- CenBRAIN Lab, School of Engineering, Westlake University, Hangzhou, China
Increasing population is suffering from neurological disorders nowadays, with no effective therapy available to treat them. Explicit knowledge of network of neurons (NoN) in the human brain is key to understanding the pathology of neurological diseases. Research in NoN developed slower than expected due to the complexity of the human brain and the ethical considerations for in vivo studies. However, advances in nanomaterials and micro-/nano-microfabrication have opened up the chances for a deeper understanding of NoN ex vivo, one step closer to in vivo studies. This review therefore summarizes the latest advances in lab-on-chip microsystems for ex vivo NoN studies by focusing on the advanced materials, techniques, and models for ex vivo NoN studies. The essential methods for constructing lab-on-chip models are microfluidics and microelectrode arrays. Through combination with functional biomaterials and biocompatible materials, the microfluidics and microelectrode arrays enable the development of various models for ex vivo NoN studies. This review also includes the state-of-the-art brain slide and organoid-on-chip models. The end of this review discusses the previous issues and future perspectives for NoN studies.
1 Introduction
The population that suffers from neurological disorders has been increasing rapidly in recent years. However, no therapy is available yet to effectively treat the majority of these neural diseases, including epilepsy, Alzheimer’s, and stroke (Pitkanen et al., 2015; Golyala and Kwan, 2017; Tang et al., 2017). Also, an increasing number of people have been diagnosed with depression and other mental illnesses, with no suitable medicine to choose from (Fiest et al., 2017). Such a situation makes it more urgent to understand the connections/networks between the brain’s neurons before carefully investigating the pathology of neural diseases. However, traditional methods such as electroencephalogram signals and functional near infrared spectroscopy do not provide sufficient accuracy and precision to study the brain. For example, electroencephalograms, the brainwave signals produced by active brain neurons, are recorded by electrodes around the head, forming neuroimages (Ramos-Argüelles et al., 2009; Kearney et al., 2018). A typical electroencephalogram recording device contains 20–40 electrodes. This indicates that one electrode records the entire signals from one particular large area but fails to focus on individual neurons with high resolution (Okamoto et al., 2004). To enable detailed ex vivo studies on networks of neurons (NoN), researchers are dedicated to building advanced lab-on-chip microsystems to better understand neurological diseases (Wong, 2011).
Microfluidic chips have been widely used to study neural cells and NoN and to build various ex vivo organ-on-chip models (Geraili et al., 2018; Holloway et al., 2021), including kidney (Asif et al., 2020), bone (George et al., 2018; Sheyn et al., 2019; Truesdell et al., 2020), heart (Kofron and Mende, 2017), liver (Maschmeyer et al., 2015), muscle (Morimoto et al., 2013), and brain (Kaneko et al., 2020). The advantages of microfluidics in high-throughput, high-efficiency integration, miniaturization, flexible architecture, and low costs in fabrication speed up the microfluidics-based ex vivo NoN studies (Sharma et al., 2013; Kou et al., 2016). Organ-on-chip devices, on the one hand, can better study the organs, and on the other hand, can be applied for drug discovery and toxicity tests to screen promising therapeutic drugs (Cao and Köhler, 2015; Osaki et al., 2018). Importantly, organs on chips can be customized as needed by manipulating cells inside the microfluidic chips (Yao et al., 2019).
Despite the advances in building advanced brain models with microfluidics, there remains a challenge in continuously recording the neural activities for real-time NoN studies ex vivo. Currently, patch clamp electrophysiology remains the gold standard for electrophysiological characterization of individual neurons from cells owing to its low noise and high resolution (Bébarová, 2012; Barthmes et al., 2014). This method however requires large equipment and complicated operations, which might damage the cells, and most undesirably, records the activity of a single cell, rather than the activities of cell populations each time (Accardi et al., 2016; Gao et al., 2021a). To address this challenge, microelectrode arrays (MEAs) have been proposed in recent years to monitor neural cell activities in real time (Newberry et al., 2016). Integration of cell culture with signals recorded in parallel by a well-designed MEA seems a more promising technique for ex vivo study of the NoN (Regehr et al., 1989; Johnstone et al., 2010). Compared to the patch clamp, MEAs can monitor thousands of cells on one single chip but cause no damage to cells. Also, MEA chips are small in size and easy to operate. One limitation of MEAs is that compared to a patch clamp that records intercellular potential, MEA records extracellular potential, which may lose some sensitivity. However, this limitation has been minimized recently through elaborate treatment on microelectrode surfaces (Choi et al., 2021). For example, the latest works showed that three-dimensional (3D) structured electrodes (Desbiolles et al., 2019; Saito, 2019; Yoo et al., 2020; Xu et al., 2021) enable MEAs to record the intercellular potential by membrane permeabilization or inserting electrodes into cells.
This review aims to systematically summarize the latest advances in lab-on-chip microsystems for ex vivo NoN studies. For a clear understanding, we divide the review into three sections: advanced materials for ex vivo NoN studies, advanced techniques for ex vivo NoN studies, and advanced models for ex vivo NoN studies. The schematic illustration of this review is briefly shown in Figure 1.
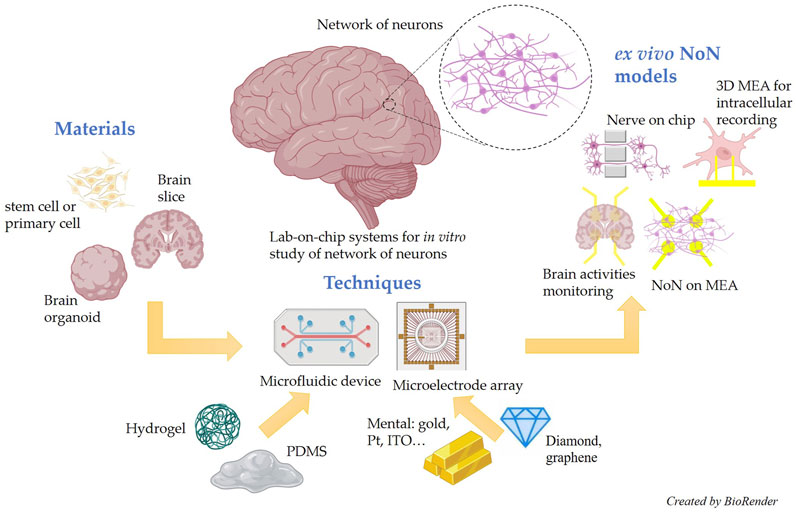
FIGURE 1. Schematic illustration of the state-of-the-art materials, techniques, and models for lab-on-chip microsystem-based ex vivo NoN studies.
2 Advanced Materials for Ex Vivo NoN Studies
2.1 Functional Biomaterials for Building Models
Human bodies cannot be studied directly for multiple reasons, including ethical and safety issues. To better study human diseases, human stem cells are a better alternative than animal-derived cells to build functional organ-on-chip models. This is more often the case in the fields of drug discovery and toxicity tests as there is a rather big gap between what happens in human bodies versus in animals. Previous research in sepsis showed that molecular mechanisms differ significantly between mice and humans, despite their similar disease phenotype (Seok et al., 2013). For brain-on-chip models, microfluidic chips with specific shapes have been proposed to culture various types of neural cells. The two main types of cells used in neural models are primary cells and stem cells (Grainger et al., 2018). Compared to stem cells, primary cells represent the organs inside the body better but are harder to obtain and proliferate slower. Also, primary neural cells are usually generated by animals, instead of humans. On the contrary, stem cells can reproduce themselves indefinitely and differentiate into any cell type in a suitable environment (Takahashi and Yamanaka, 2006; Takahashi et al., 2007). Stem cells, especially induced pluripotent stem cells (iPSC), are derived from human somatic cells and exhibit humanoid morphology. Disease models can be improved by using stem cells from patients without ethical considerations.
Glial cells also play an essential role in neural activity since they support neural growth and biochemical transportation. Previous research indicated that glial cells could be related to many neurodegenerative diseases (Miller et al., 2004). For instance, astrocytes, the most abundant glial cells in the brain, regulate blood flow in the blood–brain barrier to keep the central neural system stable. Meanwhile, they are also quite sensitive to pathological triggers like infection and stroke. Regarding brain-on-chip models, co-culture of glial cells with neural cells can provide neurons with a better microenvironment and promote axon growth. Ahn et al. proposed a blood–brain barrier model by endothelial cells, pericytes, and astrocytes, as shown in Figure 2A (Ahn et al., 2020). The chip had two microchannels, combining a 2D endothelial monolayer with a 3D brain microenvironment, which mimicked the in vivo environment very well. This model was a promising tool for testing neural drugs and studying the blood–brain barrier in both physiological and pathological conditions.
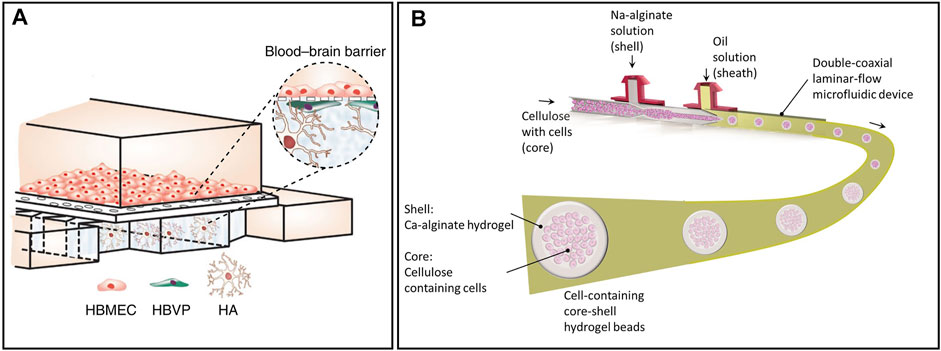
FIGURE 2. Representative advanced materials to build brain models ex vivo. (A) A blood–brain barrier model by endothelial cells, pericytes, and astrocytes (HBMEC: human brain microvascular endothelial cells, HBVP: human brain vascular pericytes; HA: human astrocytes) (Ahn et al., 2020). (B) A coaxial flow-focusing capillary-assembled microfluidic device to fabricate spherical hydrogel beads with cells in it (Liu et al., 2020).
Culturing neural cells in vitro helps us observe the developmental process of neural cells and the connections between neurons. Given that the brain is much more complicated than artificial NoN on a chip, it is impossible to be fully reconstructed in vitro. In addition, various neural cells, such as neurons, astrocytes, and microglia, are present in the human brain and interact with each other to play important roles in brain activity (Goshi et al., 2020). Artificial neural circuits help us understand the fundamental mechanisms of brain function but fail to achieve high-order functions such as emotion, memory, and recognition. In this respect, brain slides from animals can be an excellent alternative to establishing neural disease models as their structures are much closer to the human brain than planar NoN.
2.2 Functional Biocompatible Materials for Device Fabrication
The organ-on-chip model has become a hot topic in recent years as it mimics what happens inside the body to a great extent, with a low-cost and easy-to-fabricate process (Benam et al., 2015; Kim et al., 2015; Xu et al., 2016; Zhang et al., 2009). In contrast to traditional cells cultured in a petri dish, real organs are usually cultured in 3D structures. As such, scaffolds are required to culture the cells in 3D structures to mimic organs in the body (Murphy et al., 2017; Zhang et al., 2019). Hydrogel is one of the most popular scaffold materials in the 3D cell culture due to its excellent biocompatibility and softness. Additionally, the mechanical properties of hydrogen are similar to those of human organs under certain conditions (Pek et al., 2010; Zhu and Marchant, 2011; Mahoney and Anseth, 2006). Other materials like fibrous and solid porous are also popular for cell culture use for various applications (Hayman et al., 2005; Cao et al., 2009). Liu et al. proposed a coaxial flow-focusing capillary-assembled microfluidic device to fabricate spherical hydrogel beads with cells in them, as shown in Figure 2B (Liu et al., 2020). Alginate, one kind of hydrogel that could be solidified with calcium iron, was used as the shell of the sphere. Cells inside the spheres could grow well due to the permeability, biocompatibility, and softness of hydrogel. It was a promising tool that could be used to culture brain organoids. Some other hydrogels, such as matrigel and collagen, are mainly used to coat the surface of culture dishes or chips to promote cell adherence and growth.
As learned from the abovementioned messages, MEA is widely used to record signals from cells in a given small area. To fabricate MEA, gold is considered an ideal material because of its high conductivity and biocompatibility. For fluorescence immunoassay experiments that require high transparency of the substrate for better observation, indium tin oxide glass, as a combination of electrical conduction and optical transparency, is more often used in fabricating such microelectrodes. However, the relatively high impedance to obtain high transparency leads to high background noise and a low signal-to-noise ratio. Poly(3,4-ethylenedioxythiophene) polystyrene sulfonate (PEDOT: PSS) (Koutsouras et al., 2017; Kshirsagar et al., 2019; Susloparova et al., 2021) is a new interfacial material for modern bioelectronics and is better than ITO in some aspects, such as conductivity and flexibility. It is still facing some challenges like longevity and multichannel (Liang et al., 2021). As such, new materials have been developed in recent years to improve the electrodes, such as boron-doped diamond (McDonald et al., 2017) and graphene (Koerbitzer et al., 2016; Thunemann et al., 2018). Their excellent properties in better transparency and higher conductivity make them attractive for various applications in the near future.
