- 1Unidad de Investigación en Enfermedades Metabólicas, Centro Médico Nacional Siglo XXI, Instituto Mexicano del Seguro Social, Mexico City, Mexico
- 2Instituto de Ciencias Aplicadas y Tecnología (ICAT), Universidad Nacional Autónoma de México (UNAM), Mexico City, Mexico
- 3Instituto de Investigaciones Filosóficas, Universidad Nacional Autónoma de México (UNAM), Mexico City, Mexico
- 4Instituto Nacional de Pediatría, Mexico City, Mexico
Proteins are some of the most fascinating and challenging molecules in the universe, and they pose a big challenge for artificial intelligence. The implementation of machine learning/AI in protein science gives rise to a world of knowledge adventures in the workhorse of the cell and proteome homeostasis, which are essential for making life possible. This opens up epistemic horizons thanks to a coupling of human tacit–explicit knowledge with machine learning power, the benefits of which are already tangible, such as important advances in protein structure prediction. Moreover, the driving force behind the protein processes of self-organization, adjustment, and fitness requires a space corresponding to gigabytes of life data in its order of magnitude. There are many tasks such as novel protein design, protein folding pathways, and synthetic metabolic routes, as well as protein-aggregation mechanisms, pathogenesis of protein misfolding and disease, and proteostasis networks that are currently unexplored or unrevealed. In this systematic review and biochemical meta-analysis, we aim to contribute to bridging the gap between what we call binomial artificial intelligence (AI) and protein science (PS), a growing research enterprise with exciting and promising biotechnological and biomedical applications. We undertake our task by exploring “the state of the art” in AI and machine learning (ML) applications to protein science in the scientific literature to address some critical research questions in this domain, including What kind of tasks are already explored by ML approaches to protein sciences? What are the most common ML algorithms and databases used? What is the situational diagnostic of the AI–PS inter-field? What do ML processing steps have in common? We also formulate novel questions such as Is it possible to discover what the rules of protein evolution are with the binomial AI–PS? How do protein folding pathways evolve? What are the rules that dictate the folds? What are the minimal nuclear protein structures? How do protein aggregates form and why do they exhibit different toxicities? What are the structural properties of amyloid proteins? How can we design an effective proteostasis network to deal with misfolded proteins? We are a cross-functional group of scientists from several academic disciplines, and we have conducted the systematic review using a variant of the PICO and PRISMA approaches. The search was carried out in four databases (PubMed, Bireme, OVID, and EBSCO Web of Science), resulting in 144 research articles. After three rounds of quality screening, 93 articles were finally selected for further analysis. A summary of our findings is as follows: regarding AI applications, there are mainly four types: 1) genomics, 2) protein structure and function, 3) protein design and evolution, and 4) drug design. In terms of the ML algorithms and databases used, supervised learning was the most common approach (85%). As for the databases used for the ML models, PDB and UniprotKB/Swissprot were the most common ones (21 and 8%, respectively). Moreover, we identified that approximately 63% of the articles organized their results into three steps, which we labeled pre-process, process, and post-process. A few studies combined data from several databases or created their own databases after the pre-process. Our main finding is that, as of today, there are no research road maps serving as guides to address gaps in our knowledge of the AI–PS binomial. All research efforts to collect, integrate multidimensional data features, and then analyze and validate them are, so far, uncoordinated and scattered throughout the scientific literature without a clear epistemic goal or connection between the studies. Therefore, our main contribution to the scientific literature is to offer a road map to help solve problems in drug design, protein structures, design, and function prediction while also presenting the “state of the art” on research in the AI–PS binomial until February 2021. Thus, we pave the way toward future advances in the synthetic redesign of novel proteins and protein networks and artificial metabolic pathways, learning lessons from nature for the welfare of humankind. Many of the novel proteins and metabolic pathways are currently non-existent in nature, nor are they used in the chemical industry or biomedical field.
Introduction
Protein science witnesses the most exciting and demanding revolution of its own field; the magnitude of its genetic–epigenetic—molecular networks, inhibitors, activators, modulators, and metabolite information—is astronomical. It is organized in an open “protein self-organize, adjustment and fitness space”; for example, a protein of 100 amino acids would contain 20100 variants, and a process of searching–finding conformations in a protein of 100 amino acids can adopt ∼1046 conformation and a unique native state, the protein data exceeding many petabytes (1 petabyte is 1 million gigabytes) (Kauffman, 1992).
Therefore, the use of artificial intelligence in protein science is creating new avenues for understanding the ways of organizing and classifying life within its organisms to eventually design, control, and improve this organization. In this respect, protein synthesis is a case in point. Indeed, the discovery of the underlying mechanism of protein synthesis is an inter-field discovery, that is, “a significant achievement of 20th century biology that integrated results from two fields: molecular biology and biochemistry” (Baetu, 2015). More recently, the field of protein science is, in turn, another inter-field enterprise, this time between molecular biology and computer science, or better said, between a cross-functional team of researchers (biochemists, protein scientists, protein engineers, system biology scientists, bioinformatics, between others). Nowadays, it is possible to classify, share, and use a significant number of structural biology databases helping researchers throughout the world. Once the mechanism of DNA for protein synthesis is deduced, it will then be possible to replicate it via computational strategies through artificial intelligence (AI) and machine learning (ML) algorithms that can provide important information such as pattern recognition, nearest neighbors, vector profiles, back propagation, among others. AI has been used to exploit this novel knowledge to predict, design, classify, and evolve known proteins with improved and enhanced properties and applications in protein science (Paladino et al., 2017; Wardah et al., 2019;Cheng et al., 2008; Bernardes and Pedreira, 2013), which, in turn, makes its way to solve complex problems in the “fourth industrial revolution” and open new areas of protein research, growing at a very fast speed.
The techniques of machine learning are a subfield of AI, which has become popular due to the linear and non-linear processed data and the large amount of available combinatorial spaces. As a result, sophisticated algorithms have emerged, promoting the use of neural networks (Gainza et al., 2016) However, in spite of the large amount of research done in protein science, as far as we know, there are neither systematic reviews nor any biochemical meta-analysis in the scientific literature informing, illuminating, and guiding researchers on the best available ML techniques for particular tasks in protein science; albeit there have been recent reviews such as the work of AlQuraishi (2021), Dara et al. (2021), and Hie and Yang (2022), which prove that this inter-field is on evolution. By a biochemical meta-analysis, we mean an analysis resulting from two processes: identification and prediction. The former consists of identifying AI applications into the protein field where we classify and identify active and allosteric sites, molecular signatures, and molecular scaffolding not yet described in nature.
Each structural signature, pattern, or profile constitutes a singular part of the whole “lego-structure-kit” that is the protein space that includes the catalytic task space and shape space, which Kauffman (1992) defines as an abstract representation or mapping of all shapes and chemical reactions that can be catalyzed onto a space of task. The latter process is an analysis of the resulting predictions of structures, molecular signatures, regulatory sites, and ligand sites. Both processes are related to each other in the sense that the proteins in the identification process are searching targets of the 3D-structure for the prediction process that predicts the protein conformation multiple times from a template family or using model-free approach. The biochemical meta-analysis includes formulating the research question, searching and classifying protein tasks in the selected studies, gathering AI–PS information from the studies, evaluating the quality of the studies, analyzing and classifying the outcomes of studies, building up tables and figures for the interpretation of evidence, and presenting the results.
This study puts forward the use of ML classes and methods to address complex problems in protein science. Our point of departure is the state of the art of the AI–PS binomial; by binomial, we mean a biological name consisting of two terms that are partners in computational science as well as in biomedical or biotechnological science as a “two-feet principle” in order to understand, enhance, and control protein science development from an artificial intelligence perspective. Our cross-functional team aims at accelerating the steps of translating the basic scientific knowledge from protein science laboratories into AI applications. Here, we report a comprehensive, balanced systematic review for the literature in the inter-field and a biochemical meta-analysis, which includes a classification of screened articles: 1) by the ML techniques, they use and narrowing down the subareas, 2) by the classes, methods, algorithms, prediction type and programming language, 3) by some protein science queries, 4) by protein science applications, and 5) by protein science problems. Moreover, we present the main contributions of AI in several tasks, as well as a general outline of the processes that are carried out throughout the construction of the models and their applications. We outline a discussion on the best practices of validation, cross-validation, and individual control of testing ML models in order to assess the role that they play in the progress of ML techniques, integrating several data types and developing novel interpretations of computational methodology, thus enabling a wider range of protein’s-universe impacts. Finally, we provide future direction for machine learning approaches in the design of novel proteins, metabolic pathways, and synthetic redesign of protein networks.
Materials and Methods
A systematic review of the scientific literature found in the period (until February 2021) was carried out for this study (Figures 1–3) following the PIO (participants/intervention/outcome) approach and according to PRISMA declaration (Preferred Reporting Items for Systematic Reviews and Meta-Analyses) Supplementary. No ethical approval or letter of individual consent was required for this research.
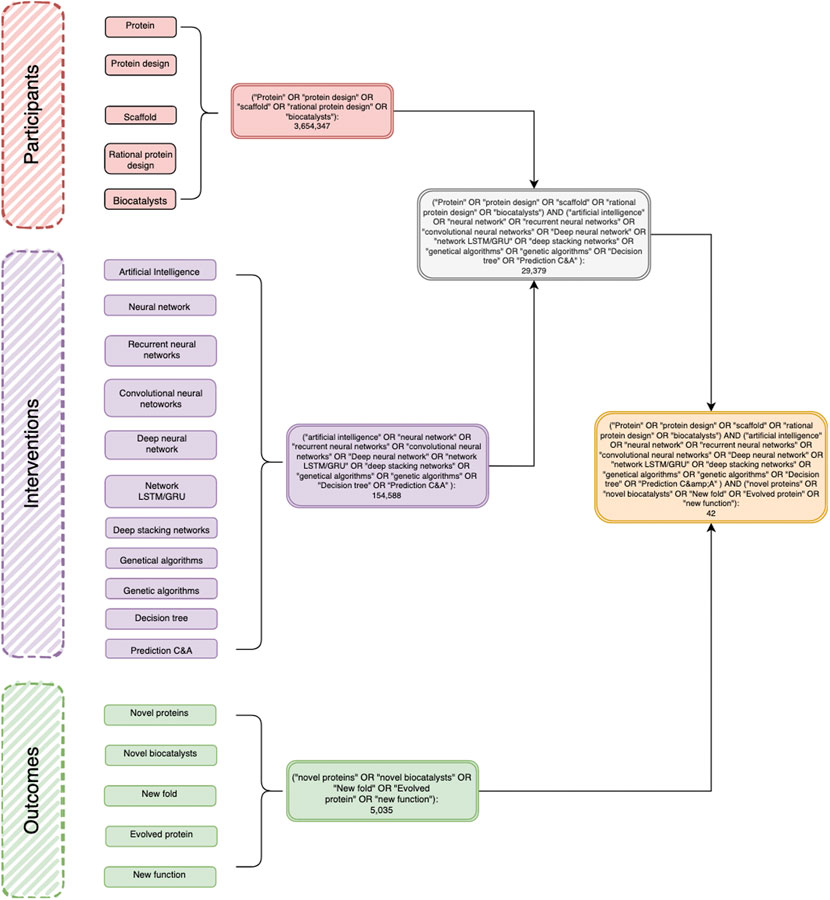
FIGURE 1. A representative decision diagram showing the articles retrieved using the PIO strategy in the PubMed database. P (participants): Protein, Protein Design, Scaffold, Rational protein design, Biocatalysts. I (intervention): Networks: Neural networks, Recurrent neural networks, Networks LSTM/GRU, Convolutional neural network, Deep belief networks, Deep stacking networks C5.0; Genetic algorithms; Artificial intelligence; Decision trees; Classification; Prediction C&A; Software: Weka, RapidMiner, IBM Modeler; Programming Languages: Python, Java, OpenGL, C++ Shell; Development platform: Caffe Deep Learning, TensorFlow, IBM Distributed Deep Learning (DDL); Paradigm: Supervised Learning, Unsupervised learning, Reinforced learning, new function.
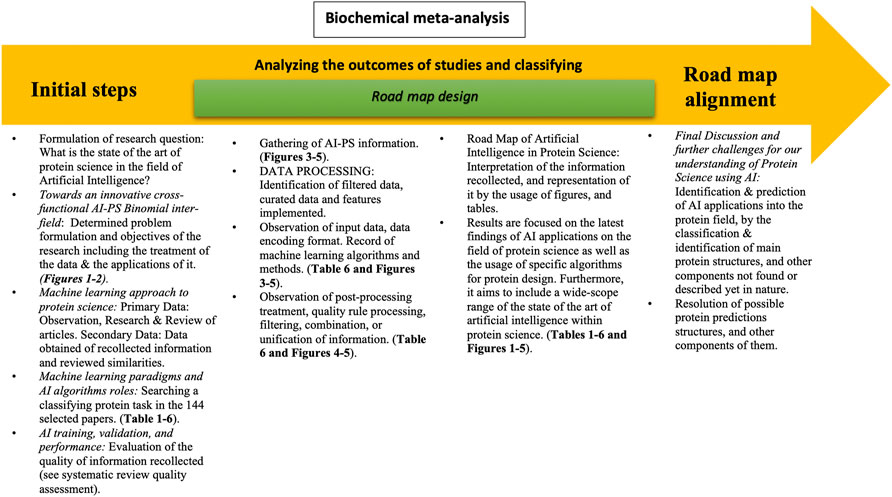
FIGURE 2. Flowchart of article scaffold. Representation of the process throughout the entire article. The biochemical meta-analysis consists of three main steps: the systematic review, the road map design, and the road map alignment. In the systematic review, the research question is formulated in order to set the basis and objectives of the project. It also includes the observation and synthesis of information obtained from a variety of articles and the correlation made between them. The latter followed by the quality evaluation of the collected information. The road map design consists of analyzing the outcome of the studies and classifying them, thus being able to interpret the information recollected and represent it through the usage of figures and tables. This aims to include a wide range of the state of the art or artificial intelligence. Finally, the road map alignment includes the final discussion and further changes for our understanding of protein science using AI and the resolution of possible protein science application targets.
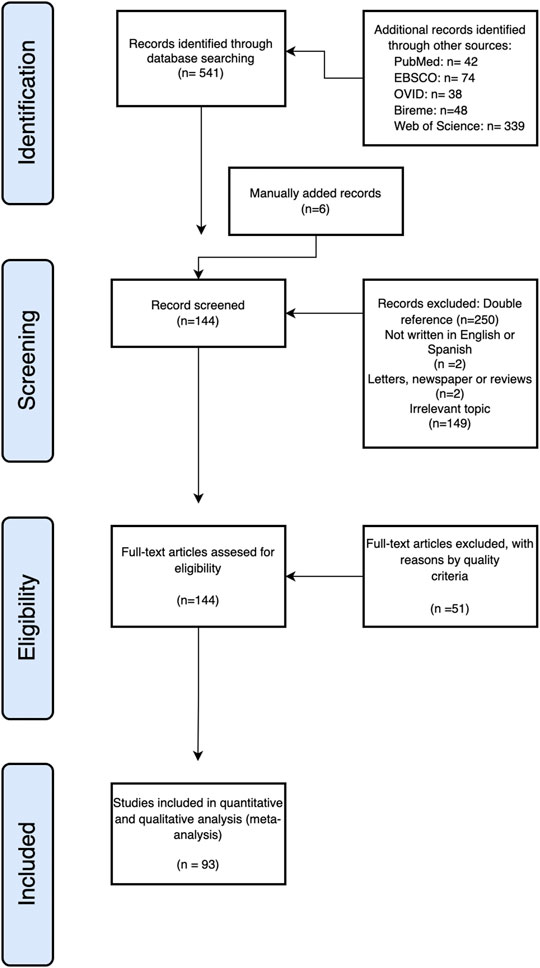
FIGURE 3. Flowchart of the review process. A PRISMA flowchart of the systematic review on AI for protein sciences.
