- 1Ningbo Innovation Center, School of Mechanical Engineering, Zhejiang University, Ningbo, China
- 2Faculty of Science and Engineering, University of Nottingham Ningbo, Ningbo, China
- 3Nottingham Ningbo China Beacons of Excellence Research and Innovation Institute, University of Nottingham Ningbo, Ningbo, China
- 4Nottingham University Business School China, University of Nottingham Ningbo China, Ningbo, Zhejiang, China
- 5Business School, NingboTech University, Ningbo, China
In recent years, a huge number of individuals all over the world, elderly people, in particular, have been suffering from Alzheimer’s disease (AD), which has had a significant negative impact on their quality of life. To intervene early in the progression of the disease, accurate, convenient, and low-cost detection technologies are gaining increased attention. As a result of their multiple merits in the detection and assessment of AD, biosensors are being frequently utilized in this field. Behavioral detection is a prospective way to diagnose AD at an early stage, which is a more objective and quantitative approach than conventional neuropsychological scales. Furthermore, it provides a safer and more comfortable environment than those invasive methods (such as blood and cerebrospinal fluid tests) and is more economical than neuroimaging tests. Behavior detection is gaining increasing attention in AD diagnosis. In this review, cutting-edge biosensor-based devices for AD diagnosis together with their measurement parameters and diagnostic effectiveness have been discussed in four application subtopics: body movement behavior detection, eye movement behavior detection, speech behavior detection, and multi-behavior detection. Finally, the characteristics of behavior detection sensors in various application scenarios are summarized and the prospects of their application in AD diagnostics are presented as well.
1 Introduction
By 2020, more than 50 million people suffer from dementia globally, and this number will keep increasing at a high rate, rising to 82 million in 2030 and 152 million in 2050. Among them, developing countries will account for the majority of the growth. By 2050, 71% of dementia patients would be residents of low- and middle-income nations, up from the current 60% (Prince et al., 2015). Alzheimer’s disease, the most prevalent cause and well-known type of dementia, accounts for 60–80% of incidences (Gauthier et al., 2021). Currently, there is no effective pharmacological medication that can halt the progression of the pathology, making early diagnosis and intervention extremely crucial (Mancioppi et al., 2019). Therefore, the early diagnosis of AD is becoming an important issue and is attracting increasing attention worldwide. However, the existing main techniques used to accurately diagnose AD include Cerebrospinal fluid (CSF) testing and neuroimaging methods, which are costly, time-consuming, and out of reach for the majority of people. In this context, biosensors are considered as potential substitutes for low-cost, quick, comfortable, and straightforward method for AD diagnosis (Brazaca et al., 2019).
Biosensors are analysis tools that transform biological responses into quantifiable signals (Amine et al., 2006; Brazaca et al., 2019), they have significant potential to revolutionize a wide range of industries, especially are extensively utilized in the field of medical testing, such as health monitoring, motion detection, activity identification, etc. (Liu et al., 2016; Sprint et al., 2016; Petra et al., 2018). Among them, the physiological biosensor is one of the most common categories that are utilized to detect biochemical indicators, e.g., pH, glucose, ions, sweat, skin interstitial fluid in the human biofluids, and physical indicators, such as pulse, heart rate, blood pressure, temperature, electrocardiogram (ECG), etc. (Brazaca et al., 2019; Guo et al., 2020; Kodintsev et al., 2020; Zhang et al., 2021a; Zhang et al., 2021b; Huang et al., 2021; Sheng et al., 2022). For instance, the graphene FET sensors has been developed by Bungon et al. to detect protein biomarker clusterin of Alzheimer’s disease (Bungon et al., 2020). Besides, due to the development of health monitoring technology, behavior detection biosensors, such as motion sensors and sound sensors are being widely used in this field to measure various behavioral biomarkers of humans (Bouchard et al., 2014; Chaccour et al., 2016; Vippalapalli and Ananthula, 2016; Stone and Skubic, 2017; Wang et al., 2017; Nweke et al., 2018; Wang et al., 2019 Lima et al., 2019; Fan et al., 2020; Yu and Wang, 2020), e.g., person’s movement and speech behavior, etc. (Yu and Wang, 2020). Moreover, ambient biosensors play an essential role in human activity detection and risk assessment by monitoring environmental conditions, including humidity, radiation, gas, pressure, temperature, etc. (Sunny et al., 2020; Karthikeyan et al., 2021).
Among these three basic categories of biosensors, behavioral detection sensors are gaining increasing attention because of numerous demands in society, such as safety, comfort, natural interaction, entertainment, assisted living, etc. (Yu and Wang, 2020). For instance, the aging problem urges the demand for home-based health care for the elderly (Merilahti et al., 2015). In order to promote the integration of smart homes with elderly care, Donghwa Shon et al. investigated the possibility of embedding healthcare services into smart homes in a non-invasive manner, and propose medical scenarios that can be applied to each smart home room (Choi et al., 2019). The monitoring of activities of daily living (ADL) using the household sensors and networks have been investigated (Nathan et al., 2018). Fleury et al. propose a support vector machine (SVM)-based ADL recognition mechanism. Lots of sensors such as microphones, contact sensors, infrared (IR) sensors, accelerometer and magnetometer are used to monitor the activities of older persons (Fleury et al., 2010). There are usually more than one member in a family, to identify each of the dwellers, Wang et al. presented a multiuser activity recognition system using wearable audio sensors, altimetry sensors and RFID tags, and high accuracy was obtained (Wang et al., 2011). To ensure they live in a safer environment, behavior monitoring, such as anomalous behavior detection, is urgently needed for elderly people, especially for those living alone (Alemdar and Ersoy, 2010; Rashidi and Mihailidis, 2013; Hoque et al., 2015; Chaccour et al., 2016; Stone and Skubic, 2017; Deep et al., 2020). In addition, biosensor-based behavior detection approaches are also commonly applied to identify behavioral disorders in certain types of diseases, such as Parkinson’s disease (PD), Stroke, Paralysis, etc. (Kang et al., 2010; Leclair-Visonneau et al., 2017; Alvarez et al., 2018; Loopez-Larraz et al., 2018; Migliaccio et al., 2020; Borzì et al., 2021; Diaconu et al., 2021). Notably, many recent studies have revealed that behavioral biomarkers might be vital indicators of Alzheimer’s disease (AD) in its initial phases (de et al., 2014; Nardone et al., 2022; Staal et al., 2021), symptoms like motor behavior alteration occur before profound memory deficits (Tippett and Sergio, 2006; Casper et al., 2015). Thus, given the fact that motor behavior deficits are generally regarded as a consequence of aging (Tippett and Sergio, 2006), biosensor-based behavior detection is vital for both the early diagnosis of AD, and for assisting to explore the underlying causes of the daily activity dysfunction of the elderly in a quantitative way. Therefore, this objective and effective approach to detecting the behavior biomarkers of potential AD patients by using biosensors is becoming a promising diagnostic method for AD (Verheij et al., 2012; Abe et al., 2013; Alam et al., 2017). As a result, a variety of wearable and ambient non-invasive biosensors for AD diagnosis are emerging (Wams et al., 2017; Mancioppi et al., 2019). Especially, biosensors oriented to motion behavior monitoring, physiological signal capturing, and environment information detecting have drawn particular attention among AD diagnosis and other behavior detection applications (Lara and Labrador, 2013; Bulling et al., 2014; Liu et al., 2021).
In this review, the most recent research into biosensors for behavior detection in the diagnosis of Alzheimer’s disease is discussed. An electronic database search was performed using the Web of Science, PubMed, Elsevier Science Direct, Scopus R, and Biomed Central databases to identify and select articles concerning the early diagnosis of Alzheimer’s disease using biosensors. The following key words are used: Alzheimer’s disease, diagnosis, biosensor, Behavior. Repetitive articles are eliminated, articles published by the same author are compared, and the articles most relevant to the theme of this article are selected. The applications of behavior detection biosensors are categorized into four groups: body motion behavior detection of Alzheimer’s disease, eye movement behavior detection of Alzheimer’s disease, speech and language behavior detection of Alzheimer’s disease, and multimodal behavior detection of Alzheimer’s disease. Body motion behavior sensors can detect body motions, for example, gait, walking speed, walking stride, balance, foot kicking, upper limb motion, and other activities of daily living. Eye movement behavior sensors are employed to measure gaze, fixation, saccade, eye blinks, and pupil response metrics, etc. Speech and language behavior sensors are mainly used to record and identify acoustic features. While multimodal behavior sensors can sense multiple combined behavior parameters, e.g., physiological indicators, eye movement, sound, body motions, etc. Together with various environmental information. Moreover, this review provides an outlook on future research directions of biosensor-based behavior detection technology and methodology for the diagnosis of Alzheimer’s disease. Notably, the challenges of data security, quality and privacy, and the regulatory requirements are still exist. Therefore, ethical review must be done prior to testing on patients, patient consent must be sought before data collection can take place, and data involving privacy cannot be published without patient consent.
2 Applications of biosensors toward behavior detection of alzheimer’s disease
2.1 Body motion behavior detection of alzheimer’s disease
Body motion behavior has been widely studied in detecting AD based on a range of sensors (Robben et al., 2016). Those utilized biosensors are mainly wearable sensors, including feet and waist-mounted inertial sensors, ankle-mounted accelerometric sensors, wrist-mounted accelerometers, leg-mounted force sensors, etc. In addition, to realize real-time monitoring and data analysis (Catarinucci et al., 2015; Jain et al., 2021), these biosensor devices tend to be integrated into the internet of things (IoT) devices (Helen et al., 2018; Yamini, 2020; Machado et al., 2021). Besides, with the increasingly improved communication technology, the use of a biosensor to detect body motion behavior in a VR environment offers a more promising way to conduct relevant experiments, which enables subjects to perform the tasks more interactively and safely in a relatively ideal immersive experimental environment (García-Betances et al., 2015; Montenegro and Argyriou, 2017; Mohammadi et al., 2018; Montenegro et al., 2020).
Wearable biosensors are widely employed in body motion detection of AD patients, and most of them are used to detect lower limbs’ motion parameters. Scholars collected body movement data from normal and Alzheimer’s patients, extracted the characteristics of the patient’s movement data and the characteristics of the normal person’s body movement data set to distinguish them with machine learning, and grouped them to determine where the test target belonged. In particular, gait analysis has received great attention in the study of cognitive impairment (Laske et al., 2015). For instance, Hsu et al. suggested a method to conduct objective quantitative measurement of foot movement in AD. In their study, wearable inertial sensor-based devices put on the feet and waist are employed to process gait and balance metrics with the aid of gait and balance analyzing algorithm. The outcomes of the trial demonstrate the effectiveness of this wearable sensor system for early-stage diagnosis of Alzheimer’s disease. It indicates that AD patients present greater variability in gait parameters and worse balance ability than healthy controls (Hsu et al., 2014). In addition, Kirste et al. proposed ankle-mounted three-axes accelerometric sensors to study motion behavior in AD patients’ daily life. All participants are required to conduct a 50 continuous hours’ daily activity recording within 3 days wearing the accelerometric sensor on their ankles. By analyzing the spatial trajectories, subjects’ activity level has been detected. The findings show that this method can discriminate between AD and HC (healthy control) with an accuracy of 91%, and motion behaviors data have a significant correlation with MMSE and CMAI (Cohen-Mansfield Agitation Inventory) scores (Kirste et al., 2014).
