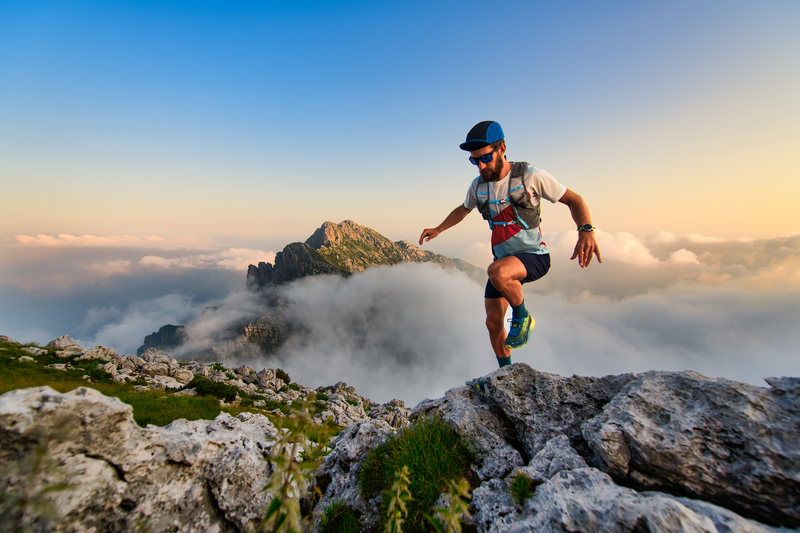
95% of researchers rate our articles as excellent or good
Learn more about the work of our research integrity team to safeguard the quality of each article we publish.
Find out more
REVIEW article
Front. Bioeng. Biotechnol. , 10 February 2022
Sec. Cell and Gene Therapy
Volume 10 - 2022 | https://doi.org/10.3389/fbioe.2022.836984
This article is part of the Research Topic New Technologies for Women’s Health View all 12 articles
Ovarian cancer has the highest mortality of all of the gynecological malignancies. There are several distinct histotypes of this malignancy characterized by specific molecular events and clinical behavior. These histotypes have differing responses to platinum-based drugs that have been the mainstay of therapy for ovarian cancer for decades. For histotypes that initially respond to a chemotherapeutic regime of carboplatin and paclitaxel such as high-grade serous ovarian cancer, the development of chemoresistance is common and underpins incurable disease. Recent discoveries have led to the clinical use of PARP (poly ADP ribose polymerase) inhibitors for ovarian cancers defective in homologous recombination repair, as well as the anti-angiogenic bevacizumab. While predictive molecular testing involving identification of a genomic scar and/or the presence of germline or somatic BRCA1 or BRCA2 mutation are in clinical use to inform the likely success of a PARP inhibitor, no similar tests are available to identify women likely to respond to bevacizumab. Functional tests to predict patient response to any drug are, in fact, essentially absent from clinical care. New drugs are needed to treat ovarian cancer. In this review, we discuss applications to address the currently unmet need of developing physiologically relevant in vitro and ex vivo models of ovarian cancer for fundamental discovery science, and personalized medicine approaches. Traditional two-dimensional (2D) in vitro cell culture of ovarian cancer lacks critical cell-to-cell interactions afforded by culture in three-dimensions. Additionally, modelling interactions with the tumor microenvironment, including the surface of organs in the peritoneal cavity that support metastatic growth of ovarian cancer, will improve the power of these models. Being able to reliably grow primary tumoroid cultures of ovarian cancer will improve the ability to recapitulate tumor heterogeneity. Three-dimensional (3D) modelling systems, from cell lines to organoid or tumoroid cultures, represent enhanced starting points from which improved translational outcomes for women with ovarian cancer will emerge.
Globally, ovarian cancer is the eighth most frequently diagnosed malignancy and cause of cancer-related death in women (Sung et al., 2021). The classification of ovarian cancer includes distinct histological subtypes with varied sites of origin underpinned by defining molecular events affecting tumor suppressors and oncogenes. These events drive specific patterns of clinical behavior characteristic of histotypes, including response to chemotherapeutic agents and molecular target drugs. Malignant histological subtypes arising from epithelial cells include high-grade serous ovarian cancer (HGSOC), ovarian clear cell carcinoma (OCCC), endometrioid ovarian cancer (EnOC), mucinous ovarian cancer (MOC), low-grade serous ovarian cancer (LGSOC) and malignant Brenner cell tumors (Shih Ie and Kurman, 2004; Kurman and Shih Ie, 2010). Ovarian carcinosarcomas (OCS), also known as malignant mixed mullerian tumors (MMMT), have epithelial and mesenchymal components (Harris et al., 2003). Ovarian sex-cord stromal tumors (SCST), the most common of which are granulosa cell tumors (GCT) along with the rarer Sertoli–Leydig cell tumors, are of stromal cell origin (Fuller et al., 2017). An extremely rare subtype of ovarian cancer primarily affecting women under 40 years of age is small cell carcinoma of the ovary, hypercalcemic type (SCCOHT), an ovarian rhabdoid tumor (Auguste et al., 2020).
Almost all HGSOC have a somatic TP53 mutation and p53 immunohistochemistry is a surrogate marker for TP53 mutation in these tumors (Bell, 2011; Cole et al., 2016; Köbel and Kang, 2021). TP53 mutations are also observed in MOC (Köbel and Kang, 2021), ovarian carcinosarcomas (Trento et al., 2020) and less frequently in OCCC (Parra-Herran et al., 2019). BRCA1 and BRCA2 mutations occur in HGSOC, including in the germline of affected patients (Alsop et al., 2012), and rarely in patients with OCCC (De Pauw et al., 2021). ARID1A and ARID1B encode members of the SWI/SNF (SWItch/Sucrose Non-Fermentable) ATP-dependent chromatin remodeling complex important for interaction of this complex with DNA, both genes being mutated in OCCC and endometrioid ovarian cancers (McCluggage and Stewart, 2021). The SWI/SNF complex is also disrupted in the very rare SCCOHT with mutation of SMARCA4 and epigenetic silencing of SMARCA2 that encode catalytic subunits important for nucleosome sliding and eviction (Jelinic et al., 2016; Xue et al., 2021). OCCC and endometrioid carcinomas also have in common a disrupted PTEN-PI3K pathway with mutations observed in PTEN and PIK3CA, as well as mutations in CTNNB1 (Kuo et al., 2009; Hollis et al., 2020). The RAS/MAPK pathway has been implicated in LGSOC, with mutations identified in KRAS, NRAS, and BRAF (Moujaber et al., 2021). Mutations in the RAS/MAPK pathway are also observed in EnOC (Hollis et al., 2020), OCCC (Kim et al., 2018), and MOC (Cheasley et al., 2021). Adult ovarian GCTs are characterized predominantly by mutation of FOXL2, and Sertoli-Leydig Cell Tumors (SLCTs) harbor mutations in DICER1 (Fuller et al., 2017; De Paolis et al., 2021). Epithelial and stromal cell ovarian cancer histotypes and associated genes known to be mutated are summarized in Figure 1.
FIGURE 1. Ovarian cancer histotypes and gene mutations. Epithelial ovarian cancers constitute approximately 90% of all malignant ovarian tumors and are made up of different histotypes: high-grade serous ovarian cancer (HGSOC), endometrioid ovarian cancer (EnOC), ovarian clear cell carcinoma (OCCC), low-grade serous ovarian cancer (LGSOC) and mucinous ovarian cancer (MOC). Ovarian carcinosarcomas (OCS)/malignant mixed mullerian tumors (MMMT) have epithelial and mesenchymal components. Stromal cell tumors include granulosa cell tumors (GCT, adult and juvenile) as well as Sertoli-Leydig cell tumors (SLCTs). Small cell carcinoma of the ovary, hypercalcemic type (SCCOHT) are a rare histotype. Gene mutations, copy number amplifications, methylation and other epigenetic silencing are noted against histotypes.
In addition to mutations, methylation of BRCA1 is also observed in HGSOC, as is amplification of CCNE1 (Bell, 2011; Patch et al., 2015). Along with defects in BRCA1 and BRCA2, other genes that function in homologous recombination repair (HRR) are mutated in ovarian cancer, albeit at lower frequencies, including RAD51C, RAD51D, BRIP1, PALB2, and ATM (Pennington et al., 2014). Defects in HRR can lead to the presence of “genomic scars” caused by the cancer cell’s inability to accurately repair sites of double strand breaks (DSBs). These include extensive loss of heterozygosity (LOH), large scale transitions (LST) and telomeric allelic imbalance (TAI) (Watkins et al., 2014; Chao et al., 2018; Nguyen et al., 2020). Tumors with defects in HRR are responsive to poly adenosine diphosphate-ribose polymerase inhibitors (PARPis), functioning in a synthetic lethal manner to inhibit repair of single strand breaks via the base excision repair pathway (Dedes et al., 2011). As a predictive DNA marker of defective HRR, genomic scars are helpful, but not all tumors with a genomic scar will respond to a PARPi. Reasons for this include reversion of a BRCA mutation, occurrence of a secondary mutation that restores wild-type function or changes in methylation patterns of an HRR gene that in effect functionally restores HRR but the genomic scar remains (Patch et al., 2015). Functional analyses, alongside molecular assays, are required to confirm the predicted response of women with ovarian cancer to PARPis such as the FDA-approved drugs olaparib, niraparib and rucaparib (Dickson et al., 2021; Lee et al., 2021; Valabrega et al., 2021).
Predicting which women will respond to a PARPi is particularly important given the unprecedented improvement seen in Progression Free Survival (PFS) and Overall Survival (OS) for subsets of women administered these drugs, including as maintenance therapy (Audeh et al., 2010; Ledermann et al., 2012; Ledermann J. et al., 2014; Moore et al., 2018). Ovarian cancers with germline or somatic mutation of BRCA1 or BRCA2, mutation of RAD51C or RAD51D, methylation of BRCA1, or high LOH have all been reported to respond to PARP inhibition (Audeh et al., 2010; Wang et al., 2012; Ledermann J. et al., 2014; Kondrashova et al., 2017; Swisher et al., 2017; Moore et al., 2018). Furthermore, loss of RAD51C methylation has recently been implicated as a mechanism of PARPi resistance (Nesic et al., 2021). The combination of molecular testing and functional analyses conducted in robust tumor or ascites models has the power to strongly predict whether a woman is likely to respond to a PARPi at each stage of her disease progression.
Unlike for PARPis, there are no clinically approved biomarkers to predict responses of women with ovarian cancer to the anti-angiogenic bevacizumab, although numerous studies have focused on this area (Buechel et al., 2021; Gao et al., 2021; Hsu et al., 2021). Given the advances seen in PFS and OS of some women receiving this monoclonal antibody that targets vascular endothelial growth factor (VEGF), development of functional assays to predict the likelihood that a woman will respond to bevacizumab would represent a major advance (Burger et al., 2011; Perren et al., 2011; Oza et al., 2015).
The majority of women with HGSOC will respond initially to carboplatin, although some tumors display innate platinum resistance (Davis et al., 2014). There are no robust markers to predict response to platinum drugs, including through the development of acquired chemoresistance, although defects in HRR in primary tumors have been associated with a more favorable response (Konstantinopoulos et al., 2010; Muggia and Safra, 2014). The use of platinum drugs in histotypes other than HGSOC must be questioned given frequent low response rates. Only 11–27% of OCCC respond initially to platinum therapy, dropping to only 1-2% response rates in recurrent disease (Sugiyama et al., 2000; Mabuchi et al., 2016; Tan et al., 2019). LGSOC also display a poor response to platinum drugs; however, the presence of activating mutations in mitogen-activated protein kinase (MAPK) pathway genes KRAS, BRAF, and NRAS has seen favorable responses in LGSOC with the MEK inhibitor trametinib (reviewed in Moujaber et al. (2021)).
The mutations and genomic variations described above offer opportunities to develop new molecular-based therapeutic strategies to treat ovarian cancer subtypes. Some molecular events, such as those described in the HRR pathway, are already being targeted clinically by FDA-approved PARPis. For both discovery science and translational approaches to predicting which women are likely to benefit from which therapies, robust models are needed that expand upon traditional 2D cell culture and pre-clinical models, and include both molecular profiling and functional analyses. In this review, we discuss methods of 3D modelling that are either currently being employed in ovarian cancer cell lines, primary or metastatic tumor tissue and ascites, or have the potential to be used into the future for these purposes.
There are numerous factors to examine when considering the microenvironment that supports the initiation, development and metastasis of ovarian cancer, not least being the very first microenvironment of these malignancies and that is the site of origin of the initial lesion. Many, perhaps most, HGSOC originate in the fallopian tube as Serous Tubal Intraepithelial Carcinomas (STICs), shedding onto the surface of the ovary and establishing a tumor (Crum et al., 2007; Karst et al., 2011; Perets and Drapkin, 2016; Zhang et al., 2019). This discovery has led to the generation of important models of non-cancerous fallopian tube epithelial cells transformed with c-Myc, H-RasV12 or SV40 large T antigen (SV40 TAg) (Karst et al., 2011; Perets and Drapkin, 2016). These models complement those of normal ovarian surface epithelial (OSE) cells immortalized with factors including SV40 Tag, human telomerase (hTERT) and HPV-E6/E7 (Tsao et al., 1995; Davies et al., 2003; Kalli et al., 2004; Shin et al., 2018). OCCC and EnOC have been associated with endometriosis (Samartzis et al., 2020). While MOC has previously been reported as originating from metastatic deposits of primary tumors of the colon, stomach, pancreas and uterus, evidence now shows that these tumors do in fact arise in the ovary following a progression model commencing with benign and borderline precursor lesions (Ledermann J. A. et al., 2014; Cheasley et al., 2019). While constituting ∼10% of all ovarian cancers, tumors arising in ovarian stromal cells have different considerations. In contrast to epithelial cell tumors, the sex cord stromal tumor GCT arise in granulosa cells that produce estrogen (Jamieson and Fuller, 2012). Knowledge of the sites of origin of ovarian tumors is imperative to ensure selection of models that best address both research questions and translational approaches.
The microenvironment of an ovarian cancer consists of both tumor and non-tumor cells, including immune cells. Patient-derived organoids or tumoroids retain cellular heterogeneity and immune cells, thus are able to more strongly recapitulate a three-dimensional (3D) tumor microenvironment ex vivo compared to homogeneous cell lines (Hill et al., 2018; Kopper et al., 2019). Ovarian tumoroid cultures have been established from both ascitic fluid and primary tumors, to date primarily from the more common HGSOC but also from LGSOC, MOC, OCCC, and EnOC (Hill et al., 2018; De Witte et al., 2020; Nanki et al., 2020). These models show great promise for conducting ex vivo drug assays to predict therapeutic response in the women from which they were established (De Witte et al., 2020; Maenhoudt et al., 2020; Nanki et al., 2020; Gorski et al., 2021).
Given the location of ovaries, there are no anatomical barriers preventing metastasis to organs in the pelvic cavity including the uterus, bladder, rectum, and small intestine, as well as beyond the peritoneal cavity to organs such as the liver and lung (Motohara et al., 2019). Ovarian cancer cells detach from the primary tumor and are attracted to adipose-rich omental tissue. They disseminate by forming aggregates of multicellular spheroids that float in malignant ascitic fluid alongside fibroblasts, adipocytes, mesothelial, endothelial and inflammatory cells, as well as cell-free DNA, before “seeding” onto new microenvironments and establishing metastatic deposits (Ford et al., 2020). Given that many commercially available ovarian cancer cell lines are in fact established from ascites rather than primary tumors (Table 1, Supplementary Tables S1,S2) consideration should be given to including these cell types when establishing three dimensional models of ovarian cancer. This is especially relevant for the study of ovarian cancer cell metastasis. An example of this is the organotypic model used by Ford and colleagues to determine the ability of ovarian cancer cell lines undergoing depletion of genes of interest to metastasize and adhere to omental-type tissue (Henry et al., 2017).
To date, the majority of in vitro studies in ovarian cancer have relied on the use of 2D cell culture of immortalized cell lines derived from primary ovarian cancers, pleural effusion, ascitic fluid from the peritoneal cavity or a distant metastatic site. Many cell lines have been well characterized morphologically and molecularly and, when able to be tested, maintain unique features of their derivative sample. Several studies have attempted to determine “the best” ovarian cancer cell line models for investigators to use for both fundamental discovery science and translational projects (Anglesio et al., 2013; Domcke et al., 2013; Beaufort et al., 2014; Papp et al., 2018; Barnes et al., 2021). Comparison of the molecular profiles of ovarian cancer cell lines with that of primary tumors has led to the histotype reclassification of a number of frequently used ovarian cancer cell lines, including SK-OV-3 and A2780. Still, there remains conflicting reports in the field as to the accuracy of some ovarian cancer cell lines. We have summarized the current state of knowledge of site and histotype origin of a group of ovarian cancer cell line models, as well as models of normal cells representing sites of origin (Table 1, Supplementary Tables S1–S3).
With the exception of the PEO series of HGSOC, few ovarian cancer cell line models allow insight into the development and progression of ovarian cancer (Langdon et al., 1988). The PEO1 drug sensitive cell line has the pathogenic BRCA2 mutation, c.5193C > G, derived after initial treatment with cisplatin, 5-Fluorouracil (5-FU) and chlorambucil. The PEO4 cell line represents malignant cells after the patient developed chemoresistance, having a secondary BRCA2 reversion mutation which restores wild-type BRCA2 function. The PEO6 cell line was collected from the same patient before death (Langdon et al., 1988). Other ovarian cancer cell lines have been made resistant to cisplatin in vitro, including A2870/A2780CisR (Behrens et al., 1987), TYK-nu/TYK-nu.CP-r (Yoshiya et al., 1989) and CaOV3/CaOV3CisR (Joshi et al., 2021). The A2780/A2780VeliR lines were made resistant in vitro to the PARPi veliparib (Dickson et al., 2021). Still, often phenotypes such as drug response observed in vitro have been unable to mirror in vivo models, likely due to factors missing in the tumor microenvironment that are absent from homogeneous cell lines cultures such as stromal and immune cells (Beaufort et al., 2014).
Ovarian cancer cell lines show variable ability to grow in nude mice when implanted either subcutaneously (SC), intraperitoneally (IP) or intrabursally (Hernandez et al., 2016). Further, while some cell lines grow well in both SC and IP locations, others show a strong propensity to grow in one location only, suggesting a preference for a particular microenvironment. Ovarian cancer cell lines demonstrated to grow in mice are noted in Table 1 and Supplementary Tables S1,S2.
Animal models continue to be the most physiologically relevant pre-clinical models to study disease pathogenesis and drug response, encompassing a whole-body system, including immune system, tumor microenvironment and vascularization. A number of non-mammalian models including fruit flies, the African clawed frog (Xenopus) and the laying hen, have been utilized for the study of ovarian cancer development (reviewed by (Johnson and Giles, 2013; Rosales-Nieves and González-Reyes, 2014; Bernardo et al., 2015; Tudrej et al., 2019)). The most widely used mammalian model is the mouse (Mus musculus), sharing 85 percent protein-encoding gene homology with humans (Makałowski et al., 1996), although concerns with translatability of disease mechanisms and drug responses between species remain. Further, the natural occurrence of ovarian cancer is low in the aging mouse, with rapid progression times contrasting with the development of human ovarian cancer (Sale and Orsulic, 2006). Nevertheless, genetically engineered mouse (GEM) models, syngeneic and patient-derived xenograft (PDX) models have enabled a greater understanding of ovarian cancer development and treatment responses.