PIO Strategy
One of the main objectives is to discuss new information in the latest findings about the functions of AI in protein design. Furthermore, this review and meta-analysis intend to include a wide scope of the status of artificial intelligence in protein science. The PIO (participants, intervention, and outcome) strategy was used to systematically search all databases and was the methodology to address the following research questions: What is the state of art in the use of artificial intelligence in the protein science field? What is the use of neural networks in the rational design of proteins? Which neural networks are used in the rational design of proteins? Protein design is currently considered a challenge. As artificial intelligence makes progress, this is presented as a solution to various issues toward addressing how this new branch can be used for the creation of high precision models in protein design. Following the PIO strategy, the next terms were used for the research.
Participants: articles about proteins and their MeSH terms in general were considered for inclusion; we gave special consideration to protein design and their related terms such as scaffold (as a main structure or template), rational design, and biocatalysts (as a main task target for protein evolution and design in the chemical–biotechnological industry and biomedical field):
• protein
• protein design
• scaffold
• rational protein design
• biocatalysts
Intervention: studies with any types of algorithms, software, programming language, platform, or paradigm using alone or in combination were selected.
Types of algorithms:
• neural networks
• recurrent neural networks
• network LSTM/GRU
• convolutional neural network
• deep belief networks
• deep stacking networks C5.0
• genetic algorithms
• artificial intelligence
• decision trees
• classification
• prediction C&A
Software:
• Weka
• RapidMiner
• IBM Modeler
Programming languages:
• Python
• Java
• OpenGL
• C++
• Shell
Development platform:
• Caffe
• DeepLearning4j
• TensorFlow
• IBM distributed deep learning (DDL)
Paradigm:
• supervised learning
• unsupervised learning
• reinforced learning
Outcomes:
• novel proteins
• protein structure prediction
• novel biocatalysts
• new fold
• evolved protein
• new function
Databases and Searches
The electronic databases used were PubMed, Bireme, EBSCO, and OVID. The concepts with similarity were searched with “OR,” and within the groups of each element of the PIO research, they were searched with the word “AND.” Next, a diagram was constructed in order to show the history of searches and concepts used (figure tree diagram). This figure describes in full detail the searching strategy in the PubMed database as well as all keywords used. Moreover, it includes the number of resulting articles. Subsequently, the results obtained from these searches were recorded. The references themselves were then downloaded into the Mendeley database. All references were taken, organized, and saved in Mendeley, eliminating duplicates for the final result.
Biochemical Meta-analysis
The biochemical meta-analysis included formulating the research question, searching and classifying protein tasks in the 144 selected studies, gathering AI–PS information from the 144 studies, evaluating the quality of the studies (as described in the systematic review, see flowchart of PRISMA), analyzing and classifying the intervention and outcome of studies (networks, software, programming languages, development platforms, paradigms, novel proteins, novel scaffold, new fold, etc.), and building up tables and figures for the interpretation of evidence and presenting the results.
By a biochemical meta-analysis, we mean an analysis resulting from two processes: identification and prediction. The former consists of identifying AI applications into the protein field: classify and identify active and allosteric sites, molecular signatures, and molecular scaffolding not yet described in nature, each of which constitute a single part of a grand-type Lego structure. The latter is an analysis of resulting predictions: structures, molecular signatures, regulatory and ligand sites, etc.
Biochemical Meta-Analysis and Designing the Road Map
PRELIMINARY: we determined the formulation of the problem and objectives of the research within the figure, which includes the treatment of the data and their applications. Note: the information was acquired from a list of various databases from which data were analyzed.
DATA COLLECTION: primary data: observation, research and review of articles. Secondary data: data of the reviewed articles and information shared among keywords.
DATA PRE-PROCESSING (ETL and training): identification of filtered data, curated data, and features implemented; machine learning input relationship with protein science servers.
DATA PROCESSING (training data and feature extraction): observation of input data and data encoding format. Record of machine learning algorithms and methods. Recognition of key information for processing data within databases.
DATA POST-PROCESSING: observation of post-processing treatment, rule quality processing, filtering, combination, or unification of information.
MEASURE: explanation of the process, the values of different metrics for the quantification of magnitudes, and the contribution for the completion within the process of information.
ANALYZE: identify the application of machine learning algorithm in which the input of the dataset to process data format, training set, and 3D structures.
IMPROVE: determine the set to whom these new forms will be applied in models of the researched data and contribute to future implementations in protein science.
Concerning the computational aspects as to how articles were classified, three initial divisions were made and are displayed in Table 1: Pre-process, process, and post-process, each of which contain, in turn, the following items:

TABLE 1. An overview of the included articles on study and algorithm features based in their characteristics, strengths, limitations, and measure of precision.
pre-process
database, pretreatment, and input
process
machine learning paradigm and input, algorithm and development software, three aspects of the neural network used (characteristics, strengths, and limitations) and output.
post-process
input and web server when applied.
Most of the research reported in these articles performs a pretreatment over the protein database used, that is, processes of randomization and training, in order to leave the data prepared for the computational process itself, for when the algorithm is to be executed on a software platform and within a particular machine learning paradigm (mostly supervised, unsupervised, and deep learning, as shown in Figure 4). We also reported special characteristics as well as strengths and limitations of the neural networks used. Finally, part of the post-process, when applied, concerns the web server where research results are stored. Moreover, some of these aspects are also registered in Tables 2–6 as well as some others (programming language and software license type).
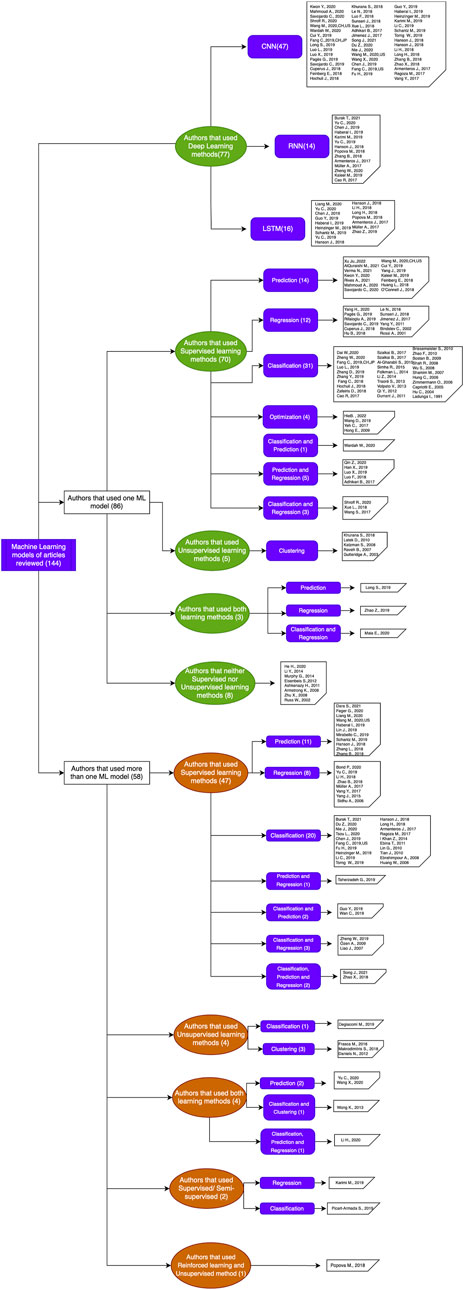
FIGURE 4. Machine Learning paradigms: superviser learning, unsupervised learning, reinforcement learning.
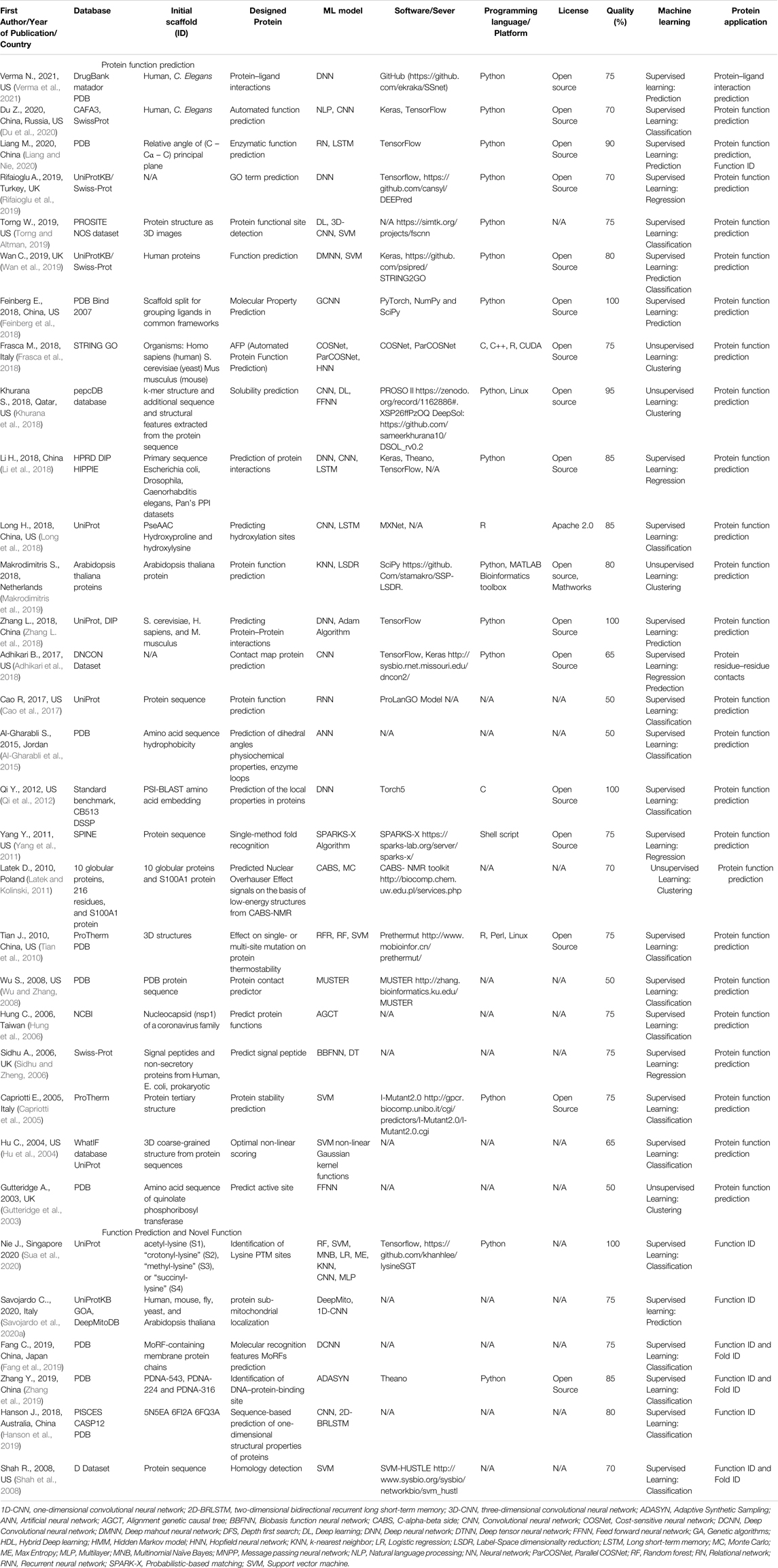
TABLE 3. An overview of the protein function prediction, function prediction, and novel function articles with the quality assessment.
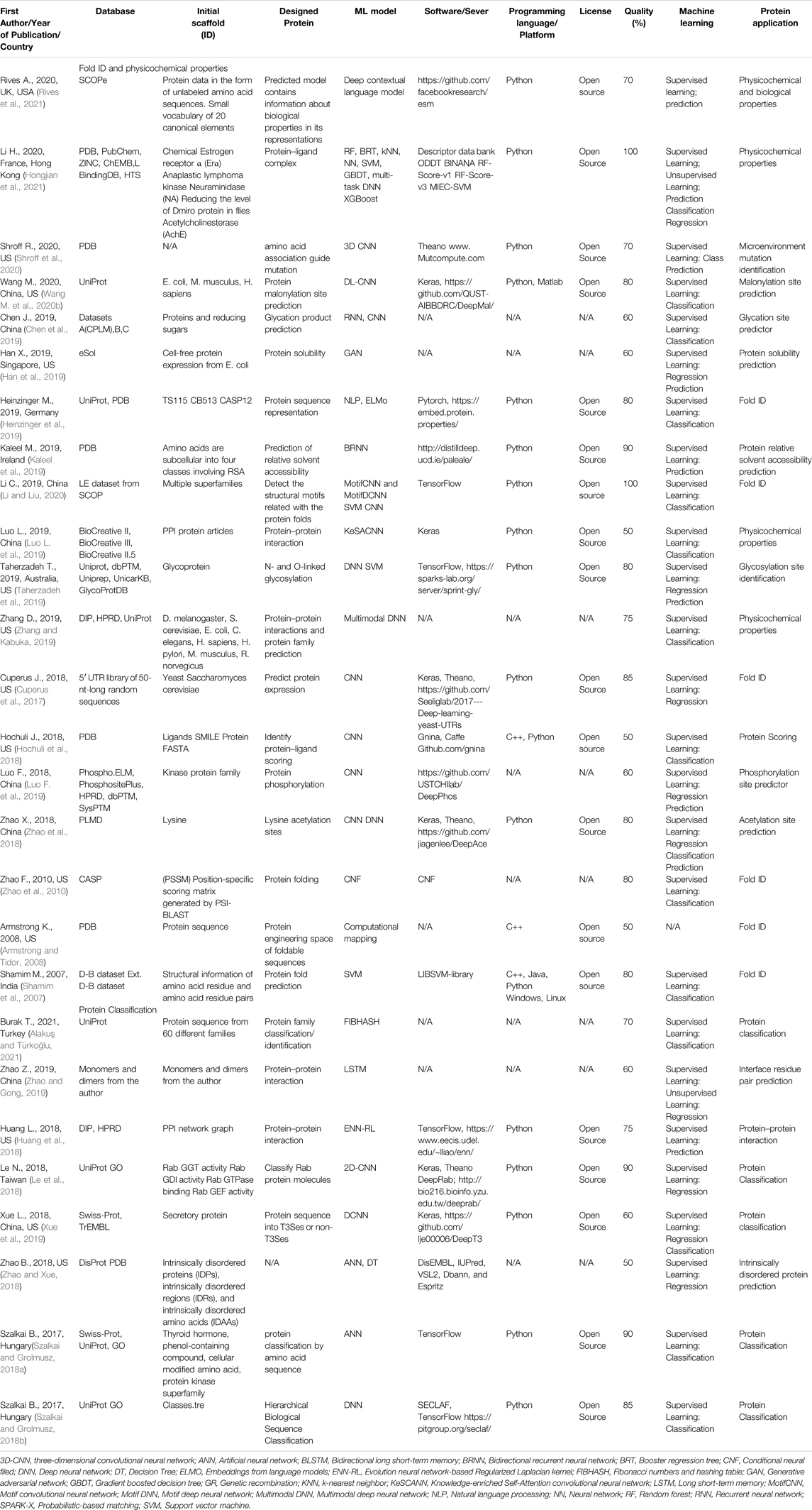
TABLE 4. An overview of the fold id, physicochemical properties, and protein classification articles with the quality assessment.