For microfluidic chips, the most common material is polydimethylsiloxane (PDMS) because of its excellent elasticity, optical transparency, and biocompatibility (Wang et al., 2014). It also has high resolution on microstructure fabrication and has been widely used to build microchannels and microwells by molding. The basements are usually silicon wafers with microstructures on them. If the precision demand is not high, 3D-printed soft lithography is a good choice due to its low cost and ease of fabrication (Wu et al., 2017).
3 Advanced Techniques for Ex Vivo NoN Studies
3.1 Microfluidic Systems
Micro-/nano-fabrication techniques such as photoetching and thin film deposition have been developing rapidly recently. Microfluidic systems are getting popular in the bioengineering field for neural degeneration disease modeling, neuroprotective mechanism modeling, and neural circuit establishment (Lassus et al., 2018). Single-cell manipulation can be achieved in the microfluidic chip, and microenvironment around cells can be controlled precisely, making microfluidic systems an up-and-coming tool to conduct neural study (Chen et al., 2011).
3.1.1 Cell Manipulation for Single-Cell Study
The advance of modern technologies is overturning some conclusions drawn in previous studies. In our traditional cognition, only nerve cells are involved in neural communication. Increasing shreds of evidence show that gliocytes also play an essential role in neural communication, although the effect of gliocytes on the whole process remains to be understood (Liu and Wang, 2016; Zhang et al., 2016). In this case, cell manipulation techniques provide a promising way to study cell communication at a single cell level (Yun et al., 2013). Fluorescence-activated cell sorting is a classic method for single-cell analysis in biological labs, which requires expensive equipment and complicated sample pretreatment. The character of instantaneous detection made this technique impossible to achieve long-term monitoring. In this respect, the microfluidic chip is a promising platform for cell manipulation and long-term continuous monitoring due to its biocompatibility, design, and low cost. Various microfluidic chips have been designed to manipulate cells in the past 10 years (Sen et al., 2013; Chu et al., 2015; Miled et al., 2015; Zhao et al., 2020).
One of the most popular methods for manipulating cells is dielectrophoresis (DEP). Neutral particles will polarize under a non-uniform electric field and be subject to dielectrophoretic force. After that, they move to a position where electric field is stronger or weaker, depending on the permittivities of the cells and solution (Green et al., 2000). The equation of DEP can be expressed as follows (Giugiulan et al., 2014):
where dc is the diameter of cells or neutral particles; Erms is the root mean square of electric field intensity; and
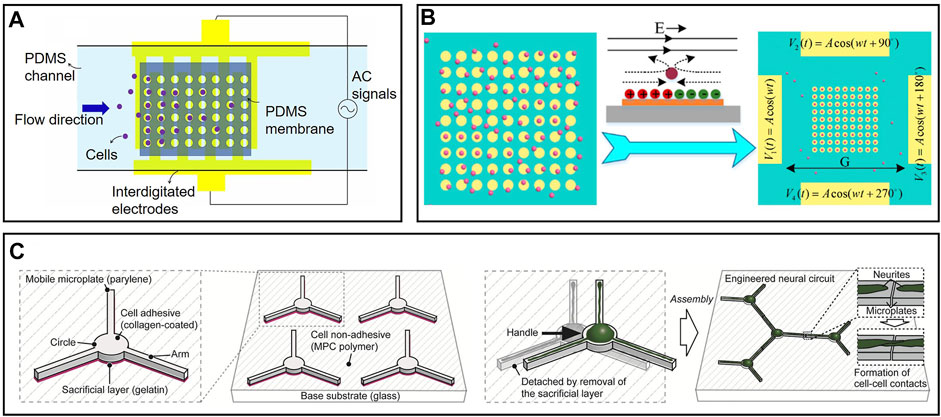
FIGURE 3. Representative cell manipulation on microfluidic chips: (A) Cell manipulation chip based on dielectrophoresis via interdigitated electrodes. Cells were attracted by non-uniform electrical field and fall into microwells (Farasat et al., 2021). (B) Cell manipulation based on induced charge electroosmosis. Particles moved with the flow and trapped in the center of electrodes (Wu et al., 2016). (C) Cells would move to cell-adhesive mobile plate automatically. Neural circuits could be establishment by manipulating these microplates (Yoshida et al., 2016).
Recently, induced-charge electroosmosis has also gained increasing attention in manipulating cells (Nam et al., 2015; Ren et al., 2015; Ren et al., 2016). Electroosmosis and electrophoresis achieve similar effects, but the mechanisms behind them are different. For electrophoresis, particles are driven by electrophoresis directly, and the solution is stable; for electroosmosis, particles remain stationary relative to the solution as driven by electroosmosis. Wu et al. (2016) proposed a simple cell capture device on MEAs based on induced charge electroosmosis, as shown in Figure 3B. Four driving electrodes are energized with four sine signals with different phases to create a rotating electric field. On the surface of each microelectrode in a rotating electric field, flow vortices are formed that transport particles to the center of the electrode. Compared to the DEP method mentioned above, this device is easier to fabricate and infinitely expandable. Cells can be trapped in any place as desired in the rotating electric field by simply adding a microelectrode. The limitation of this device lies in its low efficiency as particles flow with the solution.
Except for passive movement driven by external forces, cells can sometimes migrate to cell-adhesive areas from non-cell-adhesive areas spontaneously. Various shapes of cell-adhesive patterns have been designed on microfluidic chips to control the growth of neurons or axons (Merz and Fromherz, 2002; Onoe and Takeuchi, 2008; Fricke et al., 2011; Roth et al., 2012; Benzina et al., 2014). In addition, pieces of evidence show that circular-shaped and linear-shaped micropatterns have different extents of adhesion to neural soma and neurites. Yoshida et al. proposed an exciting method to establish freely combinable neural circuits in vitro via a modified mobile microplate, as shown in Figure 3C (Yoshida et al., 2016). These microplates have a circular body and several linear arms, corresponding to neural soma and axons, respectively. They were placed on a large planar cell-repulsive substrate so that cells could spontaneously migrate to the mobile microplates. The mobility of microplates enabled neural circuits to be built up by moving their arms close by. Compared to traditional fixed cell-adhesive pattens, this method is extensible, controllable, and more efficient as microplates without cells can be easily removed from the basement.
We should notice that most of the cell manipulations based on electrical fields require tuning of the conductivity of the solution. Increased conductivity may turn DEP from being positive to being negative. Conductivity also determines which electrophoresis and electroosmosis are dominant. Commonly, a small number of irons are added to the solution to adjust the conductivity, and sucrose is used to adjust the osmotic pressure. Also, any changes in the solution or external electric field may affect cells, especially neural cells. In order to minimize the external influences on cells, microstructure-based cell manipulation, which does not require any electrical field or adjustment of conductivity, has become great interest to researchers (Carlo et al., 2006; Frimat et al., 2011; Pang et al., 2020). Such microstructures include cup-shaped traps (Di Carlo et al., 2006), micropillars (Tan et al., 2009), and pneumatic valves (Kim and Kim, 2014). A surface acoustic wave, as a mechanical wave, is also used to arrange cells without the requirement of solution conductivity (Collins et al., 2015). Acoustic wells with minimum local potential are generated in the acoustic field where particles fall into place. The density of acoustic wells can be adjusted by changing the ratio of acoustic wavelength to particle diameter. This method not only avoids direct contact between cells and microstructures but also has no requirements on the properties of particles, showing high potential in cell manipulation.
3.1.2 Microenvironment Control on a Chip
The environment close to the cells is critical as one tiny factor may cause remarkable effects on cells, particularly for stem cells. The microenvironment decides the direction of cell differentiation, cellular vitality, and lifetime. Plenty of methods have been proposed for long-term cell culture and maintaining a stable extracellular microenvironment (Choi et al., 2015; Oyama et al., 2018; Mun et al., 2020). Scientists may change the extracellular environment deliberately to build up a certain disease model in order to show how the changes in the microenvironment in the brain cause the onset of a disease (Osaki et al., 2018). Stroke, the most significant cause of adult disability, is caused by a lack of blood or oxygen in the brain. Still, no effective neuroprotective therapy has been proposed to treat stroke (O'Collins et al., 2006). Chen et al. proposed a microfluidic device that can preciously generate an oxygen gradient in the microchannel by chemical reactions, as shown in Figure 4A (Chen et al., 2011). A PDMS with high gas permeability was patterned by a soft lithography technique and adhered to a glass substrate. Three microchannels were formed and separated by thin PDMS membranes with a thickness less than 50 µm. Two channels on the side were used to produce and scavenge oxygen, respectively, by chemical reactions. One wider channel in the middle was used for cell culture, where an oxygen gradient is generated and stably maintained. Compared to conventional gas control devices that require complicated equipment and a large volume of gas, microfluidic devices are easy to build, small in size, low cost, and energy efficient. Besides, it avoids direct contact between cells and chemicals, which minimizes the effects of the latter. Additionally, this device can produce a gradient of other gases by changing the reaction chemicals, enhancing its rationality and usability.
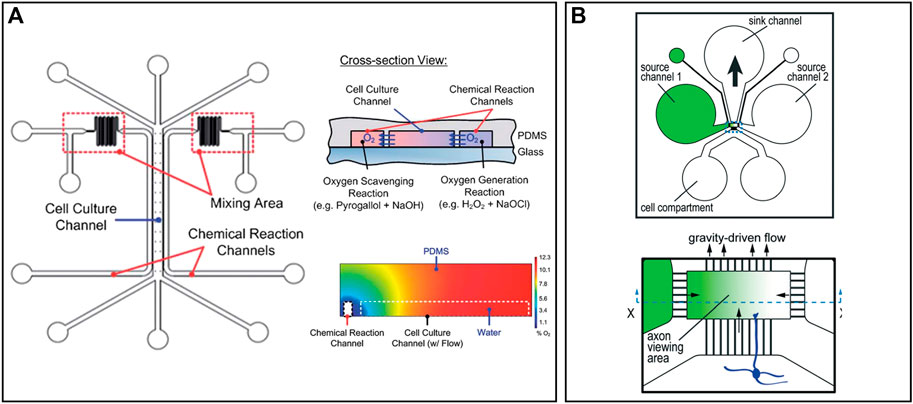
FIGURE 4. Representative microenvironment control and MEAs on chips: (A) A microfluidic device that can precisely generate an oxygen gradient in the microchannel by chemical reactions (Chen et al., 2011). (B) Five microfluidic chambers connected by microtunnels to create a solution concentration gradient. Axons grow through microchannels and effected by the concentration gradient (Taylor et al., 2015).
Solution concentration gradient generation is a little different from gas because liquid cannot go through the PDMS. Taylor et al. designed a microfluidic gradient chamber to study the influence of growth factors on mouse neocortical neurons, as shown in Figure 4B (Taylor et al., 2015). A total of four channels were built around the axon viewing area, where a gradient of growth factor concentration was produced. Left and right channels were used to input culture solutions with different concentrations of growth factors. The height of the axon viewing area is much smaller than other channels, which decreases the flow rate to stabilize the gradient. The solution will flow out through the upper channel due to gravity. Neural cells were cultured in the channel below and axons grew through the microgrooves, which reduced the influence of the initial growth direction of axons. Compared to traditional methods with a concentration gradient in different petri dishes, this microfluidic chip simplified the operation and better controlled the initial conditions of axons.
3.2 Microelectrode Array Systems
Commercially available planar MEAs are 8
Noise could be an issue during the electrophysiology recordings since the electrical signals produced by cells are rather small. Except for the system noise, the majority of the noise comes from the change of the ion environment near electrodes (Hai et al., 2010; Mateus et al., 2019). Thus, increasing the contact area between cells and electrodes is a promising strategy to increase the signal-to-noise ratio. Abbott et al. proposed a nanoelectrode array coated with platinum-black, which not only increased the surface area but also lowered the impedance of electrodes (Abbott et al., 2020a; Abbott et al., 2020b). A cell–electrode interface model was also built, as shown in Figure 4C. Additionally, intracellular signals can be detected through membrane permeabilization, induced by a small faradaic current. In this way, tiny signals can be recorded, which is the key to investigating the synaptic connectivity between neurons. One individual electrode performs similarly to the patch clamp, but the entire electrode array is scalable, subminiaturized, and of high density, which is beyond the reach of the patch clamp. Cell stimulation can also be achieved in each electrode by a small current. The stable and precise recording makes network-wide mapping of neurons possible, which certainly promotes further research into the human brain ex vivo.