GEM models have enabled specific gene knockout to be modelled in a whole-body system, contributing to the understanding of individual and combinations of genes commonly mutated in ovarian cancer. Conditional knockout mice, using the Cre-lox system for cell type specificity, have been used to reproduce oncogenic mutations and HR defects to study ovarian cancer development and responses to clinically relevant treatments such as platin-based drugs and PARPis (Szabova et al., 2012; Perets et al., 2013; Szabova et al., 2014; Zhai et al., 2017). Extensive overviews of GEM models of ovarian cancer have been published in recent years, highlighting comparisons and translatability to the human condition (Stuckelberger and Drapkin, 2018; Maniati et al., 2020; Zakarya et al., 2020). Syngeneic mouse models transplant mouse cell lines into a recipient from the same genetic background, enabling the study of immune response, immunotherapies and tumor vascularization (Zhang et al., 2002; Yu et al., 2018). The murine ID8 ovarian cancer cell line (C57Bl/6 background) (Roby et al., 2000), has been used for a number of syngeneic mouse models, achieving primary ovarian tumors and ascites within 90 days (Greenaway et al., 2008). This model has also been used to study metastasis and immune infiltrates at the trocar site, where an incision is made into the abdomen for laparoscopic surgery (Wilkinson-Ryan et al., 2019). Injection with M0505 cells (derived from spontaneously transformed OSE of FVB/N mice) resulted in Pax8+ tumors with similar histology to human HGSOC (McCloskey et al., 2014). Generation of multiple fallopian tube epithelial cell lines with combinations of common mutations in HGSOC (Tp53, Brca1, Brca2, Ccne1, Akt2, Brd4, Smarca4, Kras, Myc, Nf1, and Pten) using CRISPR/Cas9 recapitulated histopathological and clinical features observed in HGSOC patients, such as ascites and peritoneal metastases (Iyer et al., 2021). Overall, GEM and syngeneic models have proven their value for discovery studies and research into the origin of ovarian cancer.
PDX models are the most useful in vivo model for testing response to targeted therapies of primary tumors attributed to their unique molecular profiles, to enable a precision medicine approach. The major advantage of using PDX models is the ability to reproduce histology of the human tumor (Colombo et al., 2015), although alterations in steroid hormone receptors and immune response genes have been reported, irrespective of the maintained mutational profile (Dong et al., 2016). Another advantage of PDX models is that they bypass the in vitro culture of tumor cells that may inadvertently drive phenotype divergence from the original tumor (Siolas and Hannon, 2013).
Limitations of primary tumor tissue implanted heterotypically into immunodeficient mice include the inability to recapitulate immune responses, site-specific tumor microenvironment interactions and lastly, that the tumor may not metastasize (Jin et al., 2010). The general methodology of producing PDX models requires multiple in vivo passages, leading to extended model creation times (Morton and Houghton, 2007). The reported engraftment rate can be variable and heavily influenced by ovarian cancer histotype; treatment history; stage and site of malignancy, with higher engraftment rates observed in non-epithelial histotypes (Wu et al., 2019). Platinum resistance has also been found to predict PDX engraftment success, with successful engraftment correlating with shorter PFS and OS of the derivative patient (Heo et al., 2017).
An extensive review of ovarian cancer PDX models by Scott et al. (2013), highlighted a number of gaps in the field related to variations in methodologies, genetic stability over multiple generations, representation of few ovarian cancer histotypes and the limitation of using immunocompromised mice as hosts. More recent developments have addressed some of these concerns, with higher rates of successful engraftment and propagation of rarer ovarian cancer histotypes such as LGSOC (De Thaye et al., 2020) and MOC (Ricci et al., 2020) as well as evaluation of drug responses for homologous recombination deficient (HRD) mutated ovarian cancers (George et al., 2017) and enabling the evaluation of immunotherapies through the use of humanized mouse models (Odunsi et al., 2020). Furthermore, the ability to label tumor cells with luciferin prior to transplantation has enabled tumor growth tracking via bioluminescence imaging (Liu et al., 2017).
Two-dimensional (2D) growth of cancer cells as monolayers may fail to recapitulate aspects of the derivative cell behavior and morphology. Differential drug responses in 2D versus 3D cultures have been observed in many in vitro models of cancer, including ovarian cancers. Previously, high costs of materials, significant manual labor and low levels of reproducibility and matrix tunability rendered 3D culture models less favorable to 2D, irrespective of their higher physiological relevance (Jensen and Teng, 2020). Recent technological advances have enabled higher degrees of control over the creation of 3D cell cultures in areas including matrix stiffness and composition, spatial orientation and creation in an automated and high-throughput manner.
A number of different methodologies and techniques are being used in order to more efficiently create 3D cell culture models of cancer, including ovarian cancers. While there is still no “perfect” 3D in vitro model that can replace in vivo preclinical models, methodological advances are moving the field towards more accurate representations of human tumors, including in regards to drug response. Further, efforts towards creation of high-throughput in vitro and ex vivo models for drug screening have been a focus in recent years, and will eventually replace 2D cultures. An overview of techniques used to create 3D in vitro ovarian cancer models and their considerations is summarized in Table 2.
Liquid overlay techniques, such as the use of ultra-low attachment (ULA) plates or low-attachment coatings enable spheroid formation attributed to the hydrophilic properties of the neutrally charged polystyrene plastic or polymer coating, causing cells to adhere to each other rather than on a 2D surface. By preventing attachment to a surface, use of low attachment plates and coatings present a cost-effective and timely method for spheroid formation or maintenance of existing spheroid structures. Two methods have been used extensively for ovarian cancer spheroid culture to identify mechanisms of progression and various stages of disease, from primary tumor modelling to the generation of spheroids of metastatic ascites. The attachment and disaggregation of these spheroids on top of an ECM or in an immunodeficient mouse also allows assessment of the metastatic potential of the cancer.
The use of flat-bottomed ULA plates (Figure 2) enables heterogenous multicellular aggregate formation from cell suspensions of adherent cells and can be used for short-term maintenance of primary ascites-derived spheroids. This method is often combined with secondary metastatic invasion assays involving the transfer of spheroids to regular tissue culture plastic plates or onto an extracellular matrix (ECM).
FIGURE 2. Techniques to create scaffold-free 3D in vitro cancer models. Creation of 3D cell models in the absence of scaffolds promotes cell-cell interactions in three dimensions that mediate cell behavior and drug response when compared to (A) 2D monolayers. Use of (B) liquid overlay techniques with i) flat or ii) round-bottomed ULA plates (C), hanging drop techniques and (D) rotating bioreactors such as i) spinner flasks and ii) horizontal rotating vessels have been used as time and cost-effective spheroid creation methods or to investigate drug response and other factors that my influence ovarian cancer progression, such as fluid shear stress and hypoxia. Created with Biorender.com.
Culture of ovarian cancer cell lines in ULA plates as “ascitic” spheroids has been used as a model to investigate the efficacy of an oncolytic virus-based therapeutic on ovarian cancer metastasis (Tong et al., 2015). Patient-derived ovarian cancer ascites cells, when maintained on ULA plates, demonstrated epithelial-mesenchymal transition (EMT) during spheroid formation (Rafehi et al., 2016). Patient-derived solid-tumor and ascites-derived ovarian cancer cell lines both form spheroids similar to those found in patient ascites when grown on ULA plates, and were shown to be self-renewing through serial passaging over a 6-month period (Yamawaki et al., 2021). Patient-derived spheroids were also more tumorigenic in immunodeficient mice, more stem-like and more invasive than their parental cell line (Liao et al., 2014). A direct comparison of cisplatin and paclitaxel sensitive and resistant A2780 cells grown as 2D monolayers versus 3D aggregates formed in ULA plates, identified gene expression changes attributed to conformation that may lead to drug resistance (Nowacka et al., 2021).
Poly(2-hydroxyethyl methacrylate), known as poly-HEMA, is a non-ionic polymer coating that discourages the formation of ECM and creates a low-adhesion environment that favors spheroid formation (Ivascu and Kubbies, 2006). A poly-HEMA coating in culture flasks has been used for the production of 3D heterotypic models of normal ovary and to study early ovarian cancer development (Lawrenson et al., 2012). Ovarian cancer cell lines grown under poly-HEMA conditions in the presence of activated platelet releasate, formed spheroids faster and were more resistant to the chemotherapeutic agents cisplatin, carboplatin and paclitaxel (Casagrande et al., 2021).
While flat-bottomed, ULA plates and non-adherent coatings present a cost-effective method for production and maintenance of ovarian cancer spheroids, these methods result in heterogenous spheroid morphologies which may impact on downstream analysis, reproducibility and drug response. As such, we suggest that this method is best suited for investigations into drivers of spontaneous spheroid formation.
The use of round-bottomed ULA plates (Figure 2) facilitates single spheroid formation by gravity, and is often supplemented with ∼2% Matrigel to fast track cell aggregations over periods of time less than 72 h. This method allows high consistency between replicates from a cell line or patient sample, as well as facilitating the ability to section and morphologically analyze biologically-relevant structures. Formation of single large spheroids (>500 μm diameter) enables the formation of a hypoxic core, and drug, oxygen and metabolite gradients that mimic solid tumor physiology (Vinci et al., 2012; Heredia-Soto et al., 2018).
The use of round-bottomed, ULA plates have assisted in the identification of Nectin-4 as essential for adhesion events in early spheroid formation of HGSOC cell lines, and as potential targets to improve chemotherapeutic sensitivity (Boylan et al., 2020). Pre-formed OVCAR-8 spheroids grown in round-bottomed ULA plates and further embedded as single spheroids in Matrigel, developed vascularization after subcutaneous transplantation into athymic nude mice, enabling the evaluation of therapeutics such as the anti-angiogenic bevacizumab and the nano-drug Doxil® (Singh et al., 2020).
This method of spheroid formation has been utilized for speed, reproducibility and commercial availability, although spheroid morphology and overall size may differ due to intrinsic cell line-related characteristics. In a comparison of hanging drop arrays, liquid overlay on ULA plates and liquid overlay on ULA plates with a nutator device to produce three dimensional agitation, both the hanging drop and ULA plates with agitation demonstrated higher cellular compaction, higher ECM content and increased resistance to cisplatin compared with cultures on liquid overlaid ULA plates only (Raghavan et al., 2016). Addition of agitation may improve the comparability to other scaffold-free spheroid formation techniques, although this is yet to be tested on primary ovarian cancer cells.
Hanging drop cultures rely on surface tension and gravity to form homogenous, multicellular spheroid/aggregate cultures without the need for specialized equipment (Figure 2). Suspended cells in media are seeded either on the lid of a culture dish or in hang drop vessels, gather at the base of the droplet by gravity, aggregate by cell-cell integrin bridges and further mature by cell-mediated contraction to form compact spheroids within days (Sodek et al., 2009).
The hanging drop technique has been used in numerous studies investigating ovarian cancer morphology and phenotypes. The simplicity of the model has enabled it to act as a “low-stiffness” model as compared to traditional polystyrene plastic culture dishes (Mieulet et al., 2021). An advantage of this technique, particularly for low-volume primary tumor samples, is the formation of spheroids within days with high viability. Further, cell numbers as low as 10 cells per droplet will form spheroids that are uniform in both volume and circularity, amenable to many downstream analyses including high throughput analysis of drug responses (Raghavan et al., 2015).
The use of the hanging drop technique has led to the identification of mechanisms that promote ovarian cancer progression, chemoresistance and recurrence. A study of six ovarian cancer cell lines found a positive relationship between EMT status, spindle-like morphology and compactness of the formed spheroid, with more mesenchymal ovarian cancer cells exhibiting greater invasive and chemoresistant phenotypes (Sodek et al., 2009). Serial passaging of OVCAR-3 and ascites-derived spheroids in the hang drop system showed increasing stemness, proliferation, resistance to cisplatin and tumorigenicity in vivo with passage age (Ward Rashidi et al., 2019). Using a high-throughput 384-well hang drop array culture, increasing spheroid size and cell count was associated with resistance to cisplatin in A2780 and OVCAR-3 cells, which has impact particularly in ascitic spheroids escaping chemotherapeutic treatment (Raghavan et al., 2015). The stem-like changes and chemoresistance observed in this simple, multicellular spheroid model that would not be observed in traditional 2D cultures, emphasizes the importance of three-dimensional cell-cell interactions when modelling drug response. 3D heterotypic multicellular tumor spheroids generated by the hanging-drop technique using the cell lines HEY or SK-OV-3 in co-culture with the mouse fibroblast line NIH3T3, were used to identify off-target effects of drugs targeting cancer cells relative to neighboring stromal cells (Weydert et al., 2020).
While simple in design, using the hanging drop technique for spheroid creation has logistical issues with media replacements, drug addition, evaporation and downstream analysis of individual spheroids per hanging drop (Kunz-Schughart et al., 2004; Mehta et al., 2012). These models are therefore limited to short-term culture and require frequent attention. Improvements to the efficiency and reproducibility of this method include development of hanging drop arrays for use with liquid handling robotics and single cell seeding in nanoliter-sized wells in a microchip format (Raghavan et al., 2015; Ganguli et al., 2021). Creation of an open-source, 3D printable multi-purpose hanging drop “dripper” for use with standard tissue culture plates enables metastasis and migration assays as well as co-cultures of cells within the same plate (Zhao L. et al., 2019).
Inclusion of scaffolds in 3D multicellular in vitro models of ovarian cancer adds another level of model complexity, allowing for the recreation of the physical and mechanical tumor microenvironment that can influence ovarian cancer cell behavior. Several methodologies pertaining to the use of scaffolds relevant to ovarian cancer are described here and in Figure 3.
FIGURE 3. Techniques to create 3D in vitro cancer models using scaffolds. Addition of extracellular matrix (ECM) as scaffolds for 3D cell cultures enables both cell-cell and cell-ECM interactions for a more physiologically relevant 3D cancer cell model. Methods include (A) ECM/hydrogels with cancer cells i) on top of, or ii) encapsulated within an ECM, iii) organotypic omental co-culture model and iv) organoid propagation. (B) 3D bioprinting techniques such as i) extrusion-based bioprinting enables creation of 3D cell-laden models in hydrogels in a layer-by-layer manner, and ii) droplet-based bioprinting enabling high-throughput creation of 3D cell models in hydrogels with higher spatial control for more complex co-culture. (C) Tumor-on-a-chip microfluidic devices have been used to model the effects of fluid shear stress, as well as simulating nutrient, gas and drug gradients, for ascites metastasis modelling. Created with Biorender.com.
Naturally derived ECM hydrogels such as Matrigel, collagens, alginate, and agarose have been favored in 3D cell culture models due to their history of high biocompatibility with various cell types, including ovarian cancer cell lines and tumor organoids, although they are limited in their mechanical tunability and composition consistency.
Matrigel, derived from the murine Engelbreth-Holm-Swarm (EHS) sarcoma, has been used for over 40 years as a mimic for the basement membrane and a structural support for many cell types (Orkin et al., 1977). The major constituents of this ECM are laminins, collagen IV, entactin, and the heparin sulfate proteoglycan perlecan (Hughes et al., 2010), though ratios often differ by batch, raising the need for caution when interpreting results of cells cultured in Matrigel (Vukicevic et al., 1992). Matrigel or EHS matrix may also contain collagens I, XVIII, VI, and III, alongside growth factors and enzymes such as TGF-β, FGF, and matrix metalloproteinases (MMPs) (Hughes et al., 2010).
Matrigel has been widely used for in vitro ovarian cancer models as the ECM components overlap with those found in vivo. As a matrix, Matrigel has been utilized for the assessment of in vitro ovarian cancer cell invasion by cell penetration through Matrigel-coated transwell inserts (Woo et al., 2007; Fujisawa et al., 2012; Hallas-Potts et al., 2019). Metastatic outgrowth of ovarian cancer spheroids embedded within Matrigel has also been assessed (Sodek et al., 2008). Numerous ovarian cancer cell lines have been reported to require encapsulation within Matrigel or prior growth in Matrigel for successful seeding as in vivo tumors into athymic nude mice (Mullen et al., 1996). Growth of ovarian cancer cells on top of a Matrigel bed has been used to model seeding of metastatic ovarian cancer onto the peritoneum, resulting in ovarian cancer nodules in the absence of vascularization, and modelling chemoresistant metastases in the presence of a hypoxic core (Evans et al., 2011). At a 2-2.5% concentration in culture media, Matrigel has been utilized to promote efficient formation of dense, single spheroids of a number of cancer cell types, including ovarian, over 24 h in poly-HEMA treated, non-adherent, round-bottom plates for high-throughput toxicity studies (Ivascu and Kubbies, 2006). Further, Matrigel has been used as a scaffold to propagate primary cell types associated with ovarian cancer such as mesothelial cells and fibroblasts (Kenny et al., 2007), as well as to establish ovarian cancer tumoroids from patient biopsies that maintain key characteristics of the primary tumor (Maenhoudt and Vankelecom, 2021).
Aside from commercial availability and high biocompatibility with a wide range of cell types, there continues to be concerns regarding the use of Matrigel in a number of areas, including: batch-to-batch variation, potential for xenogeneic contaminants derived from mouse on human cells, inefficient cell retrieval requiring temperature reversal to 4°C, potential DNA/RNA contamination from the matrix, and matrix autofluorescence that reduces signal-to-noise ratio when imaging fluorescently stained cells in situ (Graf and Boppart, 2010; Hughes et al., 2010). The reported average modulus value of Matrigel was found to be approximately 450 Pa (Soofi et al., 2009), which remains below the average stiffness of human or mouse ovary or omentum (Alkhouli et al., 2013; Mckenzie et al., 2018; Hopkins et al., 2021). Further, the polymerization temperature for Matrigel of 22–37°C, mediated by entactin interaction with laminins and collagen IV, also limits its bioprintability in the absence of temperature-controlled environments or as a hybrid bioink (Fan et al., 2016).
Overall, Matrigel continues to be one of the most versatile ECMs for in vitro modelling of ovarian cancer cells. Synthetic alternatives are becoming more prominent but have yet to meet the standards of enabling the propagation of patient-derived spheroids and organoids as well as having mechanical properties to enable bio-printing for highly reproducible and high-throughput ovarian cancer model development. Until this time, Matrigel’s major advantages lie with its rich ECM composition for biocompatibility with a wide range of cell types, including for ovarian cancers.
Collagens are the most abundant ECM proteins in the body and are also the most widely used ECM for in vitro cell cultures due to high biocompatibility and commercial availability. Collagens provide structural support and facilitate cell adhesion, proliferation, differentiation and migration both in vivo and in vitro. Made up of 28 identified collagen types, the low antigenicity of collagen has enabled cross-species compatibility and in turn, availability from a variety of biological sources, including rat, bovine, porcine, and recombinant human (Lynn et al., 2004; Yang et al., 2004). Excessive macrofibrillar collagen-I produced by resident fibroblasts has been implicated in the promotion of cancer progression, metastatic and chemoresistant tumor microenvironments (Gurler et al., 2015). Crosslinking of collagen that increases stromal stiffness has also been implicated in chemoresistance (Sterzyńska et al., 2018). While there have been anti-cancer therapeutic strategies developed to enhance the efficiency of chemotherapy (Zhao Y. et al., 2019), collagen is abundant in both pathophysiological and normophysiological states and therefore, anti-collagen therapies require a targeted method of delivery (Xu et al., 2019).
Collagens, particularly collagen-I, have been used extensively in 3D ovarian cancer spheroid and organotypic cultures. OVCAR-5 spheroids plated on a collagen-I coating, were observed to readily disaggregate with increasing collagen concentration, compared to laminin or collagen-IV coatings, thus highlighting its properties as a promoter of cancer cell adhesion and invasion (Burleson et al., 2004). Rat-tail collagen-I has been used as the ECM to support primary mesothelial and fibroblast cells in an omental model of ovarian cancer metastasis (Kenny et al., 2007). Encapsulation of human ovarian cancer cell lines derived from primary tumors in collagen-I hydrogels showed enhanced mesenchymal traits, invasiveness and drug resistance (Liu et al., 2018). Microfibrillar collagen-VI, in addition to collagen-I, has also been reported to promote platinum resistance, cancer cell survival and HGSOC relapse both in vivo and ex vivo in collagen hydrogels (Pietilä et al., 2021). Collagen-XI has been implicated in tumor aggressiveness and poor clinical outcome of patients with ovarian cancer (Wu et al., 2014), although it has not been used as a scaffold for 3D ovarian cancer cultures. Marine collagen sources, such as from jellyfish species, have been found to have high amino acid similarity to collagen-I from mammals, showing similar in vitro cell behavior responses to traditional collagen-I matrices, and as such, are being investigated as a more sustainable collagen source (Paradiso et al., 2019).
Alginate is a natural polysaccharide derived from the brown algae Phaeophycota. Features such as high biocompatibility, biodegradability, low immunogenicity, and low cost have been the drivers behind its use in tissue engineering and drug delivery studies (Gombotz and Wee, 1998). Control of the degree of gelation, and in turn stiffness, can be modulated by multivalent cations including Ca2+, Fe3+, or Sr2+ for crosslinking. These cations can also mediate individual biofunctional properties such as cell attachment or absorption of serum proteins (Machida-Sano et al., 2009). However, proteins are unable to interact with the matrix, and as such ECM-cell signaling is lacking in alginate models and is considered a more synthetic ECM that can be biofunctionalized by the addition of peptides (Rowley et al., 1999). Further, certain cations may also induce cytotoxicity and may differ in stability.