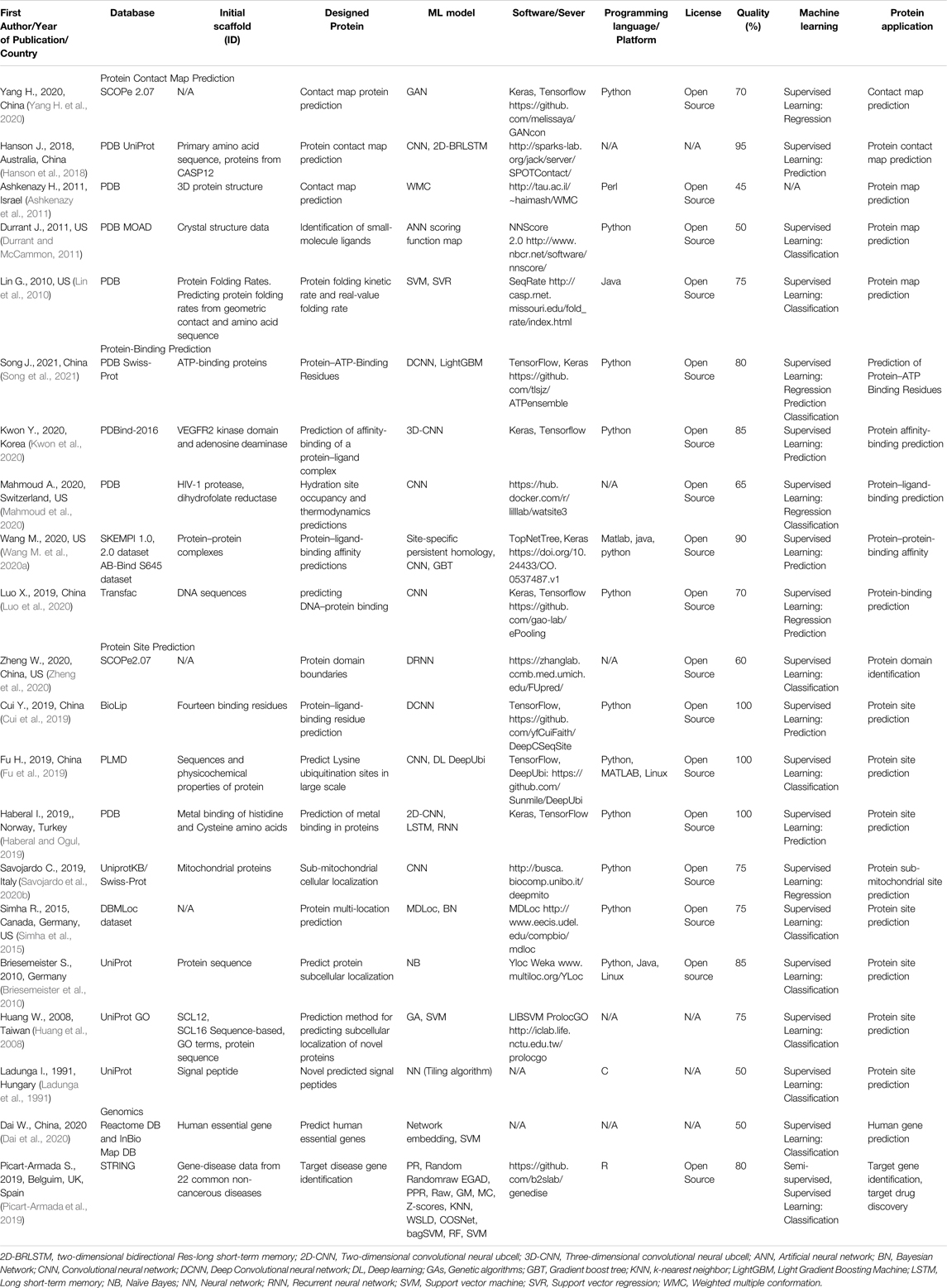
TABLE 6. An overview of the protein contact map prediction, protein-binding prediction, protein site prediction, and genomics articles with the quality assessment.
Results
Article Scaffolding
This article is arranged as follows (Figure 2): first, we provide a representation of the process in designing, preparing, and describing of the guideline throughout the article. Secondly, we review the presented formulation of the research question toward the determined problem formulation and objectives of the research, including the treatment of the data and the applications of it. Thirdly, the article processes the observation, research, and review of a series of articles to further study the data obtained and review similarities. Furthermore, the gathering of AI–PS information, within this processing of the identification of filtered data, curated data and features implemented, the observation of input data, data encoding format, recording of machine learning algorithms and methods, as so the post-processing treatment, quality rule processing, filtering, combination, or unification of information, which passes into the interpretation of the information recollected, and representation of it by the usage of figures and tables, portrays the results, which are focused on the latest findings of AI applications in the field of protein science as well as the usage of specific algorithms for protein design. Therefore, this aims to include a wide-scope range of the state of the art of artificial intelligence within protein science; this leads us to a latter analysis and discussion regarding the identification and prediction of AI applications into the protein field, by classification and identification of main protein structures, and other components not found or described yet in nature, and the resolution of possible protein prediction structures and other components of them are plausible outcomes of future research.
Toward an Innovative Cross-Functional AI–PS Binomial Inter-field
This systematic review and meta-analysis are focused on the latest findings of AI applications to the field of protein science as well as specific algorithms used for protein design. Furthermore, it aims to include a wide scope of the state of the art of artificial intelligence in protein science. PIO is the methodology used to address the following research question: What is the state of the art in the use of artificial intelligence in the protein science field? Figure 1 shows the total number of articles retrieved using the PIO strategy in the PubMed database.
The systematic review process began with 541 references obtained from five electronic databases: 42 were from PubMed, 74 were from Ebsco, 48 were from Bireme, 38 were from OVID, and 339 were from Web of Science. In the first screening, 403 articles were removed: 250 articles with a double reference; 2 not written in Spanish or English; 149 whose topic was irrelevant to the review; and two newspapers, letters, or reviews. This election process left 138 references, and manually we added 6, thus getting a total of 144 articles for the review (Figure 3).
A second screening (eligibility) was performed using the following set of quality criteria:
1. Clear research questions and objectives.
2. Definition of the measured concepts.
3. Reliability and feasibility of the instruments to be measured.
4. Detailed description of the method.
5. Scaffolding and enhanced protein information.
6. Characteristics of scaffolding and its realization.
7. Appropriate system and learning approach.
8. Journal impact.
A total of 93 articles were included for further analysis, and 51 studies were removed based on quality criteria.
Machine Learning Approach to Protein Science
Proteins are influenced by epigenetic phenomena (cellular stress, aging, etc.) because of their multiple structure-folding-function within protein science (PS), phenomena that can be challenged through the use of artificial intelligence (AI).There are several questions within this interdisciplinary approach such as How do proteins evolve? How do proteins fold and get their tridimensional structure? What are their networks within proteins? Given the astronomical numbers of possibilities for protein structures, configurations, and functions that require the use of AI as a tool to fully understand protein behavior.
A total of 144 articles were assessed for quality (Tables 2–6) resulting in 93 articles (Table 1), those articles that were greater or equal to 75 in the quality percentage qualifications were kept for the final biochemical meta-analysis. For this review and meta-analysis, we identified five main applications of AI into PS (Tables 2–6 and Figures 4–6)
I. Protein design and drug design (Table 2)
a) De novo protein design.
b) Novel biocatalyst design.
c) Novel function and ligand interaction.
d) Evolution of non-existent proteins in nature.
e) Chemical structure and properties.
f) Drug–drug interaction.
g) Drug–receptor interaction.
h) Drug effects.
II. Protein function, function prediction, and novel function (Table 3)
a) Protein–ligand interactions.
b) Hydroxylation site prediction.
c) Prediction of the local properties in proteins.
d) Enzymatic function prediction.
e) Predicting protein–protein interactions.
f) Function prediction.
g) Molecular property prediction.
III. Fold ID, physicochemical properties, and protein classification (Table 4)
a) Fold Id.
b) Glycation site predictor.
c) Phosphorylation site predictor.
d) Protein–protein interaction.
e) Intrinsically disordered protein prediction.
IV. Protein structure prediction (Table 5)
a) Protein structure prediction: primary, secondary, and 3D-structures; domains, active sites, allosteric sites, and structural feature prediction.
b) Protein structure classification: folds, structural families, intrinsically disorder proteins, etc.
c) Protein–protein interactions and protein networks.
d) Protein–ligand interactions: substrates, inhibitors, activators, ions, etc.
V. Protein contact map prediction, protein-binding prediction, protein site prediction, and genomics (Table 6)
1) Contact map prediction.
2) Protein sub-mitochondrial site prediction.
3) Genomics.
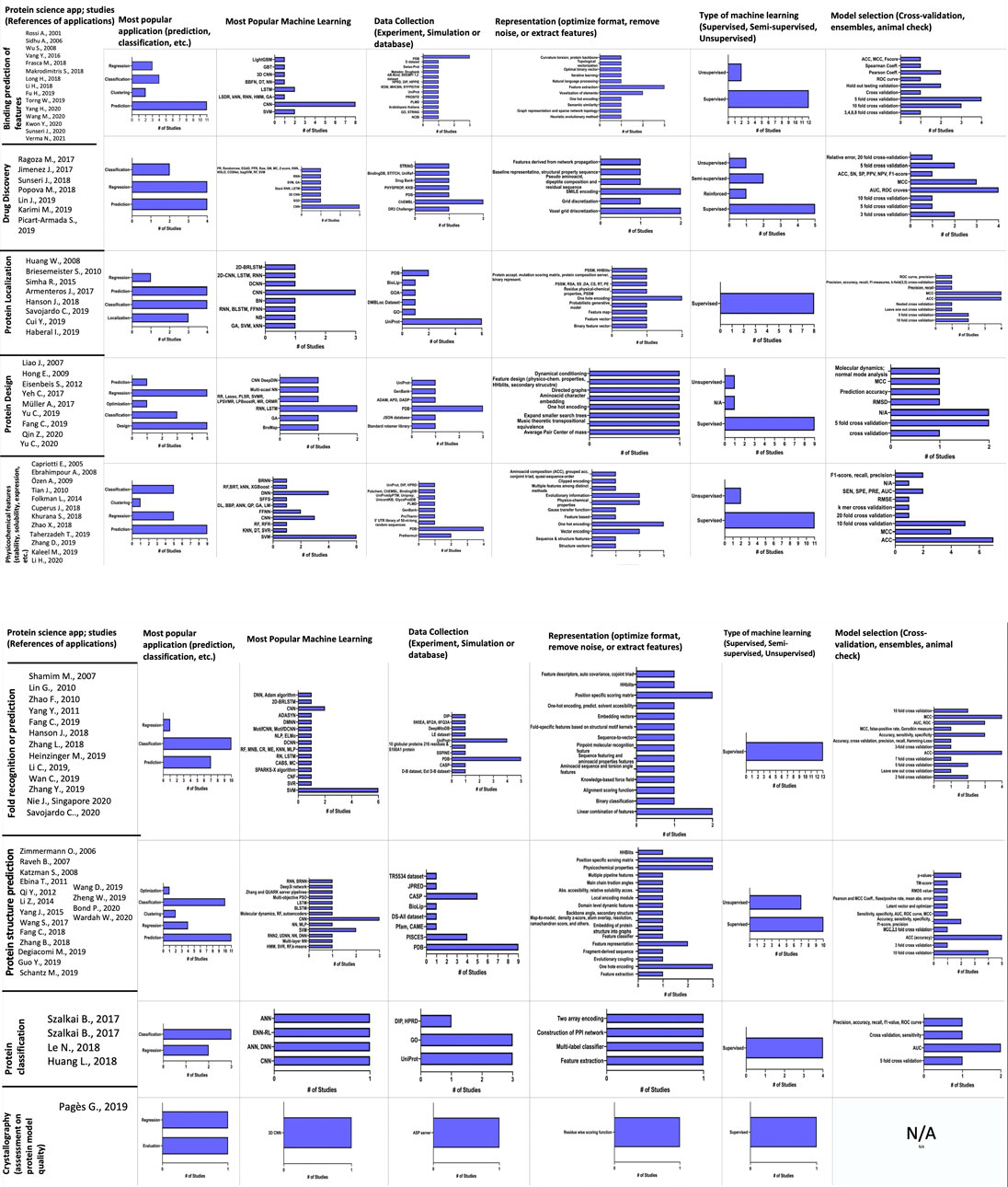
FIGURE 5. Machine learning and artificial intelligence applications to protein sciences. Information includes the number of studies, applications, databases, methods, and validation used.
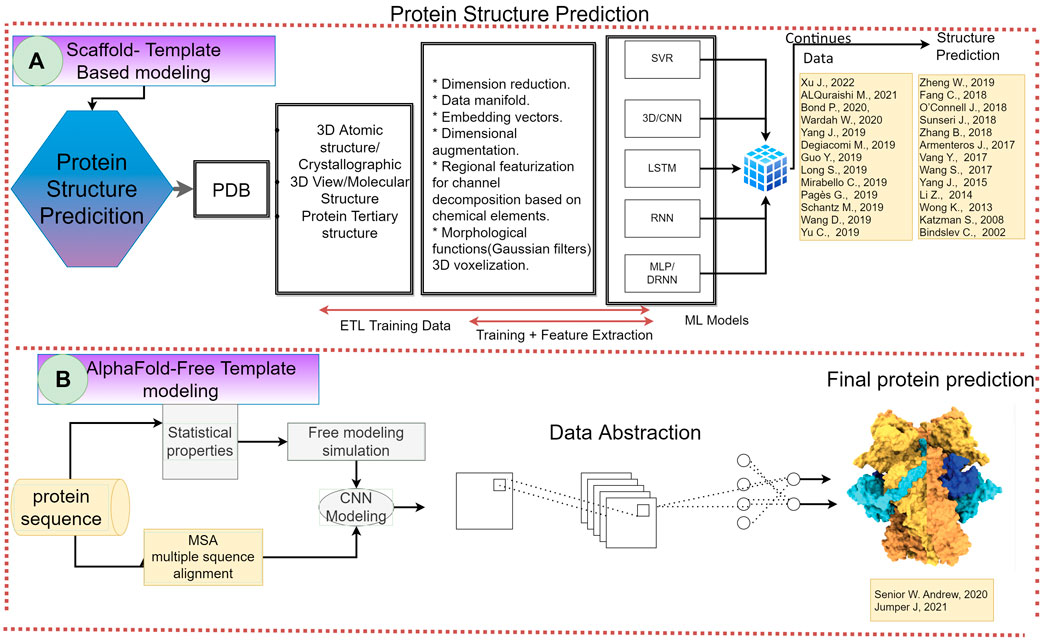
FIGURE 6. Representation of the specific case for protein structure prediction in the supervised learning framework. Revealing the most common flow followed by the studies analyzed. From extraction, training data, feature extraction procedures and data continuity. Including the PDB database, the most common supervised algorithms, SVM, SVR, 3DCNN.