Varatharajan et al. presented a wearable internet of things (IoT) device to detect early Alzheimer’s disease. As shown in Figure 1I, the force sensor-based leg movement monitoring system is used to collect AD patients’ walking patterns in real-time, and with the help of the dynamic time warping (DTW) algorithm the participants’ various foot movement data, such as walking speed and gait are processed. Moreover, the middle-level cross identification (MidCross) function is applied to classify cognitively normal participants and AD patients by comparing their gait signals. In this study only foot movement is measured, it still proved the effectiveness of this method to diagnose Alzheimer’s disease (Varatharajan et al., 2017).
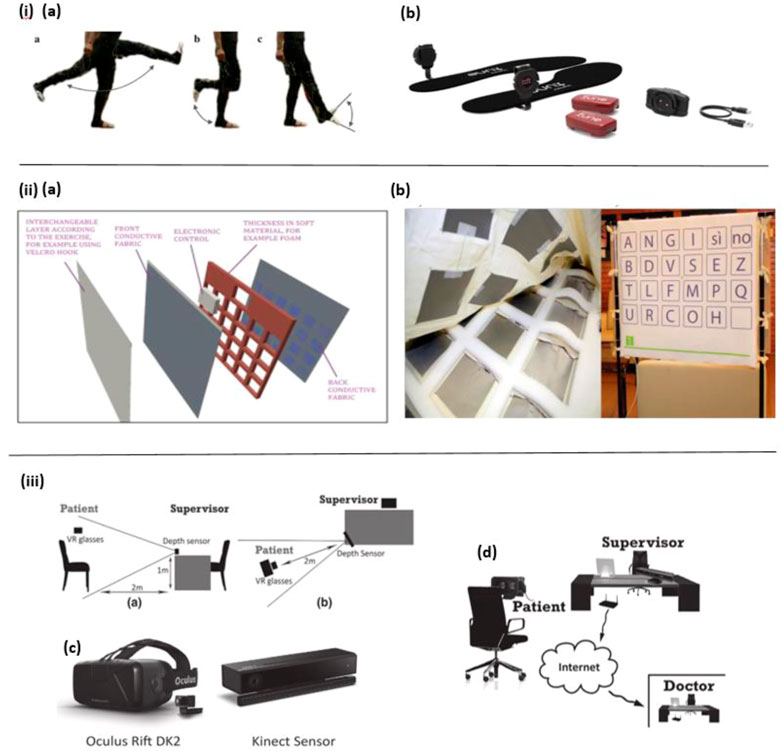
FIGURE 1. i) (A) Leg movement. (B) Motion detection device (Varatharajan et al., 2017). ii) (A) The SmartTapestry hardware. (B) Sensing components in the soft foundation layer (Left) and the SmartTapestry test set-up (Right). (Maselli et al., 2017). iii) (A) Side perspective displaying the layout of the experimental set-up. (B) Perspective from above displaying the layout of the experimental set-up. (C) Virtual reality glasses (Oculus Rift DK2) and depth sensor (Microsoft Kinect 2). (D) Early detection setup (Montenegro and Argyriou, 2017).
Moreover, in the work of Fiorini et al. an innovative method of walking behavior detection to diagnose MCI is presented. In this study, aerobic activities are combined with traditional cognitive tools: the TAP test (Test for Attentional Performances), to investigate the feasibility of classifying between MCI and healthy controls. For this reason, the SmartWalk test is adopted to integrate walking behavior and auditory sustained attention by using a wearable 9-axis inertial sensor (a 3-axis accelerometer, a 3-axis gyroscope, and a 3-axis magnetometer), which is fixed on the dominant foot of subjects. As a result, the SmartWalk test shows a positive correlation with the TAP test in cognitive assessment (Fiorini et al., 2017). In another work by Fiorini et al., a similar combined cognitive-physical SmartWalk tool (Sensor foot) is developed, which adds aerobic activity to the traditional cognitive protocols, to explore its correlation with a traditional test in measurement and stimulate cognitive function. In this study, a wider range of subjects has been recruited to participate in the test. Similarly, a wearable 9-axis inertial sensor (a 3-axis accelerometer, a 3-axis gyroscope, and a 3-axis magnetometer) is taped to subjects’ dominant foot to collect walking data. Consequently, the findings suggest that the SmartWalk test is positively associated with the traditional one, and could be useful in cognitive decline intervention (Fiorini et al., 2019).
Besides, daily physical activity behavior has been investigated in some studies. For instance, a wrist-mounted accelerometer is developed in the study of Fleming et al. to record habitual physical activity behavior in non-demented participants with down syndrome (DS). Based on the measured data, the correlation study between participants’ physical activity behavior, cognitive functioning, and imaging biomarkers of Alzheimer’s disease have been conducted. The experimental results revealed that time spent in sedentary behavior is negatively correlated with cognitive functioning, while time spent in moderate-to-vigorous activity behavior correlates with cognitive functioning positively (Fleming et al., 2021). In a similar study by Lu et al., patterns of physical activity and sedentary behavior are compared among participants with AD, MCI, and normal control group in Hong Kong. To obtain these biomarkers a wrist-worn accelerometer has been mounted on every subject’s wrist for 7 days. The results show that AD subjects have longer time sedentary behavior and more sedentary bout than other groups during the day (Lu et al., 2018).
The wearable biosensor offers many advantages, such as portability, comfort, convenience, and allowing for continuous point-of-care testing (Zhang et al., 2021a). At the same time, it has some drawbacks, for instance, some of them are bulky to wear, distracting, and cause tension (Jonell et al., 2021). As a result, in certain cases, some non-wearable biosensors are introduced to create an experimental environment with minimal interference to subjects and maintain the ecological validity of the recorded data, such as ambient-based in-home wireless sensors and Kinect (depth) sensors, etc. (Jonell et al., 2021). For example, Maselli et al. designed a sensor-based tapestry to assess and train cognitive functions by combining the measurement of episodic memory and motor (upper limb articulation movement) in the tasks. The SmartTapestry consists of a sensitive base, interchangeable layers, a laptop and a mobile support structure for the tapestry. Based on this device, subjects’ cognitive and motor functions are assessed and trained simultaneously (Figure 1II ). Compared with the traditional approach, this novel tool suggests that the SmartTapestry plays an equivalent role in the assessment and rehabilitation of physical and cognitive function (Maselli et al., 2017).
Furthermore, Urwyler et al. constructed an in-home wireless sensor system, comprising ten unobtrusive senor boxes inside the apartment to detect subjects’ activities of daily living (ADL). These ten sensor boxes were installed in ten different places of the apartment and used to capture light, temperature, humidity, movement and acceleration values with his five ambient sensors. The results show that the recognized ADL data is useful to discriminate between dementia patients and healthy participants with an accuracy of 95% (Urwyler et al., 2017).
Besides, in the study of Fernandez Montenegro et al. a depth sensor is used to track the subjects’ body movements and animate them to improve subjects’ interactivity in the VR environment (Figure 1III). In this way, the subjects wearing the VR glasses can perform the required cognitive tasks in the full-immersive VR environment. In this work, although body behaviors were only recorded and animated, not analyzed and used for diagnosis of AD, it still presents a promising solution for behavior detection in AD diagnosis (Montenegro and Argyriou, 2017).
2.2 Eye movement behavior detection of Alzheimer’s disease
Eye movement parameters play a significant role in cognitive function assessment, they can reflect human cognitive and mental state more easily compared to other bio-signals (Zhang et al., 2016), and abnormal viewing behavior has been found among subjects with neurodegenerative conditions in eye movement studies (Anderson and Macaskill, 2013). With the advances in eye-tracking technologies, eye movement parameters, such as gaze, saccade, blink etc. Have gained growing interest in various medical fields, including Alzheimer’s disease diagnosis (Crawford et al., 2013; Imaoka et al., 2021). Studies have shown that the brain aggregates information about the position of the eyes and hands in the posterior parietal cortex (PPC), and that anatomical changes in the brain regions of AD patients can lead to damage in the PPC region, which can affect hand-eye coordination tasks. In visuomotor tasks, detecting whether a target’s performance has deficits that are not present relative to age-matched controls provides a preliminary diagnosis of whether the target is ill. On the other hand, specific indicators of the eye and pupil can reflect to some extent the level of cognition, and the analysis of indicators such as the number of gaze durations during visual gaze can also be used to assist in the detection of disease (Skaramagkas et al., 2021).
In many related studies, biosensor-based devices have been constructed in an experimental setup to record eye movement data of AD patients. These biosensors are mainly infrared sensing devices, including wearable devices, e.g., eye-tracking glasses, head-mounted VR eye trackers etc., and non-wearable devices, e.g., desk-mounted eye trackers, handheld pupillometer, infrared eye-tracking camera etc. For example, in the work of Fraser et al. a desk-mounted eye tracker is constructed for the measurement of eye-movement features in reading tasks. By analyzing the recorded data, 13 gazes, saccade, and fixation-related features are considered. Ultimately, with the help of machine learning analysis, the healthy control and AD subjects can be distinguished at an accuracy rate of 86% (Fraser et al., 2017).
Nam et al. constructed a sensor-based experimental setup to detect eye movement, head pose and their correlation (Figure 2I). To measure gaze behavior naturally, a camera rather than a wearable eye-tracker is equipped to record participants’ face video data. The findings suggest that AD patients’ eyes move with the head in the vertical orientation at the same time, whereas the healthy control did not show such behavior features. In conclusion, this eye and head movement behavior detection could be significantly useful in screening for AD (Nam et al., 2020).
