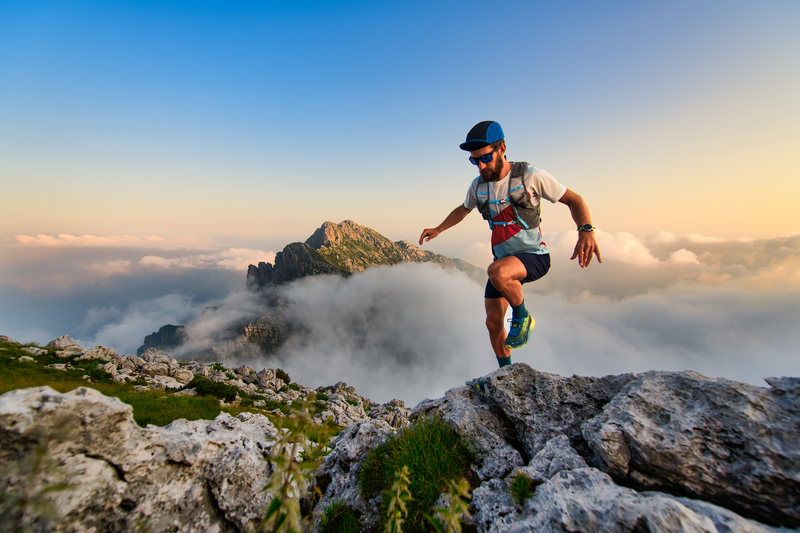
94% of researchers rate our articles as excellent or good
Learn more about the work of our research integrity team to safeguard the quality of each article we publish.
Find out more
ORIGINAL RESEARCH article
Front. Bioeng. Biotechnol. , 07 January 2022
Sec. Synthetic Biology
Volume 9 - 2021 | https://doi.org/10.3389/fbioe.2021.779405
This article is part of the Research Topic Synthetic Biology for the Sustainable Production of Biochemicals in Engineered Microbes View all 17 articles
Microbial production of chemicals is a more sustainable alternative to traditional chemical processes. However, the shift to bioprocess is usually accompanied by a drop in economic feasibility. Co-production of more than one chemical can improve the economy of bioprocesses, enhance carbon utilization and also ensure better exploitation of resources. While a number of tools exist for in silico metabolic engineering, there is a dearth of computational tools that can co-optimize the production of multiple metabolites. In this work, we propose co-FSEOF (co-production using Flux Scanning based on Enforced Objective Flux), an algorithm designed to identify intervention strategies to co-optimize the production of a set of metabolites. Co-FSEOF can be used to identify all pairs of products that can be co-optimized with ease using a single intervention. Beyond this, it can also identify higher-order intervention strategies for a given set of metabolites. We have employed this tool on the genome-scale metabolic models of Escherichia coli and Saccharomyces cerevisiae, and identified intervention targets that can co-optimize the production of pairs of metabolites under both aerobic and anaerobic conditions. Anaerobic conditions were found to support the co-production of a higher number of metabolites when compared to aerobic conditions in both organisms. The proposed computational framework will enhance the ease of study of metabolite co-production and thereby aid the design of better bioprocesses.
Recent years have seen several advances in the usage of bioprocessing to produce a wide range of chemicals (Erickson et al., 2012). Microorganisms can produce diverse and complex products from simple carbon sources. Nevertheless, there are many challenges in designing economically feasible bioprocesses. The advancements in synthetic biology have enabled the metabolic engineering of organisms to improve yield and productivity (Yadav et al., 2019). Various computational strain design algorithms have been developed to identify the genetic manipulations required to over-produce a single product (Burgard et al., 2003; Rocha et al., 2008; Yang et al., 2011). Despite the increase in yield achieved through such rational strain design, the bioprocesses are unable to compete with the traditional chemical processes in many cases (Cai and Bennett, 2011). This is due to two main reasons: 1) the cost of raw materials and 2) the maximum yield achievable for a given product in a given organism and environment is limited by the number of genetic manipulations that can be successfully implemented in a single strain (Silva et al., 2012). The former issue can be reduced by using agricultural waste as feedstock instead of a synthetic nutrient medium. The latter can be overcome by co-producing multiple products in the same bioprocess (da Silva et al., 2014).