The 40% (57/144) of the protein studies by AI applications were the following ones: myoglobin, silk protein, amyloid proteins, Rab family, cathepsin S family, kinases family, K proteinase, barnase, apolipoprotein family, protein DND_4HB, and antimicrobial peptides. Studies in enzymes should be pointed out, oxidoreductases, transferases, hydrolases, lyases, isomerases, ligases, NOS (nitric oxide synthase), lysozyme, which are included in the columns of the initial scaffold (Tables 2–6). These proteins are very useful in the industry as well as in the biomedical fields. With respect to the type of organisms, the more explored are the following ones: E. coli, Drosophila, Caenorhabditis elegans, Homo sapiens, S. cerevisiae yeast, Mus musculus (mouse), Geobacillus, and Coronavirus.
Tables 2–6 present the lists of the most commonly used databases in AI applications on PS. Of all the studies reviewed, the single use of main databases and datasets used is as follows:
1) PDB (30/144) 21%.
2) Author’s dataset construction (21/144)15%.
3) UniProt either UniProtKB or UniProtKB/SwissProt (12/144)8%.
4) CASP (critical assessment of protein structure prediction) database (5/144)3%.
5) SCOP (structural classification of proteins) (4/144)3%.
6) N/A, GenBank (4/144) 3%.
7) Protherm (3/144) 2%.
8) BioLip (biologically relevant ligand–protein) (2/144) 1%.
9) PLMD (protein lysine modifications database) (2/144) 1%.
10) And each of the next databases ChEMBL, eSol, GEO, DSSP, Drugbank, BioCreative, Transfac, STRING, BRENDA, SPINE, PISCES, NCBI, D3R Grand challenge 3, and KEGG with a (1/144)1%.
From the studies reviewed, (23/144), 16% use two databases. Of these, the latter (11/23) 48% uses a combination of the PDB and HSPP, PISCES, ProTherm, MOAD, SPx dataset, ChEMBL, DisProt, and UniProt/SwissProt; (4/23)17% use a combination of the GO database with UniProt or STRING; (4/23)17% uses a combination of the UniProt/SwissProt database with ENZYME, DIP, TrEMBL, and CAFA database; and a (2/23)9% combination among DIP, HPRD, SKEMPI database, and SPx dataset. The rest (24/144)17% belongs to a combination of three or more databases with PDB, UniProt, among others.
Moreover, several authors (Shamim et al., 2007; Simha et al., 2015; Yang et al., 2015; Li et al., 2018; Torng and Altman, 2019) focused on using previously constructed datasets, while others chose the creation of their own, based on their own design and outcome, for example, NOS, PPI’s, SPX, DBMLoc, D-B, and Extended D-B (Tables 2–6 and Figure 5).
The following tables show the principal protein categories that were found in this study. Table 2 shows the result of each of the 38 articles that were considered in the protein and drug design category.
Table 3 shows 26 studies that are related to protein function prediction and 6 studies related to function prediction and novel function.
Table 4 shows 19 studies that are related to fold ID and physicochemical properties and 8 studies related to protein classification.
Table 5 shows 26 studies that are related to protein structure prediction.
Table 6 shows five studies for protein contact map prediction, five studies for protein-binding prediction, nine studies for protein site prediction, and two studies for genomics.
Table 1 shows the overview of the extracted information of the selected studies based on the quality criteria.
Machine Learning Paradigms and AI Algorithm Roles
The most applied approach we found as a result of our review and meta-analysis corresponds to supervised learning (123/144)85%, which focuses on classification algorithms (CNN, NB, KNN, RF, SVM, etc.) and regression algorithms (SVR, RFR, DT, ANN, DNN, etc.) that are used for a variety of tasks: detection of functional sites, hydroxylation sites, amino acid composition, DNA expression sequences, protein interaction, biomarker finding, protein design, drug design, 3D structure prediction, and protein folding (Tables 2–6 and Figures 4, 5). Within supervised machine learning (123), we found that classification techniques overrule, by far, regression ones (31/123) (for reference, see Tables 2–6). On a closer look, we see that these methods are generally very good at prediction tasks, although complexity may be significantly increased by the execution time required, something that is often reported as a drawback of this method (AlQuraishi, 2021).
In contrast to supervised learning, it is only (17/144)12% focusing on unsupervised learning, using clustering algorithms (CNN, FFNN, LSDR, DL, HMM, MRF, NN, etc.) for various purposes, such as protein solubility prediction, protein prediction of new functions, discovery of DNA motifs, detection of protein structures, and prediction of the nuclear Overhauser effect at low energies. Of the eight articles using this approach, two of them report an improvement in performance as an advantage, one of them in time reduction (Frasca et al., 2018) and the other one in the acceleration of automated protein function prediction methods in general (Makrodimitris et al., 2019). At the same time, however, a disadvantage reported is that time execution may be increased, a fact that should not surprise us, for it is well known that unsupervised learning algorithms are characterized by being computationally very complex methods (Table 1 and Figures 4–7).
On the other hand, supervised machine learning is used just a little more than deep learning techniques. Moreover, it is interesting to note that roughly (77/144)53% of the deep learning articles combine two clustering algorithms: CNN (47/77)61% and LSTM (16/77)21%. Of course, some articles put forward optimization procedures in an algorithmic genetic fashion (Figures 4–7).
Regarding hybrid algorithms using neural networks, we found that all 11 articles explicitly stating their use of hybrid algorithms belong to the deep learning paradigm, combining CNN and LSTM or RNN and CNN. One of them (Almagro Armenteros et al., 2017) goes even further; in that, it uses a combination of these two neural networks to predict protein subcellular localization and then an attention mechanism to identify protein regions important for subcellular localization (Table 1 and Figures 4–6).
It is interesting to note as well that nine articles are used for prediction (glycation product prediction (Chen et al., 2019), protein secondary structure (Guo et al., 2019), prediction of metal binding in proteins (Haberal and Ogul, 2019), compound–protein affinity prediction (Karimi et al., 2019), prediction of protein structural features (Klausen et al., 2019), protein contact map prediction (Hanson et al., 2018), prediction of protein interactions (Huang et al., 2018), predicting hydroxylation sites (Long et al., 2018), and predicting protein subcellular localization (Almagro Armenteros et al., 2017)), of which two perform prediction from original sequences (Almagro Armenteros et al., 2017;Li et al., 2018).
Moreover, one of them highlights that one of its applications is for the design of new drugs and one of them performs this task (Karimi et al., 2019).
It is tempting to put forward the claim that hybrid algorithms in deep learning are very good for prediction tasks as well as for applications in the new drug design. It is noteworthy to mention that these articles belong to the last 3 years of our revision, something that suggests that there is a tendency for the use of hybrid methods in the near future (Table 1).
AI Training, Validation, and Performance
Validation process allows obtaining a quantitative measure of the models’ efficiency. In this systematic review, several methodologies were used to train and validate in the machine and deep learning proposed by means of hold-out and k-fold cross-validation; The most utilized was the k-fold cross-validation, each one with a different folding proposal, e.g., 2-, 3-, 5-, and 10-fold (Szalkai and Grolmusz, 2018a), trained and validated its algorithm utilizing two validations: 3- and 5-fold cross-validations. Several articles used a graphics processing unit (GPU) that was employed to accelerate the deep learning training and validation process. The most utilized AI algorithm in these articles was CNN, with a 33% occurrence, followed by DNN with 9%, both programmed with Python. The performance of the AI algorithms for protein design was evaluated using parameters such as sensitivity, specificity, true-positive rate, false-positive rate, accuracy, recall, precision, F1-score, area under the curve (AUC), receiver operating characteristic (ROC) curve, and Matthew’s correlation coefficient (MCC). For the case of the hold-out validation, a percentage of the data that is taken and that percentage is randomly removed from the dataset is selected. This methodology, in particular, is computationally very simple; however, it suffers from a high variance because it is not known that data will end up in the test set or in the training one and of the importance that these data might have. In hold-out validation, datasets, which for this review are the databases of proteins, genes, peptides, etc. (see Tables 2–6 and Figures 4–6), are randomly divided into two partitions with different proportions (50, 70, or 75% training—50, 30, or 25% validation), which are mutually exclusive. The first part of the database is used to feed the input vectors of the methods and train the machine or deep learning algorithms, while the rest is used to evaluate and validate the results obtained with their proposed algorithms. In contrast, with this type of validation technique, hold-out takes a long time for computational processing, especially for large datasets, in particular case, the large protein databases. As a result of our meta-analysis, we found the use of the hold-out methodology to train and validate their AI proposals, as CNN, RNN, LSTM, and FFNN (Tables 1–6 and Figures 4–6) in the prediction of expressions, interactions, and subcellular localization of proteins and also in the prediction of the peptide binding.
Another technique for evaluating the performance of AI methods, particularly for large databases such as protein design, is cross-validation. Cross-validation is a technique used to (generally) obtain the ability of a model to fit an unknown dataset given a collected dataset. In this context, the k-fold cross-validation is an iterative process that consists of dividing the dataset randomly into k groups of approximately the same size. In this sense, although not all possible combinations of sets are examined, an estimate of the average accuracy more than acceptable can be obtained by training the model only k-fold. The first set is used to train the AI models and the other is used to test and validate them, doing this process k times using a different group for validation in the iteration. Although cross-validation is computationally an intensive method of training and validation, its advantages are the reduction of computational time because the process is repeated k times, where all the data are tested once and used for training, maintaining a reduced variance and bias. Of the total 93 articles in this review, 41 of them (47%) used the following cross-validation schemes: leave-one-out, 2-fold, 3-fold, 4-fold, 5-fold, 6-fold, 7-fold, 8-fold, 10-fold, and 20-fold cross-validations. For most of them, the use of 5-fold and 10-fold cross-validations to analyze the performance of their AI proposals predominated, with 16 and 17 articles, respectively. This method was preferred for the evaluation to the performance of CNN and SVM algorithms, with databases such as PBD, ProTherm, UniProt, GO, and ChEMBL. Additionally, in seven articles (17%), they carried out various types of cross-validations to obtain more information on the performance of their proposals. Another variant to evaluate performance was observed in three articles (7%), which combined the use of both hold-out and cross-validation methodologies in their proposals, which provide them more effective comparison of results in terms of validation schemes.
In contrast, in 22 articles of this review, 25% did not mention neither their training methods nor the validation performed to evaluate the performance of their algorithms used. Likewise, 7% of the articles evaluated their methods using various types of cross-validations at the same time to obtain more information on the performance of their proposals, e.g., 4-fold, 6-fold, 8-fold, and 1-fold, or 3-fold, 5-fold, 7-fold, and 1-fold, or 10- and 20-fold, for databases of PDB, UniProt, GO, ChEMBL, ProTherm, PISCES, GenBank, STRING, and new databases as NOS, SPx, D-B, and Ext D-B.
In general, the performance of all proposed AI algorithms was evaluated using several parameters such as sensitivity, specificity, true-positive rate, false-positive rate, accuracy, recall, precision, root-mean-square error (RMSE), R2, F1-score, area under the curve (AUC), receiver operating characteristic (ROC) curve, and Matthew’s correlation coefficient (MCC) (Table 1).
Of the 87 articles selected as finalists, we have the following: 32 use one single algorithm and 55 use a combination of two or three algorithms sequentially. In machine learning, we found 30; in deep learning, we found 20 applying machine learning (SVM); 11 deep learning (RNN); and 6 using optimization through genetic algorithms.
Regarding the programming language in which each study was developed, we found 47 articles do not specify what language they are based on, 75 articles are based on the Python language, of which 57 are based entirely on Python and 18 are in combination with other software; see Tables 2–6.
Twelve articles are based on the C++ language of which only three are based exclusively on that language and nine in combination with Python, with C, R, and CUDA and C++ language in the Linux environment.
Other nine articles are based on MATLAB of which only four are based exclusively on that language and five in combination in conjunction with Python and Bioinformatics and with Python and C++.
Six articles are based on the C language of which three are based exclusively on that language and three in combination in conjunction with C++, R, and CUDA, with Java and Python and one with Linux and Windows environment.
Finally, seven articles are based on the Java language of which two are written exclusively in this language and five in combination with TensorFlow and with C and Python.
Regarding software licenses, 90 articles were found to be Open Source. An article is licensed by Neural Power version 2.5. One article specifies an open license type belonging to IBM and GNU, respectively. Unfortunately, 45 items did not specify the type of license they own.
Road Map of Artificial Intelligence in Protein Science
The goal of this analysis is to provide a road map to apply machine learning and AI techniques in protein science. One of the results of our meta-analysis, for example, in protein structure prediction, is shown in Figure 6 in which we can observe the two main strategies for protein structure prediction. In Figure 2, we show the scaffold-template-based modeling that is the most commonly used for the scientist in this field with very good results. However, recently Senior and collaborators using a free modeling approach successfully developed an AlphaFold algorithm using a deep neural network. They generated an outstanding accuracy of the 3D structure of a protein with an unknown fold in CASP14 (Senior et al., 2020). This led to an unsolved big question about the importance of the starting point in protein structure prediction, in particular, and in protein science, in general.
The road map of this research is an evolving and a dynamic process (Figure 7). It begins by obtaining information from a list of several databases, followed by a pre-treatment step over the extracted data, including those steps for eliminating redundancies within sequences, structure threshold based on RMSD values, and the like. Further steps contribute to the required pre-processing to complete the reporting process, and then proceed to the data process of the information itself, which includes the input data and the application of the machine learning algorithm, in which the input data are set to be processed into FASTA sequences, training sets, or 3D structures, depending on the function of algorithm in turn. The algorithms used fall into four categories: supervised learning, unsupervised learning, deep learning, and optimization, where each of these categories include a set of their own subparts, which are then combined and configured to predict new ways to model previous data and contribute to future implementations in protein science. The post-processing of data and the support of the new data acquired are made up of models and sequences that were loaded on the platforms to servers such as “DeepUbi, DeepSol, COSNet, Gnina, among others”, in which these servers are used for the storage or implementation of their respective methods. Figure 7 shows that more than half of the reported research completed the three pre-process, process and post-process steps we set forward, so this sequence may be applied to protein science including protein design, classification, physicochemical properties, functionalities, folding properties, and new functions such as homology prediction, domain prediction, subcellular localization, drug design, sensitivity, and other enhancers that can provide new catalysts and new functions, all of which provide any future development for biomolecular enhancement within protein science through machine learning. Model development is intrinsically related to the protein application to be developed. Data extraction varies depending on the architecture of the model to be developed since the data become more complex as the transformation, training, and feature extraction process unfold. The extraction ranges from obtaining the amino acid sequence, secondary structure to the 3D atomic model, using the atomic coordinates. Transforming data emphasizes on performing an adequate filtering for the use of the information for the training of the model, which leads to the feature extraction for the use of machine learning model and finally generating a final output. The process road map includes the fusion of these different applied AI learnings, models, and classifications into a connected deep learning layer that will be included in future research and test datasets to cover the terms of AI science, proteins, and their applications.