While more studies have used alginate as a drug delivery system, alginate and alginate mixes, such as chitosan-alginate, have also been used as 3D hydrogel scaffolds for the enrichment of a cancer stem cell (CSC) phenotype in prostate, breast and hepatocellular carcinomas (Florczyk et al., 2016). Alginate encapsulation has been used as a method for ex vivo culture of murine ovary slices for the investigation of ovarian surface epithelial changes that may drive cancer development (King et al., 2011). Many reports have also combined alginate with other ECMs, both natural and synthetic, to create more biofunctionalized scaffolds to support a variety of ovarian cell types. Shin and colleagues combined alginate, for its biocompatible properties, with marine collagen and agarose to create a hydrogel that supported the growth of A2780 endometrioid ovarian cancer cells (Shin et al., 2016). A double network of alginate and polyethylene glycol (PEG)-based hydrogels was shown to increase doxorubicin resistance and CSC markers in SK-OV-3 cells compared to 2D cultures (Zhou et al., 2020). SK-OV-3 cells grown in these scaffolds were also reported to be more tumorigenic in a triple immunodeficient mouse model NCG (NOD-Prkdcem26Cd52Il2rgem26Cd22/NjuCrl) (Zhou et al., 2020).
Agarose is a natural linear polysaccharide derived from the marine red algae Rhodophyceae, made from repeating monomeric units of agarobiose (Zarrintaj et al., 2018). In comparison, agar, famously used for microbiological growth purposes, is comprised of a heterogenous mixture of agarobiose and agaropectin. Their features of high biocompatibility, commercial availability, ability to adjust stiffness with concentration and reversible polymerization enabled the use of agar and agarose as in vitro and in vivo cell scaffolds that mimic soft tissue stiffness (Varoni et al., 2012). Whilst normally inert to cell interaction, agarose can be bioengineered to integrate biofunctional peptides that supports cell adhesion and cell viability (Yamada et al., 2020).
The use of soft agar to determine tumorgenicity and invasiveness of cancer cell lines has been employed for more than 50 years (Shin et al., 1975). Early work utilized soft agar assays where clusters of cancer cells from malignant effusions from ovarian cancer patients were propagated as clones on an agar base and responded to the anti-cancer drugs cisplatin and 5-FU in a similar manner to their derivative patient (Ozols et al., 1980). Agarose, as a hydrogel for ovarian cancer cells, has been used to show physiological cancer characteristics such as elevated expression of HIF-1α, VEGF-A, profibrogenic MMP-2 and -9 when compared to 2D SK-OV-3 monolayers (Xu et al., 2014). In contrast, an agarose coating was used as a non-adherent surface on which the liquid overlay technique of spheroid formation was used. HEY and OVHS1 ovarian cancer cells grown by liquid overlay technique with an agarose low-attachment base, formed spheroids more readily than the normal ovarian cell line IOSE29, and further, showed metastatic features such as disaggregation and MMP activation when transferred onto an ECM (Shield et al., 2007).
Synthetic ECMs are becoming a more popular in vitro ECM option compared to gold standard EHS-derived ECMs, due to a higher degree of control over properties such as stiffness, pore size and biofunctionalization.
Hydrogels based on bioinert polyethylene glycol (PEG) have been widely utilized for in vitro 3D models for their ability to customize biofunctionalization by peptides and hydrogel stiffness control. The inclusion of MMP-degradable crosslinkers also enables more physiological proteolytic ECM remodeling by cancer cells (Lutolf et al., 2003). A 4-arm PEG-maleimide based hydrogel was designed to recreate the omentum ECM with GFOGER (collagen-I), PHSRN-RGD (fibronectin), RGD (fibronectin, vitronectin, collagen-VI), and DGEA (collagen-IV) biofunctionalization peptide motifs, adjusting stiffness to a physiological level of ∼2.9 kPa for omental tissue and incorporating MMP-degradable crosslinkers (Brooks et al., 2019). This hydrogel supported cell growth and viability of SK-OV-3 multicellular spheroids that were pre-made in non-adherent polydimethylsiloxane (PDMS)-coated grids and spheroids from patient-derived ascites, with paclitaxel and doxorubicin treatments mimicking the drug responses seen in patients. PEG-crosslinked poly(methyl vinyl ether-alt-maleic acid) or PEMM/alginate network hydrogels induced EMT, a CSC-like phenotype and chemoresistance in encapsulated SK-OV-3 spheroids, driven by hydrogel stiffness, porosity and cation of choice for crosslinking (Zhou et al., 2020). This pro-metastatic phenotype was confirmed when hydrogels were implanted into immunodeficient mice. An extension of this work by the same authors showed higher RGD (arginine-glycine-aspartic acid) concentrations encouraged spheroid cell dispersion and drug sensitivity, whereas hydrogels with lower RGD concentrations had preserved cell aggregations with CSC-like changes and drug resistance when grown on PEMM hydrogel discs with varying RGD concentrations (Zhou et al., 2021). When taken together to account for mechanical properties, lower stiffness hydrogels with low RGD levels promoted a CSC-like phenotype with drug resistance. While this study highlighted the importance of cell-ECM interactions in drug resistance, the hydrogels studied were of a stiffness much higher than in vivo physiological conditions ranging from 60–240 kPa.
GelMA is a semi-synthetic bioengineered, biocompatible material with high batch-to-batch consistency, control of mechanophysical properties, innate RGD responsive peptide motifs and ability to be cleaved by MMPs, having been designed as an alternative to Matrigel (Zhu et al., 2019). GelMA requires modification with a photocrosslinker (typically UV or visible light responsive) for efficient polymerization and to prevent degradation. Visible light, as expected, promotes higher cell viability and lower free radical damage compared to UV light when using GelMA (Noshadi et al., 2017). Addition of Laminin-411 and hyaluronic acid to GelMA hydrogels of 3.4 kPa stiffness enabled ovarian cancer spheroid formation, proliferation and chemoresistance to paclitaxel (Kaemmerer et al., 2014). GelMA hydrogel models also showed similar tumorigenic responses when transplanted into NOD/SCID mice. Interestingly, peripheral blood mononuclear cells or PBMCs grown on GelMA hydrogels showed suppressed pro-inflammatory responses to stimulation with lipopolysaccharide (LPS), particularly in the presence of tumor necrosis factor (TNF), whereby cellular TNF mRNA levels were elevated, but soluble TNF was bound to the hydrogel (Donaldson et al., 2018). This study highlighted the need to consider the immunogenic properties of biomaterials to match hydrogel models for their appropriate application, and may have implications on future 3D in vitro immunotherapy models. In the 3D bioprinting field, ovarian cancer cell lines have to date only been used to test printability of GelMA with extrusion based bioprinting, prior to testing its biocompatibility with murine oocytes (Wu et al., 2021).
Self-assembling peptide-based hydrogels are increasingly being used as a biomimetic material for 3D in vitro culture for their engineerability, ability to form well-defined nanofibers with natural amino acid constituents and absence of animal-derived contaminants (Yang et al., 2020). RADA16-I peptide-based hydrogels performed as well as Matrigel and Collagen-I based hydrogels in terms of cell viability, adhesion, migration and drug resistance of encapsulated A2780 and SK-OV-3 ovarian cancer cells (Yang and Zhao, 2011). A disadvantage of some peptide-based hydrogels is their low mechanical strength, where hydrogels with encapsulated cells are able to be disrupted easily by mechanical forces such as pipetting (Song et al., 2020). A study by Hedegaard and colleagues utilized peptide amphiphile-based hydrogels with elastin mimetics, fibronectin, keratin, RGDS (arginine-glycine-aspartic acid-serine) motif for cell adhesion and GHK (glycine-histidine-lysine) motif for pro-angiogenic signaling with encapsulated OVCAR-4 cells in monoculture as well as in co-culture with HUVECs and human mesenchymal stem cells (Hedegaard et al., 2020). Tumor spheroids grown in this hydrogel were comparable in morphology, viability and drug response compared to those grown in GelMA and Matrigel. Importantly, this model is one of the first to incorporate peptides to promote angiogenesis, addressing one of the major missing components of 3D in vitro cell culture models.
Decellularized extracellular matrices (dECM) are the natural matrices from an organ that have been void of all native cellular components, preserving the biological and mechanical properties of the original ECM. These ECMs can be used to seed new cells in an organ’s native conformation in the absence of an immune response, as well as can be lyophilized and reconstituted to form hydrogels. To date, there have been no reports of using decellularized ovary ECM for the study of ovarian cancers. However, the use of ovarian dECMs might benefit 3D in vitro ovarian models, due to the preservation of ECM proteins and growth factors found in the native ovary. A handful of studies have shown high biocompatibility with ovarian cell types grown in reconstituted dECM hydrogels and scaffolds. A mixture of sodium alginate with decellularized murine ovarian tissue supported in vitro follicle survival (Nikniaz et al., 2021). Preliminary studies of hydrogels derived from decellularized porcine ovarian ECM highlighted the effect of ECM stiffness on ovarian follicle development, with stiffer matrices reducing oocyte viability and triggering premature follicle release (Buckenmeyer et al., 2016), though this effect has not been investigated with ovarian cancer cells. There are nevertheless some concerns surrounding the use of dECMs, namely donor batch differences, retention of native genetic material such as DNA, and the harsh decellularization process that may result in a loss of critical downstream biological interactions with cells (Mendibil et al., 2020).
Three-dimensional organotypic models of the human mesothelium (Figure 3) have been utilized for the study of early metastasis to the omentum and interactions with the tumor microenvironment (Kenny et al., 2007). Taking a layered approach to reproduce the architecture of the omentum as observed from histological staining of omental biopsies, this model consists of primary human fibroblasts with ECM, rat-tail collagen-I and human fibronectin, as a base, layered with primary human mesothelial cells. Ovarian cancer cells or ascites-derived spheroids can be added to investigate cell attachment and invasion into the omentum. Using this model, Kenny and colleagues identified MMP-2 mediated cleavage of fibronectin and vitronectin produced by mesothelial cells as an early response to omental metastasis (Kenny et al., 2009). Another study identified mir-193b downregulation as a driver of omental metastasis with this mesothelial model (Mitra et al., 2015a). Further, Henry and colleagues described the dynamic roles of the receptor tyrosine kinases ROR1 and ROR2 in different omental cell types that promote cancer cell adhesion in early metastasis (Henry et al., 2017). This model has also been adapted for high throughput drug screening of potential inhibitors of adhesion and invasion. Though the mesothelial models had fewer efficacious drug hits than traditional 2D models, similar drug responses were observed in vivo in PDX models (Kenny et al., 2014; Lal-Nag et al., 2017). This organotypic model was also more cost and time-efficient with similar results to in vivo models, when testing micellar-based nanoparticle therapies to prevent early metastasis (Lu et al., 2019). To add complexity to the static organotypic mesothelial model, it has been incorporated into a microfluidics device, enabling the study of hydrodynamic forces of ascites fluid flow on spheroid attachment and metastasis.
The use of the organotypic mesothelial model has been reviewed in depth and a standardized protocol published to enable reproducibility between research groups across the world (Peters et al., 2015; Watters et al., 2018). While this co-culture model has been quite successful, as a scalable, 3D pre-clinical model of ovarian cancer metastases, it lacks vasculature, as well as immune cells and cells from adipose tissue.
In order to best recapitulate the TME of ovarian cancers, the addition of cancer-associated cells types including stromal, immune, mesenchymal, mesothelial, and endothelial cells, to ovarian cancer cells in vitro enables the heterotypic cell-to-cell crosstalk that may influence chemoresistance, angiogenesis, and metastasis. Two-dimensional co-cultures, while enabling intercellular contact and cross-talk, cannot mimic the multidimensional cell interactions. Three-dimensional co-cultures, particularly when combined with ECM scaffolds, are in vitro, biomimetic models that may better represent the in vivo situation and more accurately predict cellular responses in a patient. While not an exhaustive list, recently published 2D and 3D ovarian cancer co-cultures models are summarized in Table 3.
There are two major co-culture methodologies: indirect and direct contact models. Indirect contact co-cultures, such as by the use of transwells or conditioned media, mimic cellular signaling in the absence of physical cellular contact, and enable simple segregation of cell types for ease of downstream analyses of individual cell population behavior. Direct contact co-cultures enable the study of physical interaction of several cell types and their influence on cell behavior as an “organ-like” model. Two-dimensional culture studies showed ovarian cancer cell lines in contact with normal omental fibroblasts could drive activation to a cancer-associated fibroblast (CAF) phenotype associated with TGF-β1 secretion (Cai et al., 2012).
In 3D multicellular spheroid cultures, addition of fibroblasts, mesenchymal stem cells, or endothelial cells have been shown to enhance spheroid size, chemoresistance and a stem-like phenotype compared to 3D monocultures (Hedegaard et al., 2020; Tofani et al., 2021). Co-cultures of ovarian cancer cells and macrophages have identified positive feedback loops that drive stemness, chemoresistance and spheroid formation in cancer cells and M2 polarization of macrophages (Long et al., 2018; Ning et al., 2018; Raghavan et al., 2019). Three-dimensional co-culture systems of ovarian cancers and mesothelial cells, including the organotypic omental mesothelium model (described in Section 5.2.4) have also enabled the study of mechanisms driving peritoneal and omental metastasis (Iwanicki et al., 2011; Henry et al., 2017; Shishido et al., 2018; Loessner et al., 2019).
The number of studies utilizing 3D co-cultures of ovarian cancers at the time of this publication was limited but is growing. It is clear that with increasing model complexity through addition of multiple patient-derived cell types in conjunction with physiologically relevant ECM mimics, that the most biologically appropriate and predictive in vitro models will be discovered. Integration of organoids with endothelial cells could enable more biologically appropriate, predictive studies of anti-angiogenic drugs such as bevicizumab in the presence of ovarian cancers with specific mutations. Further, utilization of microfluidics devices for emulation of fluid flow and the addition of immune cells types enable the creation of a more accurate biomimetic TME.
Patient-derived organoids are becoming an important and powerful pre-clinical model for personalized medicine (Figure 3). While primary patient-derived samples are the gold standard for prediction of treatment response, long-term culture of these samples in 2D often leads to phenotypic changes and differential responses to drug treatments (Kapałczyńska et al., 2018). Cryopreservation of patient-derived organoids allows for biobanking of unique samples for genotype/phenotype matching with future samples from the same patient as their cancer progresses to inform treatment decision making.
In comparison to xenograft models, organoid or tumoroid cultures require significantly shorter times for development from small starting samples, have a higher success rate and accurately reproduce the genetic and phenotypic features of the derivative tumor, allowing for personalized medicine strategies (Hidalgo et al., 2014). Nanki and colleagues were able to propagate primary ovarian tumor organoids from a variety of histological subtypes including HGSOC, OCCC, and EnOC with an 80% success rate and maintenance of their original tumor mutational profiles (Nanki et al., 2020). The analysis of ovarian tumor organoids derived from multiple tumor sites within the same patient showed differential drug sensitivities, emphasizing the need to account for intrapatient tumor heterogeneity (De Witte et al., 2020). When grown in a modified Matrigel bilayer, organoids from MOCs could reproduce behaviors of their derivative cancer, such as production of mucin and a cystic morphology (Maru et al., 2019).
While there is growing evidence that ovarian cancer organoids/tumoroids are becoming the new benchmark for pre-clinical models compared to traditional 2D methods, varying success rates have been reported, particularly attributed to the heterogeneity of these cancers, and propagation success has been highly dependent on starting sample volume and sample handling. In contrast to traditional organoid culture methods that utilize Wnt to maintain stem-like properties, Wnt pathway induction resulted in tumoroid growth arrest in HGSOC organoids, however was able to be rescued by BMP signaling (Hoffmann et al., 2020). The opposite was observed in fallopian tube organoids whereby shRNA downregulation of tumor suppressor genes TP53, PTEN, and RB, showed stem-like changes occurring early in tumor development. Work by Maenhoudt and colleagues identified that the addition of neuregulin-1 (NRG1) to culture medium can increase the proliferation time of primary organoid cultures from fresh and cryopreserved HGSOC and LGSOC, particularly in slower-growing cultures (Maenhoudt et al., 2020). In contrast, it has been noted that organoid propagation methods tend to be selective and result in a cell population that may not be representative of the original tumor heterogeneity and in turn, treatment response, particularly in patients who have undergone previous neoadjuvant therapy (Hill et al., 2018). Therefore, care must be taken in interpretation of data and use of the correct modelling systems. Improvements in methodologies to maintain the heterogenous phenotype that exists in patient tumors are needed, such as propagation of organoids from different regions of the same tumor (Maru et al., 2019). Furthermore, the standardization of protocols and materials for organoid culture between laboratories around the world will lead to vast improvements in reproducibility and reliability of this technique into the future.
As the initial triggers for the development of ovarian cancer is still being debated, organoids from normal ovarian tissue have also been utilized in studies of tumor development. Organoid cultures from high-risk women with germline mutations in BRCA1 or BRCA2 have been developed for research into early tumor development (Kopper et al., 2019). Kopper and colleagues have performed gene editing in normal non-tumor organoids established from fallopian tube or ovarian epithelium using CRISPR-Cas9 gene editing for modelling TP53 mutations, and determined that HRD ovarian cancer organoids with fewer RAD51 loci were more sensitive to the PARPi niraparib, analogous to responses observed in vivo (Kopper et al., 2019). An extension of this work has similarly used CRISPR-Cas9 genome editing to introduce common HGSOC gene defects into mouse oviduct and OSE cell organoids, showing that both organoid types could become carcinogenic and that drug sensitivities in HGSOC patients may be cell lineage-dependent (Lõhmussaar et al., 2020).
Three dimensional bioprinting presents an automated solution that combines both ECM components and high-throughput creation of ovarian cancer models in a spatially-controlled manner (Figure 3). As a relatively new approach to 3D cell model creation, the number of published studies is limited for ovarian cancer.
Droplet-based bioprinting systems enable the precise and agile fabrication of microtissue cultures by overlaying drops of biomaterial. Used mainly for deposition of scaffolds, this layer-by-layer technique has also been used for addition of drugs, growth factors and living cells. Xu and colleagues have used a droplet-based system to bioprint OVCAR-5 cells and MRF-5 normal fibroblasts on top of a Matrigel scaffold in a controlled spatial distribution for investigation of feedback mechanisms between tumor and stromal cells (Xu et al., 2011). While there are no publications to date utilizing drop-on-demand inkjet bioprinting, laser-assisted bioprinting or stereolithography with ovarian cancer cells, these are promising techniques that could enable high-throughput 3D ovarian cancer cell model development that would seamlessly integrate with existing high-throughput drug screening technologies (Mazzocchi et al., 2019).
Extrusion based bioprinting has been employed to fabricate a 3D bioprinted ovary that successfully supported oocyte growth using a GelMA-based bioink (Wu et al., 2021). While this study focused on oocyte maturation ex vivo, ovarian cancer cell lines were utilized during optimization of bioink biocompatibility, showing high cell viability during and after the extrusion process. Unfortunately, this was not translatable in primary murine oocytes, suggestive of the delicate nature of primary-derived cells with this technique.
An important factor of 3D bioprinting is the printability of the matrix to be deposited. The material properties of Matrigel and other natural matrices are limited in their printability as bioinks due to temperature sensitivities and pre-determined stiffnesses, highlighting the flexibility of synthetic matrices that can be modified to best mimic in vivo conditions. In contrast, synthetic matrices are limited by their biocompatibility but can be biofunctionalized with peptides and full-length ECM proteins. Overall, 3D bioprinting and automation has great potential as a future staple for in vitro and ex vivo studies driving drug development and discovery for all cancer types.
Bioreactors have largely been used to study the effects of fluid flow as a physiological mechanical stimulus on cell behavior. In the context of ovarian cancers, mechanotransduction and shear stress from ascites fluid build-up and flow in the peritoneum have been shown to drive ovarian cancer metastasis. Tumor-on-a-chip microfluidics systems have been used to model peritoneal metastases as well as vasculature in solid tumors.