Not only electrical signals can be used for neural recording and stimulation, but optical signals and chemical signals can also represent the activities of neurons. Through optogenetic modification, inserting light-sensitive genes into cells, cells can be stimulated by different colors of light (Yoo et al., 2020). Meanwhile, neural activity can be reflected by fluorescence intensity in some aspects. In addition, the optogenetic technique has already been used in vivo several years ago to control the brains of mice, creating the most famous mouse that has been featured in countless top journals (Nabavi et al., 2014). The fundamental principle of chemical recording is that neurons release neurotransmitters when they communicate with each other. Various biosensors have been proposed to monitor these chemicals in real time (Su et al., 2020). Also, some chemicals can be used to stimulate neurons. Amanda et al. proposed a bioelectronic neural pixel to stimulate neurons by chemicals and record their activities simultaneously with PEDOT:PSS electrodes because it has both conductivity and permeability (Amanda et al., 2016). A cation exchange membrane was used to deliver chemicals, and gold was used to conduct electrical signals. This bioelectronic neural pixel is a potential platform to study the effects of different neurotransmitters on neurons.
Furthermore, culturing cells on MEAs for the long-term is essential for NoN development, as axons and connections between cells take time to grow. The major obstacle to long-term culture is infection from the environment, which can be minimized with the use of a clean environment and standard operation. Previous research demonstrated that hyperosmolality, caused by medium evaporation, is the second reason for neuron apoptosis (Potter and DeMarse, 2001). Many devices for long-term culture of neural cells have therefore been equipped with their own gas supply system or membrane-sealed chamber to avoid evaporation.
4 Advanced Models for Ex Vivo NoN Studies
4.1 Microfluidic-Based Models for Ex Vivo NoN Studies
Kramer et al. fabricated a lollipop-shaped container using dual hydrogels to develop a myelinated peripheral nerve model, as shown in Figure 5A (Khoshakhlagh et al., 2018; Kramer et al., 2020). Neural cells and gliocytes are co-cultured in a spheroid microplate to form a cell sphere that will be moved to the circle part of the container. Axons will grow through the channel in a few weeks. In order to mimic the human brain to a great extent, researchers also applied human iPSC to build a peripheral nerve model (Sharma et al., 2019). It is a desirable platform to study neurodegenerative diseases and perform drug-related tests. After four weeks of culture, a patch clamp was used to monitor the electrophysiology of their nerve model, including nerve conduction velocity and amplitude, which are gold standards for evaluating chemotherapy-induced peripheral neuropathy (Brewer et al., 2016; Stagg et al., 2016). Meanwhile, the nerve model was challenged by six types of drugs with or without neuropathic properties, and the results were as expected, demonstrating its reliability in drug tests.
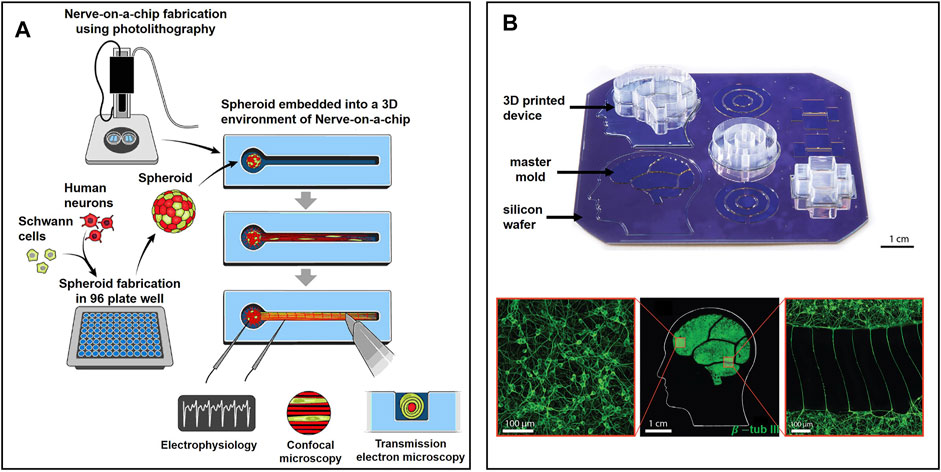
FIGURE 5. Representative state-of-the-art microfluidic-based models for ex vivo NoN studies: (A) Lollipop-shaped container made by dual hydrogel to develop myelinated peripheral nerve model by human iPSC (Sharma et al., 2019). (B) Micro-chambers and -tunnels made by 3D-printed soft lithography to study Parkinson’s disease. Neurons were connected by axons through microtunnels (Kajtez et al., 2020).
It is nearly impossible to decrypt the brain even with modern state-of-the-art technologies due to the complexity of our brain. In this case, guiding neurites by microtunnels between two isolated microfluidic regions provides an alternative way to study the NoNs (Jean-Michel et al., 2011; Renault et al., 2015; Samson et al., 2016a; Samson et al., 2016b; Renault et al., 2016). Such type of microfluidic device can be used to study synaptic plasticity, disease processes, and communication between different brain regions that are related to certain neural diseases. For example, in a generalized seizure, epileptic seizures spread to the whole brain, while in a focal seizure, the seizures stay within a certain small area (Fisher et al., 2017). The effects of the central nervous system on other tissues, such as muscles and blood vessels, can also be studied on the chip by guiding axons to germinate in the corresponding tissues through microchannels while preventing cell migration (Gilbert-Honick et al., 2020). However, the development of this technology is limited due to the complicated operations of soft lithography, which generally includes the pouring of liquid PDMS into a micro-structured wafer and tearing it off manually once solidified. Such a process can damage the microstructures and limit the design options, as structures in 3D and with large aspect ratios cannot be molded.
Three-dimensional-printed soft lithography provides a better option to fabricate complex microfluidic structures, as PDMS can be extruded everywhere on the chip with high resolution. Kajtez et al. proposed a prototype composed of several chambers which are connected by microtunnels (Kajtez et al., 2020). Gasket ink and compartment ink were developed for extrusion in a master mold to generate tall “walls” between chambers, as shown in Figure 5B. Human neural stem cells were cultured on the microfluidic chip to study Parkinson’s disease via modeling of nigrostriatal pathways by guiding the growth of dopaminergic projections through microchannels. Compared with traditional lithography methods, 3D-printed soft lithography has high precision and high resolution and greatly simplifies the fabrication steps. These advantages make it widely applied for printing the framework for cell growth and cell communication (Achille et al., 2021). Three-dimensional bioprinting was developed on the basis of 3D printing. Cells and scaffolds are generally made of polymers and extruded from a nozzle and cultured in 3D (Elalouf, 2021; Jamee et al., 2021). Many organs, such as bone (Arrigoni et al., 2017; Kim et al., 2021; Alcala-Orozco et al., 2022), lung (Hu et al., 2021), and skin (Gao et al., 2021c), have already been generated by 3D bioprinting, showing plenty of potential in the future. Cell delivery (Hwang et al., 2022) and tumor modeling (Samadian et al., 2021) can also be achieved by bioprinting. Today, 3D printing is still in its initial stage and requires much effort to improve, especially in cell co-culture technique, bioink properties, and fabrication methods. Nevertheless, the great benefits of flexible design and rapid prototyping will certainly ensure a bright future for 3D bioprinting in the coming years.
Except for building neuron models in microfluidic systems, the system can even perform like a neuron after elaborate design. Levi et al. proposed a microfluidic neuron that is similar to a biological neuron (Levi and Fujii, 2016). Each microfluidic neuron had a chamber representing an intracellular environment. Chambers were connected by microtunnels, representing axons that transmit signals. The exchange of irons was controlled by Quake valves and selective ion permeable membranes in microtunnels. Thus, the membrane potentials were formed and measured by integrated electrodes. It could be a promising tool to explore in neuromorphic engineering.
4.2 3D MEA-Based Models for Ex Vivo NoN Studies
Large-scale MEAs can monitor the neural activities of the whole NoN, but it remains challenging to attain high resolution and to record potential changes in a single cell. Decreasing the size of the electrode is an alternative solution to achieve high-resolution recording. In this way, noise can also be reduced as the entire surface of the electrode is covered by cells, which avoids any potential negative effects from iron in solution. Inspired by the morphology of a synapse, Wijdenes et al. proposed a novel bio-mimicking and high-resolution planar MEAs for long-term neural recordings, as shown in Figure 6A (Wijdenes et al., 2016). Several electrodes were applied to record one single cell simultaneously, increasing the resolution by 14 times as compared to the traditional planar electrode. Additionally, the edge of each electrode was modified with gold nanostructures to increase the contact area between neurons and electrodes. This nano-edge structure prevented current leakage into the environment, which reduced the noise and obtained high-quality signals.
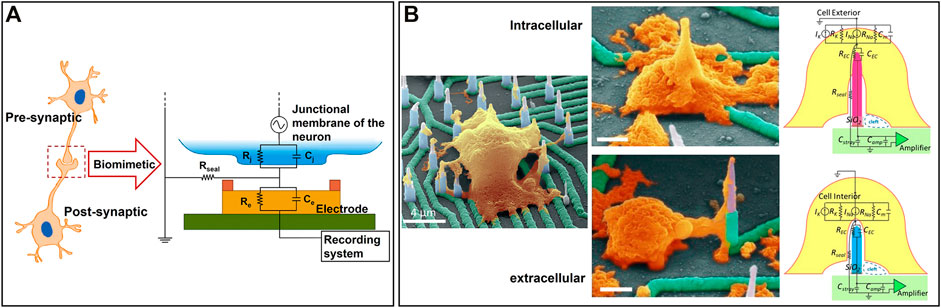
FIGURE 6. Representative state-of-the-art 3D MEA-based models for ex vivo NoN studies: (A) A bio-mimicking and high-resolution planar MEAs for long-term neural recordings (Wijdenes et al., 2016). (B) A 3D high-density nanowire array for both exocellular and intracellular signals recording (Liu et al., 2017a).
In order to further improve the signal quality and the sensitivity of MEAs, 3D microstructures can be modified on the top of the electrodes, such as nanostraws (VanDersarl et al., 2012), nanowires (Robinson et al., 2012), and nanopillars (Xie et al., 2012). The main advantage of 3D structured electrodes is that intracellular action potential can be detected, which reflects the information of ion channels and synapses of a single cell (Mechler et al., 2011). Although some of the aforementioned planar electrodes can record intracellular signals by electroporation (Abbott et al., 2020a; Buzsáki, 2004), they may disturb spontaneous cell activities at the same time as the membrane is damaged. Also, such electrodes require complicated circuit design and operation. In this case, 3D structured electrodes provide a harmless, simple way to measure high-quality exocellular and intracellular potential changes. Liu et al. generated a 3D high-density nanowire array to record both exocellular and intracellular signals (Liu et al., 2017a). Electrical circuit models were built, as shown in Figure 6B. Planar circuits were made of Ni and patterned by photolithography and electron beam lithography on top of a transparent sapphire substrate. A Ni mask and plasma etch technology were used to generate the Si nanowire with a proper height of 10 µm and SiO2 being deposited as the insulating layer. Human iPSC were cultured on the nanowire and induced into neurons. Nanowire electrodes under the soma entered into cells during the growth phase to record intracellular signals; electrodes near neurites were able to record exocellular signals. Casanova et al. fabricated nanowire probes on a chip through a CMOS-compatible large-scale fabrication process based on conventional lithography tools (Casanova et al., 2018). It could achieve a high surface-to-volume ratio and have a large signal-to-noise ratio. Compared to planar electrodes, 3D-empowered electrodes led to improved signal quality with reduced background noise and increased signal amplitude.
Gu et al. designed an elaborate scalable field-effect transistor (FET) array that can switch between 2D and 3D as shown in Figure 6C (Gu et al., 2021). The process of transformation is accomplished by compressive buckling technique, a pre-strained elastomer substrate was used to buckle the 2D electrode into 3D. It was able to record the signals both from planar NoN and 3D tissues. Due to the fact that the distance between two electrodes was smaller than the length of cardiomyocytes, it could also record the intercellular signal conduction. They found that the conduction velocity of intracellular signals in cardiomyocytes is about five times the conduction velocity of intercellular signals. It is a very promising platform to study the signal conduction pathways and screen drugs.
Despite the limited damage of 3D electrodes on cells, some effects may still happen to cell electrophysiology. Mateus et al. proposed a mushroom-liked 3D electrode array to further improve electrophysiology recordings (Mateus et al., 2019). The mushrooms were wrapped in cells so that, on the one hand, signal quality was much higher than that of planar electrodes; on the other hand, only very limited noise was obtained from the environment since all the surface of the electrode was covered by cells. Despite their capability of measuring exocellular signals only, 3D electrodes were totally non-invasive and performed better than most planar electrodes. Topography effects on neural cells were demonstrated in this study as well. Besides, this chip is compatible with commercial MEA data acquisition systems, which shows excellent application value.