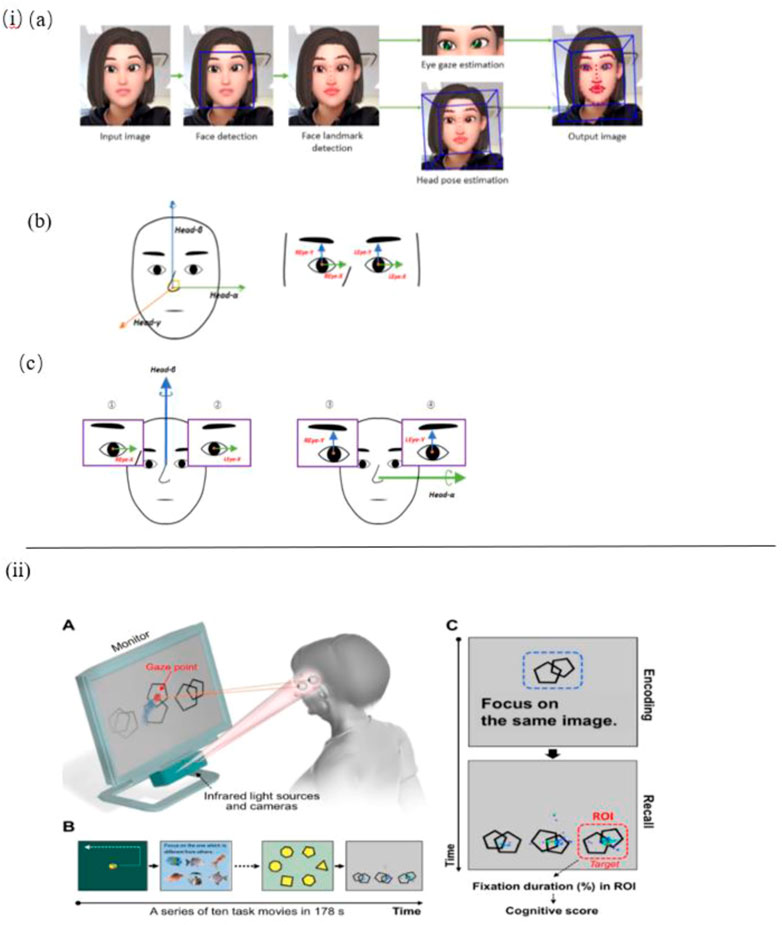
FIGURE 2. i) (A) Facial and eye movement extraction OpenFace 2.0. (B) Coordinate axes: face and eye. (C) A pair of axes was used to obtain the correlation coefficient: horizontal and vertical (Nam et al., 2020). ii) (A) Eye-tracking system. (B) Tasks for rapid cognitive assessment. (C) An example of a working memory task and representative gaze plots (Oyama et al., 2019).
In the work of Tadokoro et al. a novel infrared-based eye tracking camera is constructed to capture gaze points in the eye tracking test. Participants are asked to perform this 3-min image task displayed on a computer monitor with an audio explanation, the data recorded is used to analyze eye tracking scores and classify NC (Normal Control), MCI (Mild Cognitive Impairment), and AD subjects. As a result, it shows that eye tracking scores are correlative with mini-mental state examination (MMSE) scores and it is an effective and rapid method to detect early cognitive impairment (Tadokoro et al., 2021). In another work by Oyama et al., the very same eye-tracking device is used to perform a rapid cognitive assessment and show good diagnostic performance (Figure 2II) (Oyama et al., 2019).
In the study of Granholm et al., a handheld infrared sensor-based pupillometer is utilized to record pupil response evoked in cognitive tasks to detect biomarkers of early mild cognitive impairment (MCI) and AD risk prediction. By using these pupillometer devices MCI and cognitively normal (CN) participants can be differentiated, whose results are correlative with those in locus coeruleus examination (Granholm et al., 2017).
Apart from the non-wearable eye-tracking sensors mentioned above, various wearable eye trackers are applied in some works. For instance, Sciarrone et al. present a wearable sensor-based glasses to measure the Essential Tremor (ET) of the head and the number of Eye Blinks (EBs) simultaneously for early-stage AD patients (Figure 3I). These behavioral symptoms can be precisely detected, with an accuracy of 97% for ET, and Root Mean Square Error (RMSE) around 0.4 for EBs (Sciarrone et al., 2020).
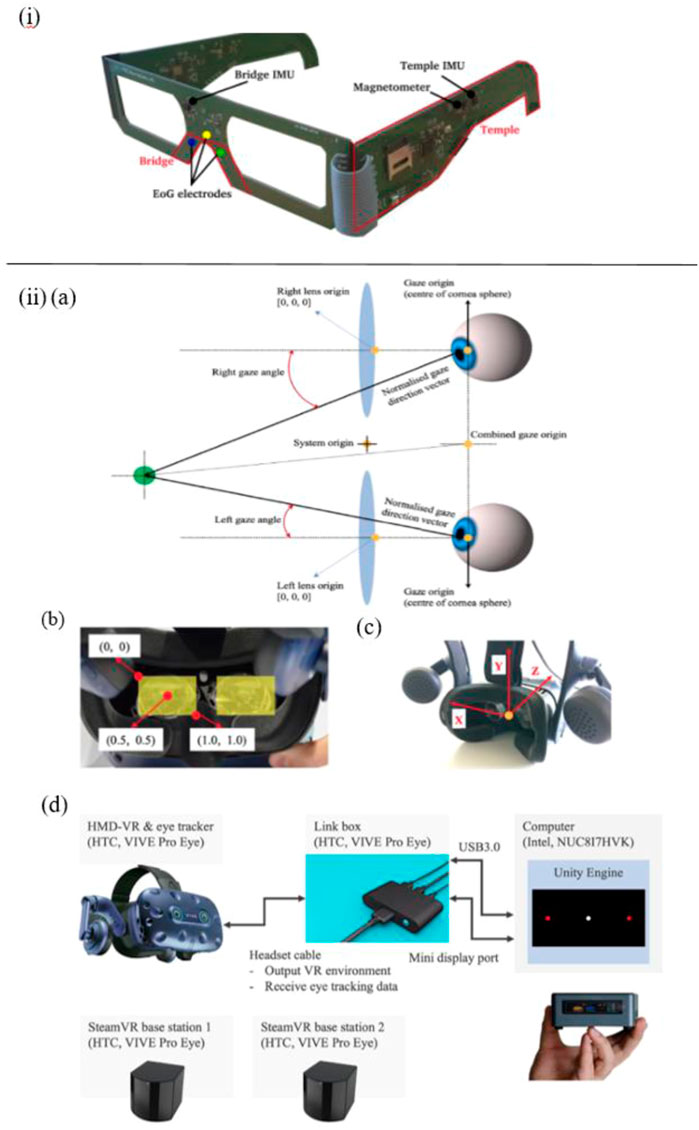
FIGURE 3. i) The primary parts of the glasses and an illustration of the employed sensors (Sciarrone et al., 2020). ii) (A) VIVE Pro Eye’s coordinated eye tracking system. (B) Coordinate system of pupil position data from the user’s perspective. (C) Coordinate system of gaze direction vector from user’s perspective. (D) Experimental technology for measuring saccadic eye movement utilizing HTC VIVE Pro Eye (Yu et al., 2020).
In addition, Davis et al. reported a feasibility study of using virtual reality (VR) and eye tracking techniques in Way-finding tasks for Alzheimer’s disease research. In this study, wearable sensor-based eye tracking glasses with two cameras were applied to record video. One camera was used to record VR environments, which were projected on a 12-foot screen. Another one was used to capture subjects’ gaze data via the optical sensor device. In this way, 60% of eye-tracking videos of subjects who finished all trails were complete and usable, which proved the feasibility of using projected VR and eye tracking in large-scale wayfinding tasks for AD detection, but some limitations should be taken into consideration, such as joystick issues, simulation sickness, and calibration issues, etc. (Davis, 2021). Besides, compared with the eye tracker constructed in the projected VR environment mentioned in the study of Davis et al., Yu et al. employed a more immersive eye tracking device: a novel head-mounted VR device, in which a sensor-based eye tracker is embedded (Figure 3II). To be specific, biosensors installed in this VR device include steam VR tracking, accelerometer, gyroscope, proximity, interpupillary distance (IPD) sensor, near-infrared LED, and infrared camera. In their work, this wearable VR device is utilized to record saccade and other eye movement metrics in pro- and anti-saccade tasks. Consequently, the data analysis results indicate that it is able to measure saccadic eye movement parameters despite the technical limitations on time-linked parameters assessment (Yu et al., 2020).
2.3 Speech behavior detection of Alzheimer’s disease
Speech testing plays a critical part in the medical diagnosis of several neurodegenerative diseases (Veronica et al., 2017). Over the past years, studies have proved that a considerable percentage of AD patients suffer from vocal communication problems (Habash et al., 2012), and impairment in speech and language possibly be a powerful predictor of MCI and AD (Ammar and Ayed, 2020), some studies even suggested that speech behavior changes might be one of the earliest indicators of cognitive decline, frequently noticeable years before other cognitive impairments become apparent (Beltrami et al., 2018). The researchers analyzed a large number of language samples to extract the features, quantified the language automatically and combined it with machine learning classification methods to distinguish healthy controls from AD patients by detecting target language skills. Meanwhile, due to the non-invasive, convenient, and low-cost properties of speech analysis techniques (Pulido et al., 2020), various works have focused on conducting speech behavior analysis to obtain early indicators for the diagnosis of early Alzheimer’s disease (Samrah et al., 2013; Laske et al., 2015). For this purpose, various acoustic sensors have been employed to capture vocal signals from subjects’ speech.
For instance, López-de-Ipiña et al. proposed an automatic spontaneous speech analysis (ASSA) method for early Alzheimer’s disease diagnosis. Based on an automatic Voice Activity Detection (VAD) the recorded spontaneous speech and emotional speech analysis are conducted automatically (Figure 4I). By processing these vocal parameters, including duration, time domain, frequency domain, acoustic, and voice quality features, AD patients’ vocal features are identified (López-de-Ipia et al., 2013).
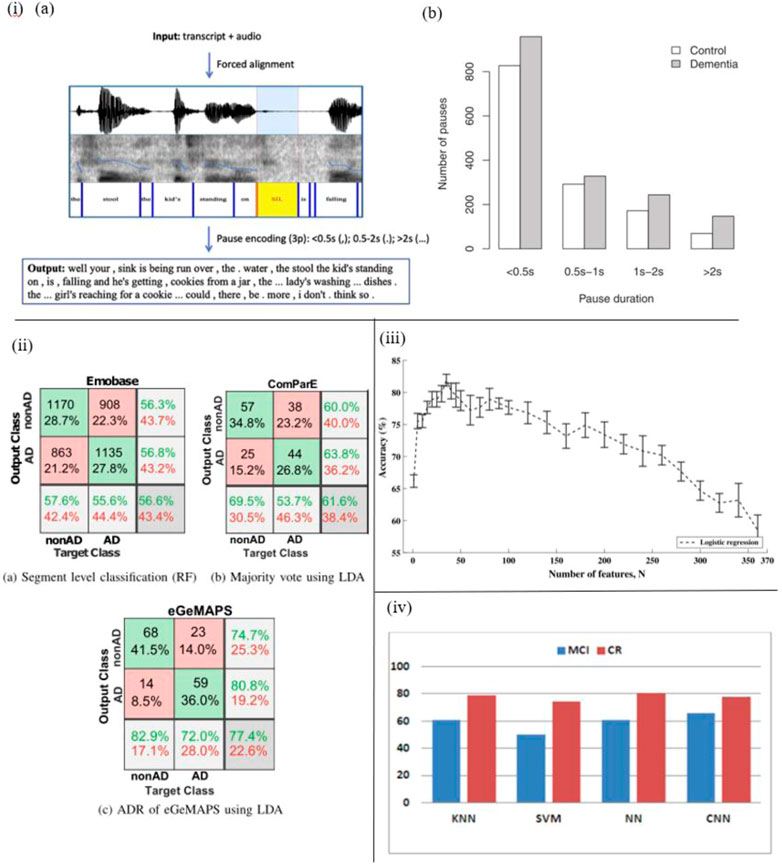
FIGURE 4. i) (A) The pause encoding process. (B) AD patients pause more often (in all duration bins) (95). ii) Confusion matrices that include the top outcomes from every experiment, as well as precision, recall, and total accuracy (93). iii) Accuracy and standard errors, divided by the N predictor characteristics employed (91). iv) CER (%) for classes and selected classifiers, including convolutional neural network, k-nearest neighbors, support vector machines, multilayer perceptron with L layers and N neurons (96).
