids Surf. B Biointerfaces 170, 729–735. doi:10.1016/j.colsurfb.2018.03.042
Wilson, E., Knudson, W., and Newell-Litwa, K. (2020). Hyaluronan regulates synapse formation and function in developing neural networks. Sci. Rep. 10, 16459–16473. doi:10.1038/s41598-020-73177-y
Wu, S., Xu, R., Duan, B., and Jiang, P. (2017). Three-dimensional hyaluronic acid hydrogel-based models for in vitro human iPSC-derived NPC culture and differentiation. J. Mater Chem. B 5, 3870–3878. doi:10.1039/C7TB00721C
Xiao, W., Biswas, S., Cong, Z.-Q., Wen, H., Liao, Y.-H., Lam, K. S., et al. (2019). Alginate hydrogel modified with a ligand interacting with α3β1 integrin receptor promotes the differentiation of 3D neural spheroids toward oligodendrocytes in vitro. ACS Appl. Mater Interfaces 11, 5821–5833. doi:10.1021/acsami.8b19438
Xu, C., Dai, G., and Hong, Y. (2019). Recent advances in high-strength and elastic hydrogels for 3D printing in biomedical applications. Acta Biomater. 95, 50–59. doi:10.1016/J.ACTBIO.2019.05.032
Yi, H.-G., Jeong, Y. H., Kim, Y., Choi, Y.-J., Moon, H. E., Park, S. H., et al. (2019). A bioprinted human-glioblastoma-on-a-chip for the identification of patient-specific responses to chemoradiotherapy. Nat. Biomed. Eng. 3, 509–519. doi:10.1038/s41551-019-0363-x
Yu, F., and Choudhury, D. (2019). Microfluidic bioprinting for organ-on-a-chip models. Drug Discov. Today 00 24, 1248–1257. doi:10.1016/j.drudis.2019.03.025
Yue, K., Trujillo-de Santiago, G., Alvarez, M. M., Tamayol, A., Annabi, N., and Khademhosseini, A. (2015). Synthesis, properties, and biomedical applications of gelatin methacryloyl (GelMA) hydrogels. Biomaterials 73, 254–271. doi:10.1016/j.biomaterials.2015.08.045
Zhang, D., Teixeira, A. I., Falk, A., Pekkanen-Mattila, M., Herland, A., and Shahsavani, M. (2013). A 3D Alzheimer’s disease culture model and the induction of P21-activated kinase mediated sensing in iPSC derived neurons. Biomaterials 35, 1420–1428. doi:10.1016/j.biomaterials.2013.11.028
Zhang, Y., Chen, H., Long, X., and Xu, T. (2022). Three-dimensional-engineered bioprinted in vitro human neural stem cell self-assembling culture model constructs of Alzheimer’s disease. Bioact. Mater 11, 192–205. doi:10.1016/J.BIOACTMAT.2021.09.023
Zhang, Z.-N., Freitas, B. C., Qian, H., Lux, J., Acab, A., Trujillo, C. A., et al. (2016). Layered hydrogels accelerate iPSC-derived neuronal maturation and reveal migration defects caused by MeCP2 dysfunction. Proc. Natl. Acad. Sci. 113, 3185–3190. doi:10.1073/pnas.1521255113
Zhou, T., Tan, L., Cederquist, G. Y., Fan, Y., Hartley, B. J., Mukherjee, S., et al. (2017). High-content screening in hPSC-neural progenitors identifies drug candidates that inhibit Zika virus infection in fetal-like organoids and adult brain. Cell. Stem Cell. 21, 274–283.e5. doi:10.1016/j.stem.2017.06.017
Zhuang, P., Chua, C. K., Chew, S. Y., An, J., and Sun, A. X. (2017). 3D neural tissue models: from spheroids to bioprinting. Biomaterials 154, 113–133. doi:10.1016/j.biomaterials.2017.10.002
Keywords: neurodegenerative diseases, iPSC cell culture, 3D in vitro models, bioprinting, microfluidic device
Citation: Pereira I, Lopez-Martinez MJ and Samitier J (2023) Advances in current in vitro models on neurodegenerative diseases. Front. Bioeng. Biotechnol. 11:1260397. doi: 10.3389/fbioe.2023.1260397
Received: 17 July 2023; Accepted: 23 October 2023;
Published: 06 November 2023.
Edited by:
Qun Wang, Iowa State University, United StatesReviewed by:
Louis Jun Ye Ong, Queensland University of Technology, AustraliaCopyright © 2023 Pereira, Lopez-Martinez and Samitier. This is an open-access article distributed under the terms of the Creative Commons Attribution License (CC BY). The use, distribution or reproduction in other forums is permitted, provided the original author(s) and the copyright owner(s) are credited and that the original publication in this journal is cited, in accordance with accepted academic practice. No use, distribution or reproduction is permitted which does not comply with these terms.
*Correspondence: Josep Samitier, anNhbWl0aWVyQGliZWNiYXJjZWxvbmEuZXU=
Disclaimer: All claims expressed in this article are solely those of the authors and do not necessarily represent those of their affiliated organizations, or those of the publisher, the editors and the reviewers. Any product that may be evaluated in this article or claim that may be made by its manufacturer is not guaranteed or endorsed by the publisher.
Research integrity at Frontiers
Learn more about the work of our research integrity team to safeguard the quality of each article we publish.