Rotating wall vessel bioreactors or rotary cell culture systems (Figure 2) utilize low shear, low turbulence biomechanical forces to influence cell differentiation and aggregation in three dimensions as a suspension (Schwarz et al., 1992). Originally designed to mimic microgravity, this technique has been important for studies of transcoelomic ovarian cancer metastases (Shield et al., 2009). Microgravity, as a biomechanical force, has been reported to reduce metastatic markers, change cell metabolism and chemosensitivity in a variety of cancer cell lines, though the in vivo effect is unknown (Takeda et al., 2009; Vidyasekar et al., 2015). LN1 cells, derived from a mixed Mullerian tumor, were grown in a horizontally-oriented high aspect rotating vessel (HARV) with microcarrier beads as a scaffold to investigate the potential for metastatic growth as spheroids or aggregates (Becker et al., 1993). This study also showed that there is growth selection for certain cell types from a mixed population in 3D compared to 2D. Further work confirmed the production of chondroitin sulphate in the 3D culture similar to that observed in the in vivo tumor; however, this was not observed in 2D culture (Goodwin et al., 1997; Grun et al., 2009). These spheroids also showed varying degree of oncogene expression not seen in 2D, although the variation was likely due to the mixed populations present. Low passage primary ovarian and endometrial cancer cell lines propagated as multicellular spheroids in a rotating cell culture system, showed similar histological markers to the primary tumor with differentially expressed markers including prohibitin, VDAC1 and annexin 4 identified in 2D versus 3D culture methods (Grun et al., 2009).
Orbital shakers, traditionally used for bacterial cultures, have also been employed to investigate fluid shear stress on ovarian cancer spheroid formation. Masiello and colleagues adapted the orbital shaker to rotate at physiologically relevant speeds and utilized typical culture dishes to investigate the effects of rotational speeds, cell density and well size on spheroid formation (Masiello et al., 2018). This study resulted in more rounded and consistent spheroid formation, amenable to the analyses of functional endpoints. Patient-derived explants of multiple ovarian cancer histotypes have been propagated for up to 30 days in orbital shakers, resulting in maintenance of tumor architectures, epithelial, fibroblasts and immune cells, as well as ECM (Abreu et al., 2020). Studies using these rotational techniques have contributed to the understanding of shear stress on ovarian cancer metastasis, drivers of spheroid formation in ascites and long-term propagation of ovarian cancer cells.
The majority of bioreactor-based models for ovarian cancer have been used for the study of fluid shear stress pressures on cancer invasion and proliferation. Investigation into compressive stress to model external compressive stimuli such as ascites accumulation that increases hydrostatic pressure as a driver of metastasis is a relatively new approach and its effect on treatment is still unknown. Novak and colleagues created a custom bioreactor to mimic the hydrostatic compression pressures on ovarian cancer metastasis from the primary tumor (Novak et al., 2020). This was modelled by the HGSOC cell line OVSAHO that was encapsulated in an agarose-collagen-I hydrogel on top of a membrane where air can be pumped to mimic the compressive forces from ascites fluid build-up in both a static and cyclic manner. This work identified CDC42 as a driver of chemoresistance and proliferation under compressive stimuli. Recently, Onal and colleagues developed a microfluidic platform with micro-pistons that enable the application of dynamic compression to the system, for investigations of cyclic and pressure-controlled compressive stress on SK-OV-3 cell damage (Onal et al., 2021). While this study used ovarian cancer cells as a monolayer, the authors iterate that their platform can be easily modified to include hydrogel ECM and spheroid models.
Tumor-on-a-chip devices utilize microfluidics to simulate effects of vascular flow in solid tumors and more specifically in the context of ovarian cancer, mimicking the hydrodynamics that influence ascitic seeding in the peritoneum and contributing to metastases (Figure 3). Microfluidic devices consist of networks of microchannels where fluids including cell media with cells, or media with drugs or cytokines can be injected and evacuated in an automated manner, enabling nutrient and gas exchange. Microchannels may be laden with matrix and/or cells to mimic a lumen. Culture within a microfluidic system is often short-term and high-throughput.
Tumor-on-a-chip microfluidic systems have been used to model various aspects of ovarian cancer progression, both as solid primary tumors and drivers of cell survival in metastases. Microfluidic chips have been used to model 3D tumor nodule development in the peritoneum by ascitic spheroids, showing that the flow stream was a factor that drove OVCAR-5 cell attachment to Matrigel-laden walls via EMT (Rizvi et al., 2013). Using the same system, SK-OV-3 spheroids were flowed through a poly-HEMA-coated chamber to investigate the effects of shear stress on EMT status changes in cancer spheroids, similarly showing that perfusion promoted spheroid viability and stemness (Ip et al., 2016). These findings were further validated when transplanted into nude mice as xenografts, with perfused spheroids forming larger subcutaneous tumors that were found to be chemoresistant, regulated by the PI3K/AKT signaling pathway. Microfluidic chips have also been used to investigate platelet extravasion in the presence of both primary endothelial cell and ovarian cancer cells, whereby platelets were found to induce cancer cell mediated disruption of the endothelial layer (Saha et al., 2020). This disruption was partially rescued by addition of atorvastatin, a statin drug used for preservation of endothelial junctions, with results reproduced using primary HGSOC cells with prior atorvastatin treatment in vivo. Induction of inflammatory cytokines such as MCP-1 and TNF also mirrored the pro-inflammatory vascular microenvironment observed in the vicinity of ovarian tumors in vivo and inferred some regulation of cancer progression.
Advantages of microfluidic chip systems include the ability to utilize small amounts of starting material, homogenous creation of large numbers of spheroids, portable sizing, and the potential to control drug or chemokine gradients with integration of multiple wells or chips. An orthogonal microfluidic chip developed for seeding into PDMS-based microwells, was superior to standard low-attachment plates and Matrigel seeding in cell viability and maintenance of patient-derived epithelial ovarian cancer cell phenotypes, even when starting with low starting cell numbers (Dadgar et al., 2020). The multi-well microfluidic chip also enabled simpler cytotoxicity measurements by imaging analyses when compared to Matrigel methods, and allowed simultaneous drug gradients in a single microfluidic chip. A direct comparison of spheroid formation and carboplatin sensitivity in four ovarian cancer cell lines in either PDMS-based microfluidic devices with microwells, ULA plates or hanging drop showed microfluidic devices created more homogeneous spheroids with similar carboplatin sensitivities to ULA-plate derived spheroids (Patra et al., 2020). Microfluidic systems have also been used to study macrophage recruitment by ovarian cancer cells via integration of chemokine concentration gradients to mimic macrophage infiltration into the tumor with both HGSOC cell lines and patient-derived xenografts (Scott et al., 2021). The study showed correlation between macrophage recruitment and tumor invasiveness and results were replicated in in vivo PDX models, with future work looking to identify correlations between macrophage infiltration and chemoresistance.
While microfluidic devices have great potential as more physiological and reproducible 3D in vitro models of ovarian cancer, there are still a number of drawbacks to this new technology, namely in costing. There is also a high level of variability between studies due to the majority of devices being fabricated in-house to meet specific researchers’ needs, though commercially available options ranging from single to multichannel perfusion systems are becoming increasingly obtainable, that assists with method standardization.
The concept of a personalized medicine approach where specific treatments are tailored for individuals, is the overarching goal of cancer therapy. As already discussed, ovarian cancer is in fact an umbrella term for multiple histotypes, with differing sites of origin, different genetic and epigenetic events and survival rates. Further, ethnicity and different occurrence rates of histotypes between ethnicities adds another layer of complexity (Peres et al., 2018). Given these variables, homogenous, immortalized 2D cell lines as in vitro models of ovarian cancer only address few of these variables at a single given timepoint of the disease and in a defined microenvironment dissimilar to the in vivo situation. Therefore, there is a need for more representative in vitro and ex vivo models of ovarian cancer for a more personalized prediction of therapeutic efficacy.
There is growing evidence that 2D cultures are more divergent and 3D cultures are better models to reproduce and predict patient drug responses. Patient-derived spheroids grown in 384-well ULA plates derived from primary debulking surgery formed over 24 h and after a further 72 h of growth predicted patient response to first-line adjuvant chemotherapies with 89% accuracy (Shuford et al., 2019). A study by Jabs and colleagues documented that primary ovarian cancers cells grown as organoids had HRD scores, growth and drug cytotoxicity more strongly correlated with the original tumor compared to monolayer cultures, indicative that 3D tumoroid cultures are better mimics of patient tumor behavior (Jabs et al., 2017). Further, numerous studies have shown the utility of ovarian cancer organoids of various histotypes for drug screening, with maintenance of mutational profiles and accurate reproduction of treatment responses when challenged with FDA-approved clinically utilized drugs (Kopper et al., 2019; Maenhoudt et al., 2020; Nanki et al., 2020).
For the prediction of drug response, HGSOC tumor organoids from primary and interval debulking surgeries showed histological concordance with the original tumor and reliably predicted carboplatin sensitivity and resistance (Gorski et al., 2021). RNA sequencing of these tumoroids identified cell-specific pathways that may contribute to carboplatin sensitivity or resistance, and has potential for use in stratification of patients, to guide treatment strategies before clinical recurrence (Gorski et al., 2021). High-throughput development of patient-derived ovarian cancer organoids grown in a ring-shaped geometry of Matrigel, was able to identify responses to 240 kinase inhibitor compounds within a week of cell isolation (Phan et al., 2019). The ring-shaped geometry is particularly advantageous as it eliminates the need for sample transfer or tumoroid dissociation, and can utilize very small starting cell numbers. Further, tumoroids grown by this method were able to maintain heterogeneity with distinct cytomorphologies from mixed type carcinomas. This method could enable rapid screening and identification of clinically actionable drugs as well as identify clinical trial eligibility. Hill and colleagues employed organoids from HGSOC for DNA repair profiling and were able to predict the therapeutic sensitivity in patients to PARP inhibitors. This valuable study highlights the use of organoids as a tool to guide treatment decisions that may have the most benefit (Hill et al., 2018).
Given the high mortality and recurrence rate of ovarian cancers, there is a clear need for more physiologically relevant models that can accurately predict the likelihood that a patient will respond to a particular therapy, both at diagnosis and relapse in order to guide therapeutic strategy. These models will need to be constructed across all of the ovarian cancer histotypes, including those that are very rare, and generate results in a timeframe that facilitates rapid and targeted translation to patients. A vision for the future would encompass automated functional assays of ovarian tumoroids including tumor proliferation, metastatic spread, spheroid formation and cell death that routinely complement molecular studies to provide strong predictions of patient response to new molecular targeted therapies such as described in Figure 4. Furthermore, discovery-based science that will identify new drugs to treat ovarian cancer, will also be conducted in models that more strongly mimic in vivo condition. These studies will not only identify new therapies, but also diagnostic and prognostic markers.
FIGURE 4. A bench-to-bedside approach using 3D cell cultures to fast track personalized therapies for ovarian cancers. Utilization of (A) samples from multiple patient tumor sites, (B) isolation of cancer cells ex vivo for (C) molecular profiling and (D) propagation as 3D cell cultures can identify clues regarding a patient’s unique tumor phenotype. Based on these findings, (E) a high-throughput drug screen of molecularly relevant drugs in 3D cell cultures can be employed to predict drug efficacy and utilized to guide a personalized medicine approach. Created with Biorender.com.
The field of 3D bioprinting, currently in its infancy, is rapidly emerging as an answer to the manual, low throughput methods of creating 3D cell models, including organoids and tumoroids. In the future, the challenges of including common components missing in most 3D in vitro models today of ovarian cancer such as incorporation of vasculature, immune cells and hydrostatic forces will be met as models are created to best recapitulate the patient environment and to better inform the clinical management of women with ovarian cancer.
CY and DJM contributed to all aspects of the article and led the team efforts. CY and K-AD created the tables, and all authors (CY, K-AD, MNM, YM, and DJM) collected the literature, provided revision suggestions, reviewed and commented on the manuscript.
CY was supported by grants to DJM: Medical Research Future Fund (APP1199620) and Cancer Council NSW (2019/TPG1002).
The authors declare that the research was conducted in the absence of any commercial or financial relationships that could be construed as a potential conflict of interest.
All claims expressed in this article are solely those of the authors and do not necessarily represent those of their affiliated organizations, or those of the publisher, the editors, and the reviewers. Any product that may be evaluated in this article, or claim that may be made by its manufacturer, is not guaranteed or endorsed by the publisher.
The Supplementary Material for this article can be found online at: https://www.frontiersin.org/articles/10.3389/fbioe.2022.836984/full#supplementary-material
Abreu, S., Silva, F., Mendes, R., Mendes, T. F., Teixeira, M., Santo, V. E., et al. (2020). Patient-derived Ovarian Cancer Explants: Preserved Viability and Histopathological Features in Long-Term Agitation-Based Cultures. Sci. Rep. 10 (1), 1. doi:10.1038/s41598-020-76291-z
Alkhouli, N., Mansfield, J., Green, E., Bell, J., Knight, B., Liversedge, N., et al. (2013). The Mechanical Properties of Human Adipose Tissues and Their Relationships to the Structure and Composition of the Extracellular Matrix. Am. J. Physiology-Endocrinology Metab. 305 (12), E1427–E1435. doi:10.1152/ajpendo.00111.2013
Alsop, K., Fereday, S., Meldrum, C., deFazio, A., Emmanuel, C., George, J., et al. (2012). BRCAMutation Frequency and Patterns of Treatment Response inBRCAMutation-Positive Women with Ovarian Cancer: A Report from the Australian Ovarian Cancer Study Group. Jco 30 (21), 2654–2663. doi:10.1200/jco.2011.39.8545
Anglesio, M. S., Wiegand, K. C., Melnyk, N., Chow, C., Salamanca, C., Prentice, L. M., et al. (2013). Type-specific Cell Line Models for Type-specific Ovarian Cancer Research. PLoS One 8 (9), e72162. doi:10.1371/journal.pone.0072162
Audeh, M. W., Carmichael, J., Penson, R. T., Friedlander, M., Powell, B., Bell-McGuinn, K. M., et al. (2010). Oral poly(ADP-Ribose) Polymerase Inhibitor Olaparib in Patients with BRCA1 or BRCA2 Mutations and Recurrent Ovarian Cancer: a Proof-Of-Concept Trial. The Lancet 376 (9737), 245–251. doi:10.1016/s0140-6736(10)60893-8
Auguste, A., Blanc-Durand, F., Deloger, M., Le Formal, A., Bareja, R., Wilkes, D. C., et al. (2020). Small Cell Carcinoma of the Ovary, Hypercalcemic Type (SCCOHT) beyond SMARCA4 Mutations: A Comprehensive Genomic Analysis. Cells 9 (6), 1496. doi:10.3390/cells9061496
Barnes, B. M., Nelson, L., Tighe, A., Burghel, G. J., Lin, I.-H., Desai, S., et al. (2021). Distinct Transcriptional Programs Stratify Ovarian Cancer Cell Lines into the Five Major Histological Subtypes. Genome Med. 13 (1), 140. doi:10.1186/s13073-021-00952-5
Beaufort, C. M., Helmijr, J. C. A., Piskorz, A. M., Hoogstraat, M., Ruigrok-Ritstier, K., Besselink, N., et al. (2014). Ovarian Cancer Cell Line Panel (OCCP): Clinical Importance of In Vitro Morphological Subtypes. PLoS ONE 9 (9), e103988. doi:10.1371/journal.pone.0103988
Becker, J. L., Prewett, T. L., Spaulding, G. F., and Goodwin, T. J. (1993). Three-dimensional Growth and Differentiation of Ovarian Tumor Cell Line in High Aspect Rotating-wall Vessel: Morphologic and Embryologic Considerations. J. Cel. Biochem. 51 (3), 283–289. doi:10.1002/jcb.240510307
Behrens, B. C., Hamilton, T. C., Masuda, H., Grotzinger, K. R., Whang-Peng, J., Louie, K. G., et al. (1987). Characterization of a cis-Diamminedichloroplatinum(II)-resistant Human Ovarian Cancer Cell Line and its Use in Evaluation of Platinum Analogues. Cancer Res. 47 (2), 414–418.
Bell, D. (2011). Integrated Genomic Analyses of Ovarian Carcinoma. Nature 474, 609–615. doi:10.1038/nature10166
Bernardo, A. D. M., Thorsteinsdóttir, S., and Mummery, C. L. (2015). Advantages of the Avian Model for Human Ovarian Cancer. Mol. Clin. Oncol. 3 (6), 1191–1198. doi:10.3892/mco.2015.619
Boylan, K. L. M., Manion, R. D., Shah, H., Skubitz, K. M., and Skubitz, A. P. N. (2020). Inhibition of Ovarian Cancer Cell Spheroid Formation by Synthetic Peptides Derived from Nectin-4. Ijms 21 (13), 4637. doi:10.3390/ijms21134637
Brooks, E. A., Gencoglu, M. F., Corbett, D. C., Stevens, K. R., and Peyton, S. R. (2019). An Omentum-Inspired 3D PEG Hydrogel for Identifying ECM-Drivers of Drug Resistant Ovarian Cancer. APL Bioeng. 3 (2), 026106. doi:10.1063/1.5091713
Buechel, M. E., Enserro, D., Burger, R. A., Brady, M. F., Wade, K., Secord, A. A., et al. (2021). Correlation of Imaging and Plasma Based Biomarkers to Predict Response to Bevacizumab in Epithelial Ovarian Cancer (EOC). Gynecol. Oncol. 161 (2), 382–388. doi:10.1016/j.ygyno.2021.02.032
Buick, R. N., Pullano, R., and Trent, J. M. (1985). Comparative Properties of Five Human Ovarian Adenocarcinoma Cell Lines. Cancer Res. 45 (8), 3668–3676.