In another work by Fraser et al., with the help of sound sensor short narrative samples are extracted by performing a picture description task, and processed to form linguistic and acoustic variables. Moreover, these variables are used to train the machine learning classifier to classify AD and healthy subjects. Based on experiments, four linguistic features including semantic impairment, acoustic abnormality, syntactic impairment, and information impairment are proved to be useful in the recognition of AD, and the accuracy of this method reaches up to 81% (Fraser et al., 2015) (Figure 4III).
Laszlo et al. applied a dedicated automatic speech recognition (ASR) tool in automatic MCI screening. By analyzing the spontaneous speech of participants in two tasks, acoustic parameters are extracted automatically. In addition, machine learning algorithms are applied to assist distinguish MCI from healthy subjects. The results demonstrate that the speech tempo in the delayed recall task and the number of pauses in the question-answering task are the most notable differences between these two groups (Laszlo et al., 2018).
Haider et al. studied purely paralinguistic acoustic features of subjects’ spontaneous speech using a microphone and voice activity detection system. Based on this, four feature sets, e.g., Emobase, ComParE, eGeMAPS, and MRCG functionals are extracted and processed for AD detection with Machine Learning and Active Data Representation (ADR) methods. As a result, this work shows that such comprehensive acoustic feature sets of spontaneous speech are correlated with cognitive function, and might contribute to AD screening and diagnosis (Haider et al., 2020) (Figure 4II).
In the work of Calzà et al., linguistic feature modifications caused by cognitive decline have been studied to detect Mild Cognitive Impairment (MCI) and dementia via Natural Language Processing (NLP) techniques. To this aim, an Olympus-Linear PCM Recorder LS-5 is employed to record speech data of subjects during the implementation of three spontaneous speech tasks. In addition, acoustical, rhythmical, lexical, morpho-syntactic features and readability domains are extracted automatically by means of created algorithms, and then automatic classifiers are trained to discriminate between healthy control and MCI subjects (Figure 4III). The findings suggest that this automatic diagnosis system is able to distinguish healthy subjects from MCI (high F1 score, approximately 75%) (Calzà et al., 2021). The same recorder (Olympus-Linear PCM Recorder LS-5) has been applied by Beltrami et al. to record spontaneous speech during three tasks designed for 96 participants. As a result, many speech features, such as acoustic lexical and syntactic parameters are transcribed and analyzed by Natural Language Processing (NLP) to discriminate between healthy controls and cognitively impaired participants. The results indicate that this method might be a promising tool to recognize early-stage cognitive deficits (Beltrami et al., 2018).
Besides, several researchers studied disfluencies and pauses in speech to detect cognitive impairment (Yuan et al., 2021) (Figure 4I). López-de-Ipiña proposed an automatic analysis method of speech and disfluencies to assist MCI diagnosis. For this purpose, 40 speech samples from the MCI group and 60 from the control group are recorded by a sensor-based sound recorder, and automatically segmented into disfluencies via the VAD algorithm. Employing non-linear multi-feature modeling and deep learning approaches, the experiment shows hopeful results and provides a novel research direction (Lopez-De-Ipina et al., 2017) (Figure 4IV). Similarly, Yuan et al. studied disfluencies and language problems in Alzheimer’s Disease as well, by using fine-tuning Transformer-based pre-trained language models, e.g., BERT and ERNIE. In this work, 108 speakers’ speech samples in the training set and 48 speakers’ speech samples in the test set are recorded by recorders. As a result, 89.6% accuracy on the test set of the Alzheimer’s Dementia Recognition through Spontaneous Speech has been achieved and the conclusion is drawn: AD patients speak uh more frequently than speak um (Yuan et al., 2020).
2.4 Multimodal detection of Alzheimer’s disease
In the studies mentioned above, most of the sensors have been used in isolation to detect behavior parameters. However, every single sensor has its merits as well as demerits, they cannot measure all the metrics needed alone (Alberdi et al., 2016). Therefore, a technology that can fuse all sensors is getting more attention in this field (Yang et al., 2022). Recently, the multi-sensor detection model is gaining more popularity in behavior detection (Hoque et al., 2015; Alberdi et al., 2016). Many studies have revealed that it is feasible to obtain comprehensive information on patients’ activity behavior in an indoor or experimental environment, based on the dense sensing system and sensor fusion technology (Abe et al., 2015; Stavropoulos et al., 2015; Chen et al., 2019). Thus, this strategy greatly improves the efficiency of patients’ behavior detection and has a more effective analyzing mechanism in assessing patients’ activity behavior with variable intensities compared to the conventional method (Deep et al., 2020).
As a result, in various indoor environments, multimodal detection sensors have been deployed to record and assess multiple behavioral signals of AD patients. For example, Jonell et al. proposed a multimodal capture method to detect AD patients’ behavior in a real clinical environment by using nine sensors, which include three smartphone cameras, a tablet, an eye tracker, a microphone array, a health wristband, a thermal camera, and an overview camera. To minimize distraction to the subjects and ensure the ecological validity of the recorded data, most of the installed sensors except for the health wristband are non-wearable. With their help, multimodal behavioral data are collected, such as facial gestures recorded by the patient camera (Smartphone Camera), gaze and pupil dilation by Eye Tracker, voice quality (breathiness, vocal strength), pauses, speech rate by Microphone Array, pen movement and pen pressure by Tablet, thermal emission data by Thermal Emission Camera, heart rate, galvanic skin response and acceleration by Health Wristband in clinical condition. In this way, the clinical feasibility of this sensor system is demonstrated by relating these digital biomarkers to traditional clinical assessment methods and established biomarkers (Jonell et al., 2021).
In addition, Alvarez et al. developed a novel multimodal sensing system to capture and analyze AD patients’ physical abnormal behavior in daily motion. In this system various sensors are employed, including multisensory smart bands to offer blood pressure and skin temperature, an accelerometer, gyroscope, and magnetometer to capture motion data, a Binary sensor to detect whether doors and drawers are open, an RGB-D camera (Kinect) to collect deep motion data, a Zenith camera to record 360-degree panoramic view and Wireless sensor network (WSN) anchor or beacons to extract radio signals from wearable devices (Figure 5I). Therefore, based on these technical approaches, this system integrated with Internet of things devices and user interactions is able to provide automatic, distant monitoring of AD patients (Alvarez et al., 2018). In another work by Alvarez et al., the very same sensor-based devices were integrated into an ICT4LIFE platform to capture visual, motion and depth data of AD patients’ abnormal behavior (Figure 5IV ). Based on these data, behavior patterns are recognized and reported to the interested party to take appropriate actions (Alvarez et al., 2017).
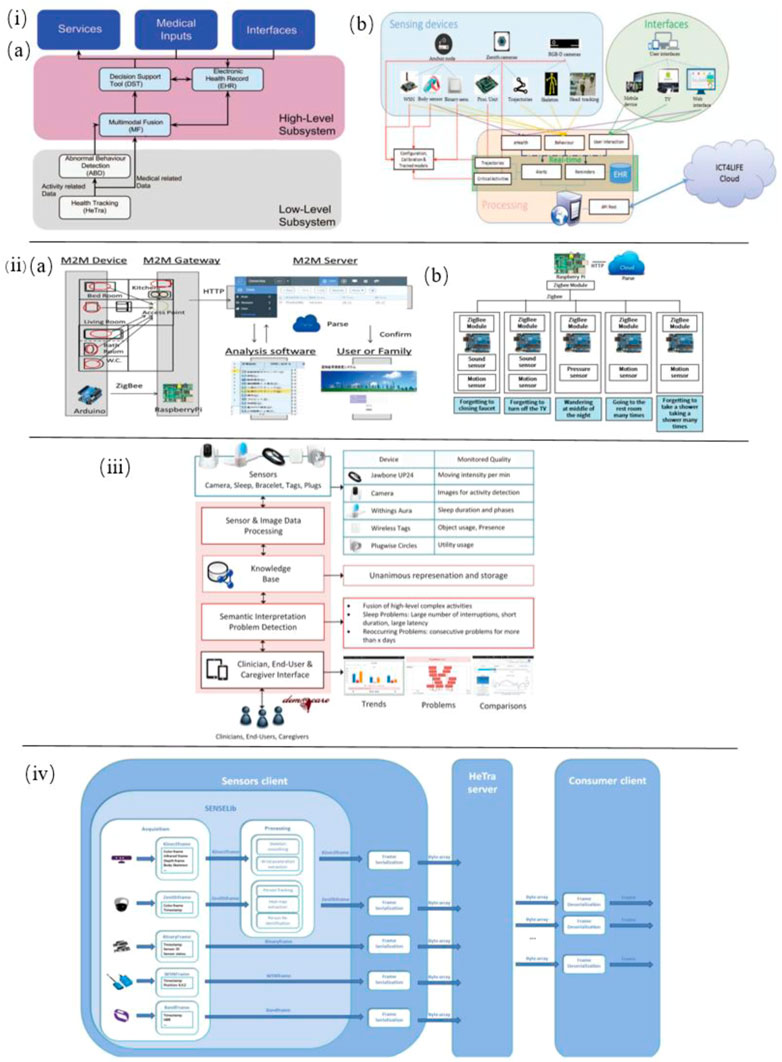
FIGURE 5. i) (A) Overview of the complete system architecture. (B) Low-level subsystem architecture for sensor-based health tracking (Alvarez et al., 2018). ii) (A) Schematic of the M2M System. (B) M2M device gateway (Ishii et al., 2016). iii) Architecture of the smart home monitoring system (Lazarou et al., 2016). iv) Sensor-based HeTra subsystem architecture overview (Alvarez et al., 2017).
Ishii et al. proposed a sensor network system toward the early detection of dementia for elderly people living alone. Inside their house, various sensors were installed to identify behaviors indicating initial symptoms of dementia. With the help of the M2M (Machine-to-Machine)/IoT (Internet of Things) platform these sensors’ data are recorded and analyzed for diagnosis of dementia. In this system, sensors such as sound sensor, pressure sensor, and motion sensor are applied to determine whether forgetting to close a faucet or turn off the TV, wandering in the middle of the night, going to the restroom many times, forgetting to take a shower and taking a shower many times (Figure 5II) (Ishii et al., 2016).