4.3 Microfluidic MEA Combined Models for Ex Vivo NoN Studies
Synapse plays an important role in neural communication since it can transmit the excitatory impulses produced by the neuron body to other neurons or effectors (Lines et al., 2017). MEAs can record most electrical activities from somas, but signals from axons are hard to measure due to the limited diameters of axons. In this case, the microfluidic device is a powerful tool to enhance the recording of signals from neurites (Narula et al., 2017). Shimba et al. modified microtunnels on top of MEAs to limit the neurites elongation (Shimba et al., 2019). Laminin and poly-L-ornithine (PLO), which can promote the growth of neurons, were coated on the surface of tunnels to induce the neurites. Each tunnel contains a microelectrode inside to detect the neural activity form neurites, as illustrated in Figure 6D. Compared to neurons on conventional MEAs, neurons on this advanced chip achieve longer neurites elongation. Recording of neural activities was significantly enhanced, as a result of significantly increased number of neurites per electrode. Importantly, neurites in the microtunnels can sustain for more than 1 year, demonstrating high potential of neural long-term culture and monitoring.
Neurodegenerative disease models can be potentially established on the MEA-microfluidic combined chip, as it can record signals from neurons and manipulate cells simultaneously. Interestingly, as only neurites can be induced to go through the microtunnels, the combined chip is a powerful tool to study communication between neurons and body cells. For example, scientists have classified several kinds of epilepsy, and one of them is called focal epilepsy, indicating that only part of the brain, rather than the whole area of it, suffers from seizures (Shorvon, 2011; Fisher et al., 2017). In order to study the connection between brain regions with and without seizure, Anssi et al. designed a platform that contains three compartments with microtunnels to connect them (Pelkonen et al., 2020). The microtunnels prevented liquid exchange, and therefore liquids were isolated within their own compartment. Human iPSC were cultured in all three compartments and induced to neurons to represent different brain regions. Neurons in three compartments were connected by axons through microtunnels. The convulsant and kainic acid were added to one compartment to mimic focal epilepsy, while the other two compartments were not treated with any drug. In general, the combination of MEAs with a microfluidic device empowers MEAs with excellent performance and amazing functions.
4.4 Brain Slides-On-Chip Models for Ex Vivo NoN Studies
Evidence has shown that hippocampal slices induced the onset of epilepsy spontaneously after several days of culture ex vivo (Dyhrfjeld-Johnsen et al., 2010). Jing et al. cultured the organotypic hippocampal slices in a petri dish to study the effects of the culture solution on spontaneous epileptogenesis (Liu et al., 2017b). A tungsten microelectrode was placed in a hippocampal slice to record extracellular field potential, and different culture solutions were added to the dish. Results showed that improvements in culture medium could prevent apoptosis of the brain to some extent, but seizures always happened. However, one electrode cannot detect the spikes in different parts of the hippocampal slice. Two years later, the author designed a microfluidic MEA-combined chip with simplified linear electrodes made by PDMS to better record the seizure of hippocampal slices (Liu et al., 2019). Compared to the traditional tissue slides on MEAs (Scott et al., 2013), this integrated chip has six culture wells on one plate to achieve high-throughput drug discovery and better control the environmental variants.
During the recording process on MEAs, thick brain slides (>500 µm) may face issues of lack of oxygen, nutrition, and waste removal. These issues prevent us from studying the chronic toxicity of drugs and the subsequent progression of epilepsy. To address them, Killian et al. designed a long-term perfusion and imaging microfluidic device on the basis of MEAs, as shown in Figure 7A (Killian et al., 2016). Perforated MEAs were used to record signals from brain slices and to perfuse the culture medium from the bottom. Meanwhile, culture solution was extracted, with a volume equal to perfusion, to keep the environment stable. Compared to culture in dish methods, the tissue slides survive much longer and facilitate long-term experiment conductance.
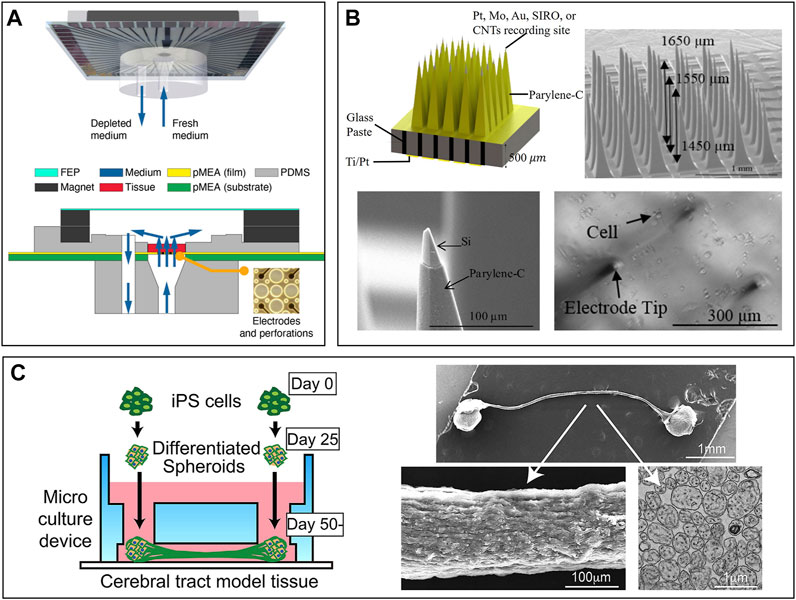
FIGURE 7. Brain slides and organoid-on-chips based models for ex vivo NoN studies: (A) Long-term perfusion, imaging microfluidic device based on MEA (Killian et al., 2016). (B) Micro-probe arrays used in vivo to record cell activity in the brain (Ghane Motlagh et al., 2016). (C) A microfluidic chip to build a cerebral tract model connecting two cortical regions. Organoids were first cultured in spheroid chambers and axons would grow through the microchannels (Kirihara et al., 2019).
4.5 Organoid-On-Chip Models for Ex Vivo NoN Studies
An organoid, a 3D cultured cell sphere, has characteristics between a mature brain and a planar NoN. Research demonstrated that neurons in 3D culture could have longer axons than in 2D culture, indicating that the function of neurons is limited in planar NoNs (Kong et al., 2021). In contrast to the real brain, which has blood vessels to provide nutrition for neurons, the organoid is made of neural cells only. Thus, one big issue in organoid culturing is the lack of oxygen in the center. Although some research shows that co-culture organoid with vasculature can promote the maturation and differentiation of neurons, the oxygen issue still remains (Isshiki et al., 2020). Thus, the organoid is much more complex than planar NoN due to its 3D structure but simpler than the human brain due to its limited functions. MEA was used to record the neural activities on the surface of organoid. The perfusion microfluidic devices previously introduced can also be used for culturing organoids and prolonging their survival time (Killian et al., 2016).
Although no technique is available yet to record the neural activities inside an organoid, 3D MEAs hold great potential in studying the organoid. Guihua et al. proposed a microneedle with five cellular-scale electrodes to detect glutamate concentration and cell activity in the brain simultaneously (Xiao et al., 2021). Five electrodes can be regarded as detecting one individual region of the brain because their positions are very close to each other. From the authors’ perspective, such a microneedle could also be inserted into the organoid to record both neural signals and glutamate concentration inside the brain. One new challenge would be how to insert the needle into the organoid without damaging cells. Micro-probe arrays are widely used in vivo to detect the cell activity of one particular region of the brain (Zhang et al., 2020). Motlagh et al. fabricated a high-density 3D pyramid-shaped MEA intended to record signals in our brain, as shown in Figure 7B (Ghane Motlagh et al., 2016a). In their follow-up study, polyethylene glycol, a biocompatible polymer, was coated on the surface of electrodes to improve the biocompatibility (Ghane-Motlagh et al., 2016b). If an organoid was cultured in these 3D MEAs and wrapped well around the needles, signals inside the 3D NoN could be tracked precisely and serve us better to understand more about the secrets of the human brain.
Organoids can also connect to each other through a robust fascicle consisting of axons (Kawada et al., 2017). It could be a platform for drug screening, even better than 2D NoN. Kirihara et al. proposed a microfluidic chip to build a cerebral tract model connecting two cortical regions by using hiPSC (Kirihara et al., 2019). The two spheroid chambers on the chip, each with a 2 mm diameter, were used for organoid culture, and they were connected by a microchannel. Axons could grow through the channel and connect two organoids, as shown in Figure 7C. Importantly, two organoids were electrically connected through the axon fascicle, which provides a platform for compound evaluation and drug screening.
5 Conclusion
In this study, we reviewed the latest designs in studying NoN and human brain models ex vivo. Multiple types of microsystems are discussed, including various microfluidic systems and MEAs. For better comparison, we summarized the representative nerve on chip models reported in prior art publications, including their advantage and limitations in Table 1. On the one hand, various neural models have been established on the basis of microfluidics owing to their parallel functions in controlling the microenvironment of cells and manipulating particles, including cells. On the other hand, various structures of MEAs have been designed for high-quality neural activity recordings to better understand neural interconnections inside the brain. Despite the fact that remarkable progress has been made in neuroscience in recent years, there are still many challenges that remain to be addressed. First, central hypoxia, the main reason for cell death in 3D cell culture, greatly limits research on organoids. In this respect, biomaterials that enable smooth nutrient transport and innovative cultivation are required. Second, co-culture of neural cells with other cells of interest is still hard to achieve due to different nutrient requirements per cell type. This greatly hinders the study of nerve–organ connections. Third, whether an artificial NoN can be trained to achieve desirable functions is still an unknown factor. Despite global efforts in recording the signals and stimulating the neurons of NoN on chip, none of these studies reported NoN systems obtained a simple real function. Some research studies have built biomimetic neural networks with computers, providing a new potential strategy to train the artificial NoN (Khoyratee et al., 2019).
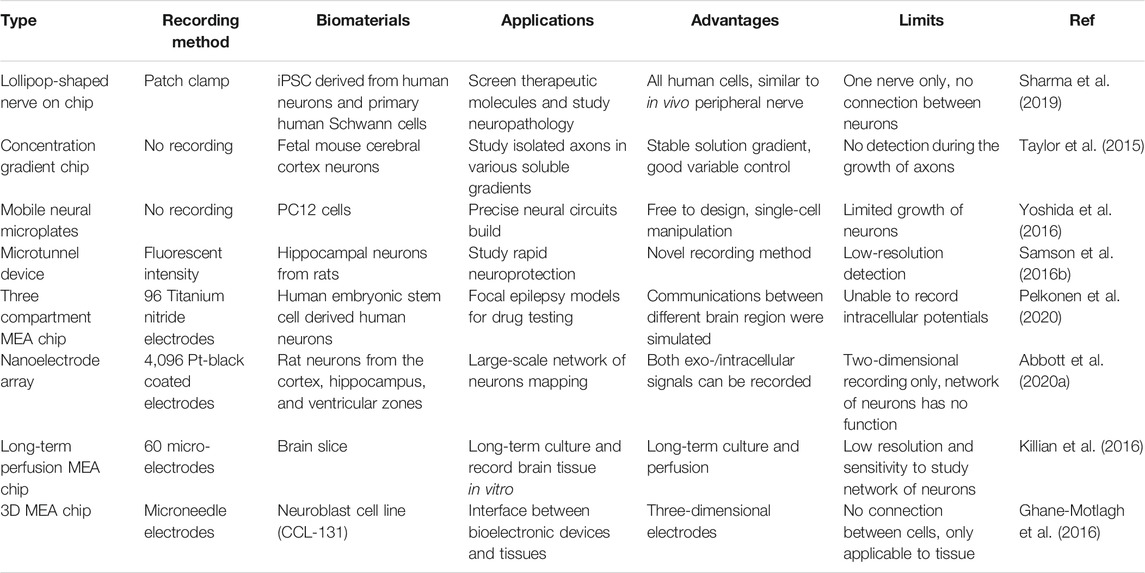
TABLE 1. Comparison of the performance of representative nerve on chip models reported in prior art publications.
Presently, there are many directions we can work to further improve the NoN models ex vivo. First, there is still room to further improve the regular and long-term cell culture techniques. For example, neurons differentiated from hiPSC do not perform as good as primary neurons in our body. Differentiation protocols or devices that better mimic the microenvironment in vivo are required urgently to increase the differentiation efficiency and accuracy, which can both greatly reduce the sacrifice of rats and improve ex vivo brain models. Although some studies have achieved the culture of neurons on chip up to 1 year, neural activity gradually decreases after two or 3 months, indicating that cells are senescent. Second, materials with multiple functions, including high transparency, low impedance, and permeability, are welcome. Better flexibility in fabrication allows us to design amazing MEAs and microfluidics more freely. If allowed, central hypoxia could be addressed by inserting blood vessel-like new materials into organoids. Third, a rapid, high-resolution, and 3D fabrication method is needed for better building the chips for organoid culture. Also, a rapid, high-resolution 3D bioprinter technique is much needed to print elaborate brain-shaped scaffolds. In this case, neuron cells can be embedded on the scaffolds and survive for up to 10 years. Eventually, we may build an advanced artificial brain ex vivo and train it via current or various chemical stimulations. “brain in a vat” may not be a dream anymore.