Burger, R. A., Brady, M. F., Bookman, M. A., Fleming, G. F., Monk, B. J., Huang, H., et al. (2011). Incorporation of Bevacizumab in the Primary Treatment of Ovarian Cancer. N. Engl. J. Med. 365 (26), 2473–2483. doi:10.1056/NEJMoa1104390
Burleson, K. M., Casey, R. C., Skubitz, K. M., Pambuccian, S. E., Oegema, T. R., and Skubitz, A. P. N. (2004). Ovarian Carcinoma Ascites Spheroids Adhere to Extracellular Matrix Components and Mesothelial Cell Monolayers. Gynecol. Oncol. 93 (1), 170–181. doi:10.1016/j.ygyno.2003.12.034
Cai, J., Tang, H., Xu, L., Wang, X., Yang, C., Ruan, S., et al. (2012). Fibroblasts in Omentum Activated by Tumor Cells Promote Ovarian Cancer Growth, Adhesion and Invasiveness. Carcinogenesis 33 (1), 20–29. doi:10.1093/carcin/bgr230
Casagrande, N., Borghese, C., Agostini, F., Durante, C., Mazzucato, M., Colombatti, A., et al. (2021). In Ovarian Cancer Multicellular Spheroids, Platelet Releasate Promotes Growth, Expansion of ALDH+ and CD133+ Cancer Stem Cells, and Protection against the Cytotoxic Effects of Cisplatin, Carboplatin and Paclitaxel. Ijms 22 (6), 3019. doi:10.3390/ijms22063019
Chao, A., Lai, C.-H., Wang, T.-H., Jung, S.-M., Lee, Y.-S., Chang, W.-Y., et al. (2018). Genomic Scar Signatures Associated with Homologous Recombination Deficiency Predict Adverse Clinical Outcomes in Patients with Ovarian clear Cell Carcinoma. J. Mol. Med. 96 (6), 527–536. doi:10.1007/s00109-018-1643-8
Cheasley, D., Nigam, A., Zethoven, M., Hunter, S., Etemadmoghadam, D., Semple, T., et al. (2021). Genomic Analysis of Low‐grade Serous Ovarian Carcinoma to Identify Key Drivers and Therapeutic Vulnerabilities. J. Pathol. 253 (1), 41–54. doi:10.1002/path.5545
Cheasley, D., Wakefield, M. J., Ryland, G. L., Allan, P. E., Alsop, K., Amarasinghe, K. C., et al. (2019). The Molecular Origin and Taxonomy of Mucinous Ovarian Carcinoma. Nat. Commun. 10 (1), 3935. doi:10.1038/s41467-019-11862-x
Cole, A. J., Dwight, T., Gill, A. J., Dickson, K.-A., Zhu, Y., Clarkson, A., et al. (2016). Assessing Mutant P53 in Primary High-Grade Serous Ovarian Cancer Using Immunohistochemistry and Massively Parallel Sequencing. Sci. Rep. 6, 26191. doi:10.1038/srep26191
Colombo, P.-E., du Manoir, S., Orsetti, B., Bras-Gonçalves, R., Lambros, M. B., MacKay, A., et al. (2015). Ovarian Carcinoma Patient Derived Xenografts Reproduce Their Tumor of Origin and Preserve an Oligoclonal Structure. Oncotarget 6 (29), 28327–28340. doi:10.18632/oncotarget.5069
Conover, C. A., Hartmann, L. C., Bradley, S., Stalboerger, P., Klee, G. G., Kalli, K. R., et al. (1998). Biological Characterization of Human Epithelial Ovarian Carcinoma Cells in Primary Culture: the Insulin-like Growth Factor System. Exp. Cell Res. 238 (2), 439–449. doi:10.1006/excr.1997.3861
Crum, C. P., Drapkin, R., Kindelberger, D., Medeiros, F., Miron, A., and Lee, Y. (2007). Lessons from BRCA: the Tubal Fimbria Emerges as an Origin for Pelvic Serous Cancer. Clin. Med. Res. 5 (1), 35–44. doi:10.3121/cmr.2007.702
Dadgar, N., Gonzalez-Suarez, A. M., Fattahi, P., Hou, X., Weroha, J. S., Gaspar-Maia, A., et al. (2020). A Microfluidic Platform for Cultivating Ovarian Cancer Spheroids and Testing Their Responses to Chemotherapies. Microsyst Nanoeng 6 (1), 1. doi:10.1038/s41378-020-00201-6
Davies, B., Steele, I. A., Edmondson, R. J., Zwolinski, S. A., Saretzki, G., von Zglinicki, T., et al. (2003). Immortalisation of Human Ovarian Surface Epithelium with Telomerase and Temperature-Senstitive SV40 Large T Antigen. Exp. Cell Res. 288 (2), 390–402. doi:10.1016/s0014-4827(03)00218-0
Davis, A., Tinker, A. V., and Friedlander, M. (2014). "Platinum Resistant" Ovarian Cancer: What Is it, Who to Treat and How to Measure Benefit? Gynecol. Oncol. 133 (3), 624–631. doi:10.1016/j.ygyno.2014.02.038
De Haven Brandon, A., Box, G., Hallsworth, A., Court, W., Matthews, N., Herodek, B., et al. (2020). Identification of Ovarian High-Grade Serous Carcinoma Cell Lines that Show Estrogen-Sensitive Growth as Xenografts in Immunocompromised Mice. Sci. Rep. 10 (1), 1. doi:10.1038/s41598-020-67533-1
De Paolis, E., Paragliola, R. M., and Concolino, P. (2021). Spectrum of DICER1 Germline Pathogenic Variants in Ovarian Sertoli-Leydig Cell Tumor. Jcm 10 (9), 1845. doi:10.3390/jcm10091845
De Pauw, A., Naert, E., Van de Vijver, K., Philippe, T., Vandecasteele, K., and Denys, H. (2021). A Clearer View on Ovarian Clear Cell Carcinoma. Acta Clinica Belgica 17, 1–13. doi:10.1080/17843286.2021.1964051
De Thaye, E., Van de Vijver, K., Van der Meulen, J., Taminau, J., Wagemans, G., Denys, H., et al. (2020). Establishment and Characterization of a Cell Line and Patient-Derived Xenograft (PDX) from Peritoneal Metastasis of Low-Grade Serous Ovarian Carcinoma. Sci. Rep. 10 (1), 6688. doi:10.1038/s41598-020-63738-6
De Witte, C. J., Espejo Valle-Inclan, J., Hami, N., Lõhmussaar, K., Kopper, O., Vreuls, C. P. H., et al. (2020). Patient-Derived Ovarian Cancer Organoids Mimic Clinical Response and Exhibit Heterogeneous Inter- and Intrapatient Drug Responses. Cell Rep. 31 (11), 107762. doi:10.1016/j.celrep.2020.107762
Dedes, K. J., Wilkerson, P. M., Wetterskog, D., Weigelt, B., Ashworth, A., and Reis-Filho, J. S. (2011). Synthetic Lethality of PARP Inhibition in Cancers lackingBRCA1andBRCA2mutations. Cell Cycle 10 (8), 1192–1199. doi:10.4161/cc.10.8.15273
DelloRusso, C., Welcsh, P. L., Wang, W., Garcia, R. L., King, M.-C., and Swisher, E. M. (2007). Functional Characterization of a Novel BRCA1-Null Ovarian Cancer Cell Line in Response to Ionizing Radiation. Mol. Cancer Res. 5 (1), 35–45. doi:10.1158/1541-7786.Mcr-06-0234
Dickson, K.-A., Xie, T., Evenhuis, C., Ma, Y., and Marsh, D. J. (2021). PARP Inhibitors Display Differential Efficacy in Models of BRCA Mutant High-Grade Serous Ovarian Cancer. Ijms 22 (16), 8506. doi:10.3390/ijms22168506
Domcke, S., Sinha, R., Levine, D. A., Sander, C., and Schultz, N. (2013). Evaluating Cell Lines as Tumour Models by Comparison of Genomic Profiles. Nat. Commun. 4, 2126. doi:10.1038/ncomms3126
Donaldson, A. R., Tanase, C. E., Awuah, D., Vasanthi Bathrinarayanan, P., Hall, L., Nikkhah, M., et al. (2018). Photocrosslinkable Gelatin Hydrogels Modulate the Production of the Major Pro-inflammatory Cytokine, TNF-α, by Human Mononuclear Cells. Front. Bioeng. Biotechnol. 6 (116), 1. doi:10.3389/fbioe.2018.00116
Dong, R., Qiang, W., Guo, H., Xu, X., Kim, J. J., Mazar, A., et al. (2016). Histologic and Molecular Analysis of Patient Derived Xenografts of High-Grade Serous Ovarian Carcinoma. J. Hematol. Oncol. 9 (1), 92. doi:10.1186/s13045-016-0318-6
Evans, C. L., Abu-Yousif, A. O., Park, Y. J., Klein, O. J., Celli, J. P., Rizvi, I., et al. (2011). Killing Hypoxic Cell Populations in a 3D Tumor Model with EtNBS-PDT. PLoS ONE 6 (8), e23434. doi:10.1371/journal.pone.0023434
Fan, R., Piou, M., Darling, E., Cormier, D., Sun, J., and Wan, J. (2016). Bio-printing Cell-Laden Matrigel-Agarose Constructs. J. Biomater. Appl. 31 (5), 684–692. doi:10.1177/0885328216669238
Flont, M., Jastrzębska, E., and Brzózka, Z. (2020). A Multilayered Cancer-On-A-Chip Model to Analyze the Effectiveness of New-Generation Photosensitizers. Analyst 145 (21), 6937–6947. doi:10.1039/D0AN00911C
Florczyk, S. J., Kievit, F. M., Wang, K., Erickson, A. E., Ellenbogen, R. G., and Zhang, M. (2016). 3D Porous Chitosan-Alginate Scaffolds Promote Proliferation and Enrichment of Cancer Stem-like Cells. J. Mater. Chem. B 4 (38), 6326–6334. doi:10.1039/C6TB01713D
Ford, C. E., Werner, B., Hacker, N. F., and Warton, K. (2020). The Untapped Potential of Ascites in Ovarian Cancer Research and Treatment. Br. J. Cancer 123 (1), 9–16. doi:10.1038/s41416-020-0875-x
Fujisawa, T., Joshi, B. H., and Puri, R. K. (2012). IL-13 Regulates Cancer Invasion and Metastasis through IL-13Rα2 via ERK/AP-1 Pathway in Mouse Model of Human Ovarian Cancer. Int. J. Cancer 131 (2), 344–356. doi:10.1002/ijc.26366
Fuller, P. J., Leung, D., and Chu, S. (2017). Genetics and Genomics of Ovarian Sex Cord-Stromal Tumors. Clin. Genet. 91 (2), 285–291. doi:10.1111/cge.12917
Ganguli, A., Mostafa, A., Saavedra, C., Kim, Y., Le, P., Faramarzi, V., et al. (2021). Three-dimensional Microscale Hanging Drop Arrays with Geometric Control for Drug Screening and Live Tissue Imaging. Sci. Adv. 7 (17), eabc1323. doi:10.1126/sciadv.abc1323
Gao, J., Li, F., Liu, Z., Huang, M., Chen, H., Liao, G., et al. (2021). Multiple Genetic Variants Predict the Progression-free Survival of Bevacizumab Plus Chemotherapy in Advanced Ovarian Cancer. Medicine (Baltimore) 100 (35), e27130. doi:10.1097/md.0000000000027130
George, E., Kim, H., Krepler, C., Wenz, B., Makvandi, M., Tanyi, J. L., et al. (2017). A Patient-Derived-Xenograft Platform to Study BRCA-Deficient Ovarian Cancers. JCI Insight 2 (1), 1. doi:10.1172/jci.insight.89760
Gombotz, W., and Wee, S. (1998). Protein Release from Alginate Matrices. Adv. Drug Deliv. Rev. 31 (3), 267–285. doi:10.1016/S0169-409X(97)00124-5
Goodwin, T. J., Prewett, T. L., Spaulding, G. F., and Becker, J. L. (1997). Three-dimensional Culture of a Mixed Mullerian Tumor of the Ovary: Expression of In Vivo Characteristics. In Vitro Cell.Dev.Biol.-Animal 33 (5), 366–374. doi:10.1007/s11626-997-0007-4
Gorai, I., Nakazawa, T., Miyagi, E., Hirahara, F., Nagashima, Y., and Minaguchi, H. (1995). Establishment and Characterization of Two Human Ovarian clear Cell Adenocarcinoma Lines from Metastatic Lesions with Different Properties. Gynecol. Oncol. 57 (1), 33–46. doi:10.1006/gyno.1995.1097
Gorski, J. W., Zhang, Z., McCorkle, J. R., DeJohn, J. M., Wang, C., Miller, R. W., et al. (2021). Utilizing Patient-Derived Epithelial Ovarian Cancer Tumor Organoids to Predict Carboplatin Resistance. Biomedicines 9 (8), 1021. doi:10.3390/biomedicines9081021
Graf, B. W., and Boppart, S. A. (2010). Imaging and Analysis of Three-Dimensional Cell Culture Models. Methods Mol. Biol. (Clifton, N.J.) 591, 211–227. doi:10.1007/978-1-60761-404-3_13
Greenaway, J., Moorehead, R., Shaw, P., and Petrik, J. (2008). Epithelial-stromal Interaction Increases Cell Proliferation, Survival and Tumorigenicity in a Mouse Model of Human Epithelial Ovarian Cancer. Gynecol. Oncol. 108 (2), 385–394. doi:10.1016/j.ygyno.2007.10.035
Grun, B., Benjamin, E., Sinclair, J., Timms, J. F., Jacobs, I. J., Gayther, S. A., et al. (2009). Three-dimensionalin Vitrocell Biology Models of Ovarian and Endometrial Cancer. Cell Prolif. 42 (2), 219–228. doi:10.1111/j.1365-2184.2008.00579.x
Gurler, H., Yu, Y., Choi, J., Kajdacsy-Balla, A., and Barbolina, M. (2015). Three-Dimensional Collagen Type I Matrix Up-Regulates Nuclear Isoforms of the Microtubule Associated Protein Tau Implicated in Resistance to Paclitaxel Therapy in Ovarian Carcinoma. Ijms 16 (2), 3419–3433. doi:10.3390/ijms16023419
Hallas-Potts, A., Dawson, J. C., and Herrington, C. S. (2019). Ovarian Cancer Cell Lines Derived from Non-serous Carcinomas Migrate and Invade More Aggressively Than Those Derived from High-Grade Serous Carcinomas. Sci. Rep. 9 (1), 1. doi:10.1038/s41598-019-41941-4
Hamilton, T. C., Young, R. C., McKoy, W. M., Grotzinger, K. R., Green, J. A., Chu, E. W., et al. (1983). Characterization of a Human Ovarian Carcinoma Cell Line (NIH:OVCAR-3) with Androgen and Estrogen Receptors. Cancer Res. 43 (11), 5379–5389.
Harris, M. A., Delap, L. M., Sengupta, P. S., Wilkinson, P. M., Welch, R. S., Swindell, R., et al. (2003). Carcinosarcoma of the Ovary. Br. J. Cancer 88 (5), 654–657. doi:10.1038/sj.bjc.6600770
Hedegaard, C. L., Redondo-Gómez, C., Tan, B. Y., Ng, K. W., Loessner, D., and Mata, A. (2020). Peptide-protein Coassembling Matrices as a Biomimetic 3D Model of Ovarian Cancer. Sci. Adv. 6 (40), eabb3298. doi:10.1126/sciadv.abb3298
Henry, C., Hacker, N., and Ford, C. (2017). Silencing ROR1 and ROR2 Inhibits Invasion and Adhesion in an Organotypic Model of Ovarian Cancer Metastasis. Oncotarget 8 (68), 112727–112738. doi:10.18632/oncotarget.22559
Heo, E. J., Cho, Y. J., Cho, W. C., Hong, J. E., Jeon, H.-K., Oh, D.-Y., et al. (2017). Patient-Derived Xenograft Models of Epithelial Ovarian Cancer for Preclinical Studies. Cancer Res. Treat. 49 (4), 915–926. doi:10.4143/crt.2016.322
Heredia-Soto, V., Redondo, A., Berjón, A., Miguel-Martín, M., Díaz, E., Crespo, R., et al. (2018). High-throughput 3-dimensional Culture of Epithelial Ovarian Cancer Cells as Preclinical Model of Disease. Oncotarget 9 (31), 21893–21903. doi:10.18632/oncotarget.25098
Hernandez, L., Kim, M. K., Lyle, L. T., Bunch, K. P., House, C. D., Ning, F., et al. (2016). Characterization of Ovarian Cancer Cell Lines as In Vivo Models for Preclinical Studies. Gynecol. Oncol. 142 (2), 332–340. doi:10.1016/j.ygyno.2016.05.028
Hidalgo, M., Amant, F., Biankin, A. V., Budinská, E., Byrne, A. T., Caldas, C., et al. (2014). Patient-derived Xenograft Models: an Emerging Platform for Translational Cancer Research. Cancer Discov. 4 (9), 998–1013. doi:10.1158/2159-8290.Cd-14-0001
Hill, S. J., Decker, B., Roberts, E. A., Horowitz, N. S., Muto, M. G., Worley, M. J., et al. (2018). Prediction of DNA Repair Inhibitor Response in Short-Term Patient-Derived Ovarian Cancer Organoids. Cancer Discov. 8 (11), 1404–1421. doi:10.1158/2159-8290.Cd-18-0474
Hoffmann, K., Berger, H., Kulbe, H., Thillainadarasan, S., Mollenkopf, H. J., Zemojtel, T., et al. (2020). Stable Expansion of High‐grade Serous Ovarian Cancer Organoids Requires a low‐Wnt Environment. Embo J. 39 (6), 1. doi:10.15252/embj.2019104013
Hollis, R. L., Thomson, J. P., Stanley, B., Churchman, M., Meynert, A. M., Rye, T., et al. (2020). Molecular Stratification of Endometrioid Ovarian Carcinoma Predicts Clinical Outcome. Nat. Commun. 11 (1), 4995. doi:10.1038/s41467-020-18819-5
Hopkins, T. I. R., Bemmer, V. L., Franks, S., Dunlop, C., Hardy, K., and Dunlop, I. E. (2021). Micromechanical Mapping of the Intact Ovary interior Reveals Contrasting Mechanical Roles for Follicles and Stroma. Biomaterials 277, 121099. doi:10.1016/j.biomaterials.2021.121099
Hsu, P.-C., Chao, T.-K., Chou, Y.-C., Yu, M.-H., Wang, Y.-C., Lin, Y.-H., et al. (2021). AIM2 Inflammasome in Tumor Cells as a Biomarker for Predicting the Treatment Response to Antiangiogenic Therapy in Epithelial Ovarian Cancer Patients. Jcm 10 (19), 4529. doi:10.3390/jcm10194529
Hughes, C. S., Postovit, L. M., and Lajoie, G. A. (2010). Matrigel: a Complex Protein Mixture Required for Optimal Growth of Cell Culture. Proteomics 10 (9), 1886–1890. doi:10.1002/pmic.200900758
Ip, C. K. M., Li, S.-S., Tang, M. Y. H., Sy, S. K. H., Ren, Y., Shum, H. C., et al. (2016). Stemness and Chemoresistance in Epithelial Ovarian Carcinoma Cells under Shear Stress. Sci. Rep. 6 (1), 26788. doi:10.1038/srep26788
Ivascu, A., and Kubbies, M. (2006). Rapid Generation of Single-Tumor Spheroids for High-Throughput Cell Function and Toxicity Analysis. J. Biomol. Screen. 11 (8), 922–932. doi:10.1177/1087057106292763
Iwanicki, M. P., Davidowitz, R. A., Ng, M. R., Besser, A., Muranen, T., Merritt, M., et al. (2011). Ovarian Cancer Spheroids Use Myosin-Generated Force to Clear the Mesothelium. Cancer Discov. 1 (2), 144–157. doi:10.1158/2159-8274.cd-11-0010
Iyer, S., Zhang, S., Yucel, S., Horn, H., Smith, S. G., Reinhardt, F., et al. (2021). Genetically Defined Syngeneic Mouse Models of Ovarian Cancer as Tools for the Discovery of Combination Immunotherapy. Cancer Discov. 11 (2), 384–407. doi:10.1158/2159-8290.CD-20-0818
Jabs, J., Zickgraf, F. M., Park, J., Wagner, S., Jiang, X., Jechow, K., et al. (2017). Screening Drug Effects in Patient‐derived Cancer Cells Links Organoid Responses to Genome Alterations. Mol. Syst. Biol. 13 (11), 955. doi:10.15252/msb.20177697
Jamieson, S., and Fuller, P. J. (2012). Molecular Pathogenesis of Granulosa Cell Tumors of the Ovary. Endocr. Rev. 33 (1), 109–144. doi:10.1210/er.2011-0014
Jelinic, P., Schlappe, B. A., Conlon, N., Tseng, J., Olvera, N., Dao, F., et al. (2016). Concomitant Loss of SMARCA2 and SMARCA4 Expression in Small Cell Carcinoma of the Ovary, Hypercalcemic Type. Mod. Pathol. 29 (1), 60–66. doi:10.1038/modpathol.2015.129
Jensen, C., and Teng, Y. (2020). Is it Time to Start Transitioning from 2D to 3D Cell Culture? Front. Mol. Biosci. 7 (33), 1. doi:10.3389/fmolb.2020.00033
Jin, K., Teng, L., Shen, Y., He, K., Xu, Z., and Li, G. (2010). Patient-derived Human Tumour Tissue Xenografts in Immunodeficient Mice: a Systematic Review. Clin. Transl Oncol. 12 (7), 473–480. doi:10.1007/s12094-010-0540-6
John, B., Naczki, C., Patel, C., Ghoneum, A., Qasem, S., Salih, Z., et al. (2019). Regulation of the Bi-directional Cross-Talk between Ovarian Cancer Cells and Adipocytes by SPARC. Oncogene 38 (22), 4366–4383. doi:10.1038/s41388-019-0728-3
Johnson, P. A., and Giles, J. R. (2013). The Hen as a Model of Ovarian Cancer. Nat. Rev. Cancer 13 (6), 432–436. doi:10.1038/nrc3535
Joshi, N., Liu, D., Dickson, K.-A., Marsh, D. J., Ford, C. E., and Stenzel, M. H. (2021). An Organotypic Model of High-Grade Serous Ovarian Cancer to Test the Anti-metastatic Potential of ROR2 Targeted Polyion Complex Nanoparticles. J. Mater. Chem. B 9 (44), 9123–9135. doi:10.1039/d1tb01837j
Kaemmerer, E., Melchels, F. P. W., Holzapfel, B. M., Meckel, T., Hutmacher, D. W., and Loessner, D. (2014). Gelatine Methacrylamide-Based Hydrogels: An Alternative Three-Dimensional Cancer Cell Culture System. Acta Biomater. 10 (6), 2551–2562. doi:10.1016/j.actbio.2014.02.035
Kalli, K. R., Chen, B.-K., Bale, L. K., Gernand, E., Overgaard, M. T., Oxvig, C., et al. (2004). Pregnancy-associated Plasma Protein-A (PAPP-A) Expression and Insulin-like Growth Factor Binding Protein-4 Protease Activity in normal and Malignant Ovarian Surface Epithelial Cells. Int. J. Cancer 110 (5), 633–640. doi:10.1002/ijc.20185
Kapałczyńska, M., Kolenda, T., Przybyła, W., Zajączkowska, M., Teresiak, A., Filas, V., et al. (2018). 2D and 3D Cell Cultures - a Comparison of Different Types of Cancer Cell Cultures. aoms 14 (4), 910–919. doi:10.5114/aoms.2016.63743
Karnezis, A. N., Chen, S. Y., Chow, C., Yang, W., Hendricks, W. P. D., Ramos, P., et al. (2021). Re-assigning the Histologic Identities of COV434 and TOV-112D Ovarian Cancer Cell Lines. Gynecol. Oncol. 160 (2), 568–578. doi:10.1016/j.ygyno.2020.12.004
Karst, A. M., Levanon, K., and Drapkin, R. (2011). Modeling High-Grade Serous Ovarian Carcinogenesis from the Fallopian Tube. Proc. Natl. Acad. Sci. 108 (18), 7547–7552. doi:10.1073/pnas.1017300108
Kashiyama, T., Oda, K., Ikeda, Y., Shiose, Y., Hirota, Y., Inaba, K., et al. (2014). Antitumor Activity and Induction of TP53-dependent Apoptosis toward Ovarian clear Cell Adenocarcinoma by the Dual PI3K/mTOR Inhibitor DS-7423. PLoS ONE 9 (2), e87220. doi:10.1371/journal.pone.0087220
Kenny, H. A., Chiang, C.-Y., White, E. A., Schryver, E. M., Habis, M., Romero, I. L., et al. (2014). Mesothelial Cells Promote Early Ovarian Cancer Metastasis through Fibronectin Secretion. J. Clin. Invest. 124 (10), 4614–4628. doi:10.1172/jci74778
Kenny, H. A., Dogan, S., Zillhardt, M., Mitra, A. A., Yamada, S. D., Krausz, T., et al. (2009). “Organotypic Models of Metastasis: A Three-Dimensional Culture Mimicking the Human Peritoneum and Omentum for the Study of the Early Steps of Ovarian Cancer Metastasis,” in Cancer Treatment and Research (US: Springer), 335–351. doi:10.1007/978-0-387-98094-2_16
Kenny, H. A., Krausz, T., Yamada, S. D., and Lengyel, E. (2007). Use of a Novel 3D Culture Model to Elucidate the Role of Mesothelial Cells, Fibroblasts and Extra-cellular Matrices on Adhesion and Invasion of Ovarian Cancer Cells to the Omentum. Int. J. Cancer 121 (7), 1463–1472. doi:10.1002/ijc.22874
Khalil, C., Moussa, M., Azar, A., Tawk, J., Habbouche, J., Salameh, R., et al. (2019). Anti-proliferative Effects of Mesenchymal Stem Cells (MSCs) Derived from Multiple Sources on Ovarian Cancer Cell Lines: an In-Vitro Experimental Study. J. Ovarian Res. 12 (1), 70. doi:10.1186/s13048-019-0546-9
Kidera, Y., Yoshimura, T., Ohkuma, Y., Iwasaka, T., and Sugimori, H. (1985). Establishment and Characterization of a Cell Line Derived from Mucinous Cystadenocarcinoma of Human Ovary. Nihon Sanka Fujinka Gakkai Zasshi 37 (9), 1820–1824.