Zhi et al. studied the elderly’s behavior to predict the risk of Alzheimer’s disease by using IoT room sensors for location capture. Based on this, behavioral analysis models are developed to detect the three main variables of elderly behavior: sleeping patterns, excess active levels, and repetitive actions. In this work, 20 elderly people living independently participated in this 6-months-period experiment, and their accommodations five sensors are deployed. The experimental results show these behavior variables detected by IoT sensors are useful in predicting the early symptoms of potential Alzheimer’s disease (Zhi et al., 2017).
Lazarou et al. developed a multi-sensor-based intelligent home monitoring system for the elderly with cognitive deficits (Figure 5III). Data on sleep behavior and physical behavior of daily living have been collected and visualized. The results suggest that REM sleep in sleep quality is a key indicator to assess cognitive status among the detected abnormalities (Lazarou et al., 2016).
Apart from indoor living environments, multi-sensors have been utilized in specific experimental tasks. For instance, Staal et al. introduced a novel non-invasive, and time-saving method to predict Mild Cognitive Impairment (MCI) and Alzheimer’s Disease (AD) by detecting visuomotor network dysfunctions as potential biomarkers. In this study, three eye tasks and five eye-hand tasks were executed with the help of a touchscreen to display the stimulus, a head-mounted infrared eye-tracking system to record eye movement data, and an infrared motion capture system to record hand movements data. The data processed suggest that eye-hand tasks perform better in accuracy, sensitivity, and specificity than eye tasks to classify control, MCI, and AD. In addition, visuomotor features are potential biomarkers to predict MCI and AD (Staal et al., 2021). Moreover, visuomotor behavior has also been shown to be impaired in the early stage of Alzheimer’s Disease by Hawkins et al. by employing a touchscreen to perform four visuomotor tasks (Figure 6II) (Hawkins and Sergio, 2014).
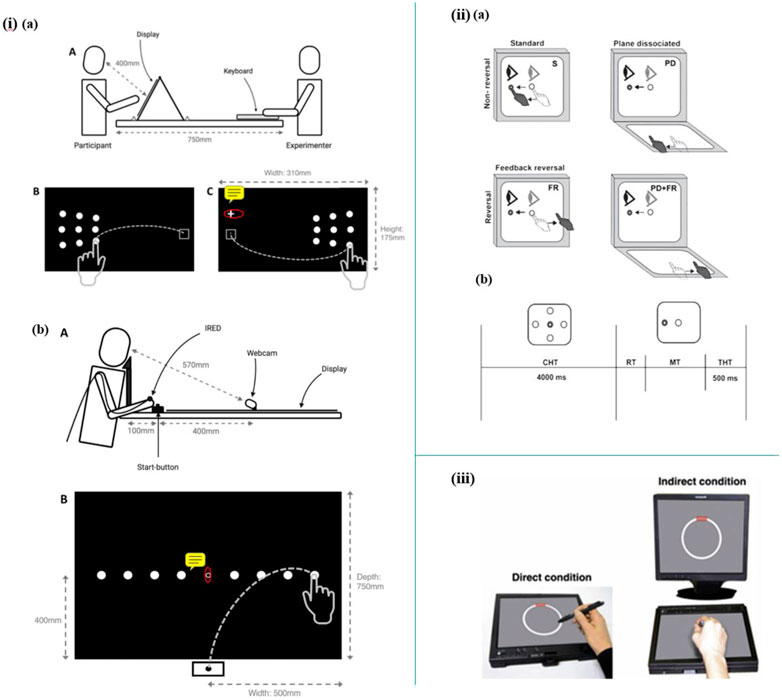
FIGURE 6. i) (A) Schematic diagram of lateral reaching task. (B) Schematic diagram of radial reaching task (Mitchell, Rossit, Pal, Hornberger, Warman, Kenning, Williamson, Shapland, McIntosh). ii) (A) Schematic diagram illustrating the four experimental conditions. (B) Trial timing (Hawkins and Sergio, 2014). iii) Setup of the circle-tracing task (Kirsty et al., 2017).
Mitchell et al. reported a novel research on peripheral reaching movements in AD and MCI. In the peripheral reaching tasks, participants are required to reach stimuli displayed in their peripheral vision. Meanwhile, the reaching movements data were tracked by using an infrared motion-tracking camera, which sampled the 3D position of infrared-emitting diodes (IREDs) taped to subjects’ fingernails (Figure 6I). The results show that movement features observed in these peripheral reaching tasks are not common behavior markers of AD. However, the prolonged reaching time is possibly correlated with visuomotor impairments and may indicate a potential risk of AD and MCI (Mitchell, Rossit, Pal, Hornberger, Warman, Kenning, Williamson, Shapland, McIntosh).
Kirsty et al. established a sensor-based setup to explore whether the deficits in visuomotor integration behavior are early-stage symptoms of cognitive impairment of autosomal dominant familial AD (FAD). In this work, a sensor-based tablet screen and a stylus are employed in 12 circle-tracing tasks, which include six direct tracing tasks and six indirect tracing tasks (Figure 6III). As a result, the analyzed data suggest that measurement of visuomotor integration deficits could be a promising approach to predict FAD (Kirsty et al., 2017).
Additionally, in the work of Shinkawa et al., the combination of speech and gait behavioral data has been studied with the help of three microphones (a throat microphone, a lavalier microphone, and the iPad’s internal microphone) and a motion capture system. Based on the collected multimodal behavior data: voice and body position data, healthy control and MCI group have been discriminated with an accuracy of 82.4%, increased by 5.9% than in single modality condition (Shinkawa et al., 2019).
3 Conclusion and future perspectives
In this review, the state-of-the-art applications of sensors toward behavior detection in the diagnosis of Alzheimer’s disease have been overviewed from four different perspectives: body motion behavior detection, eye movement behavior detection, speech behavior detection, and multi-behavior detection. The specific examples of these applications have been tabulated in Tables 1–4 in detail. As can be seen from the above table, the results of the multimodal integrated test is the most accurate method in the diagnosis of Alzheimer’s disease and is usually able to distinguish between normal and abnormal behaviours with good adaptability, the eye-movement behavioural tracking approach requires an experimental environment and has high requirements for the testing environment, but has a good future as it can reach an accuracy of up to 97%. Lastly, physical behavioural monitoring, with its good correlation between experimental results and those of traditional tools and low requirements for the diagnostic environment, is a good diagnostic tool for Alzheimer’s disease.
At this stage, there are three main methods to detect Alzheimer’s disease: 1. The neurologist assesses the target’s cognitive status using a special assessment form.; 2. Through puncture sampling to check for two typical biomarkers in the cerebrospinal fluid such as TAU protein and beta amyloid (Hyman et al., 2012) 3. Through magnetic resonance plus angiography of the head to detect neurodegeneration, atrophy, etc (Braak and Braak, 1994). All of these methods require specialist personnel or specialist instruments, even for the working environment, and some of the results can be subjective to the level of expertise of the assessor, making the development of a variety of wearable, portable, small, non-intrusive sensing devices an urgent challenge.
For the detection of physical behaviour, the sensors can often be worn for longer periods of time and observed for longer periods of time, but the relationship between prolonged physical activity and cognitive function still requires further research, as there are relatively more factors that can affect physical activity and more interfering factors that cannot be accurately ruled out (Fleming et al., 2021).
Traditional screen-based eye-tracking technology usually requires the head to be immobilised in order to obtain information about eye movements and visual attention, which restricts the subject’s movement and reduces the experience of using the device. The new VR-style device is relatively more tolerable for testing equipment, so that the subject’s movement is no longer restricted and can better cooperate with the test (Davis, 2021).
Language impairment is present in a wide range of neurodegenerative diseases and can be assessed by natural language tests or by detecting speech signals in conversations etc. The two can be cross-checked and for the extraction of speech signals it is even possible to differentiate between differences caused by the primary disease, ageing and dementia, making the assessment results more accurate, but the level of speech is influenced by the speaker’s prior or unconscious, while environmental sounds can also influence speech Acoustic detection (Yang et al., 2022) (Coupland, 2007) (Chen et al., 2019). For people with different cultural and educational backgrounds, picture description tasks are not fully applicable and can affect the accuracy of clinical applications.
In general, non-invasive biosensors are favored in most recent behavior detection related studies of AD diagnosis, considering the safety and comfort factors. Besides, both wearable and non-wearable biosensors have been extensively applied in different research settings, respectively. Specifically, biosensors employed in body motion detection are mainly wearable biosensors, such as foot-mounted sensors (e.g., SmartWalk) and wrist-mounted sensors (wrist-mounted accelerometer), which allow subjects to move their body at different positions as required in an experiment, and record their physical behavior data in real-time. Meanwhile, the biosensors used for eye movement behavior detection include both wearable (e.g., eye-tracking glasses) and non-wearable (e.g., desk-mounted eye-tracker), because in some motionless experiment settings, to obtain more natural eye movement behavior data non-wearable sensors are favored (Nam et al., 2020), while some body motion required tasks or virtual experimental environments wearable sensors are preferred devices (Yu et al., 2020; Davis, 2021). However, when it comes to speech behavior and multi-behavior detection, the majority of biosensors introduced are non-wearable categories, to reduce interference with the subjects, which may include, for instance, desk-placed voice recorders, Kinect (depth) sensors, smartphone cameras, and microphone, etc. (Jonell et al., 2021). Therefore, they are mostly unobtrusively installed in the surrounding environment.
In the future, several more prominent trends will emerge in the use of behavior detection sensors in AD diagnostics. Firstly, more sensor-based behavior detection experiments will be integrated into the Internet of Things (IoT) platform. Significant advances in IoT technology have been achieved in medical detection, and it will be more widely used in behavior detection due to its great advantages (Javdani and Kashanian, 2017). IoT systems can generate and transmit signals to professionals, which allows them to gather behavior data in real-time and to continuously monitor and assess patients’ behavior remotely 24/7. Based on this technology, AD patients, especially those elderly patients living alone can be monitored continuously, their abnormal behavior can also be detected at any time. In this way, it provides a possibility to detect potential risks of disease among them at an early stage.
Secondly, multi-behavior sensing technology will be favored in various detection and monitoring experiment. Compare to the single modality approach, multimodal sensing has higher accuracy in AD assessment (Shinkawa et al., 2019). In the future, the development trend of behavior detection in AD diagnosis is to sense large-scale multimodal information based on dense-sensing system and sensor fusion technology (Gravina et al., 2016) and capture various digital markers from physiological signals, environmental information, and body motion data simultaneously. By collecting and analyzing such comprehensive data, behavior detection is a more promising method in AD diagnosis at an early stage.
Finally, Virtual Environments (VEs) and Virtual Reality (VR) technology will gain more popularity in medical diagnosis and rehabilitation therapies owing to the advance in state-of-the-art technology in computer and communication science (Konstantinidis et al., 2017). As a result, based on immersive technology and Human-Computer Interaction (HCI), novel Alzheimer’s screening tests in virtual environments has been introduced and is drawing more attention to AD diagnosis (Montenegro and Argyriou, 2016). This is because VR technology makes it possible to provide an alternative solution to the traditional cognitive testing approach in AD, due to its safe, immersive experimental scenarios and the possibility of manipulating reality (Montenegro et al., 2020). Up to now, the virtual environment applied in AD assessment is mainly classified into two categories: full-immersive (Yu et al., 2020) and semi-immersive (Davis, 2021). Among them, the most commonly used sensor-based device is head-mounted VR, which can be embedded in an eye-tracking module to detect eye movement and connected with a Kinect (depth) sensor to detect body motion behavior. In the future, more multi-sensors will be added to it to capture a greater variety of behavioral signals, such as sound and physiological information, etc.