Co-production equips us to exploit the system in a better fashion and produce more valuable products from the same raw materials. A high-value, low-volume chemical can be co-produced with a low-value, high-volume product in order to increase the economic feasibility, as in the case of riboflavin and butanol, respectively (Cai and Bennett, 2011). Co-production is essential when a cocktail of metabolites need to be produced together, rather than a single metabolite, as in the case of biofuels and fatty acids (Xin et al., 2018). A mixture of different alcohols or fatty acids of varying chain length need to be co-optimized in such cases. It can also balance carbon metabolism, as in the case of uridine, and acetoin (Fan et al., 2018). High carbon inflow towards uridine causes excess production of acetate, which hampers the growth of the organism. Conversion of acetate to acetoin prevents over-acidification of the nutrient medium and thereby improves growth and uridine production. There are many studies that have successfully achieved co-production of a variety of products with/without genetic manipulation of the organisms. Polyhydroxyalkanoates are a common class of metabolites that are co-produced with other metabolites (Li et al., 2017; Kumar and Kim, 2018; Yadav et al., 2021). Butanol and hydrogen have been co-produced in Clostridium beijerinckii (Zhang et al., 2021), and ethanol and xylitol have been co-produced in Candida tropicalis (Raj and Krishnan, 2020; de Souza Queiroz et al., 2021). The carbon source, nutrient medium, pH etc., are optimized in such cases to improve the yield of metabolites. Metabolic engineering can further expand the number of products that are co-produced and also improve their yield significantly. Multiple metabolites like ethanol, isopropanol, butanol and 2,3- butanediol have been co-produced by optimizing the acetone-butanol-ethanol (ABE) fermentation pathway in Clostridium acetobutylicum (Collas et al., 2012). Nisin and 3-phenyllactic acid, two antimicrobial agents, have been co-produced in Lactococcus lactis through genetic manipulation (Julien-Laferrière et al., 2016). Non-native metabolites can also be co-produced with other metabolites, as in the case of butanol and riboflavin, by engineering the heterologous pathway in C. acetobutylicum (Cai and Bennett, 2011).
Although many strain design algorithms have been successfully employed for metabolically engineering organisms to optimize a single product (Pharkya et al., 2003; Kumelj et al., 2019), few studies have applied it for co-production. The studies listed above only use existing literature and readily apparent deletion targets to achieve co-production. This limits the robustness of the bioprocesses that are designed. There is a lack of algorithms that can be easily applied to study co-production. In this study, we have extended the Flux Scanning based on Enforced Objective Flux (FSEOF) (Choi et al., 2010) algorithm to study co-production. Further, while deletion targets can be obtained for metabolites independently using existing algorithms like OptKnock (Burgard et al., 2003), OptGene (Rocha et al., 2008), there are very few algorithms that can identify amplification targets (Ranganathan et al., 2010). In order to identify amplification targets in addition to knock-out targets for co-optimizing a set of metabolites, we propose a new methodology, co-FSEOF, adapting the FSEOF algorithm. Co-FSEOF has a simple computational framework that can be easily modified, and it also provides the entire set of potential intervention strategies in a single run while many algorithms are sequential, returning one intervention target per run. The utility of the potential intervention strategies obtained was further assessed using Flux Variability Analysis (FVA). We applied co-FSEOF to evaluate all possible pairs of secretory metabolites in Escherichia coli and Saccharomyces cerevisiae. The different pairs of metabolites that can be co-produced through a single reaction deletion or amplification were obtained. This analysis helps us choose favorable pairs of metabolites for which higher-order intervention strategies can be obtained. We have demonstrated this by identifying the amplification targets, knock-out targets and mixed intervention strategies of size up to three to co-optimize the production of isobutanol and succinic acid in S. cerevisiae. Higher-order intervention strategies were able to achieve better yield with very little reduction in growth rate. Overall, our analyses provide an overall picture of the biosynthetic capabilities of an organism, particularly highlighting key interdependencies in metabolism.
FBA is a widely used steady-state constraint-based modelling approach to predict the metabolic capabilities of a variety of organisms (Varma and Palsson, 1994; Kauffman et al., 2003; Orth et al., 2010). The metabolic network of an organism, which comprises all reactions known to occur in the organism, is represented as a stoichiometric matrix
where
FIGURE 1. Framework of co-FSEOF. (A) The GSMM is represented as a stoichiometric matrix, which is used for FBA. (B) The flux through the product is increased in steps, and flux changes through all other reactions are studied. The reactions that have increased flux with an increase in product flux are potential amplification targets. The reactions that have decreased flux are potential deletion targets, while those with unchanged or oscillatory fluxes are excluded. (C) The targets common to products A and B are the potential targets for co-optimization. *The union of all potential targets for products A and B is used for higher-order intervention strategies.
