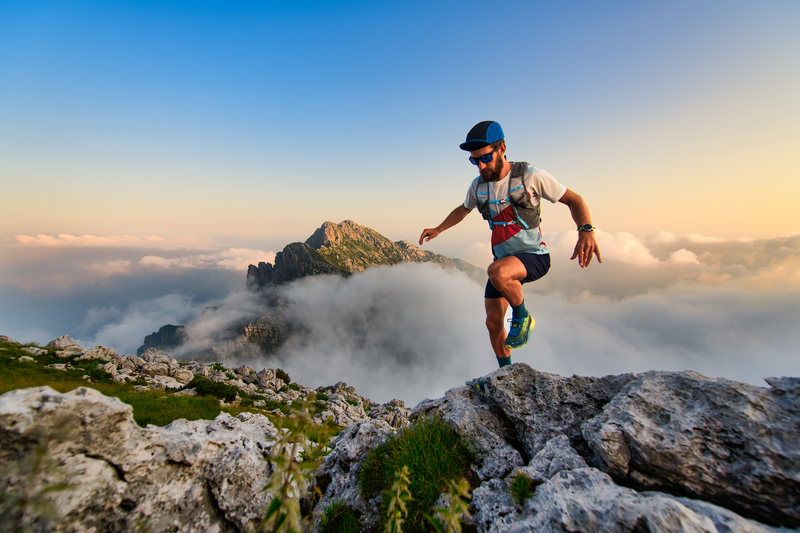
94% of researchers rate our articles as excellent or good
Learn more about the work of our research integrity team to safeguard the quality of each article we publish.
Find out more
EDITORIAL article
Front. Bioeng. Biotechnol. , 06 September 2022
Sec. Cell and Gene Therapy
Volume 10 - 2022 | https://doi.org/10.3389/fbioe.2022.1019929
This article is part of the Research Topic Single Cell Intelligence and Tissue Engineering View all 10 articles
Editorial on the Research Topic
Single cell intelligence and tissue engineering
Single-cell sequencing has emerged as a powerful technology to dissect the heterogeneity of complex biological tissues at genomic, epigenomic, and transcriptomic levels, and has been extensively applied in various biological researches particularly in disease mechanisms and developmental biology (Paik et al., 2020; Gohil et al., 2021; Lei et al., 2021). Since the first single-cell RNA-sequencing (scRNA-seq) publication in 2009 (Tang et al., 2009), single-cell-based technologies have generated massive datasets, offering great opportunities to fully address biomedical problems as well as posing a challenge to computational analysis. At the same time, machine learning methods have been successfully used in processing many kinds of big data, including scRNA-seq data analysis (Petegrosso et al., 2020; Flores et al., 2022).
Nevertheless, more in-depth studies by using elegant methods and strategies to analyze the massive data obtained from the sequencing are still in need to improve our understanding of complex disorders. To make the best use of the single-cell-based data, researchers would first demand efficient and accurate computational pipelines to cluster, annotate cell types, uncover the marker genes and perform functional analysis. Besides, proper study design, including dataset selection and cross-validation, should be conducted to ensure that the evidence is convincing. In this context, this research topic included nine research articles focusing on methods development and clinical application of single-cell technology, giving more examples of data analysis and application in biomedical research.
One of the most common applications of single-cell approaches is to identify and distinguish cell types, and more related computational methods are demanded. Li et al. trained several classifiers and obtained optimal models from in vitro cultured human hepatocyte single-cell RNA data, and identified biomarkers for distinct differentiated hepatic cell types. By uncovering qualitative features for different stages of differentiation of liver cells, this study aimed to provide potential targets for cell transplantation to treat liver diseases. Similarly, Li et al. applied several different machine learning methods to expression profiling data of human pancreatic islet cells at single-cell resolution from both type 2 diabetes (T2D) patients and non-diabetic donors and discovered several T2D-associated genes. These two studies showed the promising applications of machine learning using single-cell expression profiling datasets to understand complex diseases.
Clustering is a critical step in single-cell data analysis to reveal heterogeneities and recognize cell types, requiring efficient and accurate computational algorithms. Tian et al. utilized an enhanced consensus-based clustering model and developed a novel computation method scMelody to cluster cells with single-cell DNA methylation data, such as scME-seq, scBS-seq, scWGBS, scTrio-seq, scNOMe-seq and snmC-seq. By using seven real single-cell methylation datasets and a variety of simulated datasets with different initial settings, scMelody showed better clustering performance and scalability when compared to other existing methods. In the two case studies, scMelody was able to uncover novel cell clusters from human hematopoietic cells and mouse neuron datasets.
Another two studies focused on developing prediction models for diagnosis using machine learning methods. Wang et al. explored mutation signatures with pan-cancer whole exome sequencing data, and constructed a logistic regression model to distinguish cancer types. The proposed model was able to trace the tumor origin for metastatic cancers, and predict cancer types using plasma ctDNA. Wu et al. investigated the microbiota in lung tissue and bronchoalveolar lavage fluid from lung ground-glass opacity (GGO) patients, and constructed a model using 10 genera-based biomarkers to predict GGO.