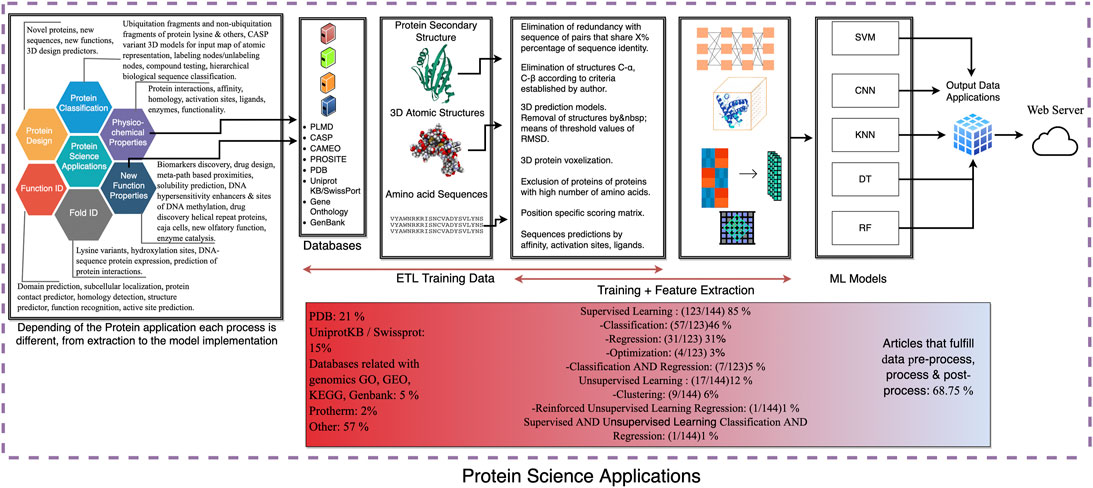
FIGURE 7. Representation of the whole AI process based on the selected protein application. The process amalgams several steps: protein application (protein design, protein classification, protein prediction, etc.), extraction (selection of database), transform (code development and filtering), and load (input of the training data) (ETL) for the training data and the feature extraction procedure is the building of the machine learning network. Outcome step and a proposal server application.
Final Discussion and Further Challenges for Our Understanding of Protein Science Using AI
Novelties and Future Direction in the Binomial PS-IA Research
The protein science field has great expectations on ML methods as indispensable tools for the biomedical sciences as well as for the chemical and biotechnology industry, for applied research is moving toward synthetic organisms with artificial metabolic networks, regulators, and so on, creating synthetic molecular factories. The binomial PS-IA research is evolving and strengthening, as shown in the Results section (Tables 1–6 and Figures 4–7). Our research reveals that road maps are most needed to solve complex problems in PS, guiding the exploration into the protein universe. As depicted in Table 1, ML techniques, which are used nowadays, are tailored to the expected results; Tables 1–6 display an array of networks of several solving problem methods, hence showing that guidance is needed in the form of road maps.
It is important to emphasize that in order to design a model algorithm bank functioning as a kit-tool, it is essential to understand the source from which the data are obtained and then used to train each model. The studies analyzed solve classification, regression, and optimization problems. As depicted in Table 1, models providing a solution make use of probabilistic inference, functions, activation functions, reduction of the hierarchical order, and logical inference. These results support the fact that machine learning models are heterogeneous, time demanding to design, and correctly evaluate complex models—since the result may not always be as expected or the method may not be carried out successfully. As illustrated in Table 3, there are some physical limitations blocking the full execution of the various models or algorithms, for example, when there is no appropriate computational equipment. Not surprisingly, several authors report that executing a model requires a high demand on execution time, computational power, extensive time to correctly evaluate the model, large memory consumption, and optimization toward GPUs (Frasca et al., 2018; Almagro Armenteros et al., 2017; Yeh et al., 2018; Jiménez et al., 2017; Lin et al., 2010). Another crucial aspect mentioned in Table 1 is the lack of input data to train the model, something that influences the model’s precision and accuracy (Pagès et al., 2019; Cuperus et al., 2017; Folkman et al., 2014; Qi et al., 2012). Moreover, there are also limitations in model construction, such as errors in the training process, manual intervention of data, overadjustment of the model, and an inadequate algorithm construction. In the studies analyzed, there are cases in which there is no description regarding the performance of the comprehensive models, generating gaps in the understanding of the behavior of the algorithms or models, like whether they are deterministic (Long et al., 2018; Ragoza et al., 2017; Makrodimitris et al., 2019). As stated in the ML and AI Algorithm section, supervised learning is the most used method, something that highlights the use of classification algorithms. Moreover, there seems to be a current trend to solve problems in protein science using techniques that require a cross-functional group of scientists, something that, in turn, highlights the fact that there is plenty of unexplored terrain in the use of unsupervised machine learning.
An interesting finding is the implementation of free code and software, as shown in the AI Training, Validation, and Performance section. Our results exhibit a tendency to create models with transparency, which means that every study implemented in a public server has access to all new models created. Another crucial result is the one depicted in the Road Map of Artificial Intelligence in Protein Science section, which is an abstraction that reduces the design of an artificial intelligence model to be used in the resolution of a specific problem in protein science. The whole process follows three steps directed to build a competent model; these steps are 1) the procedure to obtain raw data and which type of processing should be followed for the model to be adequate, 2) the type of algorithm that may be used depending on the complexity of the problem, and finally, 3) the interpretation of results.
Overall, AI displays a window of opportunities to solve complex problems in PS because of its potential in finding patterns and correlating information that requires the integration of protein data exceeding many petabytes. However, we are still far away from solving all the protein tasks computationally. As a result of our biochemical meta-analysis, we showed that AI applications are strongly directed to function identification and protein classification (Tables 1–6), for machine learning models and methods are heterogeneous and do not always draw a clear line as to whether a process should go in a certain sequence (Table 1 and Figures 4–7). It should also be noted that there is no optimal method, which is why applications have different purposes and conditions, suggesting that algorithms must be customized based on the expected outcome or query (Table 1).
The evaluation accuracy horizon is an open epistemic horizon, as shown in Table 1: the metrics for ML methods used in several applications are limited; there are no reported research articles using random forest, in which the cross-validation is unnecessary. In summary, none of the studies reported explicitly use robustly validated methods.
We end by commenting on a key problem in the binomial AI–PS. As well known, it is not possible to work directly with the protein sequences. To tackle this challenge, several studies address this limitation by representing the sequence of a protein as an input to the deep learning model (Almagro Armenteros et al., 2017; Long et al., 2018; Fu et al., 2019). Moreover, given some featured procedures comprising what may be called the coding architecture, which is based on creating a specific-weight matrix or a bit vector that represents the sample. This practice was observed in some articles (Cuperus et al., 2017; Jiménez et al., 2017; Khurana et al., 2018; Le et al., 2018) that work with 2D convolutional neural networks in which the authors reported an increase in sensitivity and precision when using indexed datasets. A similar abstraction was observed in 3D convolutional neural networks since the structural representation of a protein is not a rotational invariant; several authors (Jiménez et al., 2017; Ragoza et al., 2017; Hochuli et al., 2018; Pagès et al., 2019; Sunseri et al., 2019; Torng and Altman, 2019) propose using a volumetric map divided into voxels centered on the backbone atoms, representing the physicochemical properties of proteins.
Regarding other review articles along the lines we have followed, the closest we found is the one by Dara et al. (2021). This review article is restricted to drug discovery, one of the five applications we analyzed (genomics, protein structure and function, protein design and evolution, and drug design).
Of a total of 38 articles we presented in Table 2 concerning protein and drug design, only 11 of them were about protein design, so the comparison is not at all fair between these two articles, as far as the analysis of the bibliography analyzed is concerned. However, we share with these authors part of the challenges for researchers in this area: data quality as well as the heterogeneity of databases to be searched for.
Optimization and the characteristics of a prediction must be carried out with a few design considerations, including how to represent the protein data and what type of learning algorithm to use. These form the establishment of a priority acquisition, standard acquisition, etc., and the generation of a protein based on a base model, with the aim that one day it would be possible to have controllable predictive models that can read and generate outputs in a consensual terminology, as revised in Hie and Yang (2022). Clearly showing a replacement of conventional methods to the use of machine learning algorithms (neural networks), attributed to improvements in design, computational power, etc., the result of a machine learning algorithm is not deterministic, but rather, it is intended to perform transformation functions in relation to the complexity of the data, as depicted in AlQuraishi (2021). There are volumes and volumes of empirical protein data. It is extremely difficult to synthesize such data for correct use in existing algorithms; however, machine learning has helped to compile a large number of methodologies, considering specific assumptions. Nevertheless, most of the empirical methodologies to demonstrate that drugs are safe and effectively continue to be used since there is a gap in the understanding of how the learning transmission of the data to the model is carried out (Dara et al., 2021).
In order to close our reflection as a research team, we believe that a landmark for the epistemic horizon in research is the reassurance that cross-functional groups of scientists from several academic disciplines, in this case including the participation of experts from the natural sciences (organic chemistry, physics and chemistry of proteins, molecular and structural biology, protein engineering, systems biology, microfluid chip engineering, and nanobiotechnology), together with those in computer science (artificial intelligence, knowledge engineering) promote the innovation process in tecno-sciences by combining tacit and explicit knowledge, sharing skills, methodologies, tools, ideas, concepts, experiences, and challenges to fully explore the binomial AI–PS promising area of research (Hey et al., 2019; Mataeimoghadam et al., 2020; Senior et al., 2020; Tsuchiya and Tomii, 2020). A very recent successful case study that highlights this approach is the team of creators of system Alphafold (Senior et al., 2020; AlQuraishi, 2021), one which in the CASP (Critical Assessment of Protein Structure Prediction) competition of three-dimensional protein structure modeling were able to determine the 3D structure of a protein from its amino acid sequence. By doing so, this group of researchers solved one of natural science’s open (until now) and most challenging problems using a deep learning approach combining template-based modeling (TBM) and free modeling (FM). The key point is that the neural network prediction encompasses backbone torsion angles and pairwise distances between residues (Senior et al., 2020). At the dawn of the year 2021, this peak of the iceberg brings fresh air and a great power to the protein science field, in particular, and to the life-sciences more broadly, encouraging the new generation of scientists to work as cross-functional teams in order to tackle novel tasks toward the understanding of nature.
One challenge for the binomial AI–PS research area is to tackle the representation of tacit knowledge and include it in the ML algorithms. The relevance of tacit knowledge in the building up of protein science knowledge has come a long way since Polanyi first noted it, extending to different fields in the search for an improvement of their practical skills. In AI, the predominant way of knowledge acquisition and performance is a formal one in which the machine learns and expresses explicitly through guidelines and that works in a focalized mean; the new task alludes to a tacit dimension (Polanyi, 1962), which remains in the edge of attention and incorporates aspects that are taught and learned mostly through practice and in a comprehensive manner (it is context-specific, spreads in the laboratory environment, and comes into play in decision-making.
Some Conclusions
To sum up, the systematic review and the biochemical meta-analysis offered in this article focused on the enormous innovation that has been made in the binomial AI–PS research, both in its applications and its road maps to solve protein structures and function prediction, protein and drug design, among other tasks. The contribution of this study is 3-fold: firstly, the setup of a cross-functional group in which computer scientists, professionals in biomedicine, and a philosopher constructed a common language and together identified relevant literature in the inter-field of AI–PS and constructed a bridge between the two fields, which can serve as a framework for further research in either area.
Secondly, we stressed the importance of a finer-grain understanding of training and validation methods of ML models and their outcomes, combining databases from several areas of knowledge (life-science experiments, in silico simulations, ML, direct evolution approach, etc.) that allowed us to classify, stratify, and contribute to the evolving protein science field. Thirdly, we showed that the binomial AI–PS, a progressive research program, as Lakatos would say and has still several challenges to tackle, such as the development of a comprehensive machine learning benchmarking enterprise, the experimental confirmation of the structure of the 3D modeling in laboratories, the classification, etc., controls the vulnerability of the neural networks, the development of a tool-kit to design novel biocatalysts not found in nature using reverse engineering, human-made metabolic routes, the design of new antibody molecular factory, novel proteostasis systems, the understanding of protein folding and protein-aggregation mechanisms, etc. Finally, we suggested that there may be a paradigm shift in the AI–PS research as a result of the recent great outcome of Alphafold, encouraging its use to the new generation of scientists.
In any case, what is clear is that a cross-functional group of scientists from several knowledge domains is required to work in coordination for sharing ideas, methodologies, and challenges toward the development of road maps and computational tools, paradigms, tacit, and explicit knowledge to fully explore and close the gap of the binomial AI–PS, a promising research area.
Data Availability Statement
The raw data supporting the conclusions of this article will be made available by the authors without undue reservation.
Author Contributions
Conceived and designed the experiments: MA-B and NA-B. Performed the systematic review: JV-A, MVA, FO-F, RZ-S, NA-B, and MA-B. Analyzed the data: JV-A, LO-T, MVA, FP-E, AA, FO-F, RZ-S, NA-B, NK-V, SVA, and MA-B. Contributed to reagents/materials/analysis tools: NK-V, NA-B, CR-M, and MA-B. Wrote the article: JV-A, LO-T, MVA, AA, FP-E, NA-B, and MA-B. Contributed to helpful discussions: JV-A, LO-T, MVA, FP-E, AA, FO-F, RZ-S, NA-B, NK-V, CR-M, SVA, and MA-B.
Conflict of Interest
The authors declare that the research was conducted in the absence of any commercial or financial relationships that could be construed as a potential conflict of interest.
Publisher’s Note
All claims expressed in this article are solely those of the authors and do not necessarily represent those of their affiliated organizations, or those of the publisher, the editors, and the reviewers. Any product that may be evaluated in this article, or claim that may be made by its manufacturer, is not guaranteed or endorsed by the publisher.
Acknowledgments
The authors would like to acknowledge the experimental support and fruitful discussions provided by Dr. Elsa de la Chesnaye. We also wish to thank Dr. Laura Bonifaz for her support. The contributions made by the assigned pre-graduate research fellows at the Universidad lberoamericana and UNAM are greatly appreciated. We are also thankful for the contributions of Perla Sueiras, Daniela Monroy, Maria Fernanda Frlas, Pablo Cardenas and Mattea Cussel for translation and proofread the manuscript, and Rogelio Ezequiel and Alonso Loyo for the artwork.