Kim, S. I., Lee, J. W., Lee, M., Kim, H. S., Chung, H. H., Kim, J.-W., et al. (2018). Genomic Landscape of Ovarian clear Cell Carcinoma via Whole Exome Sequencing. Gynecol. Oncol. 148 (2), 375–382. doi:10.1016/j.ygyno.2017.12.005
King, S. M., Quartuccio, S., Hilliard, T. S., Inoue, K., and Burdette, J. E. (2011). Alginate Hydrogels for Three-Dimensional Organ Culture of Ovaries and Oviducts. JoVE 52, 1. doi:10.3791/2804
Köbel, M., and Kang, E. Y. (2021). The Many Uses of P53 Immunohistochemistry in Gynecological Pathology: Proceedings of the ISGyP Companion Society Session at the 2020 USCAP Annual9 Meeting. Int. J. Gynecol. Pathol. 40 (1), 32–40. doi:10.1097/pgp.0000000000000725
Kondrashova, O., Nguyen, M., Shield-Artin, K., Tinker, A. V., Teng, N. N. H., Harrell, M. I., et al. (2017). Secondary Somatic Mutations Restoring RAD51C and RAD51D Associated with Acquired Resistance to the PARP Inhibitor Rucaparib in High-Grade Ovarian Carcinoma. Cancer Discov. 7 (9), 984–998. doi:10.1158/2159-8290.Cd-17-0419
Konstantinopoulos, P. A., Spentzos, D., Karlan, B. Y., Taniguchi, T., Fountzilas, E., Francoeur, N., et al. (2010). Gene Expression Profile ofBRCAness that Correlates with Responsiveness to Chemotherapy and with Outcome in Patients with Epithelial Ovarian Cancer. Jco 28 (22), 3555–3561. doi:10.1200/jco.2009.27.5719
Kopper, O., de Witte, C. J., Lõhmussaar, K., Valle-Inclan, J. E., Hami, N., Kester, L., et al. (2019). An Organoid Platform for Ovarian Cancer Captures Intra- and Interpatient Heterogeneity. Nat. Med. 25 (5), 838–849. doi:10.1038/s41591-019-0422-6
Kuo, K.-T., Mao, T.-L., Jones, S., Veras, E., Ayhan, A., Wang, T.-L., et al. (2009). Frequent Activating Mutations of PIK3CA in Ovarian clear Cell Carcinoma. Am. J. Pathol. 174 (5), 1597–1601. doi:10.2353/ajpath.2009.081000
Kunz-Schughart, L. A., Freyer, J. P., Hofstaedter, F., and Ebner, R. (2004). The Use of 3-D Cultures for High-Throughput Screening: The Multicellular Spheroid Model. J. Biomol. Screen. 9 (4), 273–285. doi:10.1177/1087057104265040
Kurman, R. J., and Shih, I.-M. (2010). The Origin and Pathogenesis of Epithelial Ovarian Cancer: a Proposed Unifying Theory. Am. J. Surg. Pathol. 34 (3), 433–443. doi:10.1097/PAS.0b013e3181cf3d79
Lal-Nag, M., McGee, L., Guha, R., Lengyel, E., Kenny, H. A., and Ferrer, M. (2017). A High-Throughput Screening Model of the Tumor Microenvironment for Ovarian Cancer Cell Growth. SLAS DISCOVERY: Advancing Sci. Drug Discov. 22 (5), 494–506. doi:10.1177/2472555216687082
Langdon, S. P., Lawrie, S. S., Hay, F. G., Hawkes, M. M., McDonald, A., Hayward, I. P., et al. (1988). Characterization and Properties of Nine Human Ovarian Adenocarcinoma Cell Lines. Cancer Res. 48 (21), 6166–6172.
Lau, T.-S., Chan, L. K.-Y., Wong, E. C.-H., Hui, C. W.-C., Sneddon, K., Cheung, T.-H., et al. (2017). A Loop of Cancer-Stroma-Cancer Interaction Promotes Peritoneal Metastasis of Ovarian Cancer via TNFα-Tgfα-EGFR. Oncogene 36 (25), 3576–3587. doi:10.1038/onc.2016.509
Lawrenson, K., Grun, B., and Gayther, S. A. (2012). Heterotypic Three-Dimensional In Vitro Modeling of Stromal-Epithelial Interactions during Ovarian Cancer Initiation and Progression. JoVE 66, e4206. doi:10.3791/4206
Ledermann, J. A., Luvero, D., Shafer, A., O’Connor, D., Mangili, G., Friedlander, M., et al. (2014b). Gynecologic Cancer InterGroup (GCIG) Consensus Review for Mucinous Ovarian Carcinoma. Int. J. Gynecol. Cancer 24 (9), S14–S19. doi:10.1097/igc.0000000000000296
Ledermann, J., Harter, P., Gourley, C., Friedlander, M., Vergote, I., Rustin, G., et al. (2014a). Olaparib Maintenance Therapy in Patients with Platinum-Sensitive Relapsed Serous Ovarian Cancer: a Preplanned Retrospective Analysis of Outcomes by BRCA Status in a Randomised Phase 2 Trial. Lancet Oncol. 15 (8), 852–861. doi:10.1016/s1470-2045(14)70228-1
Ledermann, J., Harter, P., Gourley, C., Friedlander, M., Vergote, I., Rustin, G., et al. (2012). Olaparib Maintenance Therapy in Platinum-Sensitive Relapsed Ovarian Cancer. N. Engl. J. Med. 366 (15), 1382–1392. doi:10.1056/NEJMoa1105535
Lee, C. K., Friedlander, M. L., Tjokrowidjaja, A., Ledermann, J. A., Coleman, R. L., Mirza, M. R., et al. (2021). Molecular and Clinical Predictors of Improvement in Progression‐free Survival with Maintenance PARP Inhibitor Therapy in Women with Platinum‐sensitive, Recurrent Ovarian Cancer: A Meta‐analysis. Cancer 127 (14), 2432–2441. doi:10.1002/cncr.33517
Li, S.-S., Ip, C. K. M., Tang, M. Y. H., Sy, S. K. H., Yung, S., Chan, T.-M., et al. (2017). Modeling Ovarian Cancer Multicellular Spheroid Behavior in a Dynamic 3D Peritoneal Microdevice. JoVE 120, 55337. doi:10.3791/55337
Liao, J., Qian, F., Tchabo, N., Mhawech-Fauceglia, P., Beck, A., Qian, Z., et al. (2014). Ovarian Cancer Spheroid Cells with Stem Cell-like Properties Contribute to Tumor Generation, Metastasis and Chemotherapy Resistance through Hypoxia-Resistant Metabolism. PLoS ONE 9 (1), e84941. doi:10.1371/journal.pone.0084941
Liu, J. F., Palakurthi, S., Zeng, Q., Zhou, S., Ivanova, E., Huang, W., et al. (2017). Establishment of Patient-Derived Tumor Xenograft Models of Epithelial Ovarian Cancer for Preclinical Evaluation of Novel Therapeutics. Clin. Cancer Res. 23 (5), 1263–1273. doi:10.1158/1078-0432.Ccr-16-1237
Liu, M., Zhang, X., Long, C., Xu, H., Cheng, X., Chang, J., et al. (2018). Collagen-based Three-Dimensional Culture Microenvironment Promotes Epithelial to Mesenchymal Transition and Drug Resistance of Human Ovarian Cancerin Vitro. RSC Adv. 8 (16), 8910–8919. doi:10.1039/C7RA13742G
Loessner, D., Rockstroh, A., Shokoohmand, A., Holzapfel, B. M., Wagner, F., Baldwin, J., et al. (2019). A 3D Tumor Microenvironment Regulates Cell Proliferation, Peritoneal Growth and Expression Patterns. Biomaterials 190-191, 63–75. doi:10.1016/j.biomaterials.2018.10.014
Lõhmussaar, K., Kopper, O., Korving, J., Begthel, H., Vreuls, C. P. H., Van Es, J. H., et al. (2020). Assessing the Origin of High-Grade Serous Ovarian Cancer Using CRISPR-Modification of Mouse Organoids. Nat. Commun. 11 (1), 1. doi:10.1038/s41467-020-16432-0
Long, L., Yin, M., and Min, W. (2018). 3D Co-culture System of Tumor-Associated Macrophages and Ovarian Cancer Cells. Bio-protocol 8 (8), e2815. doi:10.21769/BioProtoc.2815
Lu, M., Henry, C. E., Lai, H., Khine, Y. Y., Ford, C. E., and Stenzel, M. H. (2019). A New 3D Organotypic Model of Ovarian Cancer to Help Evaluate the Antimetastatic Activity of RAPTA-C Conjugated Micelles. Biomater. Sci. 7 (4), 1652–1660. doi:10.1039/c8bm01326h
Lutolf, M. P., Lauer-Fields, J. L., Schmoekel, H. G., Metters, A. T., Weber, F. E., Fields, G. B., et al. (2003). Synthetic Matrix Metalloproteinase-Sensitive Hydrogels for the Conduction of Tissue Regeneration: Engineering Cell-Invasion Characteristics. Proc. Natl. Acad. Sci. 100 (9), 5413–5418. doi:10.1073/pnas.0737381100
Lynn, A. K., Yannas, I. V., and Bonfield, W. (2004). Antigenicity and Immunogenicity of Collagen. J. Biomed. Mater. Res. 71B (2), 343–354. doi:10.1002/jbm.b.30096
Mabuchi, S., Sugiyama, T., and Kimura, T. (2016). Clear Cell Carcinoma of the Ovary: Molecular Insights and Future Therapeutic Perspectives. J. Gynecol. Oncol. 27 (3), e31. doi:10.3802/jgo.2016.27.e31
Machida-Sano, I., Matsuda, Y., and Namiki, H. (2009). In Vitroadhesion of Human Dermal Fibroblasts on Iron Cross-Linked Alginate Films. Biomed. Mater. 4, 025008. doi:10.1088/1748-6041/4/2/025008
Maenhoudt, N., Defraye, C., Boretto, M., Jan, Z., Heremans, R., Boeckx, B., et al. (2020). Developing Organoids from Ovarian Cancer as Experimental and Preclinical Models. Stem Cell Rep. 14 (4), 717–729. doi:10.1016/j.stemcr.2020.03.004
Maenhoudt, N., and Vankelecom, H. (2021). Protocol for Establishing Organoids from Human Ovarian Cancer Biopsies. STAR Protoc. 2 (2), 100429. doi:10.1016/j.xpro.2021.100429
Makałowski, W., Zhang, J., and Boguski, M. S. (1996). Comparative Analysis of 1196 Orthologous Mouse and Human Full-Length mRNA and Protein Sequences. Genome Res. 6 (9), 846–857. doi:10.1101/gr.6.9.846
Maniati, E., Berlato, C., Gopinathan, G., Heath, O., Kotantaki, P., Lakhani, A., et al. (2020). Mouse Ovarian Cancer Models Recapitulate the Human Tumor Microenvironment and Patient Response to Treatment. Cell Rep. 30 (2), 525–540. e527. doi:10.1016/j.celrep.2019.12.034
Maru, Y., Tanaka, N., Itami, M., and Hippo, Y. (2019). Efficient Use of Patient-Derived Organoids as a Preclinical Model for Gynecologic Tumors. Gynecol. Oncol. 154 (1), 189–198. doi:10.1016/j.ygyno.2019.05.005
Masiello, T., Dhall, A., Hemachandra, L., Tokranova, N., Melendez, J., and Castracane, J. (2018). A Dynamic Culture Method to Produce Ovarian Cancer Spheroids under Physiologically-Relevant Shear Stress. Cells 7 (12), 277. doi:10.3390/cells7120277
Matsuo, K., Nishimura, M., Bottsford-Miller, J. N., Huang, J., Komurov, K., Armaiz-Pena, G. N., et al. (2011). Targeting SRC in Mucinous Ovarian Carcinoma. Clin. Cancer Res. 17 (16), 5367–5378. doi:10.1158/1078-0432.Ccr-10-3176
Mazzocchi, A., Soker, S., and Skardal, A. (2019). 3D Bioprinting for High-Throughput Screening: Drug Screening, Disease Modeling, and Precision Medicine Applications. Appl. Phys. Rev. 6 (1), 011302. doi:10.1063/1.5056188
McCloskey, C. W., Goldberg, R. L., Carter, L. E., Gamwell, L. F., Al-Hujaily, E. M., Collins, O., et al. (2014). A New Spontaneously Transformed Syngeneic Model of High-Grade Serous Ovarian Cancer with a Tumor-Initiating Cell Population. Front. Oncol. 4 (53), 1. doi:10.3389/fonc.2014.00053
McCluggage, W. G., and Stewart, C. J. R. (2021). SWI/SNF-deficient Malignancies of the Female Genital Tract. Semin. Diagn. Pathol. 38 (3), 199–211. doi:10.1053/j.semdp.2020.08.003
Mckenzie, A. J., Hicks, S. R., Svec, K. V., Naughton, H., Edmunds, Z. L., and Howe, A. K. (2018). The Mechanical Microenvironment Regulates Ovarian Cancer Cell Morphology, Migration, and Spheroid Disaggregation. Sci. Rep. 8 (1), 1. doi:10.1038/s41598-018-25589-0
Medeiros, M., Ribeiro, A. O., Lupi, L. A., Romualdo, G. R., Pinhal, D., Chuffa, L. G. d. A., et al. (2019). Mimicking the Tumor Microenvironment: Fibroblasts Reduce miR-29b Expression and Increase the Motility of Ovarian Cancer Cells in a Co-culture Model. Biochem. Biophysical Res. Commun. 516 (1), 96–101. doi:10.1016/j.bbrc.2019.06.001
Mehta, G., Hsiao, A. Y., Ingram, M., Luker, G. D., and Takayama, S. (2012). Opportunities and Challenges for Use of Tumor Spheroids as Models to Test Drug Delivery and Efficacy. J. Control. Release 164 (2), 192–204. doi:10.1016/j.jconrel.2012.04.045
Mendibil, U., Ruiz-Hernandez, R., Retegi-Carrion, S., Garcia-Urquia, N., Olalde-Graells, B., and Abarrategi, A. (2020). Tissue-Specific Decellularization Methods: Rationale and Strategies to Achieve Regenerative Compounds. Ijms 21 (15), 5447. doi:10.3390/ijms21155447
Michael, B., Yong-Hyun, S., Aleksandar, R., and Bryan, B. (2016). The Effect of ECM Stiffness on Ovarian Follicle Development. Front. Bioeng. Biotechnol. 4, 1. doi:10.3389/conf.FBIOE.2016.01.00303
Mieulet, V., Garnier, C., Kieffer, Y., Guilbert, T., Nemati, F., Marangoni, E., et al. (2021). Stiffness Increases with Myofibroblast Content and Collagen Density in Mesenchymal High Grade Serous Ovarian Cancer. Sci. Rep. 11 (1), 1. doi:10.1038/s41598-021-83685-0
Mitra, A. K., Chiang, C. Y., Tiwari, P., Tomar, S., Watters, K. M., Peter, M. E., et al. (2015a). Microenvironment-induced Downregulation of miR-193b Drives Ovarian Cancer Metastasis. Oncogene 34 (48), 5923–5932. doi:10.1038/onc.2015.43
Mitra, A. K., Davis, D. A., Tomar, S., Roy, L., Gurler, H., Xie, J., et al. (2015b). In Vivo tumor Growth of High-Grade Serous Ovarian Cancer Cell Lines. Gynecol. Oncol. 138 (2), 372–377. doi:10.1016/j.ygyno.2015.05.040
Miyagawa, Y., Nagasaka, K., Yamawaki, K., Mori, Y., Ishiguro, T., Hashimoto, K., et al. (2020). Evaluating the Angiogenetic Properties of Ovarian Cancer Stem-like Cells Using the Three-Dimensional Co-culture System, NICO-1. JoVE 166, 1. doi:10.3791/61751
Moore, K., Colombo, N., Scambia, G., Kim, B.-G., Oaknin, A., Friedlander, M., et al. (2018). Maintenance Olaparib in Patients with Newly Diagnosed Advanced Ovarian Cancer. N. Engl. J. Med. 379 (26), 2495–2505. doi:10.1056/NEJMoa1810858
Morton, C. L., and Houghton, P. J. (2007). Establishment of Human Tumor Xenografts in Immunodeficient Mice. Nat. Protoc. 2 (2), 247–250. doi:10.1038/nprot.2007.25
Motohara, T., Masuda, K., Morotti, M., Zheng, Y., El-Sahhar, S., Chong, K. Y., et al. (2019). An Evolving story of the Metastatic Voyage of Ovarian Cancer Cells: Cellular and Molecular Orchestration of the Adipose-Rich Metastatic Microenvironment. Oncogene 38 (16), 2885–2898. doi:10.1038/s41388-018-0637-x
Motoyama, T. (1981). Biological Characterization Including Sensitivity to Mitomycin C of Cultured Human Ovarian Cancers (Author's Transl). Nihon Sanka Fujinka Gakkai Zasshi 33 (8), 1197–1204.
Moujaber, T., Balleine, R. L., Gao, B., Madsen, I., Harnett, P. R., and DeFazio, A. (2022). New Therapeutic Opportunities for Women with Low-Grade Serous Ovarian Cancer. Endocr. Relat. Cancer 29 (1), R1–r16. doi:10.1530/erc-21-0191
Muggia, F., and Safra, T. (2014). 'BRCAness' and its Implications for Platinum Action in Gynecologic Cancer. Anticancer Res. 34 (2), 551–556.