FVA is used to identify the range of fluxes of each reaction that still satisfy the constraints, where two optimization problems are solved for each flux
where
FSEOF (Choi et al., 2010) is a method used to identify potential reaction deletion and amplification targets in metabolic networks by observing the change in the reaction fluxes when the system moves from the wild-type flux of the target product to the theoretical maximum flux of the product (Figure 1B). The maximum biomass
The Genome-Scale Metabolic Models (GSMMs) of E. coli iML1515 and S. cerevisiae iMM904 were obtained from the BiGG models database (http://bigg.ucsd.edu/) (Raj and Krishnan, 2020). The simulations were done with the following constraints on uptakes: −10 mmol/gDW/h glucose and −2 mmol/gDW/h oxygen for aerobic conditions and −10 mmol/gDW/h glucose and zero oxygen uptake for anaerobic conditions. Exchange and transport reactions were removed from the search space for FSEOF to increase the relevance of the results and to reduce the computational time. The potential intervention strategies for all secretory metabolites (metabolites that can be secreted into the medium) in the organism were obtained using FSEOF as described in Section 2.3. All possible pairs of secretory metabolites were examined for co-production by identifying their common intervention strategies which were obtained through FSEOF (Figure 1C). These common deletion or amplification targets constitute the potential intervention strategies for a set of metabolites. The implementation steps of the algorithm are summarized in Figure 2.
The reliability of the potential intervention targets is analyzed by comparing the flux values of biomass and product in the mutant with those of the wild-type organism. The mutant model is obtained by deleting the reaction in case of knock-out targets or by fixing the flux bounds of the amplification target to its theoretical maximum. FVA is performed on this mutant model with biomass as objective to obtain the range of flux values for the products and biomass. Any reaction with more than a 5% increase in the maximum product flux and less than 75% decrease in biomass flux is considered a promising intervention strategy. FVA was performed using fastFVA to reduce the computational time (Gudmundsson and Thiele, 2010).
To obtain higher-order intervention strategies, the potential targets obtained earlier for a given set of metabolites were combined, and all possible combinations of intervention strategies of a certain size (up to three) were evaluated using FVA. The score for each product
where
Metabolic engineering strategies for the co-production of all pairs of secreted metabolites in E. coli and S. cerevisiae were obtained using co-FSEOF as described in Section 2.4. We identified the intervention strategies required to optimize the co-production of metabolites in both aerobic and anaerobic conditions. Anaerobic conditions favor the co-production of more pairs of metabolites when compared to aerobic conditions. The intervention strategy for each pair of metabolites is scored as in Section 2.4. The best intervention strategy can be chosen using the
E. coli is one of the well-studied model organisms and has high-quality GSMMs available. The latest GSMM, iML1515 (Monk et al., 2017), was used in this study, and the co-production of 337 secretory metabolites was studied in both aerobic and anaerobic conditions.
Co-production of all pairs of metabolites was studied in E. coli, using co-FSEOF and FVA as described in Section 2.3, 2.4. Out of
TABLE 1. Intervention strategies for co-production of pairs of metabolites in E. coli under aerobic conditions.
Anaerobic conditions support the co-production of more metabolites when compared to aerobic conditions. More than 1,000 pairs of metabolites can be co-produced, out of which few are listed in Table 2. L-lysine and cadaverine can be co-produced under anaerobic conditions too. But the maximum flux achievable is lower when compared to aerobic conditions. The yield of metabolites like acetate, formate, and hexanoate can be co-optimized by deleting acetaldehyde dehydrogenase or alcohol dehydrogenase. We also found that succinate and lactate can be co-produced by the knock-out of pyruvate formate lyase. The effect of deletion of pflB gene encoding pyruvate formate lyase has been experimentally verified in E. coli for succinate production (Zhang et al., 2009) and lactate production (Utrilla et al., 2009) through separate studies. This shows that there are multiple co-production strategies available in the existing literature that can be easily utilized to design an efficient process.
TABLE 2. Intervention strategies for co-production of pairs of metabolites in E. coli under anaerobic conditions.
Another industrially relevant and well-studied model organism is S. cerevisiae. Though heterologous pathways have not been analyzed in this study, one can easily modify the GSMM and apply co-FSEOF to identify co-production strategies for heterologous metabolites. Since S. cerevisiae is a better candidate for recombinant protein production, it is essential to study co-production in yeast (Bill, 2014). It can also produce more complex metabolites when compared to E. coli and is, therefore, a favorable candidate for bio-production. The latest GSMM iMM904 (Mo et al., 2009) was used, and the ability to optimize the co-production of 164 secretory metabolites was studied in both aerobic and anaerobic conditions.