References
Adhikari, B., Hou, J., and Cheng, J. (2018). DNCON2: Improved Protein Contact Prediction Using Two-Level Deep Convolutional Neural Networks. BioInformatics 34, 1466–1472. doi:10.1093/bioinformatics/btx781
Al-Gharabli, S. I., Agtash, S. A., Rawashdeh, N. A., and Barqawi, K. R. (2015). Artificial Neural Networks for Dihedral Angles Prediction in Enzyme Loops: A Novel Approach. Ijbra 11, 153–161. doi:10.1504/IJBRA.2015.068090
Alakuş, T. B., and Türkoğlu, İ. (2021). A Novel Fibonacci Hash Method for Protein Family Identification by Using Recurrent Neural Networks. Turk. J. Electr. Eng. Comput. Sci. 29, 370–386. Available at: http://10.0.15.66/elk-2003-116. doi:10.0.15.66/elk-2003-116
Almagro Armenteros, J. J., Sønderby, C. K., Sønderby, S. K., Nielsen, H., and Winther, O. (2017). DeepLoc: Prediction of Protein Subcellular Localization Using Deep Learning. Bioinformatics 33, 3387–3395. doi:10.1093/bioinformatics/btx431
AlQuraishi, M. (2021). Machine Learning in Protein Structure Prediction. Curr. Opin. Chem. Biol. 65, 1–8. doi:10.1016/j.cbpa.2021.04.005
Armstrong, K. A., and Tidor, B. (2008). Computationally Mapping Sequence Space to Understand Evolutionary Protein Engineering. Biotechnol. Prog. 24, 62–73. doi:10.1021/bp070134h
Ashkenazy, H., Unger, R., and Kliger, Y. (2011). Hidden Conformations in Protein Structures. Bioinformatics 27, 1941–1947. doi:10.1093/bioinformatics/btr292
Baetu, T. (2015). Carl F, Craver and Lindley Darden: In Search of Mechanisms: Discoveries across the Life Sciences. Hpls 36, 459–461. doi:10.1007/s40656-014-0038-6
Bernardes, J., and Pedreira, C. (2013). A Review of Protein Function Prediction under Machine Learning Perspective. Biot 7, 122–141. doi:10.2174/18722083113079990006
Bindslev-Jensen, C., Sten, E., Earl, L. K., Crevel, R. W. R., Bindslev-Jensen, U., Hansen, T. K., et al. (2003). Assessment of the Potential Allergenicity of Ice Structuring Protein Type III HPLC 12 Using the FAO/WHO 2001 Decision Tree for Novel Foods. Food Chem. Toxicol. 41, 81–87. doi:10.1016/S0278-6915(02)00212-0
Bond, P. S., Wilson, K. S., and Cowtan, K. D. (2020). Predicting Protein Model Correctness in Coot Using Machine Learning. Acta Cryst. Sect. D. Struct. Biol. 76, 713–723. doi:10.1107/S2059798320009080
Bostan, B., Greiner, R., Szafron, D., and Lu, P. (2009). Predicting Homologous Signaling Pathways Using Machine Learning. Bioinformatics 25, 2913–2920. doi:10.1093/bioinformatics/btp532
Briesemeister, S., Rahnenführer, J., and Kohlbacher, O. (2010). Going from where to Why-Interpretable Prediction of Protein Subcellular Localization. Bioinformatics 26, 1232–1238. doi:10.1093/bioinformatics/btq115
Cao, R., Freitas, C., Chan, L., Sun, M., Jiang, H., and Chen, Z. (2017). ProLanGO: Protein Function Prediction Using Neural Machine Translation Based on a Recurrent Neural Network. Molecules 22, 1732. doi:10.3390/molecules22101732
Capriotti, E., Fariselli, P., and Casadio, R. (2005). I-Mutant2.0: Predicting Stability Changes upon Mutation from the Protein Sequence or Structure. Nucleic Acids Res. 33, W306–W310. doi:10.1093/nar/gki375
Chen, J., Yang, R., Zhang, C., Zhang, L., and Zhang, Q. (2019). DeepGly: A Deep Learning Framework with Recurrent and Convolutional Neural Networks to Identify Protein Glycation Sites from Imbalanced Data. IEEE ACCESS 7, 142368–142378. doi:10.1109/ACCESS.2019.2944411
Cheng, J., Tegge, A. N., and Baldi, P. (2008). Machine Learning Methods for Protein Structure Prediction. IEEE Rev. Biomed. Eng. 1, 41–49. doi:10.1109/RBME.2008.2008239
Cui, Y., Dong, Q., Hong, D., and Wang, X. (2019). Predicting Protein-Ligand Binding Residues with Deep Convolutional Neural Networks. BMC Bioinforma. 20, 93. doi:10.1186/s12859-019-2672-1
Cuperus, J. T., Groves, B., Kuchina, A., Rosenberg, A. B., Jojic, N., Fields, S., et al. (2017). Deep Learning of the Regulatory Grammar of Yeast 5′ Untranslated Regions from 500,000 Random Sequences. Genome Res. 27, 2015–2024. doi:10.1101/gr.224964.117
Dai, W., Chang, Q., Peng, W., Zhong, J., and Li, Y. (2020). Network Embedding the Protein-Protein Interaction Network for Human Essential Genes Identification. Genes. 11, 153. doi:10.3390/genes11020153
Daniels, N. M., Hosur, R., Berger, B., and Cowen, L. J. (2012). SMURFLite: Combining Simplified Markov Random Fields with Simulated Evolution Improves Remote Homology Detection for Beta-Structural Proteins into the Twilight Zone. Bioinformatics 28, 1216–1222. doi:10.1093/bioinformatics/bts110
Dara, S., Dhamercherla, S., Jadav, S. S., Babu, C. H., and Ahsan, M. J. (2021). Machine Learning in Drug Discovery: A Review. Artif. Intell. Rev. 55 (3), 1947–1999. doi:10.1007/s10462-021-10058-4
Degiacomi, M. T. (2019). Coupling Molecular Dynamics and Deep Learning to Mine Protein Conformational Space. Structure 27, 1034–1040. doi:10.1016/j.str.2019.03.018
Du, Z., He, Y., Li, J., and Uversky, V. N. (2020). DeepAdd: Protein Function Prediction from K-Mer Embedding and Additional Features. Comput. Biol. Chem. 89, 107379. N.PAG--N.PAG. Available at: http://10.0.3.248/j.compbiolchem.2020.107379. doi:10.1016/j.compbiolchem.2020.107379
Durrant, J. D., and McCammon, J. A. (2011). NNScore 2.0: A Neural-Network Receptor-Ligand Scoring Function. J. Chem. Inf. Model.. 51, 2897–2903. doi:10.1021/ci2003889
Ebina, T., Toh, H., and Kuroda, Y. (2011). DROP: An SVM Domain Linker Predictor Trained with Optimal Features Selected by Random Forest. Bioinformatics 27, 487–494. doi:10.1093/bioinformatics/btq700
Ebrahimpour, A., Rahman, R. N. Z. R. A., Ean Ch'ng, D. H., Basri, M., and Salleh, A. B. (2008). A Modeling Study by Response Surface Methodology and Artificial Neural Network on Culture Parameters Optimization for Thermostable Lipase Production from a Newly Isolated Thermophilic Geobacillus Sp. Strain ARM. BMC Biotechnol. 8, 96. doi:10.1186/1472-6750-8-96
Eisenbeis, S., Proffitt, W., Coles, M., Truffault, V., Shanmugaratnam, S., Meiler, J., et al. (2012). Potential of Fragment Recombination for Rational Design of Proteins. J. Am. Chem. Soc. 134, 4019–4022. doi:10.1021/ja211657k
Fang, C., Moriwaki, Y., Tian, A., Li, C., and Shimizu, K. (2019). Identifying Short Disorder-To-Order Binding Regions in Disordered Proteins with a Deep Convolutional Neural Network Method. J. Bioinform. Comput. Biol. 17, 1950004. doi:10.1142/S0219720019500045
Fang, C., Shang, Y., and Xu, D. (2020). A Deep Dense Inception Network for Protein Beta‐turn Prediction. Proteins 88, 143–151. doi:10.1002/prot.25780
Fang, C., Shang, Y., and Xu, D. (2018). MUFOLD-SS: New Deep Inception-Inside-Inception Networks for Protein Secondary Structure Prediction. Proteins 86, 592–598. doi:10.1002/prot.25487
Feger, G., Angelov, B., and Angelova, A. (2020). Prediction of Amphiphilic Cell-Penetrating Peptide Building Blocks from Protein-Derived Amino Acid Sequences for Engineering of Drug Delivery Nanoassemblies. J. Phys. Chem. B 124, 4069–4078. doi:10.1021/acs.jpcb.0c01618
Feinberg, E. N., Sur, D., Wu, Z., Husic, B. E., Mai, H., Li, Y., et al. (2018). PotentialNet for Molecular Property Prediction. ACS Cent. Sci. 4, 1520–1530. doi:10.1021/acscentsci.8b00507
Folkman, L., Stantic, B., and Sattar, A. (2014). Feature-based Multiple Models Improve Classification of Mutation-Induced Stability Changes. BMC Genomics 15, 96. doi:10.1186/1471-2164-15-S4-S6
Frasca, M., Grossi, G., Gliozzo, J., Mesiti, M., Notaro, M., Perlasca, P., et al. (2018). A GPU-Based Algorithm for Fast Node Label Learning in Large and Unbalanced Biomolecular Networks. BMC Bioinforma. 19, 353. doi:10.1186/s12859-018-2301-4
Fu, H., Yang, Y., Wang, X., Wang, H., and Xu, Y. (2019). DeepUbi: A Deep Learning Framework for Prediction of Ubiquitination Sites in Proteins. BMC Bioinforma. 20, 86. doi:10.1186/s12859-019-2677-9
Gainza, P., Nisonoff, H. M., and Donald, B. R. (2016). Algorithms for Protein Design. Curr. Opin. Struct. Biol. 39, 16–26. doi:10.1016/j.sbi.2016.03.006
Guo, Y., Li, W., Wang, B., Liu, H., and Zhou, D. (2019). DeepACLSTM: Deep Asymmetric Convolutional Long Short-Term Memory Neural Models for Protein Secondary Structure Prediction. BMC Bioinforma. 20, 341. doi:10.1186/s12859-019-2940-0
Gutteridge, A., Bartlett, G. J., and Thornton, J. M. (2003). Using a Neural Network and Spatial Clustering to Predict the Location of Active Sites in Enzymes. J. Mol. Biol. 330, 719–734. doi:10.1016/S0022-2836(03)00515-1
Haberal, İ., and Oğul, H. (2019). Prediction of Protein Metal Binding Sites Using Deep Neural Networks. Mol. Inf. 38, 1800169. doi:10.1002/minf.201800169
Han, X., Zhang, L., Zhou, K., and Wang, X. (2019). ProGAN: Protein Solubility Generative Adversarial Nets for Data Augmentation in DNN Framework. Comput. Chem. Eng. 131, 106533. N.PAG--N.PAG. Available at: http://10.0.3.248/j.compchemeng.2019.106533. doi:10.1016/j.compchemeng.2019.106533
Hanson, J., Paliwal, K., Litfin, T., Yang, Y., and Zhou, Y. (2018). Accurate Prediction of Protein Contact Maps by Coupling Residual Two-Dimensional Bidirectional Long Short-Term Memory with Convolutional Neural Networks. Bioinformatics 34, 4039–4045. Available at: http://10.0.4.69/bioinformatics/bty481. doi:10.1093/bioinformatics/bty481
Hanson, J., Paliwal, K., Litfin, T., Yang, Y., and Zhou, Y. (2019). Improving Prediction of Protein Secondary Structure, Backbone Angles, Solvent Accessibility and Contact Numbers by Using Predicted Contact Maps and an Ensemble of Recurrent and Residual Convolutional Neural Networks. Bioinformatics 35, 2403–2410. doi:10.1093/bioinformatics/bty1006
He, H., Liu, B., Luo, H., Zhang, T., and Jiang, J. (2020). Big Data and Artificial Intelligence Discover Novel Drugs Targeting Proteins without 3D Structure and Overcome the Undruggable Targets. STROKE Vasc. Neurol. 5, 381–387. doi:10.1136/svn-2019-000323
Heinzinger, M., Elnaggar, A., Wang, Y., Dallago, C., Nechaev, D., Matthes, F., et al. (2019). Modeling Aspects of the Language of Life through Transfer-Learning Protein Sequences. BMC Bioinforma. 20, 723. doi:10.1186/s12859-019-3220-8
Hey, T., Butler, K., Jackson, S., and Thiyagalingam, J. (2019). Machine Learning and Big Scientific Data. Philos. Trans. A Math. Phys. Eng. Sci. 378 (2166), 20190054. arXiv. Available at: file:///Users/Myriam/Documents/2020/manuscritos. doi:10.1098/rsta.2019.0054
Hie, B. L., and Yang, K. K. (2022). Adaptive Machine Learning for Protein Engineering. Curr. Opin. Struct. Biol. 72, 145–152. doi:10.1016/j.sbi.2021.11.002
Hochuli, J., Helbling, A., Skaist, T., Ragoza, M., and Koes, D. R. (2018). Visualizing Convolutional Neural Network Protein-Ligand Scoring. J. Mol. Graph. Model. 84, 96–108. doi:10.1016/j.jmgm.2018.06.005
Hong, E.-J., Lippow, S. M., Tidor, B., and Lozano-Pérez, T. (2009). Rotamer Optimization for Protein Design through MAP Estimation and Problem-Size Reduction. J. Comput. Chem. 30, 1923–1945. doi:10.1002/jcc.21188
Hu, B., Wang, H., Wang, L., and Yuan, W. (2018). Adverse Drug Reaction Predictions Using Stacking Deep Heterogeneous Information Network Embedding Approach. Molecules 23, 3193. doi:10.3390/molecules23123193
Hu, C., Li, X., and Liang, J. (2004). Developing Optimal Non-linear Scoring Function for Protein Design. Bioinformatics 20, 3080–3098. doi:10.1093/bioinformatics/bth369
Huang, L., Liao, L., and Wu, C. H. (2018). Completing Sparse and Disconnected Protein-Protein Network by Deep Learning. BMC Bioinforma. 19, 103. doi:10.1186/s12859-018-2112-7
Huang, W.-L., Tung, C.-W., Ho, S.-W., Hwang, S.-F., and Ho, S.-Y. (2008). ProLoc-GO: Utilizing Informative Gene Ontology Terms for Sequence-Based Prediction of Protein Subcellular Localization. BMC Bioinforma. 9, 80. doi:10.1186/1471-2105-9-80
Hung, C.-M., Huang, Y.-M., and Chang, M.-S. (2006). Alignment Using Genetic Programming with Causal Trees for Identification of Protein Functions. Nonlinear Analysis Theory, Methods & Appl. 65, 1070–1093. doi:10.1016/j.na.2005.09.048
Jiménez, J., Doerr, S., Martínez-Rosell, G., Rose, A. S., and De Fabritiis, G. (2017). DeepSite: Protein-Binding Site Predictor Using 3D-Convolutional Neural Networks. Bioinformatics 33, 3036–3042. doi:10.1093/bioinformatics/btx350
Kaleel, M., Torrisi, M., Mooney, C., and Pollastri, G. (2019). PaleAle 5.0: Prediction of Protein Relative Solvent Accessibility by Deep Learning. Amino Acids 51, 1289–1296. Available at: http://10.0.3.239/s00726-019-02767-6. doi:10.1007/s00726-019-02767-6
Karimi, M., Wu, D., Wang, Z., and Shen, Y. (2019). DeepAffinity: Interpretable Deep Learning of Compound-Protein Affinity through Unified Recurrent and Convolutional Neural Networks. Bioinformatics 35, 3329–3338. Available at: http://10.0.4.69/bioinformatics/btz111. doi:10.1093/bioinformatics/btz111
Katzman, S., Barrett, C., Thiltgen, G., Karchin, R., and Karplus, K. (2008). Predict-2nd: A Tool for Generalized Protein Local Structure Prediction. Bioinformatics 24, 2453–2459. doi:10.1093/bioinformatics/btn438
Kauffman, S. A. (1992). “Origins of Order in Evolution: Self-Organization and Selection,” in Understanding Origins (Netherlands: Springer), 153–181. doi:10.1007/978-94-015-8054-0_8