Mullen, P., Ritchie, A., Langdon, S. P., and Miller, W. R. (1996). Effect of Matrigel on the Tumorigenicity of Human Breast and Ovarian Carcinoma Cell Lines. Intl J. Cancer 67 (6), 816–820. doi:10.1002/(sici)1097-0215(19960917)67:6<816:aid-ijc10>3.0.co;2-#
Nanki, Y., Chiyoda, T., Hirasawa, A., Ookubo, A., Itoh, M., Ueno, M., et al. (2020). Patient-derived Ovarian Cancer Organoids Capture the Genomic Profiles of Primary Tumours Applicable for Drug Sensitivity and Resistance Testing. Sci. Rep. 10 (1), 1. doi:10.1038/s41598-020-69488-9
Nesic, K., Kondrashova, O., Hurley, R. M., McGehee, C. D., Vandenberg, C. J., Ho, G.-Y., et al. (2021). Acquired RAD51C Promoter Methylation Loss Causes PARP Inhibitor Resistance in High-Grade Serous Ovarian Carcinoma. Cancer Res. 81 (18), 4709–4722. doi:10.1158/0008-5472.Can-21-0774
Nguyen, L., W. M. Martens, J., Van Hoeck, A., and Cuppen, E. (2020). Pan-cancer Landscape of Homologous Recombination Deficiency. Nat. Commun. 11 (1), 5584. doi:10.1038/s41467-020-19406-4
Nikniaz, H., Zandieh, Z., Nouri, M., Daei-farshbaf, N., Aflatoonian, R., Gholipourmalekabadi, M., et al. (2021). Comparing Various Protocols of Human and Bovine Ovarian Tissue Decellularization to Prepare Extracellular Matrix-Alginate Scaffold for Better Follicle Development In Vitro. BMC Biotechnol. 21 (1), 8. doi:10.1186/s12896-020-00658-3
Ning, Y., Cui, Y., Li, X., Cao, X., Chen, A., Xu, C., et al. (2018). Co-culture of Ovarian Cancer Stem-like Cells with Macrophages Induced SKOV3 Cells Stemness via IL-8/STAT3 Signaling. Biomed. Pharmacother. 103, 262–271. doi:10.1016/j.biopha.2018.04.022
Nishi, Y., Yanase, T., Mu, Y.-M., Oba, K., Ichino, I., Saito, M., et al. (2001). Establishment and Characterization of a Steroidogenic Human Granulosa-like Tumor Cell Line, KGN, that Expresses Functional Follicle-Stimulating Hormone Receptor. Endocrinology 142 (1), 437–445. doi:10.1210/endo.142.1.7862
Noshadi, I., Hong, S., Sullivan, K. E., Shirzaei Sani, E., Portillo-Lara, R., Tamayol, A., et al. (2017). In Vitro and In Vivo Analysis of Visible Light Crosslinkable Gelatin Methacryloyl (GelMA) Hydrogels. Biomater. Sci. 5 (10), 2093–2105. doi:10.1039/c7bm00110j
Novak, C. M., Horst, E. N., Lin, E., and Mehta, G. (2020). Compressive Stimulation Enhances Ovarian Cancer Proliferation, Invasion, Chemoresistance, and Mechanotransduction via CDC42 in a 3D Bioreactor. Cancers 12 (6), 1521. doi:10.3390/cancers12061521
Nowacka, M., Sterzynska, K., Andrzejewska, M., Nowicki, M., and Januchowski, R. (2021). Drug Resistance Evaluation in Novel 3D In Vitro Model. Biomed. Pharmacother. 138, 111536. doi:10.1016/j.biopha.2021.111536
Nozawa, S., Yajima, M., Sasaki, H., Tsukazaki, K., Aoki, D., Sakayori, M., et al. (1991). A New CA125-like Antigen (CA602) Recognized by Two Monoclonal Antibodies against a Newly Established Ovarian clear Cell Carcinoma Cell Line (RMG-II). Jpn. J. Cancer Res. 82 (7), 854–861. doi:10.1111/j.1349-7006.1991.tb02713.x
Odunsi, A., McGray, A. J. R., Miliotto, A., Zhang, Y., Wang, J., Abiola, A., et al. (2020). Fidelity of Human Ovarian Cancer Patient-Derived Xenografts in a Partially Humanized Mouse Model for Preclinical Testing of Immunotherapies. J. Immunother. Cancer 8 (2), e001237. doi:10.1136/jitc-2020-001237
Onal, S., Alkaisi, M. M., and Nock, V. (2021). A Flexible Microdevice for Mechanical Cell Stimulation and Compression in Microfluidic Settings. Front. Phys. 9 (280), 1. doi:10.3389/fphy.2021.654918
Orkin, R. W., Gehron, P., McGoodwin, E. B., Martin, G. R., Valentine, T., and Swarm, R. (1977). A Murine Tumor Producing a Matrix of Basement Membrane. J. Exp. Med. 145 (1), 204–220. doi:10.1084/jem.145.1.204
Oza, A. M., Cook, A. D., Pfisterer, J., Embleton, A., Ledermann, J. A., Pujade-Lauraine, E., et al. (2015). Standard Chemotherapy with or without Bevacizumab for Women with Newly Diagnosed Ovarian Cancer (ICON7): Overall Survival Results of a Phase 3 Randomised Trial. Lancet Oncol. 16 (8), 928–936. doi:10.1016/s1470-2045(15)00086-8
Ozols, R. F., Willson, J. K., Grotzinger, K. R., and Young, R. C. (1980). Cloning of Human Ovarian Cancer Cells in Soft agar from Malignant and Peritoneal Washings. Cancer Res. 40 (8), 2743–2747.
Papp, E., Hallberg, D., Konecny, G. E., Bruhm, D. C., Adleff, V., Noë, M., et al. (2018). Integrated Genomic, Epigenomic, and Expression Analyses of Ovarian Cancer Cell Lines. Cell Rep. 25 (9), 2617–2633. doi:10.1016/j.celrep.2018.10.096
Paradiso, F., Fitzgerald, J., Yao, S., Barry, F., Taraballi, F., Gonzalez, D., et al. (2019). Marine Collagen Substrates for 2D and 3D Ovarian Cancer Cell Systems. Front. Bioeng. Biotechnol. 7, 1. doi:10.3389/fbioe.2019.00343
Parra-Herran, C., Bassiouny, D., Lerner-Ellis, J., Olkhov-Mitsel, E., Ismiil, N., Hogen, L., et al. (2019). p53, Mismatch Repair Protein, and POLE Abnormalities in Ovarian Clear Cell Carcinoma. Am. J. Surg. Pathol. 43 (12), 1591–1599. doi:10.1097/pas.0000000000001328
Patch, A.-M., Christie, E. L., Christie, E. L., Etemadmoghadam, D., Garsed, D. W., George, J., et al. (2015). Whole-genome Characterization of Chemoresistant Ovarian Cancer. Nature 521 (7553), 489–494. doi:10.1038/nature14410
Patra, B., Lateef, M. A., Brodeur, M. N., Fleury, H., Carmona, E., Péant, B., et al. (2020). Carboplatin Sensitivity in Epithelial Ovarian Cancer Cell Lines: The Impact of Model Systems. PLoS ONE 15 (12), e0244549. doi:10.1371/journal.pone.0244549
Pennington, K. P., Walsh, T., Harrell, M. I., Lee, M. K., Pennil, C. C., Rendi, M. H., et al. (2014). Germline and Somatic Mutations in Homologous Recombination Genes Predict Platinum Response and Survival in Ovarian, Fallopian Tube, and Peritoneal Carcinomas. Clin. Cancer Res. 20 (3), 764–775. doi:10.1158/1078-0432.Ccr-13-2287
Peres, L. C., Risch, H., Terry, K. L., Webb, P. M., Goodman, M. T., Wu, A. H., et al. (2018). Racial/ethnic Differences in the Epidemiology of Ovarian Cancer: a Pooled Analysis of 12 Case-Control Studies. Int. J. Epidemiol. 47 (2), 460–472. doi:10.1093/ije/dyx252
Perets, R., and Drapkin, R. (2016). It's Totally Tubular…Riding the New Wave of Ovarian Cancer Research. Cancer Res. 76 (1), 10–17. doi:10.1158/0008-5472.Can-15-1382
Perets, R., Wyant, G. A., Muto, K. W., Bijron, J. G., Poole, B. B., Chin, K. T., et al. (2013). Transformation of the Fallopian Tube Secretory Epithelium Leads to High-Grade Serous Ovarian Cancer in Brca;Tp53;Pten Models. Cancer Cell 24 (6), 751–765. doi:10.1016/j.ccr.2013.10.013
Perren, T. J., Swart, A. M., Pfisterer, J., Ledermann, J. A., Pujade-Lauraine, E., Kristensen, G., et al. (2011). A Phase 3 Trial of Bevacizumab in Ovarian Cancer. N. Engl. J. Med. 365 (26), 2484–2496. doi:10.1056/NEJMoa1103799
Peters, P. N., Schryver, E. M., Lengyel, E., and Kenny, H. (2015). Modeling the Early Steps of Ovarian Cancer Dissemination in an Organotypic Culture of the Human Peritoneal Cavity. JoVE 106, e53541. doi:10.3791/53541
Phan, N., Hong, J. J., Tofig, B., Mapua, M., Elashoff, D., Moatamed, N. A., et al. (2019). A Simple High-Throughput Approach Identifies Actionable Drug Sensitivities in Patient-Derived Tumor Organoids. Commun. Biol. 2 (1), 78. doi:10.1038/s42003-019-0305-x
Pietilä, E. A., Gonzalez-Molina, J., Moyano-Galceran, L., Jamalzadeh, S., Zhang, K., Lehtinen, L., et al. (2021). Co-evolution of Matrisome and Adaptive Adhesion Dynamics Drives Ovarian Cancer Chemoresistance. Nat. Commun. 12 (1), 1. doi:10.1038/s41467-021-24009-8
Pirker, R., FitzGerald, D. J., Hamilton, T. C., Ozols, R. F., Laird, W., Frankel, A. E., et al. (1985). Characterization of Immunotoxins Active against Ovarian Cancer Cell Lines. J. Clin. Invest. 76 (3), 1261–1267. doi:10.1172/JCI112082
Provencher, D. M., Lounis, H., Champoux, L., Tétrault, M., Manderson, E. N., Wang, J. C., et al. (2000). Characterization of Four Novel Epithelial Ovarian Cancer Cell Lines. In Vitro Cell Dev Biol Anim 36 (6), 357–361. doi:10.1290/1071-2690(2000)036<0357:Cofneo>2.0.Co;2
Rafehi, S., Valdes, Y. R., Bertrand, M., Mcgee, J., Préfontaine, M., Sugimoto, A., et al. (2016). TGFβ Signaling Regulates Epithelial-Mesenchymal Plasticity in Ovarian Cancer Ascites-Derived Spheroids. Endocrine-Related Cancer 23 (3), 147–159. doi:10.1530/erc-15-0383
Raghavan, S., Mehta, P., Horst, E. N., Ward, M. R., Rowley, K. R., and Mehta, G. (2016). Comparative Analysis of Tumor Spheroid Generation Techniques for Differential In Vitro Drug Toxicity. Oncotarget 7 (13), 16948–16961. doi:10.18632/oncotarget.7659
Raghavan, S., Mehta, P., Xie, Y., Lei, Y. L., and Mehta, G. (2019). Ovarian Cancer Stem Cells and Macrophages Reciprocally Interact through the WNT Pathway to Promote Pro-tumoral and Malignant Phenotypes in 3D Engineered Microenvironments. J. Immunotherapy Cancer 7 (1), 1. doi:10.1186/s40425-019-0666-1
Raghavan, S., Ward, M. R., Rowley, K. R., Wold, R. M., Takayama, S., Buckanovich, R. J., et al. (2015). Formation of Stable Small Cell Number Three-Dimensional Ovarian Cancer Spheroids Using Hanging Drop Arrays for Preclinical Drug Sensitivity Assays. Gynecol. Oncol. 138 (1), 181–189. doi:10.1016/j.ygyno.2015.04.014
Ricci, F., Guffanti, F., Affatato, R., Brunelli, L., Roberta, P., Fruscio, R., et al. (2020). Establishment of Patient-Derived Tumor Xenograft Models of Mucinous Ovarian Cancer. Am. J. Cancer Res. 10 (2), 572–580.
Rizvi, I., Gurkan, U. A., Tasoglu, S., Alagic, N., Celli, J. P., Mensah, L. B., et al. (2013). Flow Induces Epithelial-Mesenchymal Transition, Cellular Heterogeneity and Biomarker Modulation in 3D Ovarian Cancer Nodules. Proc. Natl. Acad. Sci. 110 (22), E1974–E1983. doi:10.1073/pnas.1216989110
Roby, K. F., Taylor, C. C., Sweetwood, J. P., Cheng, Y., Pace, J. L., Tawfik, O., et al. (2000). Development of a Syngeneic Mouse Model for Events Related to Ovarian Cancer. Carcinogenesis 21 (4), 585–591. doi:10.1093/carcin/21.4.585
Rosales-Nieves, A. E., and González-Reyes, A. (2014). Genetics and Mechanisms of Ovarian Cancer: Parallels between Drosophila and Humans. Semin. Cell Dev. Biol. 28, 104–109. doi:10.1016/j.semcdb.2014.03.031
Rowley, J. A., Madlambayan, G., and Mooney, D. J. (1999). Alginate Hydrogels as Synthetic Extracellular Matrix Materials. Biomaterials 20 (1), 45–53. doi:10.1016/s0142-9612(98)00107-0
Saga, Y., Saga, Y., Mizukami, H., Wang, D., Fujiwara, H., Takei, Y., et al. (2012). Cetuximab Inhibits the Growth of Mucinous Ovarian Carcinoma Tumor Cells Lacking KRAS Gene Mutations. Oncol. Rep. 27 (5), 1336–1340. doi:10.3892/or.2012.1626
Saha, B., Mathur, T., Handley, K. F., Hu, W., Afshar-Kharghan, V., Sood, A. K., et al. (2020). OvCa-Chip Microsystem Recreates Vascular Endothelium-Mediated Platelet Extravasation in Ovarian Cancer. Blood Adv. 4 (14), 3329–3342. doi:10.1182/bloodadvances.2020001632
Sakayori, M., Nozawa, S., Udagawa, Y., Chin, K., Lee, S. G., Sakuma, T., et al. (1990). Biological Properties of Two Newly Established Cell Lines (RMUG-S, RMUG-L) from a Human Ovarian Mucinous Cystadenocarcinoma. Hum. Cell 3 (1), 52–56.