We found that many industrially important metabolites like ethanol and L-alanine, and 4-aminobutanoate and L-serine can be co-produced in S. cerevisiae under aerobic conditions. We were also able to co-optimize isobutyl alcohol and 2-methyl propanal, which are long-chain alcohols that are used as biofuels. The deletion of pyruvate dehydrogenase increases the production of pyruvate and acetate, as shown in Table 3. Although the deletion of pyruvate dehydrogenase has not been experimentally verified as yet, a similar study has been carried out in E. coli (Moxley and Eiteman, 2021). In this study, it has been shown that the deletion of the genes encoding pyruvate dehydrogenase improves pyruvate production (Moxley and Eiteman, 2021). In addition to pyruvate dehydrogenase, co-FSEOF was able to identify several other amplification targets, which can also improve the production of pyruvate and acetate.
TABLE 3. Intervention strategies for co-production of pairs of metabolites in S. cerevisiae under aerobic conditions.
As in the case of E. coli, anaerobic fermentation enables the co-production of more pairs of metabolites in S. cerevisiae when compared to aerobic fermentation. 2-methyl-1-butanol, which is an important solvent used in the manufacture of pesticides and paints and isobutyl alcohol, which is a biofuel, can be co-produced by the amplification of malic enzyme, as shown in Table 4. Formate, which is used in dyeing and printing, can be co-produced with spermidine, a metabolite increasingly studied for its anti-ageing properties (Minois, 2014), through the amplification of a number of reactions. These strategies not only include readily apparent reactions that are involved in spermidine synthesis like spermidine synthase and adenosylmethionine decarboxylase but also provide some non-intuitive strategies like the amplification of aspartate transaminase or 2-keto-4-methylthiobutyrate transaminase. We also found that the deletion of pyruvate decarboxylase improves the production of succinate, isobutyl alcohol and pyruvate. The effect of deletion of pyruvate decarboxylase has been studied in S. cerevisiae, and the improvement in the production of pyruvate (van Maris et al., 2004) and succinate (Zahoor et al., 2019) has been verified experimentally in separate studies in literature.
TABLE 4. Intervention strategies for co-production of pairs of metabolites in S. cerevisiae under anaerobic conditions.
Higher-order intervention strategies can increase the maximum yield achievable for any product with a little difference in growth rate when compared to single interventions. But they are more cumbersome to identify, as the problem becomes time-consuming and computationally expensive. Instead of identifying higher-order targets for all metabolites in an organism, we have used the previous analysis to explore the metabolic capabilities of the organism and chose one set of metabolites to demonstrate the power of higher-order intervention strategies.
Isobutanol is a long-chain alcohol that is an attractive biofuel (Nanda et al., 2017). Succinic acid is an important metabolite essential for the production of various other products like biodegradable polymers, fatty acids, butyrolactone and tetrahydrofuran (Akhtar et al., 2014). The co-production of isobutanol and succinate has been proposed as a sustainable and economical process by Xu et al. (2018). They have discussed the development of various strains for the production of isobutanol and succinate separately. They emphasize how the co-production of isobutanol and succinate is not only of economic significance, but the high amount of carbon dioxide released from long-chain alcohol fermentation can be used for succinate production, and is hence also of ecological importance. But the article does not discuss any strategy to co-optimize the production of isobutanol and succinate.
Here we identified the higher-order intervention strategies (size up to three) for co-production of isobutanol and succinate in S. cerevisiae in aerobic conditions. More than 3,700 interventions can improve the yield of both the metabolites when compared to the wild-type strain. Table 5 lists a few examples of each type of intervention strategy obtained, which are also represented in Figure 3. Though most of the amplification and deletion targets are integral components of the target product and by-product synthesis respectively, co-FSEOF is also able to find intervention targets in distant pathways like those in pentose phosphate pathway, shikimate pathway, and nucleotide metabolism. This shows the ability of the algorithm to identify non-intuitive targets. Also, a number of the targets predicted here are also found in experimental studies reported in literature. For example, the deletion of pyruvate decarboxylase has been shown to improve the production of isobutanol by Kondo et al. (2012). Zahoor et al. (2019) have shown that both pyruvate decarboxylase deletion and fumarase deletion can increase the production of succinate. This shows the dependability of the results obtained using the algorithm.
TABLE 5. Higher-order intervention strategies for co-production of isobutanol and succinate in S. cerevisiae under aerobic conditions.