Khan, Z. U., Hayat, M., and Khan, M. A. (2015). Discrimination of Acidic and Alkaline Enzyme Using Chou's Pseudo Amino Acid Composition in Conjunction with Probabilistic Neural Network Model. J. Theor. Biol. 365, 197–203. doi:10.1016/j.jtbi.2014.10.014
Khurana, S., Rawi, R., Kunji, K., Chuang, G.-Y., Bensmail, H., and Mall, R. (2018). DeepSol: A Deep Learning Framework for Sequence-Based Protein Solubility Prediction. Bioinformatics 34, 2605–2613. doi:10.1093/bioinformatics/bty166
Klausen, M. S., Jespersen, M. C., Nielsen, H., Jensen, K. K., Jurtz, V. I., Sønderby, C. K., et al. (2019). NetSurfP‐2.0: Improved Prediction of Protein Structural Features by Integrated Deep Learning. Proteins 87, 520–527. doi:10.1002/prot.25674
Kwon, Y., Shin, W.-H., Ko, J., and Lee, J. (2020). AK-score: Accurate Protein-Ligand Binding Affinity Prediction Using an Ensemble of 3D-Convolutional Neural Networks. Ijms 21, 8424. doi:10.3390/ijms21228424
Ladunga, I., Czakó, F., Csabai, I., and Geszti, T. (1991). Improving Signal Peptide Prediction Accuracy by Simulated Neural Network. Bioinformatics 7, 485–487. doi:10.1093/bioinformatics/7.4.485
Latek, D., and Kolinski, A. (2011). CABS-NMR-De Novo Tool for Rapid Global Fold Determination from Chemical Shifts, Residual Dipolar Couplings and Sparse Methyl-Methyl Noes. J. Comput. Chem. 32, 536–544. doi:10.1002/jcc.21640
Le, N.-Q. -K., Ho, Q.-T., and Ou, Y.-Y. (2018). Classifying the Molecular Functions of Rab GTPases in Membrane Trafficking Using Deep Convolutional Neural Networks. Anal. Biochem. 555, 33–41. doi:10.1016/j.ab.2018.06.011
Li, C.-C., and Liu, B. (2020). MotifCNN-fold: Protein Fold Recognition Based on Fold-specific Features Extracted by Motif-Based Convolutional Neural Networks. Brief. Bioinform. 21, 2133–2141. doi:10.1093/bib/bbz133
Li, H., Gong, X.-J., Yu, H., and Zhou, C. (2018). Deep Neural Network Based Predictions of Protein Interactions Using Primary Sequences. Molecules 23, 1923. doi:10.3390/molecules23081923
Li, H., Sze, K. H., Lu, G., and Ballester, P. J. (2021). Machine‐learning Scoring Functions for Structure‐based Virtual Screening. WIREs Comput. Mol. Sci. 11. doi:10.1002/wcms.1478
Li, Y., and Cirino, P. C. (2014). Recent Advances in Engineering Proteins for Biocatalysis. Biotechnol. Bioeng. 111, 1273–1287. doi:10.1002/bit.25240
Li, Z., Yang, Y., Faraggi, E., Zhan, J., and Zhou, Y. (2014). Direct Prediction of Profiles of Sequences Compatible with a Protein Structure by Neural Networks with Fragment-Based Local and Energy-Based Nonlocal Profiles. Proteins 82, 2565–2573. doi:10.1002/prot.24620
Liang, M., and Nie, J. (2020). Prediction of Enzyme Function Based on a Structure Relation Network. IEEE ACCESS 8, 132360–132366. doi:10.1109/ACCESS.2020.3010028
Liao, J., Warmuth, M. K., Govindarajan, S., Ness, J. E., Wang, R. P., Gustafsson, C., et al. (2007). Engineering Proteinase K Using Machine Learning and Synthetic Genes. BMC Biotechnol. 7, 16. doi:10.1186/1472-6750-7-16
Lin, G. N., Wang, Z., Xu, D., and Cheng, J. (2010). SeqRate: Sequence-Based Protein Folding Type Classification and Rates Prediction. BMC Bioinforma. 11, S1. doi:10.1186/1471-2105-11-S3-S1
Lin, J., Chen, H., Li, S., Liu, Y., Li, X., and Yu, B. (2019). Accurate Prediction of Potential Druggable Proteins Based on Genetic Algorithm and Bagging-SVM Ensemble Classifier. Artif. Intell. Med. 98, 35–47. Available at: http://10.0.3.248/j.artmed.2019.07.005. doi:10.1016/j.artmed.2019.07.005
Long, H., Liao, B., Xu, X., and Yang, J. (2018). A Hybrid Deep Learning Model for Predicting Protein Hydroxylation Sites. Ijms 19, 2817. doi:10.3390/ijms19092817
Long, S., and Tian, P. (2019). Protein Secondary Structure Prediction with Context Convolutional Neural Network. RSC Adv. 9, 38391–38396. doi:10.1039/c9ra05218f
Luo, F., Wang, M., Liu, Y., Zhao, X.-M., and Li, A. (2019). DeepPhos: Prediction of Protein Phosphorylation Sites with Deep Learning. Bioinformatics 35, 2766–2773. doi:10.1093/bioinformatics/bty1051
Luo, L., Yang, Z., Wang, L., Zhang, Y., Lin, H., and Wang, J. (2019). KeSACNN: a Protein-Protein Interaction Article Classification Approach Based on Deep Neural Network. Ijdmb 22, 131–148. doi:10.1504/ijdmb.2019.099724
Luo, X., Tu, X., Ding, Y., Gao, G., and Deng, M. (2020). Expectation Pooling: an Effective and Interpretable Pooling Method for Predicting DNA-Protein Binding. Bioinformatics 36, 1405–1412. doi:10.1093/bioinformatics/btz768
Mahmoud, A. H., Masters, M. R., Yang, Y., and Lill, M. A. (2020). Elucidating the Multiple Roles of Hydration for Accurate Protein-Ligand Binding Prediction via Deep Learning. Commun. Chem. 3, 19. doi:10.1038/s42004-020-0261-x
Maia, E. H. B., Assis, L. C., de Oliveira, T. A., da Silva, A. M., and Taranto, A. G. (2020). Structure-Based Virtual Screening: From Classical to Artificial Intelligence. Front. Chem. 8. doi:10.3389/fchem.2020.00343
Makrodimitris, S., Van Ham, R. C. H. J., and Reinders, M. J. T. (2019). Improving Protein Function Prediction Using Protein Sequence and GO-Term Similarities. Bioinformatics 35, 1116–1124. doi:10.1093/bioinformatics/bty751
Mataeimoghadam, F., Newton, M. A. H., Dehzangi, A., Karim, A., Jayaram, B., Ranganathan, S., et al. (2020). Enhancing Protein Backbone Angle Prediction by Using Simpler Models of Deep Neural Networks. Sci. Rep. 10, 1–12. doi:10.1038/s41598-020-76317-6
Mirabello, C., and Wallner, B. (2019). rawMSA: End-To-End Deep Learning Using Raw Multiple Sequence Alignments. PLoS One 14, e0220182. doi:10.1371/journal.pone.0220182
Müller, A. T., Hiss, J. A., and Schneider, G. (2018). Recurrent Neural Network Model for Constructive Peptide Design. J. Chem. Inf. Model.. 58, 472–479. doi:10.1021/acs.jcim.7b00414
Murphy, G. S., Sathyamoorthy, B., Der, B. S., Machius, M. C., Pulavarti, S. V., Szyperski, T., et al. (2015). Computational De Novo Design of a Four-Helix Bundle Protein-Dnd_4hb. Protein Sci. 24, 434–445. doi:10.1002/pro.2577
O'Connell, J., Li, Z., Hanson, J., Heffernan, R., Lyons, J., Paliwal, K., et al. (2018). SPIN2: Predicting Sequence Profiles from Protein Structures Using Deep Neural Networks. Proteins 86, 629–633. doi:10.1002/prot.25489
Özen, A., Gönen, M., Alpaydın, E., and Haliloğlu, T. (2009). Machine Learning Integration for Predicting the Effect of Single Amino Acid Substitutions on Protein Stability. BMC Struct. Biol. 9. doi:10.1186/1472-6807-9-66
Pagès, G., Charmettant, B., Grudinin, S., and Valencia, A. (2019). Protein Model Quality Assessment Using 3D Oriented Convolutional Neural Networks. Bioinformatics 35, 3313–3319. doi:10.1093/bioinformatics/btz122
Paladino, A., Marchetti, F., Rinaldi, S., and Colombo, G. (2017). Protein Design: from Computer Models to Artificial Intelligence. WIREs Comput. Mol. Sci. 7, e1318. doi:10.1002/wcms.1318
Picart-Armada, S., Barrett, S. J., Willé, D. R., Perera-Lluna, A., Gutteridge, A., and Dessailly, B. H. (2019). Benchmarking Network Propagation Methods for Disease Gene Identification. PLoS Comput. Biol. 15, e1007276–24. Available at: http://10.0.5.91/journal.pcbi.1007276. doi:10.1371/journal.pcbi.1007276
Polanyi, M. (1962). Personal Knowledge. Towards a Post-Critical Philosophy. 2nd ed.. London: Routledge & Kegan Paul.
Popova, M., Isayev, O., and Tropsha, A. (2018). Deep Reinforcement Learning for De Novo Drug Design. Sci. Adv. 4, eaap7885. doi:10.1126/sciadv.aap7885
Qi, Y., Oja, M., Weston, J., and Noble, W. S. (2012). A Unified Multitask Architecture for Predicting Local Protein Properties. PLoS One 7, e32235. doi:10.1371/journal.pone.0032235
Qin, Z., Wu, L., Sun, H., Huo, S., Ma, T., Lim, E., et al. (2020). Artificial Intelligence Method to Design and Fold Alpha-Helical Structural Proteins from the Primary Amino Acid Sequence. Extreme Mech. Lett. 36, 100652. doi:10.1016/j.eml.2020.100652
Ragoza, M., Hochuli, J., Idrobo, E., Sunseri, J., and Koes, D. R. (2017). Protein-Ligand Scoring with Convolutional Neural Networks. J. Chem. Inf. Model.. 57, 942–957. doi:10.1021/acs.jcim.6b00740
Raveh, B., Rahat, O., Basri, R., and Schreiber, G. (2007). Rediscovering Secondary Structures as Network Motifs-Aan Unsupervised Learning Approach. Bioinformatics 23, e163–e169. doi:10.1093/bioinformatics/btl290
Rives, A., Meier, J., Sercu, T., Goyal, S., Lin, Z., Liu, J., et al. (2021). Biological Structure and Function Emerge from Scaling Unsupervised Learning to 250 Million Protein Sequences. Proc. Natl. Acad. Sci. U. S. A. 118, e2016239118. doi:10.1073/pnas.2016239118
Rossi, A., Micheletti, C., Seno, F., and Maritan, A. (2001). A Self-Consistent Knowledge-Based Approach to Protein Design. Biophysical J. 80, 480–490. doi:10.1016/S0006-3495(01)76030-4
Russ, W. P., and Ranganathan, R. (2002). Knowledge-based Potential Functions in Protein Design. Curr. Opin. Struct. Biol. 12, 447–452. doi:10.1016/S0959-440X(02)00346-9
Savojardo, C., Martelli, P. L., Tartari, G., and Casadio, R. (2020b). Large-scale Prediction and Analysis of Protein Sub-mitochondrial Localization with DeepMito. BMC Bioinforma. 21, 266. N.PAG--N.PAG. Available at: http://10.0.4.162/s12859-020-03617-z. doi:10.1186/s12859-020-03617-z
Savojardo, C., Bruciaferri, N., Tartari, G., Martelli, P. L., and Casadio, R. (2020a). DeepMito: Accurate Prediction of Protein Sub-mitochondrial Localization Using Convolutional Neural Networks. Bioinformatics 36, 56–64. Available at: http://10.0.4.69/bioinformatics/btz512. doi:10.1093/bioinformatics/btz512
Senior, A. W., Evans, R., Jumper, J., Kirkpatrick, J., Sifre, L., Green, T., et al. (2020). Improved Protein Structure Prediction Using Potentials from Deep Learning. Nature 577, 706–710. doi:10.1038/s41586-019-1923-7
Shah, A. R., Oehmen, C. S., and Webb-Robertson, B.-J. (2008). SVM-HUSTLE--an Iterative Semi-supervised Machine Learning Approach for Pairwise Protein Remote Homology Detection. Bioinformatics 24, 783–790. doi:10.1093/bioinformatics/btn028
Shamim, M. T. A., Anwaruddin, M., and Nagarajaram, H. A. (2007). Support Vector Machine-Based Classification of Protein Folds Using the Structural Properties of Amino Acid Residues and Amino Acid Residue Pairs. Bioinformatics 23, 3320–3327. doi:10.1093/bioinformatics/btm527
Shroff, R., Cole, A. W., Diaz, D. J., Morrow, B. R., Donnell, I., Annapareddy, A., et al. (2020). Discovery of Novel Gain-Of-Function Mutations Guided by Structure-Based Deep Learning. ACS Synth. Biol. 9, 2927–2935. doi:10.1021/acssynbio.0c00345
Sidhu, A., and Yang, Z. R. (2006). Prediction of Signal Peptides Using Bio-Basis Function Neural Networks and Decision Trees. Appl. Bioinforma. 5, 13–19. doi:10.2165/00822942-200605010-00002
Simha, R., Briesemeister, S., Kohlbacher, O., and Shatkay, H. (2015). Protein (Multi-)location Prediction: Utilizing Interdependencies via a Generative Model. Bioinformatics 31, i365–i374. doi:10.1093/bioinformatics/btv264
Song, J., Liu, G., Jiang, J., Zhang, P., and Liang, Y. (2021). Prediction of Protein-ATP Binding Residues Based on Ensemble of Deep Convolutional Neural Networks and LightGBM Algorithm. Ijms 22, 939. doi:10.3390/ijms22020939
Sua, J. N., Lim, S. Y., Yulius, M. H., Su, X., Yapp, E. K. Y., Le, N. Q. K., et al. (2020). Incorporating Convolutional Neural Networks and Sequence Graph Transform for Identifying Multilabel Protein Lysine PTM Sites. Chemom. Intelligent Laboratory Syst. 206, 104171. doi:10.1016/j.chemolab.2020.104171
Sunseri, J., King, J. E., Francoeur, P. G., and Koes, D. R. (2019). Convolutional Neural Network Scoring and Minimization in the D3R 2017 Community Challenge. J. Comput. Aided. Mol. Des. 33, 19–34. doi:10.1007/s10822-018-0133-y
Sureyya Rifaioglu, A., Doğan, T., Jesus Martin, M., Cetin-Atalay, R., and Atalay, V. (2019). DEEPred: Automated Protein Function Prediction with Multi-Task Feed-Forward Deep Neural Networks. Sci. Rep. 9, 7344. doi:10.1038/s41598-019-43708-3
Szalkai, B., and Grolmusz, V. (2018a). Near Perfect Protein Multi-Label Classification with Deep Neural Networks. METHODS 132, 50–56. doi:10.1016/j.ymeth.2017.06.034
Szalkai, B., and Grolmusz, V. (2018b). SECLAF: A Webserver and Deep Neural Network Design Tool for Hierarchical Biological Sequence Classification. Bioinformatics 34, 2487–2489. doi:10.1093/bioinformatics/bty116
Taherzadeh, G., Dehzangi, A., Golchin, M., Zhou, Y., and Campbell, M. P. (2019). SPRINT-gly: Predicting N- and O-Linked Glycosylation Sites of Human and Mouse Proteins by Using Sequence and Predicted Structural Properties. Bioinformatics 35, 4140–4146. doi:10.1093/bioinformatics/btz215
Tian, J., Wu, N., Chu, X., and Fan, Y. (2010). Predicting Changes in Protein Thermostability Brought about by Single- or Multi-Site Mutations. BMC Bioinforma. 11, 370. doi:10.1186/1471-2105-11-370
Torng, W., and Altman, R. B. (2019). High Precision Protein Functional Site Detection Using 3D Convolutional Neural Networks. Bioinformatics 35, 1503–1512. doi:10.1093/bioinformatics/bty813
Traoré, S., Allouche, D., André, I., De Givry, S., Katsirelos, G., Schiex, T., et al. (2013). A New Framework for Computational Protein Design through Cost Function Network Optimization. Bioinformatics 29, 2129–2136. doi:10.1093/bioinformatics/btt374