The study of the neural system will eventually promote the medical treatment of neural diseases and profound investigation of the human brain. With the rapid development in neuroscience, neural diseases may have a chance to be cured in the future. In related subjects of neuroscience, such as bio-inspired robots, artificial intelligence can have a qualitative leap.
Author Contributions
HZ wrote the original draft; HZ, SB, GR, and MS contributed to writing—review and editing; SB and MS supervised the study; and all authors have read and agreed to the published version of the manuscript.
Funding
This research was funded by Westlake University (Grant No. 10318A992001) and the National Natural Science Foundation of China (Grant No. 82104122).
Conflict of Interest
The authors declare that the research was conducted in the absence of any commercial or financial relationships that could be construed as a potential conflict of interest.
Publisher’s Note
All claims expressed in this article are solely those of the authors and do not necessarily represent those of their affiliated organizations, or those of the publisher, the editors, and the reviewers. Any product that may be evaluated in this article, or claim that may be made by its manufacturer, is not guaranteed or endorsed by the publisher.
References
Abbott, J., Ye, T., Krenek, K., Gertner, R. S., Ban, S., Kim, Y., et al. (2020). A Nanoelectrode Array for Obtaining Intracellular Recordings from Thousands of Connected Neurons. Nat. Biomed. Eng. 4, 232–241. doi:10.1038/s41551-019-0455-7
Abbott, J., Ye, T., Krenek, K., Qin, L., Kim, Y., Wu, W., et al. (2020). The Design of a CMOS Nanoelectrode Array with 4096 Current-Clamp/Voltage-Clamp Amplifiers for Intracellular Recording/Stimulation of Mammalian Neurons. IEEE J. Solid-state Circuits 55, 2567–2582. doi:10.1109/jssc.2020.3005816
Accardi, M. V., Pugsley, M. K., Forster, R., Troncy, E., Huang, H., and Authier, S. (2016). The Emerging Role of In Vitro Electrophysiological Methods in CNS Safety Pharmacology. J. Pharmacol. Toxicol. Methods 81, 47–59. doi:10.1016/j.vascn.2016.03.008
Achille, C., Parra‐Cabrera, C., Dochy, R., Ordutowski, H., Piovesan, A., Piron, P., et al. (2021). 3D Printing of Monolithic Capillarity‐Driven Microfluidic Devices for Diagnostics. Adv. Mater. 33, 2008712. doi:10.1002/adma.202008712
Ahn, S. I., Sei, Y. J., Park, H.-J., Kim, J., Ryu, Y., Choi, J. J., et al. (2020). Microengineered Human Blood-Brain Barrier Platform for Understanding Nanoparticle Transport Mechanisms. Nat. Commun. 11, 175. doi:10.1038/s41467-019-13896-7
Alcala-Orozco, C. R., Mutreja, I., Cui, X. L., Hooper, G. J., Lim, K. S., and Woodfield, T. B. F. (2022). Hybrid Biofabrication of 3D Osteoconductive Constructs Comprising Mg-Based Nanocomposites and Cell-Laden Bioinks for Bone Repair. Bone 154, 116198. doi:10.1016/j.bone.2021.116198
Amanda, J., Sahika, I., Ilke, U., Jonathan, R., Dion, K., Magnus, B., et al. (2016). Bioelectronic Neural Pixel: Chemical Stimulation and Electrical Sensing at the Same Site. PNAS 113, 9440. doi:10.1073/pnas.1604231113
Arrigoni, C., Gilardi, M., Bersini, S., Candrian, C., and Moretti, M. (2017). Bioprinting and Organ-On-Chip Applications towards Personalized Medicine for Bone Diseases. Stem Cel Rev Rep 13, 407–417. doi:10.1007/s12015-017-9741-5
Asif, A., Kim, K. H., Jabbar, F., Kim, S., and Choi, K. H. (2020). Real-time Sensors for Live Monitoring of Disease and Drug Analysis in Microfluidic Model of Proximal Tubule. Microfluid. Nanofluid. 24, 10. doi:10.1007/s10404-020-02347-1
Barthmes, M., Jose, M. D. F., Birkner, J. P., Brüggemann, A., Wahl-Schott, C., and Koçer, A. (2014). Studying Mechanosensitive Ion Channels with an Automated Patch Clamp. Eur. Biophys. J. 43, 97–104. doi:10.1007/s00249-014-0944-2
Bébarová, M. (2012). Advances in Patch Clamp Technique: towards Higher Quality and Quantity. gpb 31, 131–140. doi:10.4149/gpb_2012_016
Benam, K. H., Dauth, S., Hassell, B., Herland, A., Jain, A., Jang, K.-J., et al. (2015). “Engineered In Vitro Disease Models”, in Annual Review of Pathology: Mechanisms of Disease. Editors A K. Abbas, S J. Galli, and P M Howley, 10, 195–262. doi:10.1146/annurev-pathol-012414-040418
Benzina, O., Cloitre, T., Martin, M., Raoul, C., Gergely, C., and Scamps, F. (2014). Morphology and Intrinsic Excitability of Regenerating Sensory and Motor Neurons Grown on a Line Micropattern. Plos One 9, e110687. doi:10.1371/journal.pone.0110687
Berdondini, L., Imfeld, K., Maccione, A., Tedesco, M., Neukom, S., Koudelka-Hep, M., et al. (2009). Active Pixel Sensor Array for High Spatio-Temporal Resolution Electrophysiological Recordings from Single Cell to Large Scale Neuronal Networks. Lab. Chip 9, 2644–2651. doi:10.1039/b907394a
Brewer, J. R., Morrison, G., Dolan, M. E., and Fleming, G. F. (2016). Chemotherapy-induced Peripheral Neuropathy: Current Status and Progress. Gynecol. Oncol. 140, 176–183. doi:10.1016/j.ygyno.2015.11.011
Buzsáki, G. (2004). Large-scale Recording of Neuronal Ensembles. Nat. Neurosci. 7, 446–451. doi:10.1038/nn1233
Cao, H., Liu, T., and Chew, S. Y. (2009). The Application of Nanofibrous Scaffolds in Neural Tissue Engineering. Adv. Drug Deliv. Rev. 61, 1055–1064. doi:10.1016/j.addr.2009.07.009
Cao, J., and Köhler, J. M. (2015). Droplet-based Microfluidics for Microtoxicological Studies. Eng. Life Sci. 15, 306–317. doi:10.1002/elsc.201400074
Carlo, D. D., Wu, L. Y., and Lee, L. P. (2006). Dynamic Single Cell Culture Array. Lab. Chip 6, 1445–1449. doi:10.1039/b605937f
Casanova, A., Bettamin, L., Blatche, M.-C., Mathieu, F., Martin, H., Gonzalez-Dunia, D., et al. (2018). Nanowire Based Bioprobes for Electrical Monitoring of Electrogenic Cells. J. Phys. Condens. Matter 30, 464001. doi:10.1088/1361-648x/aae5aa
Chen, Y.-A., King, A. D., Shih, H.-C., Peng, C.-C., Wu, C.-Y., Liao, W.-H., et al. (2011). Generation of Oxygen Gradients in Microfluidic Devices for Cell Culture Using Spatially Confined Chemical Reactions. Lab. Chip 11, 3626–3633. doi:10.1039/c1lc20325h
Choi, J.-S., Lee, B.-J., Park, H.-Y., Song, J.-S., Shin, S.-C., Lee, J.-C., et al. (2015). Effects of Donor Age, Long-Term Passage Culture, and Cryopreservation on Tonsil-Derived Mesenchymal Stem Cells. Cell Physiol Biochem 36, 85–99. doi:10.1159/000374055
Choi, J. S., Lee, H. J., Rajaraman, S., and Kim, D.-H. (2021). Recent Advances in Three-Dimensional Microelectrode Array Technologies for In Vitro and In Vivo Cardiac and Neuronal Interfaces. Biosens. Bioelectron. 171, 112687. doi:10.1016/j.bios.2020.112687
Chu, H. K., Huan, Z., Mills, J. K., Yang, J., and Sun, D. (2015). Three-dimensional Cell Manipulation and Patterning Using Dielectrophoresis via a Multi-Layer Scaffold Structure. Lab. Chip 15, 920–930. doi:10.1039/c4lc01247j
Collins, D. J., Morahan, B., Garcia-Bustos, J., Doerig, C., Plebanski, M., and Neild, A. (2015). Two-dimensional Single-Cell Patterning with One Cell Per Well Driven by Surface Acoustic Waves. Nat. Commun. 6, 8686. doi:10.1038/ncomms9686
Desbiolles, B. X. E., de Coulon, E., Bertsch, A., Rohr, S., and Renaud, P. (2019). Intracellular Recording of Cardiomyocyte Action Potentials with Nanopatterned Volcano-Shaped Microelectrode Arrays. Nano Lett. 19, 6173–6181. doi:10.1021/acs.nanolett.9b02209
Di Carlo, D., Aghdam, N., and Lee, L. P. (2006). Single-cell Enzyme Concentrations, Kinetics, and Inhibition Analysis Using High-Density Hydrodynamic Cell Isolation Arrays. Anal. Chem. 78, 4925–4930. doi:10.1021/ac060541s
Dyhrfjeld-Johnsen, J., Berdichevsky, Y., Swiercz, W., Sabolek, H., and Staley, K. J. (2010). Interictal Spikes Precede Ictal Discharges in an Organotypic Hippocampal Slice Culture Model of Epileptogenesis. J. Clin. Neurophysiol. 27, 418–424. doi:10.1097/wnp.0b013e3181fe0709
Elalouf, A. (2021). Immune Response against the Biomaterials Used in 3D Bioprinting of Organs. Transpl. Immunol. 69, 101446. doi:10.1016/j.trim.2021.101446
Farasat, M., Chavoshi, S. M., Bakhshi, A., Valipour, A., and Badieirostami, M. (2021). A Dielectrophoresis-Based Microfluidic Chip for Trapping Circulating Tumor Cells Using a Porous Membrane. J. Micromech. Microeng. 32, 015008. doi:10.1088/1361-6439/ac3c89
Fiest, K. M., Sauro, K. M., Wiebe, S., Patten, S. B., Kwon, C.-S., Dykeman, J., et al. (2017). Prevalence and Incidence of Epilepsy. Neurology 88, 296–303. doi:10.1212/wnl.0000000000003509
Fisher, R. S., Cross, J. H., French, J. A., Higurashi, N., Hirsch, E., Jansen, F. E., et al. (2017). Operational Classification of Seizure Types by the International League against Epilepsy: Position Paper of the ILAE Commission for Classification and Terminology. Epilepsia 58, 522–530. doi:10.1111/epi.13670
Fricke, R., Zentis, P. D., Rajappa, L. T., Hofmann, B., Banzet, M., Offenhäusser, A., et al. (2011). Axon Guidance of Rat Cortical Neurons by Microcontact Printed Gradients. Biomaterials 32, 2070–2076. doi:10.1016/j.biomaterials.2010.11.036
Frimat, J.-P., Becker, M., Chiang, Y.-Y., Marggraf, U., Janasek, D., Hengstler, J. G., et al. (2011). A Microfluidic Array with Cellular Valving for Single Cell Co-culture. Lab. Chip 11, 231–237. doi:10.1039/c0lc00172d
Furukawa, Y., Shimada, A., Kato, K., Iwata, H., and Torimitsu, K. (2013). Monitoring Neural Stem Cell Differentiation Using PEDOT-PSS Based MEA. Biochim. Biophys. Acta (Bba) - Gen. Subjects 1830, 4329–4333. doi:10.1016/j.bbagen.2013.01.022
Gao, C., Lu, C. X., Jian, Z. A., Zhang, T. R., Chen, Z. J., Zhu, Q. G., et al. (2021). 3D Bioprinting for Fabricating Artificial Skin Tissue. Colloids Surf. B-Biointerfaces 208, 112041. doi:10.1016/j.colsurfb.2021.112041
Gao, J., Liao, C., Liu, S., Xia, T., and Jiang, G. (2021). Nanotechnology: New Opportunities for the Development of Patch‐clamps. J. Nanobiotechnol 19, 97. doi:10.1186/s12951-021-00841-4
Gao, K., Gao, F., Li, J., He, C., Liu, M., Zhu, Q., et al. (2021). Biomimetic Integrated Olfactory Sensory and Olfactory Bulb Systems In Vitro Based on a Chip. Biosens. Bioelectron. 171, 112739. doi:10.1016/j.bios.2020.112739
George, E. L., Truesdell, S. L., York, S. L., and Saunders, M. M. (2018). Lab-on-a-chip Platforms for Quantification of Multicellular Interactions in Bone Remodeling. Exp. Cel Res. 365, 106–118. doi:10.1016/j.yexcr.2018.02.027
Geraili, A., Jafari, P., Hassani, M. S., Araghi, B. H., Mohammadi, M. H., Ghafari, A. M., et al. (2018). Controlling Differentiation of Stem Cells for Developing Personalized Organ-On-Chip Platforms. Adv. Healthc. Mater. 7, 1700426 doi:10.1002/adhm.201700426
Ghane Motlagh, B., Choueib, M., Hajhosseini Mesgar, A., Hasanuzzaman, M., and Sawan, M. (2016a). Direct Growth of Carbon Nanotubes on New High-Density 3D Pyramid-Shaped Microelectrode Arrays for Brain-Machine Interfaces. Micromachines 7, 163. doi:10.3390/mi7090163
Ghane-Motlagh, B., Javanbakht, T., Shoghi, F., Wilkinson, K. J., Martel, R., and Sawan, M. (2016). Physicochemical Properties of Peptide-Coated Microelectrode Arrays and Their In Vitro Effects on Neuroblast Cells. Mater. Sci. Eng. C 68, 642–650. doi:10.1016/j.msec.2016.06.045
Gilbert-Honick, J., Iyer, S. R., Somers, S. M., Takasuka, H., Lovering, R. M., Wagner, K. R., et al. (2020). Engineering 3D Skeletal Muscle Primed for Neuromuscular Regeneration Following Volumetric Muscle Loss. Biomaterials 255, 120154. doi:10.1016/j.biomaterials.2020.120154
Giugiulan, R., Malaescu, I., Lungu, M., and Strambeanu, N. (2014). The Clausius Mossotti Factor in Low Frequency Field of the Powders Resulted from Wastes Combustion. Rom. J. Phys. 59, 862–872.