Author contributions
XS and SZ conceived and designed this review paper; XS and other authors wrote this review paper; XS and QW revised this review paper.
Conflict of interest
The authors declare that the research was conducted in the absence of any commercial or financial relationships that could be construed as a potential conflict of interest.
Publisher’s note
All claims expressed in this article are solely those of the authors and do not necessarily represent those of their affiliated organizations, or those of the publisher, the editors and the reviewers. Any product that may be evaluated in this article, or claim that may be made by its manufacturer, is not guaranteed or endorsed by the publisher.
References
Abe, Y., Toya, M., and Inoue, M. (2013). Early detection system considering types of dementia by behavior sensing. Consum. Electron.
Abe, Y., Toya, M., and Inoue, M. (2015). “Early detection system of senile dementia by behavior sensing,” in IEEE International Symposium on Consumer Electronics.
Alam, R., Gong, J., Hanson, M., Bankole, A., Anderson, M., Smith-Jackson, T., et al. (2017). Motion biomarkers for early detection of dementia-related agitation. Workshop on Digital Biomarkers, 15–20.
Alberdi, A., Aztiria, A., and Basarab, A. (2016). On the early diagnosis of alzheimer's disease from multimodal signals: A survey. Artif. Intell. Med. 71, 1–29. doi:10.1016/j.artmed.2016.06.003
Alemdar, H., and Ersoy, C. (2010). Wireless sensor networks for healthcare: A survey. Comput. Netw. 54, 2688–2710. doi:10.1016/j.comnet.2010.05.003
Alvarez, F., Popa, M., Solachidis, V., Hernandez-Penaloza, G., Belmonte-Hernandez, A., Asteriadis, S., et al. (2018). Behavior analysis through multimodal sensing for care of Parkinson's and alzheimer's patients. IEEE Multimed. 25, 14–25. doi:10.1109/mmul.2018.011921232
Alvarez, F., Popa, M., Vretos, N., Belmonte-Hernandez, A., and Daras, P. (2017). “Multimodal monitoring of Parkinson's and Alzheimer's patients using the ICT4LIFE platform,” in 2017 14th IEEE International Conference on Advanced Video and Signal Based Surveillance (AVSS).
Amine, A., Mohammadi, H., Bourais, I., and Palleschi, G. (2006). Enzyme inhibition-based biosensors for food safety and environmental monitoring. Biosens. Bioelectron. X. 21, 1405–1423. doi:10.1016/j.bios.2005.07.012
Ammar, R. B., and Ayed, Y. B. (2020). Language-related features for early detection of Alzheimer Disease. Procedia Comput. Sci. 176, 763–770. doi:10.1016/j.procs.2020.09.071
Anderson, T. J., and Macaskill, M. R. (2013). Eye movements in patients with neurodegenerative disorders. Nat. Rev. Neurol. 9, 74–85. doi:10.1038/nrneurol.2012.273
Beltrami, D., Gagliardi, G., Rossini Favretti, R., Ghidoni, E., Tamburini, F., and Calzà, L. (2018). Speech analysis by natural language processing techniques: A possible tool for very early detection of cognitive decline? Front. Aging Neurosci. 10, 369. doi:10.3389/fnagi.2018.00369
Borzì, L., Mazzetta, I., Zampogna, A., Suppa, A., and Irrera, F. (2021). Prediction of freezing of gait in Parkinson's disease using wearables and machine learning. Sensors 21, 614. doi:10.3390/s21020614
Bouchard, K., Bilodeau, J.-S., Fortin-Simard, D., Gaboury, S., Bouchard, B., and Bouzouane, A. (2014). “Human activity recognition in smart homes based on passive RFID localization,” in 7th ACM International Conference on Pervasive Technologies Related to Assistive Environments (PETRA'14).
Braak, H., and Braak, E. (1994). Morphological criteria for the recognition of Alzheimer's disease and the distribution pattern of cortical changes related to this disorder. Neurobiol. Aging 15, 355–356. doi:10.1016/0197-4580(94)90032-9
Brazaca, L. C., Sampaio, I., Zucolotto, V., and Janegitz, B. C. (2019). Applications of biosensors in Alzheimer's disease diagnosis. Talanta 210, 120644. doi:10.1016/j.talanta.2019.120644
Bulling, A., Blanke, U., and Schiele, B. (2014). A tutorial on human activity recognition using body-worn inertial sensors. ACM Comput. Surv. 46, 1–33. doi:10.1145/2499621
Bungon, T., Haslam, C., Damiati, S., O'Driscoll, B., and Awan, S. A. (2020). Graphene FET sensors for Alzheimer's disease protein biomarker clusterin detection.
Calzà, L., Gagliardi, G., Favretti, R. R., and Tamburini, F. (2021). Linguistic features and automatic classifiers for identifying mild cognitive impairment and dementia. Comput. Speech & Lang. 65, 101113. doi:10.1016/j.csl.2020.101113
Casper, D., Boer, J. M., Johan, P., Johannes, V. D., Steen, F., and Mattace, R. (2015). Delays in manual reaching are associated with impaired functional abilities in early dementia patients. Dementia geriatric cognitive Disord.
Catarinucci, L., Donno, D. d., Mainetti, L., Palano, L., Patrono, L., Stefanizzi, M. L., et al. (2015). An IoT-aware architecture for smart healthcare systems. IEEE Internet Things J. 2, 515–526. doi:10.1109/jiot.2015.2417684
Chaccour, K., Darazi, R., Hajjam, A., and Andres, E. (2016). From fall detection to fall prevention: A generic classification of fall-related systems. IEEE Sensors J., 1.
Chen, R., Maljkovic, V., Sunga, M., Song, H. H., and Yaari, R. (2019). Developing measures of cognitive impairment in the real world from consumer-grade multimodal sensor streams in The 25th ACM SIGKDD International Conference.
Choi, D., Choi, H., and Shon, D. (2019). Future changes to smart home based on AAL healthcare service. J. Asian Archit. Build. Eng.
Coupland, N. (2007). Style (language variation and identity). Style Mean. sociolinguistic Struct., 32–53. doi:10.1017/CBO9780511755064
Crawford, T. J., Higham, S., Mayes, J., Dale, M., Shaunak, S., and Lekwuwa, G. (2013). The role of working memory and attentional disengagement on inhibitory control: Effects of aging and alzheimer's disease. Age 35, 1637–1650. doi:10.1007/s11357-012-9466-y
Davis, R. (2021). The feasibility of using virtual reality and eye tracking in research with older adults with and without alzheimer's disease. Front. Aging Neurosci. 13, 607219. doi:10.3389/fnagi.2021.607219
de, Boer, C., Mattace, F., Raso, J., and van der, S. (2014). P032: Eye–hand coordination: A new behavioral biomarker for early-stage alzheimer's disease. Eur. Geriatr. Med.
Deep, S., Zheng, X., Karmakar, C., Yu, D., Hamey, L., and Jin, J. (2020). A survey on anomalous behavior detection for elderly care using dense-sensing networks. IEEE Commun. Surv. Tutorials 22, 352–370. doi:10.1109/comst.2019.2948204
Diaconu, Ș., Falup-Pecurariu, O., Tint, D., and Falup-Pecurariu, C. (2021). REM sleep behaviour disorder in Parkinson's disease (Review). Exp. Ther. Med. 22 2, 812. doi:10.3892/etm.2021.10244
Fan, Z., Yin, J., Song, Y., and Liu, Z. (2020). Real-time and accurate abnormal behavior detection in videos. Mach. Vis. Appl. 31, 72. doi:10.1007/s00138-020-01111-3
Fiorini, L., Maselli, M., Castro, E., Tocchini, S., and Cavallo, F. (2017). “Feasibility study on the assessment of auditory sustained attention through walking motor parameters in mild cognitive impairments and healthy subjects,” in 2017 39th Annual International Conference of the IEEE Engineering in Medicine and Biology Society (EMBC).
Fiorini, L., Maselli, M., Esposito, R., Castro, E., Mancioppi, G., Cecchi, F., et al. (2019). Foot inertial sensing for combined cognitive-motor exercise of the sustained attention domain. IEEE Trans. Biomed. Eng. 66, 2413–2420. doi:10.1109/tbme.2019.2906758
Fleming, V., Piro-Gambetti, B., Patrick, A., Zammit, M., Hartley, S. L., Christian, B. T., et al. (2021). Physical activity and cognitive and imaging biomarkers of alzheimer's disease in down syndrome. Neurobiol. Aging 107, 118–127. doi:10.1016/j.neurobiolaging.2021.07.016
Fleury, A., Vacher, M., and Noury, N. (2010). SVM-based multimodal classification of activities of daily living in health smart homes: Sensors, algorithms, and first experimental results. IEEE Trans. Inf. Technol. Biomed. 14, 274–283. doi:10.1109/titb.2009.2037317
Fraser, K. C., Fors, K. L., Kokkinakis, D., and Nordlund, A. (2017). “An analysis of eye-movements during reading for the detection of mild cognitive impairment,” in Proceedings of the 2017 Conference on Empirical Methods in Natural Language Processing.
Fraser, K. C., Meltzer, J. A., Rudzicz, F., and Garrard, P. (2015). Linguistic features identify alzheimer's disease in narrative speech. J. Alzheimer\"s Dis. 49, 407–422. doi:10.3233/jad-150520
García-Betances, R. I., Arredondo Waldmeyer, M. T., Fico, G., and Cabrera-Umpiérrez, M. F. (2015). A succinct overview of virtual reality technology use in Alzheimer's disease. Front. Aging Neurosci. 7, 80. doi:10.3389/fnagi.2015.00080
Gauthier, S., Rosa-Neto, P., Morais, J., and Webster, C. (2021). World alzheimer report 2021-journey through the diagnosis of dementia. Alzheimer's Dis. Int. 314.
Granholm, E. L., Panizzon, M. S., Elman, J. A., Jak, A. J., Hauger, R. L., Bondi, M. W., et al. (2017). Pupillary responses as a biomarker of early risk for alzheimer's disease. J. Alzheimer\"s Dis. 56, 1419–1428. doi:10.3233/jad-161078
Gravina, R., Alinia, P., Ghasemzadeh, H., and Fortino, G. (2016). Multi-sensor fusion in body sensor networks: State-of-the-art and research challenges. Inf. Fusion 35, 68–80. doi:10.1016/j.inffus.2016.09.005
Guo, S., Wu, K., Li, C., Wang, H., Zhao, Y., Xi, D., et al. (2020). Integrated contact lens sensor system based on multifunctional ultrathin MoS2 transistors. Matter 4, 969–985. doi:10.1016/j.matt.2020.12.002
Habash, A., Guinn, C., Kline, D., and Patterson, L. (2012). Language analysis of speakers with dementia of the Alzheimer's type.