Tsou, L. K., Yeh, S.-H., Ueng, S.-H., Chang, C.-P., Song, J.-S., Wu, M.-H., et al. (2020). Comparative Study between Deep Learning and QSAR Classifications for TNBC Inhibitors and Novel GPCR Agonist Discovery. Sci. Rep. 10, 16771. doi:10.1038/s41598-020-73681-1
Tsuchiya, Y., and Tomii, K. (2020). Neural Networks for Protein Structure and Function Prediction and Dynamic Analysis. Biophys. Rev. 12, 569–573. doi:10.1007/s12551-020-00685-6
Vang, Y. S., and Xie, X. (2017). HLA Class I Binding Prediction via Convolutional Neural Networks. Bioinformatics 33, 2658–2665. doi:10.1093/bioinformatics/btx264
Verma, N., Qu, X., Trozzi, F., Elsaied, M., Karki, N., Tao, Y., et al. (2021). SSnet: A Deep Learning Approach for Protein-Ligand Interaction Prediction. Ijms 22, 1392. doi:10.3390/ijms22031392
Volpato, V., Adelfio, A., and Pollastri, G. (2013). Accurate Prediction of Protein Enzymatic Class by N-To-1 Neural Networks. BMC Bioinforma. 14, S11. doi:10.1186/1471-2105-14-S1-S11
Wan, C., Cozzetto, D., Fa, R., and Jones, D. T. (2019). Using Deep Maxout Neural Networks to Improve the Accuracy of Function Prediction from Protein Interaction Networks. PLoS One 14, e0209958–21. Available at: http://10.0.5.91/journal.pone.0209958. doi:10.1371/journal.pone.0209958
Wang, D., Geng, L., Zhao, Y.-J., Yang, Y., Huang, Y., Zhang, Y., et al. (2020). Artificial Intelligence-Based Multi-Objective Optimization Protocol for Protein Structure Refinement. Bioinformatics 36, 437–448. doi:10.1093/bioinformatics/btz544
Wang, M., Cang, Z., and Wei, G.-W. (2020a). A Topology-Based Network Tree for the Prediction of Protein-Protein Binding Affinity Changes Following Mutation. Nat. Mach. Intell. 2, 116–123. doi:10.1038/s42256-020-0149-6
Wang, M., Cui, X., Li, S., Yang, X., Ma, A., Zhang, Y., et al. (2020b). DeepMal: Accurate Prediction of Protein Malonylation Sites by Deep Neural Networks. Chemom. Intelligent Laboratory Syst. 207, 104175. doi:10.1016/j.chemolab.2020.104175
Wang, X., Liu, Y., Lu, F., Li, H., Gao, P., and Wei, D. (2020). Dipeptide Frequency of Word Frequency and Graph Convolutional Networks for DTA Prediction. Front. Bioeng. Biotechnol. 8. doi:10.3389/fbioe.2020.00267
Wang, S., Sun, S., Li, Z., Zhang, R., and Xu, J. (2017). Accurate De Novo Prediction of Protein Contact Map by Ultra-deep Learning Model. PLoS Comput. Biol. 13, e1005324. doi:10.1371/journal.pcbi.1005324
Wardah, W., Dehzangi, A., Taherzadeh, G., Rashid, M. A., Khan, M. G. M., Tsunoda, T., et al. (2020). Predicting Protein-Peptide Binding Sites with a Deep Convolutional Neural Network. J. Theor. Biol. 496, 110278. doi:10.1016/j.jtbi.2020.110278
Wardah, W., Khan, M. G. M., Sharma, A., and Rashid, M. A. (2019). Protein Secondary Structure Prediction Using Neural Networks and Deep Learning: A Review. Comput. Biol. Chem. 81, 1–8. doi:10.1016/j.compbiolchem.2019.107093
Wong, K.-C., Chan, T.-M., Peng, C., Li, Y., and Zhang, Z. (2013). DNA Motif Elucidation Using Belief Propagation. Nucleic Acids Res. 41, e153. doi:10.1093/nar/gkt574
Wu, S., and Zhang, Y. (2008). A Comprehensive Assessment of Sequence-Based and Template-Based Methods for Protein Contact Prediction. Bioinformatics 24, 924–931. doi:10.1093/bioinformatics/btn069
Xu, J., Mcpartlon, M., and Li, J. (2021). Improved Protein Structure Prediction by Deep Learning Irrespective of Co-evolution Information. Nat. Mach. Intell. 3, 601–609. doi:10.1038/s42256-021-00348-5
Xue, L., Tang, B., Chen, W., and Luo, J. (2019). DeepT3: Deep Convolutional Neural Networks Accurately Identify Gram-Negative Bacterial Type III Secreted Effectors Using the N-Terminal Sequence. Bioinformatics 35, 2051–2057. doi:10.1093/bioinformatics/bty931
Yang, H., Wang, M., Yu, Z., Zhao, X.-M., and Li, A. (2020). GANcon: Protein Contact Map Prediction with Deep Generative Adversarial Network. IEEE ACCESS 8, 80899–80907. doi:10.1109/ACCESS.2020.2991605
Yang, J., Anishchenko, I., Park, H., Peng, Z., Ovchinnikov, S., and Baker, D. (2020). Improved Protein Structure Prediction Using Predicted Interresidue Orientations. Proc. Natl. Acad. Sci. U.S.A. 117, 1496–1503. doi:10.1073/pnas.1914677117
Yang, J., He, B.-J., Jang, R., Zhang, Y., and Shen, H.-B. (2015). Accurate Disulfide-Bonding Network Predictions Improveab Initiostructure Prediction of Cysteine-Rich Proteins. Bioinformatics 31, btv459–3781. doi:10.1093/bioinformatics/btv459
Yang, Y., Faraggi, E., Zhao, H., and Zhou, Y. (2011). Improving Protein Fold Recognition and Template-Based Modeling by Employing Probabilistic-Based Matching between Predicted One-Dimensional Structural Properties of Query and Corresponding Native Properties of Templates. Bioinformatics 27, 2076–2082. doi:10.1093/bioinformatics/btr350
Yeh, C.-T., Brunette, T., Baker, D., McIntosh-Smith, S., and Parmeggiani, F. (2018). Elfin: An Algorithm for the Computational Design of Custom Three-Dimensional Structures from Modular Repeat Protein Building Blocks. J. Struct. Biol. 201, 100–107. doi:10.1016/j.jsb.2017.09.001
Yu, C.-H., and Buehler, M. J. (2020). Sonification Based De Novo Protein Design Using Artificial Intelligence, Structure Prediction, and Analysis Using Molecular Modeling. Apl. Bioeng. 4, 016108. doi:10.1063/1.5133026
Yu, C.-H., Qin, Z., Martin-Martinez, F. J., and Buehler, M. J. (2019). A Self-Consistent Sonification Method to Translate Amino Acid Sequences into Musical Compositions and Application in Protein Design Using Artificial Intelligence. ACS Nano 13, 7471–7482. doi:10.1021/acsnano.9b02180
Zafeiris, D., Rutella, S., and Ball, G. R. (2018). An Artificial Neural Network Integrated Pipeline for Biomarker Discovery Using Alzheimer's Disease as a Case Study. Comput. Struct. Biotechnol. J. 16, 77–87. doi:10.1016/j.csbj.2018.02.001
Zhang, B., Li, J., and Lü, Q. (2018). Prediction of 8-state Protein Secondary Structures by a Novel Deep Learning Architecture. BMC Bioinforma. 19, 293. Available at: http://10.0.4.162/s12859-018-2280-5. doi:10.1186/s12859-018-2280-5
Zhang, D., and Kabuka, M. (2019). Multimodal Deep Representation Learning for Protein Interaction Identification and Protein Family Classification. BMC Bioinforma. 20, 531. doi:10.1186/s12859-019-3084-y
Zhang, L., Yu, G., Guo, M., and Wang, J. (2018). Predicting Protein-Protein Interactions Using High-Quality Non-interacting Pairs. BMC Bioinforma. 19, 525. doi:10.1186/s12859-018-2525-3
Zhang, Y., Qiao, S., Ji, S., Han, N., Liu, D., and Zhou, J. (2019). Identification of DNA-Protein Binding Sites by Bootstrap Multiple Convolutional Neural Networks on Sequence Information. Eng. Appl. Artif. Intell. 79, 58–66. doi:10.1016/j.engappai.2019.01.003
Zhao, B., and Xue, B. (2018). Decision-tree Based Meta-Strategy Improved Accuracy of Disorder Prediction and Identified Novel Disordered Residues inside Binding Motifs. Ijms 19, 3052. doi:10.3390/ijms19103052
Zhao, F., Peng, J., and Xu, J. (2010). Fragment-free Approach to Protein Folding Using Conditional Neural Fields. Bioinformatics 26, i310–i317. doi:10.1093/bioinformatics/btq193
Zhao, X., Li, J., Wang, R., He, F., Yue, L., and Yin, M. (2018). General and Species-specific Lysine Acetylation Site Prediction Using a Bi-modal Deep Architecture. IEEE ACCESS 6, 63560–63569. doi:10.1109/ACCESS.2018.2874882
Zhao, Z., and Gong, X. (2019). Protein-Protein Interaction Interface Residue Pair Prediction Based on Deep Learning Architecture. IEEE/ACM Trans. Comput. Biol. Bioinf. 16, 1753–1759. doi:10.1109/TCBB.2017.2706682
Zheng, W., Li, Y., Zhang, C., Pearce, R., Mortuza, S. M., and Zhang, Y. (2019). Deep‐learning Contact‐map Guided Protein Structure Prediction in CASP13. Proteins 87, 1149–1164. doi:10.1002/prot.25792
Zheng, W., Zhou, X., Wuyun, Q., Pearce, R., Li, Y., and Zhang, Y. (2020). FUpred: Detecting Protein Domains through Deep-Learning-Based Contact Map Prediction. Bioinformatics 36, 3749–3757. doi:10.1093/bioinformatics/btaa217
Zhu, X., and Lai, L. (2009). A Novel Method for Enzyme Design. J. Comput. Chem. 30, 256–267. doi:10.1002/jcc.21050
Zimmermann, O., and Hansmann, U. H. E. (2006). Support Vector Machines for Prediction of Dihedral Angle Regions. Bioinformatics 22, 3009–3015. doi:10.1093/bioinformatics/btl489
Glossary
1D-CNN one-dimensional convolutional neural network
2D-BRLSTM two-dimensional bidirectional recurrent long short-term memory
2D-CNN Two-dimensional convolutional neural network
3D-CNN Three-dimensional convolutional neural network
ACNN Asymmetric convolutional neural network
ADASYN Adaptive synthetic sampling
AGCT Alignment genetic causal tree
ANN Artificial neural network
BBFNN Biobasis function neural network
BBP Back back propagation
BLSTM Bidirectional long short-term memory
BN Bayesian network
BRNN Bidirectional recurrent neural network
BroMap Branch and bound map estimation
BRT Booster regression tree
CABS C-alpha–beta side
CFN Cost function network
CNF Conditional neural field
CNN Convolutional neural network
COSNet Cost-sensitive neural network
DCNN Deep convolutional neural network
DeepDIN Deep dense inception network
Deep3I Deep inception-inside-inception network
DFS Depth first search
DL Deep learning
DMNN Deep mahout neural network
DNN Deep neural network
DRNN Deep residual neural network
DROP Domain linker prediction using optimal feature
DT Decision tree
DTNN Deep tensor neural network
EASE-MM Evolutionary amino acid and structural encodings with multiple models
ELMO Embeddings from language models
ENN-RL Evolution neural network-based regularized Laplacian kernel
FFNN Feed forward neural network
FIBHASH Fibonacci numbers and hashing table
GA Genetic algorithms
GAN Generative adversarial network
GBT Gradient boost tree
GBDT Gradient boosted decision tree
GCN Graph convolutional network
GR Genetic recombination
HDL Hybrid deep learning
HMM Hidden Markov model
HNN Hopfield neural network
IBP Incremental back propagation
KeSCANN Knowledge-enriched self-attention convolutional neural network
K-merHMM K.mer Hidden Markov model
KNN k-nearest neighbor
Lasso Least absolute shrinkage and selection operator
LightGBM Light gradient boosting machine
LM Levenberg–Marquardt
LPBoostR Linear programming boosting regression
LPSVMR Linear programming support vector machine regression
LR Logistic regression
LSDR Label-space dimensionality reduction
LSTM Long short-term memory
MC Monte Carlo
ME Max entropy
ML Model
MLP Multilayer perceptron
MNB Multinomial naïve bayes
MNNN Multi-scale neighborhood-based neural network
MNPP Message passing neural network
MotifCNN Motif convolutional neural network
Motif DNN Motif deep neural network
MR Matching loss regression
MRF Markov random forest
Multimodal DNN Multimodal deep neural network
NB Naïve Bayes
NLP Natural language processing
ORMR One-norm regularization matching-loss regression
ParCOSNet Parallel COSNet
PLSR Partial least-squares regression
PNN Probabilistic neural network
PS Protein science
PSO Particle swarm optimization
PSP Predict signal pathway
QP quick prob
ReLeaSE Reinforcement learning for structural evolution
RF Random forest
RN Relational network
RNN Recurrent neural network
RNN 2 Residual neural network
RR Ridge regression
SDHINE Meta path-based heterogeneous information embedding approach
SFFS Sequential forward floating selection
SGD Stochastic gradient descent
SPARK-X Probabilistic-based matching
SPIN Sequence profiles by integrated neural network
SVM Support vector machine
SVMR Support vector machine regression
SVR Support vector regression
UDNN Ultradeep neural network
VSA Virtual screening algorithms
WMC Weighted multiple conformations
Keywords: artificial intelligence, proteins, protein design and engineering, machine learning, deep learning, protein prediction, protein classification, drug design
Citation: Villalobos-Alva J, Ochoa-Toledo L, Villalobos-Alva MJ, Aliseda A, Pérez-Escamirosa F, Altamirano-Bustamante NF, Ochoa-Fernández F, Zamora-Solís R, Villalobos-Alva S, Revilla-Monsalve C, Kemper-Valverde N and Altamirano-Bustamante MM (2022) Protein Science Meets Artificial Intelligence: A Systematic Review and a Biochemical Meta-Analysis of an Inter-Field. Front. Bioeng. Biotechnol. 10:788300. doi: 10.3389/fbioe.2022.788300
Received: 02 October 2021; Accepted: 25 May 2022;
Published: 07 July 2022.
Edited by:
Ratul Chowdhury, Harvard Medical School, United StatesReviewed by:
Neng-Zhong Xie, Guangxi Academy of Sciences, ChinaNabankur Dasgupta, Sandia National Laboratories, United States
Sudhanya Banerjee, AspenTech, United States
Copyright © 2022 Villalobos-Alva, Ochoa-Toledo, Villalobos-Alva, Aliseda, Pérez-Escamirosa, Altamirano-Bustamante, Ochoa-Fernández, Zamora-Solís, Villalobos-Alva, Revilla-Monsalve, Kemper-Valverde and Altamirano-Bustamante. This is an open-access article distributed under the terms of the Creative Commons Attribution License (CC BY). The use, distribution or reproduction in other forums is permitted, provided the original author(s) and the copyright owner(s) are credited and that the original publication in this journal is cited, in accordance with accepted academic practice. No use, distribution or reproduction is permitted which does not comply with these terms.
*Correspondence: Myriam M. Altamirano-Bustamante, bXlyaWFtYWJAdW5hbS5teA==
†These authors have contributed equally to this work