Golyala, A., and Kwan, P. (2017). Drug Development for Refractory Epilepsy: The Past 25 Years and beyond. Seizure 44, 147–156. doi:10.1016/j.seizure.2016.11.022
Goshi, N., Morgan, R. K., Lein, P. J., and Seker, E. (2020). A Primary Neural Cell Culture Model to Study Neuron, Astrocyte, and Microglia Interactions in Neuroinflammation. J. Neuroinflammation 17, 155. doi:10.1186/s12974-020-01819-z
Grainger, A. I., King, M. C., Nagel, D. A., Parri, H. R., Coleman, M. D., and Hill, E. J. (2018). In Vitro Models for Seizure-Liability Testing Using Induced Pluripotent Stem Cells. Front. Neurosci. 12, 590. doi:10.3389/fnins.2018.00590
Green, N. G., Ramos, A., González, A., Morgan, H., and Castellanos, A. (2000). Fluid Flow Induced by Nonuniform Ac Electric fields in Electrolytes on Microelectrodes. I. Experimental Measurements. Phys. Rev. E 61, 4011–4018. doi:10.1103/physreve.61.4011
Gu, Y., Wang, C., Kim, N., Zhang, J., Wang, T. M., Stowe, J., et al. (2021). Three-dimensional Transistor Arrays for Intra- and Inter-cellular Recording. Nat. Nanotechnol 23, 1040. doi:10.1038/s41565-021-01040-w
Hai, A., Shappir, J., and Spira, M. E. (2010). In-cell Recordings by Extracellular Microelectrodes. Nat. Methods 7, 200–202. doi:10.1038/nmeth.1420
Hayman, M. W., Smith, K. H., Cameron, N. R., and Przyborski, S. A. (2005). Growth of Human Stem Cell-Derived Neurons on Solid Three-Dimensional Polymers. J. Biochem. Biophysical Methods 62, 231–240. doi:10.1016/j.jbbm.2004.12.001
Holloway, P. M., Willaime‐Morawek, S., Siow, R., Barber, M., Owens, R. M., Sharma, A. D., et al. (2021). Advances in Microfluidic In Vitro Systems for Neurological Disease Modeling. J. Neurosci. Res. 99, 1276–1307. doi:10.1002/jnr.24794
Hu, Q. P., Liu, X., Liu, H. F., Yang, L., Yuan, X., Chen, Y. W., et al. (2021). 3D Printed Porous Microgel for Lung Cancer Cells Culture In Vitro. Mater. Des. 210, 110079. doi:10.1016/j.matdes.2021.110079
Hwang, D. G., Jo, Y., Kim, M., Yong, U., Cho, S., Choi, Y. M., et al. (2022). A 3D Bioprinted Hybrid Encapsulation System for Delivery of Human Pluripotent Stem Cell-Derived Pancreatic Islet-like Aggregates. Biofabrication 14, 014101. doi:10.1088/1758-5090/ac23ac
Isshiki, Y., Kaneko, T., Tamada, A., Muguruma, K., Yokokaw, R., and Ieee, (2020). Co-culture of a Brain Organoid Derived from Human IPSCS and Vasculature on a Chip. 33rd IEEE International Conference on Micro Electro Mechanical Systems (MEMS), January 18–22, 2020. Vancouver: CANADA, 1024–1027. doi:10.1109/mems46641.2020.9056422
Jamee, R., Araf, Y., Naser, I. B., and Promon, S. K. (2021). The Promising Rise of Bioprinting in Revolutionalizing Medical Science: Advances and Possibilities. Regenerative Ther. 18, 133–145. doi:10.1016/j.reth.2021.05.006
Jean-Michel, P., Berangere, D., Laure, S., Maeva, V., Paul, G., Sebastien, M., et al. (2011). Axon Diodes for the Reconstruction of Oriented Neuronal Networks in Microfluidic chambers. Lab. A. Chip 11, 3663–3673.
Johnstone, A. F. M., Gross, G. W., Weiss, D. G., Schroeder, O. H.-U., Gramowski, A., and Shafer, T. J. (2010). Microelectrode Arrays: a Physiologically Based Neurotoxicity Testing Platform for the 21st century. Neurotoxicology 31, 331–350. doi:10.1016/j.neuro.2010.04.001
Kajtez, J., Buchmann, S., Vasudevan, S., Birtele, M., Rocchetti, S., Pless, C. J., et al. (2020). 3D‐Printed Soft Lithography for Complex Compartmentalized Microfluidic Neural Devices. Adv. Sci. 7, 2001150. doi:10.1002/advs.202001150
Kaneko, N., Ullman, H., Ali, F., Berg, P., Ooi, Y. C., Tateshima, S., et al. (2020). In Vitro Modeling of Human Brain Arteriovenous Malformation for Endovascular Simulation and Flow Analysis. World Neurosurg. 141, e873–1879. doi:10.1016/j.wneu.2020.06.084
Kawada, J., Kaneda, S., Kirihara, T., Maroof, A., Levi, T., Eggan, K., et al. (2017). Generation of a Motor Nerve Organoid with Human Stem Cell-Derived Neurons. Stem Cel Rep. 9, 1441–1449. doi:10.1016/j.stemcr.2017.09.021
Kearney, K., Voss, K., Shaffer, J., Brandon Borders, R., Snyder, L., and Roche, B. (2018). Applications and Direct Comparisons of EEG Collection Methodologies in Nonclinical Studies. J. Pharmacol. Toxicol. Methods 93, 137. doi:10.1016/j.vascn.2018.01.457
Khoshakhlagh, P., Sivakumar, A., Pace, L. A., Sazer, D. W., and Moore, M. J. (2018). Methods for Fabrication and Evaluation of a 3D Microengineered Model of Myelinated Peripheral Nerve. J. Neural Eng. 15, 064001. doi:10.1088/1741-2552/aae129
Khoyratee, F., Grassia, F., Saïghi, S., and Levi, T. (2019). Optimized Real-Time Biomimetic Neural Network on FPGA for Bio-Hybridization. Front. Neurosci. 13, 377. doi:10.3389/fnins.2019.00377
Killian, N. J., Vernekar, V. N., Potter, S. M., and Vukasinovic, J. (2016). A Device for Long-Term Perfusion, Imaging, and Electrical Interfacing of Brain Tissue In Vitro. Front. Neurosci. 10, 135. doi:10.3389/fnins.2016.00135
Kim, H., and Kim, J. (2014). A Microfluidic-Based Dynamic Microarray System with Single-Layer Pneumatic Valves for Immobilization and Selective Retrieval of Single Microbeads. Microfluid. Nanofluid. 16, 623–633. doi:10.1007/s10404-013-1267-9
Kim, J., Lee, H., Selimović, Š., Gauvin, R., and Bae, H. (2015). Organ-On-A-Chip: Development and Clinical Prospects toward Toxicity Assessment with an Emphasis on Bone Marrow. Drug Saf. 38, 409–418. doi:10.1007/s40264-015-0284-x
Kim, M. H., Chalisserry, E. P., Mondal, S., Oh, J., and Nam, S. Y. (2021). Silicon-substituted Hydroxyapatite Reinforced 3D Printed Gelatin Membrane for Guided Bone Regeneration. Mater. Lett. 304, 130670. doi:10.1016/j.matlet.2021.130670
Kirihara, T., Luo, Z., Chow, S. Y. A., Misawa, R., Kawada, J., Shibata, S., et al. (2019). A Human Induced Pluripotent Stem Cell-Derived Tissue Model of a Cerebral Tract Connecting Two Cortical Regions. iScience 14, 301–311. doi:10.1016/j.isci.2019.03.012
Koerbitzer, B., Krauss, P., Nick, C., Yadav, S., Schneider, J. J., and Thielemann, C. (2016). Graphene Electrodes for Stimulation of Neuronal Cells. 2d Mater. 3, 024004. doi:10.1088/2053-1583/3/2/024004
Kofron, C. M., and Mende, U. (2017). In Vitromodels of the Cardiac Microenvironment to Study Myocyte and Non-myocyte Crosstalk: Bioinspired Approaches beyond the Polystyrene Dish. J. Physiol. 595, 3891–3905. doi:10.1113/jp273100
Kong, X., Chen, T., and Huang, Y. (2021). Analysis of Neurite Length of Hippocampal Neurons Cultured into 3D Artificial Network Patterned Microfluidic Chips. Int. J. Neurosci. 131, 40–43. doi:10.1080/00207454.2020.1733553
Kou, S., Cheng, D., Sun, F., and Hsing, I.-M. (2016). Microfluidics and Microbial Engineering. Lab. Chip 16, 432–446. doi:10.1039/c5lc01039j
Koutsouras, D. A., Hama, A., Pas, J., Gkoupidenis, P., Hivert, B., Faivre-Sarrailh, C., et al. (2017). PEDOT:PSS Microelectrode Arrays for Hippocampal Cell Culture Electrophysiological Recordings. MRS Commun. 7, 259–265. doi:10.1557/mrc.2017.34
Kramer, L., Nguyen, H. T., Jacobs, E., McCoy, L., Curley, J. L., Sharma, A. D., et al. (2020). Modeling Chemotherapy-Induced Peripheral Neuropathy Using a Nerve-On-A-Chip Microphysiological System. ALTEX 37, 350–364. doi:10.14573/altex.2001181
Kshirsagar, P., Dickreuter, S., Mierzejewski, M., Burkhardt, C. J., Chassé, T., Fleischer, M., et al. (2019). Transparent Graphene/PEDOT:PSS Microelectrodes for Electro- and Optophysiology. Adv. Mater. Technol. 4, 1800318. doi:10.1002/admt.201800318
Lassus, B., Naudé, J., Faure, P., Guedin, D., Von Boxberg, Y., Mannoury la Cour, C., et al. (2018). Glutamatergic and Dopaminergic Modulation of Cortico-Striatal Circuits Probed by Dynamic Calcium Imaging of Networks Reconstructed in Microfluidic Chips. Sci. Rep. 8, 17461. doi:10.1038/s41598-018-35802-9
Laurent, M., Ricard, J.-L., Bahain, J.-J., Voinchet, P., and Rousseau, L. (2009). Active Pixel Sensor Array for High Spatio-Temporal Resolution Electrophysiological Recordings from Single Cell to Large Scale Neuronal Networks. Lab. A Chip 9, 2644. doi:10.1039/b907394a
Levi, T., and Fujii, T. (2016). Microfluidic Neurons, a New Way in Neuromorphic Engineering. Micromachines 7, 146. doi:10.3390/mi7080146
Liang, Y., Offenhausser, A., Ingebrandt, S., and Mayer, D. (2021). PEDOT:PSS-Based Bioelectronic Devices for Recording and Modulation of Electrophysiological and Biochemical Cell Signals. Adv. Healthc. Mater. 10, e2100061. doi:10.1002/adhm.202100061
Lines, J., Covelo, A., Gómez, R., Liu, L., and Araque, A. (2017). Synapse-Specific Regulation Revealed at Single Synapses Is Concealed when Recording Multiple Synapses. Front. Cel. Neurosci. 11, 367. doi:10.3389/fncel.2017.00367
Liu, H., and Wang, X. (2016). Correlation of Iron Deposition and Change of Gliocyte Metabolism in the Basal Ganglia Region Evaluated Using Magnetic Resonance Imaging Techniques: an In Vivo Study. aoms 1, 163–171. doi:10.5114/aoms.2016.57593
Liu, J., Saponjian, Y., Mahoney, M. M., Staley, K. J., and Berdichevsky, Y. (2017). Epileptogenesis in Organotypic Hippocampal Cultures Has Limited Dependence on Culture Medium Composition. Plos One 12, e0172677. doi:10.1371/journal.pone.0172677
Liu, J., Sternberg, A. R., Ghiasvand, S., and Berdichevsky, Y. (2019). Epilepsy-on-a-Chip System for Antiepileptic Drug Discovery. IEEE Trans. Biomed. Eng. 66, 1231–1241. doi:10.1109/tbme.2018.2871415
Liu, R., Chen, R., Elthakeb, A. T., Lee, S. H., Hinckley, S., Khraiche, M. L., et al. (2017). High Density Individually Addressable Nanowire Arrays Record Intracellular Activity from Primary Rodent and Human Stem Cell Derived Neurons. Nano Lett. 17, 2757–2764. doi:10.1021/acs.nanolett.6b04752