Haider, F., Garcia, S., and Luz, S. (2020). An assessment of paralinguistic acoustic features for detection of alzheimer's dementia in spontaneous speech. IEEE J. Sel. Top. Signal Process. 14, 272–281. doi:10.1109/jstsp.2019.2955022
Hawkins, K. M., and Sergio, L. E. (2014). Visuomotor impairments in older adults at increased Alzheimer's disease risk. J. Alzheimers Dis. Jad 42, 607–621. doi:10.3233/jad-140051
Helen, R, Rostill, Ramin, Nilforooshan, Amanda, Morgan, Payam, and Barnaghi, Emma (2018). Technology integrated health management for dementia. Br. J. Community Nurs. 23, 502–508. doi:10.12968/bjcn.2018.23.10.502
Hoque, E., Dickerson, R. F., Preum, S. M., Hanson, M., Stankovic, J. A., and Holmes, A (2015). “A comprehensive anomaly detection system for daily in-home activities,” in International Conference on Distributed Computing in Sensor Systems.
Hsu, Y., Chung, P., Wang, W., Pai, M., Wang, C., Lin, C., et al. (2014). Gait and balance analysis for patients with alzheimer's disease using an inertial-sensor-based wearable instrument. IEEE J. Biomed. Health Inf. 18, 1822–1830. doi:10.1109/jbhi.2014.2325413
Huang, W., Luo, S., Yang, D., and Zhang, S. (2021). Applications of smartphone-based near-infrared (NIR) imaging, measurement, and spectroscopy technologies to point-of-care (POC) diagnostics. J. Zhejiang Univ. Sci. B 22, 171–189. doi:10.1631/jzus.b2000388
Hyman, B. T., Phelps, C. H., Beach, T. G., Bigio, E. H., Cairns, N. J., Carrillo, M. C., et al. (2012). National Institute on Aging-Alzheimer's Association guidelines for the neuropathologic assessment of Alzheimer's disease. Alzheimer's. &. Dement. 8, 1–13. doi:10.1016/j.jalz.2011.10.007
Imaoka, Y., Flury, A., and de Bruin, E. (2021). Simultaneous assessment of posture and saccadic eye movement with visual stimuli using head‐mounted display virtual reality technology in healthy older adults. Alzheimer's. Dementia 17. doi:10.1002/alz.053605
Ishii, H., Kimino, K., Aljehani, M., Ohe, N., and Inoue, M. (2016). An early detection system for dementia using the M2 M/IoT platform, Procedia Comput. Sci. 1332–1340. doi:10.1016/j.procs.2016.08.178
Jain, P., Panesar, S. F., Talwar, B. F., and Sah, M. K. (2021). IoT〣ased solutions for smart healthcare. Emerg. Technol. Healthc.
Javdani, H., and Kashanian, H. (2017). Internet of things in medical applications with a service-oriented and security approach: A survey. Health & Technol.
Jonell, P., Moll, B., Hkansson, K., Henter, G. E., Beskow, J., Mikheeva, O., et al. (2021). Multimodal capture of patient behaviour for improved detection of early dementia: Clinical feasibility and preliminary results. Front. Comput. Sci. 3, 642633. doi:10.3389/fcomp.2021.642633
Kang, U. J., Meredith, G. E., and Kang, U. J. (2010). Behavioral models of Parkinson's disease in rodents: A new look at an old problem. Mov. Disord. 21, 1595–1606. doi:10.1002/mds.21010
Karthikeyan, R., Sakthisudhan, K., Sreena, G., Veevasvan, C., and Yuvasri, S. (2021). Industry safety measurement using multi-sensing robot with IIoT. Mater. Today Proc. 45, 8125–8129. doi:10.1016/j.matpr.2021.01.919
Kirste, T., Hoffmeyer, A., Koldrack, P., Bauer, A., and Susanne, S. (2014). Detecting the effect of alzheimer's disease on everyday motion behavior. J. Alzheimer's Dis.
Kirsty, M., Philip, S. J., WestonJennifer, M., and Nicholas, E. (2017). “Visuomotor integration in presymptomatic familial alzheimer's disease,” in Alzheimer's Association International Conference.
Kodintsev, A. N., Kovtun, O. P., and Volkova, L. I. (2020). Saliva biomarkers in diagnostics of early stages of alzheimer's disease. Neurochem. J. 14, 429–438. doi:10.1134/s1819712420040042
Konstantinidis, E. I., Billis, A. S., Paraskevopoulos, I. T., and Bamidis, P. D. (2017). “The interplay between IoT and serious games towards personalised healthcare,” in International Conference on Virtual Worlds & Games for Serious Applications.
Lara, O. D., and Labrador, M. A. (2013). A survey on human activity recognition using wearable sensors. IEEE Commun. Surv. Tutorials 15, 1192–1209. doi:10.1109/surv.2012.110112.00192
Laske, C., Sohrabi, H. R., Frost, S. M., López-de-Ipia, K., Garrard, P., Buscema, M., et al. (2015). Innovative diagnostic tools for early detection of Alzheimer's disease. Alzheimer's. &. Dement. 11, 561–578. doi:10.1016/j.jalz.2014.06.004
Laszlo, T., Ildiko, H., Gabor, G., Veronika, V., Greta, S., Zoltan, B., et al. (2018). A speech recognition-based solution for the automatic detection of mild cognitive impairment from spontaneous speech. Curr. Alzheimer Res. 14, 130–138. doi:10.2174/1567205014666171121114930
Lazarou, I., Karakostas, A., Stavropoulos, T. G., Tsompanidis, T., Meditskos, G., Kompatsiaris, I., et al. (2016). A novel and intelligent home monitoring system for care support of elders with cognitive impairment. J. Alzheimer\"s Dis. 54, 1561–1591. doi:10.3233/jad-160348
Leclair-Visonneau, L., Clairembault, T., Coron, E., Dily, S. L., Vavasseur, F., Dalichampt, M., et al. (2017). REM sleep behavior disorder is related to enteric neuropathology in Parkinson disease. Neurology 89, 1612–1618. doi:10.1212/WNL.0000000000004496
Lima, W. S., Souto, E., El-Khatib, K., Jalali, R., and Gama, J. (2019). Human activity recognition using inertial sensors in a smartphone: An overview. Sensors (Basel), Switz. 19, 3213. doi:10.3390/s19143213
Liu, C., Zhang, B., Chen, W., Liu, W., and Zhang, S. (2021). Current development of wearable sensors based on nanosheets and applications. TrAC Trends Anal. Chem. 143, 116334. doi:10.1016/j.trac.2021.116334
Liu, Y., Nie, L., Liu, L., and RosenblumDavid, S. (2016). From action to activity: Sensor-based activity recognition. Neurocomputing.
Loopez-Larraz, E., Birbaumer, N., and Ramos-Murguialday, A. (2018). “A hybrid EEG-EMG BMI improves the detection of movement intention in cortical stroke patients with complete hand paralysis,” in Conference proceedings Annual International Conference of the IEEE Engineering in Medicine and Biology Society.
López-de-Ipia, K., Jesus-Bernardino, A., Travieso, C. M., Solé-Casals, J., and Harkaitz, E. (2013). On the selection of non-invasive methods based on speech analysis oriented to automatic alzheimer disease diagnosis. Sensors (Basel).
Lopez-De-Ipina, K., Martinez-De-Lizarduy, U., Calvo, P. M., Beitia, B., and Faundez-Zanuy, M. (2017). “Analysis of disfluencies for automatic detection of mild cognitive impartment: A deep learning approach,” in 2017 International Conference and Workshop on Bioinspired Intelligence (IWOBI).
Lu, Z., Harris, T. B., Shiroma, E. J., Leung, J., and Kwok, T. (2018). Patterns of physical activity and sedentary behavior for older adults with alzheimer's disease, mild cognitive impairment, and cognitively normal in Hong Kong. J. Alzheimers Dis. 66, 1453–1462. doi:10.3233/jad-180805
Machado, S. D., Tavares, J., Martins, M. G., Barbosa, J. L. V., González, G. V., and Leithardt, V. R. Q. (2021). Ambient intelligence based on IoT for assisting people with Alzheimer’s disease through context histories. Electronics 10, 1260. doi:10.3390/electronics10111260
Mancioppi, G., Fiorini, L., Sportiello, M. T., and Cavallo, F. (2019). Novel technological solutions for assessment, treatment, and assistance in mild cognitive impairment. Front. Neuroinform. 13, 58. doi:10.3389/fninf.2019.00058
Maselli, M., Fiorini, L., Castro, E., Baldoli, I., and Laschi, C. (2017). “Development and testing of a new cognitive technological tool for episodic memory: A feasibility study, engineering in medicine and biology society (embc),” in 2017 39th Annual International Conference of the IEEE.
Merilahti, J., Viramo, P., and Korhonen, I. (2015). Wearable monitoring of physical functioning and disability changes, circadian rhythms and sleep patterns in nursing home residents. IEEE J. Biomed. Health Inf. 20, 856–864. doi:10.1109/jbhi.2015.2420680
Migliaccio, R., Tangu, D., Sezer, I., and Levy, R. (2020). Cognitive and behavioural inhibition deficits in neurodegenerative dementias. Cortex 131, 265–283. doi:10.1016/j.cortex.2020.08.001
Mitchell, A. G., Rossit, S., Pal, S., Hornberger, M., Warman, A. T. B., Kenning, E. M., et al. (2020). Peripheral reaching in Alzheimer's disease and mild cognitive impairment. Cortex 149, 29–43.
Mohammadi, A., Kargar, M., and Hesami, E. (2018). Using virtual reality to distinguish subjects with multiple- but not single-domain amnestic mild cognitive impairment from normal elderly subjects. Psychogeriatrics 18, 132–142. doi:10.1111/psyg.12301
Montenegro, J., and Argyriou, V. (2016). “Diagnosis of Alzheimer's disease based on virtual environments,” in International Conference on Information.
Montenegro, J. F., and Argyriou, V. (2017). Cognitive evaluation for the diagnosis of alzheimer's disease based on turing test and virtual environments. Physiology Behav. 173, 42–51. doi:10.1016/j.physbeh.2017.01.034
Montenegro, J., Villarini, B., Angelopoulou, A., Kapetanios, E., and Argyriou, V. (2020). A survey of alzheimer's disease early diagnosis methods for cognitive assessment. Sensors 20, 7292. doi:10.3390/s20247292
Nam, U., Lee, K., Ko, H., Lee, J. Y., and Lee, E. C. (2020). Analyzing facial and eye movements to screen for alzheimer's disease. Sensors 20, 5349. doi:10.3390/s20185349
Nardone, R., Langthaler, P. B., Schwenker, K., Kunz, A. B., Sebastianelli, L., Saltuari, L., et al. (2022). Visuomotor integration in early alzheimer's disease: A tms study. J. Neurological Sci. 434, 120129. doi:10.1016/j.jns.2021.120129
Nathan, V., Paul, S., Prioleau, T., Niu, L., Mortazavi, B. J., Cambone, S. A., et al. (2018). A survey on smart homes for aging in place: Toward solutions to the specific needs of the elderly. IEEE Signal Process. Mag. 35, 111–119. doi:10.1109/msp.2018.2846286
Nweke, H., Teh, F., Wah, Y., Mohammed, Al-G., and Alo, A. (2018). Deep learning algorithms for human activity recognition using mobile and wearable sensor networks: State of the art and research challenges. Expert Syst. Appl.