Šale, S., and Orsulic, S. (2006). Models of Ovarian Cancer Metastasis: Murine Models. Drug Discov. Today Dis. Models 3 (2), 149–154. doi:10.1016/j.ddmod.2006.05.006
Samartzis, E. P., Labidi-Galy, S. I., Moschetta, M., Uccello, M., Kalaitzopoulos, D. R., Perez-Fidalgo, J. A., et al. (2020). Endometriosis-associated Ovarian Carcinomas: Insights into Pathogenesis, Diagnostics, and Therapeutic Targets-A Narrative Review. Ann. Transl Med. 8 (24), 1712. doi:10.21037/atm-20-3022a
Schwarz, R. P., Goodwin, T. J., and Wolf, D. A. (1992). Cell Culture for Three-Dimensional Modeling in Rotating-wall Vessels: an Application of Simulated Microgravity. J. Tissue Cult. Methods 14 (2), 51–57. doi:10.1007/bf01404744
Scott, A. L., Ayoola, O., and Zervantonakis, I. K. (2021). Abstract PO042: Microfluidic Modeling of Tumor-Macrophage Signaling in Ovarian Cancer. Cancer Res. 81 (5), PO042. doi:10.1158/1538-7445.TME21-PO042
Scott, C. L., Becker, M. A., Haluska, P., and Samimi, G. (2013). Patient-derived Xenograft Models to Improve Targeted Therapy in Epithelial Ovarian Cancer Treatment. Front. Oncol. 3, 295. doi:10.3389/fonc.2013.00295
Shield, K., Ackland, M. L., Ahmed, N., and Rice, G. E. (2009). Multicellular Spheroids in Ovarian Cancer Metastases: Biology and Pathology. Gynecol. Oncol. 113 (1), 143–148. doi:10.1016/j.ygyno.2008.11.032
Shield, K., Riley, C., Quinn, M. A., Rice, G. E., Ackland, M. L., and Ahmed, N. (2007). α2β1 Integrin Affects Metastatic Potential of Ovarian Carcinoma Spheroids by Supporting Disaggregation and Proteolysis. J. Carcinog 6, 11. doi:10.1186/1477-3163-6-11
Shih, I.-M., and Kurman, R. J. (2004). Ovarian Tumorigenesis. Am. J. Pathol. 164 (5), 1511–1518. doi:10.1016/s0002-9440(10)63708-x
Shin, H.-Y., Yang, W., Lee, E.-j., Han, G. H., Cho, H., Chay, D. B., et al. (2018). Establishment of Five Immortalized Human Ovarian Surface Epithelial Cell Lines via SV40 T Antigen or HPV E6/E7 Expression. PLoS ONE 13 (10), e0205297. doi:10.1371/journal.pone.0205297
Shin, S. I., Freedman, V. H., Risser, R., and Pollack, R. (1975). Tumorigenicity of Virus-Transformed Cells in Nude Mice Is Correlated Specifically with anchorage Independent Growth In Vitro. Proc. Natl. Acad. Sci. 72 (11), 4435–4439. doi:10.1073/pnas.72.11.4435
Shin, S., Ikram, M., Subhan, F., Kang, H. Y., Lim, Y., Lee, R., et al. (2016). Alginate-marine Collagen-Agarose Composite Hydrogels as Matrices for Biomimetic 3D Cell Spheroid Formation. RSC Adv. 6 (52), 46952–46965. doi:10.1039/c6ra01937d
Shishido, A., Mori, S., Yokoyama, Y., Hamada, Y., Minami, K., Qian, Y., et al. (2018). Mesothelial Cells Facilitate Cancer Stem like properties in spheroids of ovarian cancer cells. Oncol. Rep. 40 (4), 2105–2114. doi:10.3892/or.2018.6605
Shuford, S., Wilhelm, C., Rayner, M., Elrod, A., Millard, M., Mattingly, C., et al. (2019). Prospective Validation of an Ex Vivo, Patient-Derived 3D Spheroid Model for Response Predictions in Newly Diagnosed Ovarian Cancer. Sci. Rep. 9 (1), 11153. doi:10.1038/s41598-019-47578-7
Singh, M. S., Goldsmith, M., Thakur, K., Chatterjee, S., Landesman-Milo, D., Levy, T., et al. (2020). An Ovarian Spheroid Based Tumor Model that Represents Vascularized Tumors and Enables the Investigation of Nanomedicine Therapeutics. Nanoscale 12 (3), 1894–1903. doi:10.1039/c9nr09572a
Siolas, D., and Hannon, G. J. (2013). Patient-derived Tumor Xenografts: Transforming Clinical Samples into Mouse Models. Cancer Res. 73 (17), 5315–5319. doi:10.1158/0008-5472.CAN-13-1069
Sodek, K. L., Brown, T. J., and Ringuette, M. J. (2008). Collagen I but Not Matrigel Matrices Provide an MMP-dependent Barrier to Ovarian Cancer Cell Penetration. BMC Cancer 8 (1), 223. doi:10.1186/1471-2407-8-223
Sodek, K. L., Ringuette, M. J., and Brown, T. J. (2009). Compact Spheroid Formation by Ovarian Cancer Cells Is Associated with Contractile Behavior and an Invasive Phenotype. Int. J. Cancer 124 (9), 2060–2070. doi:10.1002/ijc.24188
Song, H., Cai, G.-H., Liang, J., Ao, D.-S., Wang, H., and Yang, Z.-H. (2020). Three-dimensional Culture and Clinical Drug Responses of a Highly Metastatic Human Ovarian Cancer HO-8910PM Cells in Nanofibrous Microenvironments of Three Hydrogel Biomaterials. J. Nanobiotechnol 18 (1), 1. doi:10.1186/s12951-020-00646-x
Soofi, S. S., Last, J. A., Liliensiek, S. J., Nealey, P. F., and Murphy, C. J. (2009). The Elastic Modulus of Matrigel as Determined by Atomic Force Microscopy. J. Struct. Biol. 167 (3), 216–219. doi:10.1016/j.jsb.2009.05.005
Sterzyńska, K., Klejewski, A., Wojtowicz, K., Świerczewska, M., Nowacka, M., Kaźmierczak, D., et al. (2018). Mutual Expression of ALDH1A1, LOX, and Collagens in Ovarian Cancer Cell Lines as Combined CSCs- and ECM-Related Models of Drug Resistance Development. Ijms 20 (1), 54. doi:10.3390/ijms20010054
Stuckelberger, S., and Drapkin, R. (2018). Precious GEMMs: Emergence of Faithful Models for Ovarian Cancer Research. J. Pathol. 245 (2), 129–131. doi:10.1002/path.5065
Sugiyama, T., Kamura, T., Kigawa, J., Terakawa, N., Kikuchi, Y., Kita, T., et al. (2000). Clinical Characteristics of clear Cell Carcinoma of the Ovary. Cancer 88 (11), 2584–2589. doi:10.1002/1097-0142(20000601)88:11<2584:aid-cncr22>3.0.co;2-5
Sung, H., Ferlay, J., Siegel, R. L., Laversanne, M., Soerjomataram, I., Jemal, A., et al. (2021). Global Cancer Statistics 2020: GLOBOCAN Estimates of Incidence and Mortality Worldwide for 36 Cancers in 185 Countries. CA A. Cancer J. Clin. 71 (3), 209–249. doi:10.3322/caac.21660
Swisher, E. M., Lin, K. K., Oza, A. M., Scott, C. L., Giordano, H., Sun, J., et al. (2017). Rucaparib in Relapsed, Platinum-Sensitive High-Grade Ovarian Carcinoma (ARIEL2 Part 1): an International, Multicentre, Open-Label, Phase 2 Trial. Lancet Oncol. 18 (1), 75–87. doi:10.1016/s1470-2045(16)30559-9
Szabova, L., Bupp, S., Kamal, M., Householder, D. B., Hernandez, L., Schlomer, J. J., et al. (2014). Pathway-Specific Engineered Mouse Allograft Models Functionally Recapitulate Human Serous Epithelial Ovarian Cancer. PLoS ONE 9 (4), e95649. doi:10.1371/journal.pone.0095649
Szabova, L., Yin, C., Bupp, S., Guerin, T. M., Schlomer, J. J., Householder, D. B., et al. (2012). Perturbation of Rb, P53, and Brca1 or Brca2 Cooperate in Inducing Metastatic Serous Epithelial Ovarian Cancer. Cancer Res. 72 (16), 4141–4153. doi:10.1158/0008-5472.CAN-11-3834
Takeda, M., Magaki, T., Okazaki, T., Kawahara, Y., Manabe, T., Yuge, L., et al. (2009). Effects of Simulated Microgravity on Proliferation and Chemosensitivity in Malignant Glioma Cells. Neurosci. Lett. 463 (1), 54–59. doi:10.1016/j.neulet.2009.07.045
Tan, T. Z., Ye, J., Yee, C. V., Lim, D., Ngoi, N. Y. L., Tan, D. S. P., et al. (2019). Analysis of Gene Expression Signatures Identifies Prognostic and Functionally Distinct Ovarian clear Cell Carcinoma Subtypes. EBioMedicine 50, 203–210. doi:10.1016/j.ebiom.2019.11.017
Tofani, L. B., Sousa, L. O., Luiz, M. T., Abriata, J. P., Marchetti, J. M., Leopoldino, A. M., et al. (2021). Generation of a Three-Dimensional In Vitro Ovarian Cancer Co-culture Model for Drug Screening Assays. J. Pharm. Sci. 110 (7), 2629–2636. doi:10.1016/j.xphs.2021.04.003
Tong, J. G., Valdes, Y. R., Barrett, J. W., Bell, J. C., Stojdl, D., McFadden, G., et al. (2015). Evidence for Differential Viral Oncolytic Efficacy in an In Vitro Model of Epithelial Ovarian Cancer Metastasis. Mol. Ther. - Oncolytics 2, 15013. doi:10.1038/mto.2015.13
Trento, M., Munari, G., Carraro, V., Lanza, C., Salmaso, R., Pizzi, S., et al. (2020). Mutational and Immunophenotypic Profiling of a Series of 8 Tubo-Ovarian Carcinosarcomas Revealed a Monoclonal Origin of the Disease. Int. J. Gynecol. Pathol. 39 (4), 305–312. doi:10.1097/pgp.0000000000000645
Tsao, S.-W., Mok, S. C., Fey, E. G., Fletcher, J. A., Wan, T. S. K., Chew, E.-C., et al. (1995). Characterization of Human Ovarian Surface Epithelial Cells Immortalized by Human Papilloma Viral Oncogenes (HPV-E6e7 ORFs). Exp. Cell Res. 218 (2), 499–507. doi:10.1006/excr.1995.1184
Tudrej, P., Kujawa, K. A., Cortez, A. J., and Lisowska, K. M. (2019). Characteristics of In Vivo Model Systems for Ovarian Cancer Studies. Diagnostics 9 (3), 120. doi:10.3390/diagnostics9030120
Valabrega, G., Scotto, G., Tuninetti, V., Pani, A., and Scaglione, F. (2021). Differences in PARP Inhibitors for the Treatment of Ovarian Cancer: Mechanisms of Action, Pharmacology, Safety, and Efficacy. Ijms 22 (8), 4203. doi:10.3390/ijms22084203
van den Berg-Bakker, C. A. M., Hagemeijer, A., Franken-Postma, E. M., Smit, V. T. H. B. M., Kuppen, P. J. K., Claasen, H. H. V. R., et al. (1993). Establishment and Characterization of 7 Ovarian Carcinoma Cell Lines and One Granulosa Tumor Cell Line: Growth Features and Cytogenetics. Int. J. Cancer 53 (4), 613–620. doi:10.1002/ijc.2910530415
Varoni, E., Tschon, M., Palazzo, B., Nitti, P., Martini, L., and Rimondini, L. (2012). Agarose Gel as Biomaterial or Scaffold for Implantation Surgery: Characterization, Histological and Histomorphometric Study on Soft Tissue Response. Connect. Tissue Res. 53 (6), 548–554. doi:10.3109/03008207.2012.712583
Vidyasekar, P., Shyamsunder, P., Arun, R., Santhakumar, R., Kapadia, N. K., Kumar, R., et al. (2015). Genome Wide Expression Profiling of Cancer Cell Lines Cultured in Microgravity Reveals Significant Dysregulation of Cell Cycle and MicroRNA Gene Networks. PLoS ONE 10 (8), e0135958. doi:10.1371/journal.pone.0135958
Vinci, M., Gowan, S., Boxall, F., Patterson, L., Zimmermann, M., Court, W., et al. (2012). Advances in Establishment and Analysis of Three-Dimensional Tumor Spheroid-Based Functional Assays for Target Validation and Drug Evaluation. BMC Biol. 10 (1), 29. doi:10.1186/1741-7007-10-29
Vukicevic, S., Kleinman, H. K., Luyten, F. P., Roberts, A. B., Roche, N. S., and Reddi, A. H. (1992). Identification of Multiple Active Growth Factors in Basement Membrane Matrigel Suggests Caution in Interpretation of Cellular Activity Related to Extracellular Matrix Components. Exp. Cell Res. 202 (1), 1–8. doi:10.1016/0014-4827(92)90397-q
Wang, X., Zhao, X., Wang, K., Wu, L., and Duan, T. (2013). Interaction of Monocytes/macrophages with Ovarian Cancer Cells Promotes Angiogenesisin Vitro. Cancer Sci. 104 (4), 516–523. doi:10.1111/cas.12110
Wang, Z. C., Birkbak, N. J., Culhane, A. C., Drapkin, R., Fatima, A., Tian, R., et al. (2012). Profiles of Genomic Instability in High-Grade Serous Ovarian Cancer Predict Treatment Outcome. Clin. Cancer Res. 18 (20), 5806–5815. doi:10.1158/1078-0432.Ccr-12-0857
Ward Rashidi, M. R., Mehta, P., Bregenzer, M., Raghavan, S., Fleck, E. M., Horst, E. N., et al. (2019). Engineered 3D Model of Cancer Stem Cell Enrichment and Chemoresistance. Neoplasia 21 (8), 822–836. doi:10.1016/j.neo.2019.06.005
Watkins, J. A., Irshad, S., Grigoriadis, A., and Tutt, A. N. (2014). Genomic Scars as Biomarkers of Homologous Recombination Deficiency and Drug Response in Breast and Ovarian Cancers. Breast Cancer Res. 16 (3), 211. doi:10.1186/bcr3670
Watters, K. M., Bajwa, P., and Kenny, H. A. (2018). Organotypic 3D Models of the Ovarian Cancer Tumor Microenvironment. Cancers 10 (8), 265. doi:10.3390/cancers10080265
Weydert, Z., Lal-Nag, M., Mathews-Greiner, L., Thiel, C., Cordes, H., Küpfer, L., et al. (2020). A 3D Heterotypic Multicellular Tumor Spheroid Assay Platform to Discriminate Drug Effects on Stroma versus Cancer Cells. SLAS DISCOVERY: Advancing Sci. Drug Discov. 25 (3), 265–276. doi:10.1177/2472555219880194
Wilkinson-Ryan, I., Pham, M. M., Sergent, P., Tafe, L. J., and Berwin, B. L. (2019). A Syngeneic Mouse Model of Epithelial Ovarian Cancer Port Site Metastases. Translational Oncol. 12 (1), 62–68. doi:10.1016/j.tranon.2018.08.020
Wilson, A., Dent, M., Pejovic, T., Hubbold, L., and Radford, H. (1996). Characterisation of Seven Human Ovarian Tumour Cell Lines. Br. J. Cancer 74 (5), 722–727. doi:10.1038/bjc.1996.428
Woo, M. M. M., Salamanca, C. M., Minor, A., and Auersperg, N. (2007). An Improved Assay to Quantitate the Invasiveness of Cells in Modified Boyden chambers. In Vitro Cell.Dev.Biol.-Animal 43 (1), 7–9. doi:10.1007/s11626-006-9002-4
Wu, J., Zheng, Y., Tian, Q., Yao, M., and Yi, X. (2019). Establishment of Patient‐derived Xenograft Model in Ovarian Cancer and its Influence Factors Analysis. J. Obstet. Gynaecol. Res. 45 (10), 2062–2073. doi:10.1111/jog.14054
Wu, T., Gao, Y. Y., Su, J., Tang, X. N., Chen, Q., Ma, L. W., et al. (2021). Three-dimensional Bioprinting of Artificial Ovaries by an Extrusion-Based Method Using Gelatin-Methacryloyl Bioink. Climacteric 17, 1–9. doi:10.1080/13697137.2021.1921726
Wu, Y.-H., Chang, T.-H., Huang, Y.-F., Huang, H.-D., and Chou, C.-Y. (2014). COL11A1 Promotes Tumor Progression and Predicts Poor Clinical Outcome in Ovarian Cancer. Oncogene 33 (26), 3432–3440. doi:10.1038/onc.2013.307
Xu, F., Celli, J., Rizvi, I., Moon, S., Hasan, T., and Demirci, U. (2011). A Three-Dimensional In Vitro Ovarian Cancer Coculture Model Using a High-Throughput Cell Patterning Platform. Biotechnol. J. 6 (2), 204–212. doi:10.1002/biot.201000340
Xu, G., Yin, F., Wu, H., Hu, X., Zheng, L., and Zhao, J. (2014). In Vitro ovarian Cancer Model Based on Three-Dimensional Agarose Hydrogel. J. Tissue Eng. 5 (0), 204173141352043. doi:10.1177/2041731413520438
Xu, S., Xu, H., Wang, W., Li, S., Li, H., Li, T., et al. (2019). The Role of Collagen in Cancer: from Bench to Bedside. J. Transl Med. 17 (1), 309. doi:10.1186/s12967-019-2058-1
Xue, Y., Morris, J. L., Yang, K., Fu, Z., Zhu, X., Johnson, F., et al. (2021). SMARCA4/2 Loss Inhibits Chemotherapy-Induced Apoptosis by Restricting IP3R3-Mediated Ca2+ Flux to Mitochondria. Nat. Commun. 12 (1), 5404. doi:10.1038/s41467-021-25260-9
Yamada, Y., Yoshida, C., Hamada, K., Kikkawa, Y., and Nomizu, M. (2020). Development of Three-Dimensional Cell Culture Scaffolds Using Laminin Peptide-Conjugated Agarose Microgels. Biomacromolecules 21 (9), 3765–3771. doi:10.1021/acs.biomac.0c00871
Yamawaki, K., Mori, Y., Sakai, H., Kanda, Y., Shiokawa, D., Ueda, H., et al. (2021). Integrative Analyses of Gene Expression and Chemosensitivity of Patient-Derived Ovarian Cancer Spheroids Link G6PD-Driven Redox Metabolism to Cisplatin Chemoresistance. Cancer Lett. 521, 29–38. doi:10.1016/j.canlet.2021.08.018
Yanagibashi, T., Gorai, I., Nakazawa, T., Miyagi, E., Hirahara, F., Kitamura, H., et al. (1997). Complexity of Expression of the Intermediate Filaments of Six New Human Ovarian Carcinoma Cell Lines: New Expression of Cytokeratin 20. Br. J. Cancer 76 (7), 829–835. doi:10.1038/bjc.1997.471
Yang, C., Hillas, P. J., Baez, J. A., Nokelainen, M., Balan, J., Tang, J., et al. (2004). The Application of Recombinant Human Collagen in Tissue Engineering. BioDrugs 18 (2), 103–119. doi:10.2165/00063030-200418020-00004
Yang, Z., Xu, H., and Zhao, X. (2020). Designer Self‐Assembling Peptide Hydrogels to Engineer 3D Cell Microenvironments for Cell Constructs Formation and Precise Oncology Remodeling in Ovarian Cancer. Adv. Sci. 7 (9), 1903718. doi:10.1002/advs.201903718
Yang, Z., and Zhao, X. (2011). A 3D Model of Ovarian Cancer Cell Lines on Peptide Nanofiber Scaffold to Explore the Cell–Scaffold Interaction and Chemotherapeutic Resistance of Anticancer Drugs. Ijn 6, 303–310. doi:10.2147/IJN.S15279
Yi, J., Lin, Y., Yicong, W., Chengyan, L., Shulin, Z., and Wenjun, C. (2020). Effect of Macrophages on Biological Function of Ovarian Cancer Cells in Tumor Microenvironment In Vitro. Arch. Gynecol. Obstet. 302 (4), 1009–1017. doi:10.1007/s00404-020-05719-8
Yoshiya, N., Adachi, S., Misawa, Y., Yuzawa, H., Honda, T., Kanazawa, K., et al. (1989). Isolation of Cisplatin-Resistant Subline from Human Ovarian Cancer Cell Line and Analysis of its Cell-Biological Characteristics. Nihon Sanka Fujinka Gakkai Zasshi 41 (1), 7–14.
Yu, J. W., Bhattacharya, S., Yanamandra, N., Kilian, D., Shi, H., Yadavilli, S., et al. (2018). Tumor-immune Profiling of Murine Syngeneic Tumor Models as a Framework to Guide Mechanistic Studies and Predict Therapy Response in Distinct Tumor Microenvironments. PLoS ONE 13 (11), e0206223. doi:10.1371/journal.pone.0206223
Yuan, J., Yi, K., and Yang, L. (2021). LncRNA NEAT1 Promotes Proliferation of Ovarian Cancer Cells and Angiogenesis of Co-incubated Human Umbilical Vein Endothelial Cells by Regulating FGF9 through Sponging miR-365. Medicine (Baltimore) 100 (3), e23423. doi:10.1097/md.0000000000023423
Zakarya, R., Howell, V. M., and Colvin, E. K. (2020). Modelling Epithelial Ovarian Cancer in Mice: Classical and Emerging Approaches. Ijms 21 (13), 4806. doi:10.3390/ijms21134806
Zarrintaj, P., Manouchehri, S., Ahmadi, Z., Saeb, M. R., Urbanska, A. M., Kaplan, D. L., et al. (2018). Agarose-based Biomaterials for Tissue Engineering. Carbohydr. Polym. 187, 66–84. doi:10.1016/j.carbpol.2018.01.060
Zhai, Y., Wu, R., Kuick, R., Sessine, M. S., Schulman, S., Green, M., et al. (2017). High-grade Serous Carcinomas Arise in the Mouse Oviduct via Defects Linked to the Human Disease. J. Pathol. 243 (1), 16–25. doi:10.1002/path.4927
Zhang, L., Yang, N., Conejo Garcia, J.-R., Mohamed, A., Benencia, F., Rubin, S. C., et al. (2002). Generation of a Syngeneic Mouse Model to Study the Effects of Vascular Endothelial Growth Factor in Ovarian Carcinoma. Am. J. Pathol. 161 (6), 2295–2309. doi:10.1016/s0002-9440(10)64505-1
Zhang, S., Dolgalev, I., Zhang, T., Ran, H., Levine, D. A., and Neel, B. G. (2019). Both fallopian Tube and Ovarian Surface Epithelium Are Cells-Of-Origin for High-Grade Serous Ovarian Carcinoma. Nat. Commun. 10 (1), 5367. doi:10.1038/s41467-019-13116-2
Zhao, L., Xiu, J., Liu, Y., Zhang, T., Pan, W., Zheng, X., et al. (2019a). A 3D Printed Hanging Drop Dripper for Tumor Spheroids Analysis without Recovery. Sci. Rep. 9 (1), 1. doi:10.1038/s41598-019-56241-0
Zhao, Y., Cao, J., Melamed, A., Worley, M., Gockley, A., Jones, D., et al. (2019b). Losartan Treatment Enhances Chemotherapy Efficacy and Reduces Ascites in Ovarian Cancer Models by Normalizing the Tumor Stroma. Proc. Natl. Acad. Sci. USA 116 (6), 2210–2219. doi:10.1073/pnas.1818357116
Zhou, N., Ma, X., Bernaerts, K. V., Ren, P., Hu, W., and Zhang, T. (2020). Expansion of Ovarian Cancer Stem-like Cells in Poly(ethylene Glycol)-Cross-Linked Poly(methyl Vinyl Ether-Alt-Maleic Acid) and Alginate Double-Network Hydrogels. ACS Biomater. Sci. Eng. 6 (6), 3310–3326. doi:10.1021/acsbiomaterials.9b01967
Zhou, N., Ma, X., Hu, W., Ren, P., Zhao, Y., and Zhang, T. (2021). Effect of RGD Content in Poly(ethylene Glycol)-Crosslinked Poly(methyl Vinyl Ether-Alt-Maleic Acid) Hydrogels on the Expansion of Ovarian Cancer Stem-like Cells. Mater. Sci. Eng. C 118, 111477. doi:10.1016/j.msec.2020.111477
Keywords: ovarian cancer, 3D cell culture, 3D bio-printing, organoids, tumoroid, drug screening, personalized medicine
Citation: Yee C, Dickson K-A, Muntasir MN, Ma Y and Marsh DJ (2022) Three-Dimensional Modelling of Ovarian Cancer: From Cell Lines to Organoids for Discovery and Personalized Medicine. Front. Bioeng. Biotechnol. 10:836984. doi: 10.3389/fbioe.2022.836984
Received: 16 December 2021; Accepted: 19 January 2022;
Published: 10 February 2022.
Edited by:
Dunja Aksentijevic, Queen Mary University of London, United KingdomReviewed by:
Ting-Yuan Tu, National Cheng Kung University, TaiwanCopyright © 2022 Yee, Dickson, Muntasir, Ma and Marsh. This is an open-access article distributed under the terms of the Creative Commons Attribution License (CC BY). The use, distribution or reproduction in other forums is permitted, provided the original author(s) and the copyright owner(s) are credited and that the original publication in this journal is cited, in accordance with accepted academic practice. No use, distribution or reproduction is permitted which does not comply with these terms.
*Correspondence: Christine Yee, Q2hyaXN0aW5lLlllZUB1dHMuZWR1LmF1
Disclaimer: All claims expressed in this article are solely those of the authors and do not necessarily represent those of their affiliated organizations, or those of the publisher, the editors and the reviewers. Any product that may be evaluated in this article or claim that may be made by its manufacturer is not guaranteed or endorsed by the publisher.
Research integrity at Frontiers
Learn more about the work of our research integrity team to safeguard the quality of each article we publish.