Liu, Z., Zhang, H., Zhan, Z., Nan, H., Huang, N., Xu, T., et al. (2020). Mild Formation of Core-Shell Hydrogel Microcapsules for Cell Encapsulation. Biofabrication 13, 025002. doi:10.1088/1758-5090/abd076
Lott, G. K., and Hoy, R. R. (2008). Large-Scale, High-Resolution Data Acquisition System for Extracellular Recording of Electrophysiological Activity. IEEE Trans. Biomed. Eng. 55, 1728–1732. doi:10.1109/tbme.2008.919139
Maccione, A., Garofalo, M., Nieus, T., Tedesco, M., Berdondini, L., and Martinoia, S. (2012). Multiscale Functional Connectivity Estimation on Low-Density Neuronal Cultures Recorded by High-Density CMOS Micro Electrode Arrays. J. Neurosci. Methods 207, 161–171. doi:10.1016/j.jneumeth.2012.04.002
Mahoney, M. J., and Anseth, K. S. (2006). Three-dimensional Growth and Function of Neural Tissue in Degradable Polyethylene Glycol Hydrogels. Biomaterials 27, 2265–2274. doi:10.1016/j.biomaterials.2005.11.007
Maschmeyer, I., Lorenz, A. K., Schimek, K., Hasenberg, T., Ramme, A. P., Hübner, J., et al. (2015). A Four-Organ-Chip for Interconnected Long-Term Co-culture of Human Intestine, Liver, Skin and Kidney Equivalents. Lab. Chip 15, 2688–2699. doi:10.1039/c5lc00392j
Mateus, J. C., Lopes, C. D. F., Cerquido, M., Leitão, L., Leitão, D., Cardoso, S., et al. (2019). Improved In Vitro Electrophysiology Using 3D-Structured Microelectrode Arrays with a Micro-mushrooms Islets Architecture Capable of Promoting Topotaxis. J. Neural Eng. 16, 036012. doi:10.1088/1741-2552/ab0b86
McDonald, M., Monaco, A., Vahidpour, F., Haenen, K., Giugliano, M., and Nesladek, M. (2017). Diamond Microelectrode Arrays for In Vitro Neuronal Recordings. MRS Commun. 7, 683–690. doi:10.1557/mrc.2017.62
Mechler, F., Victor, J. D., Ohiorhenuan, I., Schmid, A. M., and Hu, Q. (2011). Three-dimensional Localization of Neurons in Cortical Tetrode Recordings. J. Neurophysiol. 106, 828–848. doi:10.1152/jn.00515.2010
Merz, M., and Fromherz, P. (2002). Polyester Microstructures for Topographical Control of Outgrowth and Synapse Formation of Snail Neurons. Adv. Mater. 14, 141–144. doi:10.1002/1521-4095(20020116)14:2<141:aid-adma141>3.0.co;2-r
Miled, A., Auclair, B., Srasra, A., and Sawan, M. (2015). Reconfigurable Prototyping Microfluidic Platform for DEP Manipulation and Capacitive Sensing. IEEE Trans. Biomed. Circuits Syst. 9, 155–165. doi:10.1109/tbcas.2015.2414452
Miller, D. W., Cookson, M. R., and Dickson, D. W. (2004). Glial Cell Inclusions and the Pathogenesis of Neurodegenerative Diseases. Neuron Glia Biol. 1, 13–21. doi:10.1017/s1740925x04000043
Morimoto, Y., Kato-Negishi, M., Onoe, H., and Takeuchi, S. (2013). Three-dimensional Neuron-Muscle Constructs with Neuromuscular Junctions. Biomaterials 34, 9413–9419. doi:10.1016/j.biomaterials.2013.08.062
Mun, S. J., Hong, Y.-H., Ahn, H.-S., Ryu, J.-S., Chung, K.-S., and Son, M. J. (2020). Long-Term Expansion of Functional Human Pluripotent Stem Cell-Derived Hepatic Organoids. Ijsc 13, 279–286. doi:10.15283/ijsc20060
Murphy, A. R., Laslett, A., O'Brien, C. M., and Cameron, N. R. (2017). Scaffolds for 3D In Vitro Culture of Neural Lineage Cells. Acta Biomater. 54, 1–20. doi:10.1016/j.actbio.2017.02.046
Nabavi, S., Fox, R., Proulx, C. D., Lin, J. Y., Tsien, R. Y., and Malinow, R. (2014). Engineering a Memory with LTD and LTP. Nature 511, 348–352. doi:10.1038/nature13294
Nam, S., Cho, I., Heo, J., Lim, G., Bazant, M. Z., Moon, D. J., et al. (2015). Experimental Verification of Overlimiting Current by Surface Conduction and Electro-Osmotic Flow in Microchannels. Phys. Rev. Lett. 114, 114501. doi:10.1103/physrevlett.114.114501
Narula, U., Ruiz, A., McQuaide, M., DeMarse, T. B., Wheeler, B. C., and Brewer, G. J. (2017). Narrow Microtunnel Technology for the Isolation and Precise Identification of Axonal Communication Among Distinct Hippocampal Subregion Networks. Plos One 12, e0176868. doi:10.1371/journal.pone.0176868
Newberry, K., Wang, S., Hoque, N., Kiss, L., Ahlijanian, M. K., Herrington, J., et al. (2016). Development of a Spontaneously Active Dorsal Root Ganglia Assay Using Multiwell Multielectrode Arrays. J. Neurophysiol. 115, 3217–3228. doi:10.1152/jn.01122.2015
O'Collins, V. E., Macleod, M. R., Donnan, G. A., Horky, L. L., van der Worp, B. H., and Howells, D. W. (2006). 1,026 Experimental Treatments in Acute Stroke. Ann. Neurol. 59, 467–477. doi:10.1002/ana.20741
Okamoto, M., Dan, H., Sakamoto, K., Takeo, K., Shimizu, K., Kohno, S., et al. (2004). Three-dimensional Probabilistic Anatomical Cranio-Cerebral Correlation via the International 10-20 System Oriented for Transcranial Functional Brain Mapping. Neuroimage 21, 99–111. doi:10.1016/j.neuroimage.2003.08.026
Onoe, H., and Takeuchi, S. (2008). Microfabricated mobile Microplates for Handling Single Adherent Cells. J. Micromech. Microeng. 18, 095003. doi:10.1088/0960-1317/18/9/095003
Osaki, T., Shin, Y., Sivathanu, V., Campisi, M., and Kamm, R. D. (2018). In Vitro Microfluidic Models for Neurodegenerative Disorders. Adv. Healthc. Mater. 7, 1700489. doi:10.1002/adhm.201700489
Oyama, H., Takahashi, K., Tanaka, Y., Takemoto, H., and Haga, H. (2018). Long-term Culture of Human iPS Cell-Derived Telencephalic Neuron Aggregates on Collagen Gel. Cell Struct. Funct. 43, 85–94. doi:10.1247/csf.18002
Pang, L., Ding, J., Liu, X.-X., Yuan, H., Ge, Y., Fan, J., et al. (2020). Microstructure-based Techniques for Single-Cell Manipulation and Analysis. Trac Trends Anal. Chem. 129, 115940. doi:10.1016/j.trac.2020.115940
Pek, Y. S., Wan, A. C. A., and Ying, J. Y. (2010). The Effect of Matrix Stiffness on Mesenchymal Stem Cell Differentiation in a 3D Thixotropic Gel. Biomaterials 31, 385–391. doi:10.1016/j.biomaterials.2009.09.057
Pelkonen, A., Mzezewa, R., Sukki, L., Ryynänen, T., Kreutzer, J., Hyvärinen, T., et al. (2020). A Modular Brain-On-A-Chip for Modelling Epileptic Seizures with Functionally Connected Human Neuronal Networks. Biosens. Bioelectron. 168, 112553. doi:10.1016/j.bios.2020.112553
Pitkanen, A., Lukasiuk, K., Dudek, F. E., and Staley, K. J. (2015). Epileptogenesis. Cold Spring Harb Perspect. Med. 5, 1–18. doi:10.1101/cshperspect.a022822
PotterPotter, S. M. M., and DeMarse, T. B. (2001). A New Approach to Neural Cell Culture for Long-Term Studies. J. Neurosci. Methods 110, 17–24. doi:10.1016/s0165-0270(01)00412-5
Ramos-Argüelles, G. M. F., Egozcue, S., Pabón, R. M., and Alonso, M. T. (2009). Basic Techniques of Electroencephalography: Principles and Clinical Applications. TÉCNICAS BÁSICAS DE ELECTROENCEFALOGRAFÍA 32, 69–82. doi:10.23938/ASSN.0148
Rastegar, S., Stadlbauer, J., Pandhi, T., Karriem, L., Fujimoto, K., Kramer, K., et al. (2019). Measurement of Signal‐to‐Noise Ratio in Graphene‐based Passive Microelectrode Arrays. Electroanalysis 31, 991–1001. doi:10.1002/elan.201800745
Regehr, W. G., Pine, J., Cohan, C. S., Mischke, M. D., and Tank, D. W. (1989). Sealing Cultured Invertebrate Neurons to Embedded Dish Electrodes Facilitates Long-Term Stimulation and Recording. J. Neurosci. Methods 30, 91–106. doi:10.1016/0165-0270(89)90055-1
Ren, Y., Liu, J., Liu, W., Lang, Q., Tao, Y., Hu, Q., et al. (2016). Scaled Particle Focusing in a Microfluidic Device with Asymmetric Electrodes Utilizing Induced-Charge Electroosmosis. Lab. Chip 16, 2803–2812. doi:10.1039/c6lc00485g
Ren, Y., Liu, W., Jia, Y., Tao, Y., Shao, J., Ding, Y., et al. (2015). Induced-charge Electroosmotic Trapping of Particles. Lab. Chip 15, 2181–2191. doi:10.1039/c5lc00058k
Renault, R., Sukenik, N., Descroix, S., Malaquin, L., Viovy, J. L., Peyrin, J. M., et al. (2015). Combining Microfluidics, Optogenetics and Calcium Imaging to Study Neuronal Communication In Vitro. Plos One 10, e0120680. doi:10.1371/journal.pone.0120680
Renault, R., Durand, J.-B., Viovy, J.-L., and Villard, C. (2016). Asymmetric Axonal Edge Guidance: a New Paradigm for Building Oriented Neuronal Networks. Lab. Chip 16, 2188–2191. doi:10.1039/c6lc00479b
Robinson, J. T., Jorgolli, M., Shalek, A. K., Yoon, M.-H., Gertner, R. S., and Park, H. (2012). Vertical Nanowire Electrode Arrays as a Scalable Platform for Intracellular Interfacing to Neuronal Circuits. Nat. Nanotech 7, 180–184. doi:10.1038/nnano.2011.249
Roth, S., Bisbal, M., Brocard, J., Bugnicourt, G., Saoudi, Y., Andrieux, A., et al. (2012). How Morphological Constraints Affect Axonal Polarity in Mouse Neurons. Plos One 7, e33623. doi:10.1371/journal.pone.0033623
Saito, M. L. (2019). NanoTouch: Intracellular Recording Using Transmembrane Conductive Nanoparticles. J. Neurophysiol. 122, 2016–2026. doi:10.1152/jn.00359.2019
Samadian, H., Jafari, S., Sepand, M. R., Alaei, L., Malvajerd, S. S., Jaymand, M., et al. (2021). 3D Bioprinting Technology to Mimic the Tumor Microenvironment: Tumor-On-A-Chip Concept. Mater. Today Adv. 12, 100160. doi:10.1016/j.mtadv.2021.100160
Samson, A. J., Robertson, G., Zagnoni, M., and Connolly, C. N. (2016). Neuronal Networks Provide Rapid Neuroprotection against Spreading Toxicity. Sci. Rep. 6, 33746. doi:10.1038/srep33746
Scott, A., Weir, K., Easton, C., Huynh, W., Moody, W. J., and Folch, A. (2013). A Microfluidic Microelectrode Array for Simultaneous Electrophysiology, Chemical Stimulation, and Imaging of Brain Slices. Lab. Chip 13, 527–535. doi:10.1039/c2lc40826k
Sen, M., Ino, K., Ramon-Azcon, J., Shiku, H., and Matsue, T. (2013). Cell Pairing Using a Dielectrophoresis-Based Device with Interdigitated Array Electrodes. Lab. Chip 13, 3650–3652. doi:10.1039/c3lc50561h