In addition to the biomarker identification for disease diagnosis, it has been widely recognized that the cellular heterogeneity is prevalent in either tissue or cultured cancer cells. Li et al. first identified a shared sub-cluster cancer stem cells (CSC) using 4 scRNA-seq datasets from upper gastrointestinal cancer (UGIC) patients including head and neck squamous cell carcinoma (HNSCC), esophageal cancer (EC), and gastric cancer (GC), and then compared the specific cells to scRNA-seq datasets from other 6 cancers including glioma, melanoma, osteosarcoma, breast cancer, ovarian cancer and stellate cell cancer to validate the specificity. The UGIC-specific CSC upregulated 33 genes while downregulated 141 genes compared to other tumors analyzed in this study, involving in inflammatory and Wnt pathways. Smit et al. applied FUNseq to spatially profile human breast epithelial cell line MCF10A which is a widely used in vitro model for breast cell transformation at single-cell resolution to decipher intratumor heterogeneity. By comparing the gene expression profiles and cell-cell communication among the cell populations at outer, middle and inner regions in 2D culture, the researchers found that cells at the outermost edge are most invasive, with epithelial-to-mesenchymal transition strongly activated.
While the advent of scRNA-seq technology has enabled profiling gene expression for each cell, analyses of large sample cohorts are still limited. The integration of existing bulk transcriptomic datasets is one of the issues that deserve attention and discussion in data mining. By taking advantage of the power of single-cell approaches and the sample size of bulk methods, two studies provided novel insights for diseases. Shi et al. collected a series of single-cell transcriptome datasets from non-failure hearts, dilated cardiomyopathy and ischemic cardiomyopathy hearts, followed by a comprehensive analysis to find out key genes involved in heart failure. Furthermore, the researchers obtained bulk gene expression datasets to validate the findings from single-cell datasets. On the other hand, Yao et al. developed a practical deconvolution pipeline by constructing a signature gene matrix which was then used to estimate cell proportion from bulk data with CIBERSORTx. Using preeclampsia microarray data, the researchers found that the proportion of trophoblast cells might contribute to the pathogenesis of the disorder.
As discussed above, articles in this special issue covered the fields of single-cell intelligence analysis methods development and computational model construction, providing more options for utilizing clinical datasets. By employing single-cell transcriptome data from patients, the researchers demonstrated the power of single-cell technology in important cell type recognition and key gene identification, broadening the clinical application of the technology. We envision that the use of advanced computational analysis approaches in single-cell datasets will reveal more useful and accurate biomarkers, and greatly benefit the diagnosis and treatments of complex diseases.
All authors listed have made a substantial, direct, and intellectual contribution to the work and approved it for publication.
The authors declare that the research was conducted in the absence of any commercial or financial relationships that could be construed as a potential conflict of interest.
All claims expressed in this article are solely those of the authors and do not necessarily represent those of their affiliated organizations, or those of the publisher, the editors and the reviewers. Any product that may be evaluated in this article, or claim that may be made by its manufacturer, is not guaranteed or endorsed by the publisher.
Flores, M., Liu, Z., Zhang, T., Hasib, M. M., Chiu, Y. C., Ye, Z., et al. (2022). Deep learning tackles single-cell analysis-a survey of deep learning for scRNA-seq analysis. Brief. Bioinform. 23 (1), bbab531. doi:10.1093/bib/bbab531
Gohil, S. H., Iorgulescu, J. B., Braun, D. A., Keskin, D. B., and Livak, K. J. (2021). Applying high-dimensional single-cell technologies to the analysis of cancer immunotherapy. Nat. Rev. Clin. Oncol. 18 (4), 244–256. doi:10.1038/s41571-020-00449-x
Lei, Y., Tang, R., Xu, J., Wang, W., Zhang, B., Liu, J., et al. (2021). Applications of single-cell sequencing in cancer research: progress and perspectives. J. Hematol. Oncol. 14 (1), 91. doi:10.1186/s13045-021-01105-2
Paik, D. T., Cho, S., Tian, L., Chang, H. Y., and Wu, J. C. (2020). Single-cell RNA sequencing in cardiovascular development, disease and medicine. Nat. Rev. Cardiol. 17 (8), 457–473. doi:10.1038/s41569-020-0359-y
Petegrosso, R., Li, Z., and Kuang, R. (2020). Machine learning and statistical methods for clustering single-cell RNA-sequencing data. Brief. Bioinform. 21 (4), 1209–1223. doi:10.1093/bib/bbz063
Keywords: single-cell, computational methods, biomarker identification, clinical application, profiling, machine learning
Citation: Shao J, Jiang Y and Fang Z (2022) Editorial: Single cell intelligence and tissue engineering. Front. Bioeng. Biotechnol. 10:1019929. doi: 10.3389/fbioe.2022.1019929
Received: 15 August 2022; Accepted: 17 August 2022;
Published: 06 September 2022.
Edited and reviewed by:
Andrea Banfi, University of Basel, SwitzerlandCopyright © 2022 Shao, Jiang and Fang. This is an open-access article distributed under the terms of the Creative Commons Attribution License (CC BY). The use, distribution or reproduction in other forums is permitted, provided the original author(s) and the copyright owner(s) are credited and that the original publication in this journal is cited, in accordance with accepted academic practice. No use, distribution or reproduction is permitted which does not comply with these terms.
*Correspondence: Zhaoyuan Fang, emhhb3l1YW5mYW5nQGludGwuemp1LmVkdS5jbg==
Disclaimer: All claims expressed in this article are solely those of the authors and do not necessarily represent those of their affiliated organizations, or those of the publisher, the editors and the reviewers. Any product that may be evaluated in this article or claim that may be made by its manufacturer is not guaranteed or endorsed by the publisher.
Research integrity at Frontiers
Learn more about the work of our research integrity team to safeguard the quality of each article we publish.