Oyama, A., Takeda, S., Ito, Y., Nakajima, T., Morishita, R., Takeya, Y., et al. (2019). Novel method for rapid assessment of cognitive impairment using high-performance eye-tracking technology. Sci. Rep. 9, 12932. doi:10.1038/s41598-019-49275-x
Petra, M., Signe, T., Petre, L., Joana, M., Ana, M., Eftim, Z., et al. (2018). Technological solutions for older people with alzheimer's disease: Review. Curr. Alzheimer Res. 15, 976–984.
Prince, M., Wimo, A., Guerchet, M., Ali, G. C., and Prina, M. (2015). World alzheimer report the global impact of dementia. An analysis of prevalence, incidence, cost and trends.
Pulido, M., Hernández, J., Ballester, M., González, C., Smékal, Z., and Smekal, Z. (2020). Alzheimer's disease and automatic speech analysis: A review. Expert Syst. Appl. 150, 113213. doi:10.1016/j.eswa.2020.113213
Rashidi, P., and Mihailidis, A. (2013). A survey on ambient-assisted living tools for older adults. IEEE J. Biomed. Health Inf. 17, 579–590. doi:10.1109/jbhi.2012.2234129
Robben, S., Aicha, A. N., and Krose, B. (2016). “Measuring regularity in daily behavior for the purpose of detecting alzheimer,” in 10th EAI International Conference on Pervasive Computing Technologies for Healthcare.
Samrah, A., Anne-Marie, H., de, C., and Peter, J. (2013). Connected speech as a marker of disease progression in autopsy-proven Alzheimer's disease. Brain 136, 3727–3737. doi:10.1093/brain/awt269
Sciarrone, A., Bisio, I., Garibotto, C., Lavagetto, F., and Gambari, G. (2020). “NeuroGlasses: A wearable prototype for early detection of neurological symptoms,” in 2020 5th International Conference on Smart and Sustainable Technologies (SpliTech).
Sheng, Z., Chen, L., Xu, S. C., and Wh, E. (2022). Current Development of Materials science and engineering towards epidermal sensors. Prog. Mater. Sci. 128, 100962. doi:10.1016/j.pmatsci.2022.100962
Shinkawa, K., Kosugi, A., Nishimura, M., Nemoto, M., and Yamada, Y. (2019). Multimodal behavior analysis towards detecting mild cognitive impairment: Preliminary results on gait and speech. Stud. Health Technol. Inf. 264, 343–347. doi:10.3233/SHTI190240
Skaramagkas, V., Giannakakis, G., Ktistakis, E., Manousos, D., Tsiknakis, M., Tachos, N., et al. (2021). Review of eye tracking metrics involved in emotional and cognitive processes. IEEE Rev. Biomed. Eng., 1. doi:10.1109/rbme.2021.3066072
Sprint, G., Cook, D., Fritz, R., and Schmitter-Edgecombe, M. (2016). “Detecting health and behavior change by analyzing smart home sensor data,” in 2016 IEEE International Conference on Smart Computing (SMARTCOMP).
Staal, J., Mattace-Raso, F., Daniels, H., Steen, J., and Pel, J. (2021). To explore the predictive power of visuomotor network dysfunctions in mild cognitive impairment and alzheimer's disease. Front. Neurosci. 15, 654003. doi:10.3389/fnins.2021.654003
Stavropoulos, T. G., Meditskos, G., Kontopoulos, E., and Kompatsiaris, I. (2015). Multi-sensing monitoring and knowledge-driven analysis for dementia assessment. Int. J. E-Health Med. Commun. (IJEHMC) 6, 77–92. doi:10.4018/ijehmc.2015100106
Stone, E. E., and Skubic, M. (2017). Fall detection in homes of older adults using the microsoft Kinect. IEEE J. Biomed. Health Inf. 19, 290–301. doi:10.1109/jbhi.2014.2312180
Sunny, A. I., Zhao, A. B., Li, L., and Sakiliba, S. (2020). Low-cost IoT-based sensor system: A case study on harsh environmental monitoring. Sensors 21, 214. doi:10.3390/s21010214
Tadokoro, K., Yamashita, T., Fukui, Y., Nomura, E., Ohta, Y., Ueno, S., et al. (2021). Early detection of cognitive decline in mild cognitive impairment and Alzheimer's disease with a novel eye tracking test. J. Neurological Sci. 427, 117529. doi:10.1016/j.jns.2021.117529
Tippett, W. J., and Sergio, L. E. (2006). Visuomotor integration is impaired in early stage Alzheimer's disease. Brain Res. 1102, 92–102. doi:10.1016/j.brainres.2006.04.049
Urwyler, P., Stucki, R., Rampa, L., Müri, R., and Nef, T. (2017). Cognitive impairment categorized in community-dwelling older adults with and without dementia using in-home sensors that recognise activities of daily living. Sci. Rep. 7, 42084. doi:10.1038/srep42084
Varatharajan, R., Manogaran, G., Priyan, M. K., and Sundarasekar, R. (2017). Wearable sensor devices for early detection of Alzheimer disease using dynamic time warping algorithm. Clust. Comput. 21, 681–690. doi:10.1007/s10586-017-0977-2
Verheij, S., Muilwijk, D., Pel, J., Cammen, T., Steen, J., and van der Steen, J. (2012). Visuomotor impairment in early-stage alzheimer's disease: Changes in relative timing of eye and hand movements. J. Alzheimer's Dis. JAD 30, 131–143. doi:10.3233/jad-2012-111883
Veronica, B., Eleonora, C., Monica, C., Cristiano, C., Andrea, M., and Cappa, S. F. (2017). Connected speech in neurodegenerative language disorders: A review. Front. Psychol. 8, 269. doi:10.3389/fpsyg.2017.00269
Vippalapalli, V., and Ananthula, S. (2016). “Internet of things (IoT) based smart health care system,” in 2016 International conference on Signal Processing, Communication, Power and Embedded System (SCOPES).
Wams, E. J., Wilcock, G. K., Foster, R. G., and Wulff, K. (2017). Sleep-wake patterns and cognition of older adults with amnestic mild cognitive impairment (aMCI): A comparison with cognitively healthy adults and moderate alzheimer's disease patients. Curr. Alzheimer Res. 14, 1030–1041. doi:10.2174/1567205014666170523095634
Wang, J., Chen, Y., Hao, S., Peng, X., and Hu, L. (2017). Deep learning for sensor-based activity recognition: A survey. Pattern Recognit. Lett.
Wang, L., Gu, T., Tao, X., Chen, H., and Lu, J. (2011). Recognizing multi-user activities using wearable sensors in a smart home. Pervasive Mob. Comput.
Wang, Y., Cang, S., and Yu, H. (2019). A survey on wearable sensor modality centred human activity recognition in health care. Expert Syst. Appl. 137, 167–190. doi:10.1016/j.eswa.2019.04.057
Yamini, G. (2020). Exploring internet of things and artificial intelligence for smart healthcare solutions. Deep Neural Netw. Multimodal Imaging Biomed. Appl., 205–218. doi:10.4018/978-1-7998-3591-2.ch013
Yang, M., Sun, X., Jia, F., Rushworth, A., Dong, X., Zhang, S., et al. (2022). Sensors and sensor fusion methodologies for indoor odometry: A review. Polymers 14, 2019. doi:10.3390/polym14102019
Yu, I., Flury, A., and Bruin, E. (2020). Assessing saccadic eye movements with head-mounted display virtual reality technology. Front. Psychiatry 11, 572938. doi:10.3389/fpsyt.2020.572938
Yu, Z., and Wang, Z. (2020). Human behavior analysis: Sensing and understanding. Human behavior analysis: Sensing and understanding.
Yuan, J., Bian, Y., Cai, X., Huang, J., and Church, K. (2020). Disfluencies and fine-tuning pre-trained language models for detection of alzheimer's disease. Interspeech, 2020.
Yuan, J., Cai, X., Bian, Y., Ye, Z., and Church, K. (2021). Pauses for detection of Alzheimer’s disease. Front. Comput. Sci. 2. doi:10.3389/fcomp.2020.624488
Zhang, S., Liu, C., Zhang, G., Chen, Y., Yang, W., Xia, Q., et al. (2021). Full review: The progress and developing trends of nanosheet-based sensing applications. Coord. Chem. Rev. 433, 213742. doi:10.1016/j.ccr.2020.213742
Zhang, S., Zeng, J., Wang, C., Feng, L., Song, Z., Zhao, W., et al. (2021). The application of wearable glucose sensors in point-of-care testing. Front. Bioeng. Biotechnol. 9, 774210. doi:10.3389/fbioe.2021.774210
Zhang, Y., Wilcockson, T., Kim, K. I., Crawford, T., Gellersen, H., and Sawyer, P. (2016). “Monitoring dementia with automatic eye movements analysis,” in Intelligent decision technologies 2016. Editors I. Czarnowski, A. M. Caballero, R. J. Howlett, and L. C. Jain (Cham: Springer International Publishing), 299–309.
Keywords: biosensors, alzheimer’s disease, diagnosis, behavior detection, elderly
Citation: Sun X, Sun X, Wang Q, Wang X, Feng L, Yang Y, Jing Y, Yang C and Zhang S (2022) Biosensors toward behavior detection in diagnosis of alzheimer’s disease. Front. Bioeng. Biotechnol. 10:1031833. doi: 10.3389/fbioe.2022.1031833
Received: 30 August 2022; Accepted: 03 October 2022;
Published: 19 October 2022.
Edited by:
Muhammad J. A. Shiddiky, Griffith University, AustraliaReviewed by:
Mohammad Ashfaque, Integral University, IndiaDulce Oliveira, University of Porto, Portugal
Copyright © 2022 Sun, Sun, Wang, Wang, Feng, Yang, Jing, Yang and Zhang. This is an open-access article distributed under the terms of the Creative Commons Attribution License (CC BY). The use, distribution or reproduction in other forums is permitted, provided the original author(s) and the copyright owner(s) are credited and that the original publication in this journal is cited, in accordance with accepted academic practice. No use, distribution or reproduction is permitted which does not comply with these terms.
*Correspondence: Sheng Zhang, c3poYW5nMTk4NEB6anUuZWR1LmVkdQ==; Xu Sun, WHUuU3VuQG5vdHRpbmdoYW0uZWR1LmNu