Seok, J., Warren, H. S., Cuenca, A. G., Mindrinos, M. N., Baker, H. V., Xu, W., et al. (2013). Genomic Responses in Mouse Models Poorly Mimic Human Inflammatory Diseases. Proc. Natl. Acad. Sci. USA 110, 3507–3512. doi:10.1073/pnas.1222878110
Shaik, F. A., Ikeuchi, Y., Cathcart, G., Ihida, S., Toshiyoshi, H., and Tixier-Mita, A. (2017). Extracellular Neural Stimulation and Recording with a Thin-Film-Transistor (Tft) Array Device. 19th International Conference on Solid-State Sensors, Actuators and Microsystems (Transducers), Jun 18–22, 2017. Kaohsiung, TAIWAN, 206–209. doi:10.1109/transducers.2017.7994024
Sharma, A. D., McCoy, L., Jacobs, E., Willey, H., Behn, J. Q., Nguyen, H., et al. (2019). Engineering a 3D Functional Human Peripheral Nerve In Vitro Using the Nerve-On-A-Chip Platform. Sci. Rep. 9, 8921. doi:10.1038/s41598-019-45407-5
Sharma, S., Srisa-Art, M., Scott, S., Asthana, A., and Cass, A. (2013). Droplet-Based Microfluidics. Methods Mol. Biol. 949, 207–230. doi:10.1007/978-1-62703-134-9_15
Sheyn, D., Cohn-Yakubovich, D., Ben-David, S., De Mel, S., Chan, V., Hinojosa, C., et al. (2019). Bone-chip System to Monitor Osteogenic Differentiation Using Optical Imaging. Microfluid. Nanofluid. 23, 99. doi:10.1007/s10404-019-2261-7
Shimba, K., Sakai, K., Iida, S., Kotani, K., and Jimbo, Y. (2019). Long-Term Developmental Process of the Human Cortex Revealed In Vitro by Axon-Targeted Recording Using a Microtunnel-Augmented Microelectrode Array. IEEE Trans. Biomed. Eng. 66, 2538–2545. doi:10.1109/tbme.2019.2891310
Shorvon, S. D. (2011). The Etiologic Classification of Epilepsy. Epilepsia 52, 1052–1057. doi:10.1111/j.1528-1167.2011.03041.x
Stagg, N. J., Shen, B.-Q., Brunstein, F., Li, C., Kamath, A. V., Zhong, F., et al. (2016). Peripheral Neuropathy with Microtubule Inhibitor Containing Antibody Drug Conjugates: Challenges and Perspectives in Translatability from Nonclinical Toxicology Studies to the Clinic. Regul. Toxicol. Pharmacol. 82, 1–13. doi:10.1016/j.yrtph.2016.10.012
Su, Y., Bian, S., and Sawan, M. (2020). Real-time In Vivo Detection Techniques for Neurotransmitters: a Review. Analyst 145, 6193–6210. doi:10.1039/d0an01175d
Susloparova, A., Halliez, S., Begard, S., Colin, M., Buée, L., Pecqueur, S., et al. (2021). Low Impedance and Highly Transparent Microelectrode Arrays (MEA) for In Vitro Neuron Electrical Activity Probing. Sensors Actuators B: Chem. 327, 128895. doi:10.1016/j.snb.2020.128895
Takahashi, K., Tanabe, K., Ohnuki, M., Narita, M., Ichisaka, T., Tomoda, K., et al. (2007). Induction of Pluripotent Stem Cells from Adult Human Fibroblasts by Defined Factors. Cell 131, 861–872. doi:10.1016/j.cell.2007.11.019
Takahashi, K., and Yamanaka, S. (2006). Induction of Pluripotent Stem Cells from Mouse Embryonic and Adult Fibroblast Cultures by Defined Factors. Cell 126, 663–676. doi:10.1016/j.cell.2006.07.024
Tan, S. J., Yobas, L., Lee, G. Y. H., Ong, C. N., and Lim, C. T. (2009). Microdevice for the Isolation and Enumeration of Cancer Cells from Blood. Biomed. Microdevices 11, 883–892. doi:10.1007/s10544-009-9305-9
Tang, F., Hartz, A. M. S., and Bauer, B. (2017). Drug-Resistant Epilepsy: Multiple Hypotheses, Few Answers. Front. Neurol. 8, 301. doi:10.3389/fneur.2017.00301
Taylor, A. M., Menon, S., and Gupton, S. L. (2015). Passive Microfluidic Chamber for Long-Term Imaging of Axon Guidance in Response to Soluble Gradients. Lab. Chip 15, 2781–2789. doi:10.1039/c5lc00503e
Thunemann, M., Lu, Y., Liu, X., Kılıç, K., Desjardins, M., Vandenberghe, M., et al. (2018). Deep 2-photon Imaging and Artifact-free Optogenetics through Transparent Graphene Microelectrode Arrays. Nat. Commun. 9, 2035. doi:10.1038/s41467-018-04457-5
Tixier‐Mita, A., Ihida, S., Blanchard, D., Shinohara, M., Eiler, A. C., Cathcart, G. A., et al. (2019). 2D Dielectrophoresis Using an Active Matrix Array Made by Thin‐film‐transistor Technology. IEEJ Trans. Electr. Electron. Eng. 14, 1280–1288.
Truesdell, S. L., George, E. L., and Saunders, M. M. (2020). Cellular Considerations for Optimizing Bone Cell Culture and Remodeling in a Lab-On-A-Chip Platform. Biotechniques 68, 263–269. doi:10.2144/btn-2019-0115
Tsai, D., Sawyer, D., Bradd, A., Yuste, R., and Shepard, K. L. (2017). A Very Large-Scale Microelectrode Array for Cellular-Resolution Electrophysiology. Nat. Commun. 8, 1802. doi:10.1038/s41467-017-02009-x
VanDersarl, J. J., Xu, A. M., and Melosh, N. A. (2012). Nanostraws for Direct Fluidic Intracellular Access. Nano Lett. 12, 3881–3886. doi:10.1021/nl204051v
Wang, X., Gu, Y., Xiong, Z., Cui, Z., and Zhang, T. (2014). Silk-molded Flexible, Ultrasensitive, and Highly Stable Electronic Skin for Monitoring Human Physiological Signals. Adv. Mater. 26, 1336–1342. doi:10.1002/adma.201304248
Wijdenes, P., Ali, H., Armstrong, R., Zaidi, W., Dalton, C., and Syed, N. I. (2016). A Novel Bio-Mimicking, Planar Nano-Edge Microelectrode Enables Enhanced Long-Term Neural Recording. Sci. Rep. 6, 34553. doi:10.1038/srep34553
Wong, M. (2011). Epilepsy in a Dish: An In Vitro Model of Epileptogenesis. Epilepsy Curr. 11, 153–154. doi:10.5698/1535-7511-11.5.153
Wu, C., Chen, R., Liu, Y., Yu, Z., Jiang, Y., and Cheng, X. (2017). A Planar Dielectrophoresis-Based Chip for High-Throughput Cell Pairing. Lab. Chip 17, 4008–4014. doi:10.1039/c7lc01082f
Wu, Y., Ren, Y., Tao, Y., Hou, L., and Jiang, H. (2016). Large-Scale Single Particle and Cell Trapping Based on Rotating Electric Field Induced-Charge Electroosmosis. Anal. Chem. 88, 11791–11798. doi:10.1021/acs.analchem.6b03413
Xiao, G., Song, Y., Zhang, Y., Xing, Y., Xu, S., Lu, Z., et al. (2021). Cellular-Scale Microelectrode Arrays to Monitor Movement-Related Neuron Activities in the Epileptic Hippocampus of Awake Mice. IEEE Trans. Biomed. Eng. 68, 19–25. doi:10.1109/tbme.2020.2990356
Xie, C., Lin, Z., Hanson, L., Cui, Y., and Cui, B. (2012). Intracellular Recording of Action Potentials by Nanopillar Electroporation. Nat. Nanotech 7, 185–190. doi:10.1038/nnano.2012.8
Xu, D., Fang, J., Zhang, M., Wang, H., Zhang, T., Hang, T., et al. (2021). Synchronized Intracellular and Extracellular Recording of Action Potentials by Three-Dimensional Nanoroded Electroporation. Biosens. Bioelectron. 192, 113501. doi:10.1016/j.bios.2021.113501
Xu, Z., Li, E., Guo, Z., Yu, R., Hao, H., Xu, Y., et al. (2016). Design and Construction of a Multi-Organ Microfluidic Chip Mimicking the In Vivo Microenvironment of Lung Cancer Metastasis. ACS Appl. Mater. Inter. 8, 25840–25847. doi:10.1021/acsami.6b08746
Yao, J., Zhu, G., Zhao, T., and Takei, M. (2019). Microfluidic Device Embedding Electrodes for Dielectrophoretic Manipulation of cells‐A Review. Electrophoresis 40, 1166–1177. doi:10.1002/elps.201800440
Yoo, J., Kwak, H., Kwon, J., Ha, G. E., Lee, E. H., Song, S., et al. (2020). Long-term Intracellular Recording of Optogenetically-Induced Electrical Activities Using Vertical Nanowire Multi Electrode Array. Sci. Rep. 10, 4279. doi:10.1038/s41598-020-61325-3
Yoshida, S., Teshima, T., Kuribayashi-Shigetomi, K., and Takeuchi, S. (2016). Mobile Microplates for Morphological Control and Assembly of Individual Neural Cells. Adv. Healthc. Mater. 5, 415–420. doi:10.1002/adhm.201500782
Yun, H., Kim, K., and Lee, W. G. (2013). Cell Manipulation in Microfluidics. Biofabrication 5, 022001. doi:10.1088/1758-5082/5/2/022001
Zhang, A., Zhao, Y., You, S. S., and Lieberman, C. M. (2020). Nanowire Probes Could Drive High-Resolution Brain-Machine Interfaces. Nano. Today 31, 100821. doi:10.1016/j.nantod.2019.100821
Zhang, C., Zhao, Z., Abdul Rahim, N. A., van Noort, D., and Yu, H. (2009). Towards a Human-On-Chip: Culturing Multiple Cell Types on a Chip with Compartmentalized Microenvironments. Lab. Chip 9, 3185–3192. doi:10.1039/b915147h
Zhang, H., Yu, P., Zhong, S., Ge, T., Peng, S., Zhou, Z., et al. (2016). Gliocyte and Synapse Analyses in Cerebral Ganglia of the Chinese Mitten Crab, Eriocheir Sinensis: Ultrastructural Study. Eur. J. Histochem. 60, 2655–3203. doi:10.4081/ejh.2016.2655
Zhang, H. Y., Liu, Z. Y., Nan, H. C., and Hu, C. Z. (2019). Ieee, Development of Retina Cell-Laden Alginate Microbeads for Study of Glaucoma, 2019 14th Annual Ieee International Conference on Nano/Micro Engineered and Molecular Systems. New York: IEEE, 143–148.
Zhao, X., Zhao, N., Shi, Y., Xin, H., and Li, B. (2020). Optical Fiber Tweezers: A Versatile Tool for Optical Trapping and Manipulation. Micromachines 11, 114. doi:10.3390/mi11020114
Keywords: network of neurons, lab-on-chip, microfluidics, microelectrode arrays, ex vivo studies, neurological disorders
Citation: Zhang H, Rong G, Bian S and Sawan M (2022) Lab-on-Chip Microsystems for Ex Vivo Network of Neurons Studies: A Review. Front. Bioeng. Biotechnol. 10:841389. doi: 10.3389/fbioe.2022.841389
Received: 22 December 2021; Accepted: 17 January 2022;
Published: 16 February 2022.
Edited by:
Diming Zhang, Zhejiang Lab, ChinaReviewed by:
Zetao Chen, Zhejiang University, ChinaDaizong Ji, Fudan University, China
Timothée Levi, Université de Bordeaux, France
Copyright © 2022 Zhang, Rong, Bian and Sawan. This is an open-access article distributed under the terms of the Creative Commons Attribution License (CC BY). The use, distribution or reproduction in other forums is permitted, provided the original author(s) and the copyright owner(s) are credited and that the original publication in this journal is cited, in accordance with accepted academic practice. No use, distribution or reproduction is permitted which does not comply with these terms.
*Correspondence: Sumin Bian, biansumin@westlake.edu.cn; Mohamad Sawan, sawan@westlake.edu.cn
†These authors share first authorship