FIGURE 3. Pathway diagram for co-production of isobutanol and succinate. The pathways for production of isobutanol and succinate are represented along with the intervention strategies listed in Table 5. Auxiliary pathways that are not directly related to the biosynthesis of target products but contain intervention strategies are shown within dotted rectangles. Co-factors and other small molecules are not depicted for better clarity. Numbers on the arrows point to row numbers in Table 5.
Chemical processes based on fossil fuels are cheaper when compared to bioprocesses, which leads to reluctance in the adoption of sustainable bioprocesses in industries. To improve the economic feasibility of a bioprocess, we can optimize the process variables and/or genetically engineer the microbes (Dzurendova et al., 2020). Even then, in some cases, the bioprocess might be less lucrative when compared to their chemical counterparts (Zhang et al., 2021). In such cases, we can co-produce multiple metabolites to improve the economic feasibility and efficiency of a bioprocess. For example, in the case of biofuels and fatty acids, we need to design processes that can support the production of multiple metabolites of similar nature (Xin et al., 2018). Co-production also ensures better utilization of microbial capabilities, and better balance in the carbon metabolism (Fan et al., 2018). While there are multiple computational tools and algorithms to identify intervention strategies for a single product, there is a lack of readily appliable algorithms for co-production. As a result, almost no co-production study in existing literature was found to use computational algorithms to aid rational strain design. All of the studies rely on previous findings or readily apparent strategies to achieve co-production. This limits the intervention strategies designed.
In this study, we present co-FSEOF, by adapting the effective FSEOF algorithm to study the co-optimization of a set of metabolites. FSEOF is a well-established constraint-based modelling algorithm, which has been used to reliably predict metabolic engineering strategies for a variety of systems (Choi et al., 2010; Boghigian et al., 2012; Badri et al., 2019; Srinivasan et al., 2019). It has a simple and efficient framework and can identify both deletion and amplification targets. Flux Coupling Analysis (FCA) is yet another interesting algorithm that can identify which metabolites can be coupled together. But very few metabolites are innately coupled without interventions. Moreover, it excludes all the reactions that do not carry flux under a given set of conditions from the analysis. This affects the applicability of FCA because these reactions, though not coupled, can have an effect on target production in the presence of other interventions. co-FSEOF is able to identify more combinations of products that can be co-produced and also provides a wider range of intervention strategies for a given set of metabolites.
Using co-FSEOF, we examined the co-production of multiple pairs of metabolites, and both deletion and amplification targets were obtained in E. coli and S. cerevisiae under both aerobic and anaerobic conditions. Anaerobic fermentation enabled the co-production of a higher number of metabolites when compared to aerobic fermentation in both organisms. This could be due to the incomplete respiration in the absence of oxygen that leads to the formation of multiple by-products. Also, S. cerevisiae produces more industrially significant metabolites when compared to E. coli. Some of these proposed intervention strategies have been verified experimentally by other studies in literature, as mentioned in Section 3. This shows the efficacy of the algorithm in furnishing reliable targets. In addition to readily apparent intervention strategies, co-FSEOF also provides non-intuitive intervention strategies that are present in auxiliary biochemical pathways (as discussed in Section 3.2.2, 3.2.3).
The co-optimization analysis for all possible pairs of metabolites in the network is intended to be exploratory in order to give a larger picture of the metabolic capabilities of the organism. This analysis showed that around 200 pairs could be co-optimized in E. coli under aerobic conditions, and around 1,000 pairs of metabolites could be co-optimized in the other cases. An important class of metabolites observed in the analysis are alcohols such as ethanol, isobutanol, and 2,3-butanediol, which can be co-produced using various interventions. Co-optimization can thus enable the efficient production of biofuels (Collas et al., 2012). We can explore the metabolic capabilities of the organism to identify all possible pairs of metabolites that can be co-produced. Following this, important and commercially valuable pairs of metabolites can be further studied to obtain higher-order intervention strategies, as shown in Section 3.2.3. Exploring the higher-order strategies can expand the efficiency of the intervention targets obtained. Since the evaluation of higher-order intervention strategies is laborious and computationally expensive, we have limited the size to a maximum of three manipulations at a time. It not only enhances yield, but also provides alternate routes to achieve a similar yield. The advantageous strategies can be chosen based on the ease of manipulation in an experimental setup in such cases.
The evaluation of the results is carried out using FVA, which ensures the robustness of the targets obtained. While FBA provides one optimal solution from the solution space, FVA gives the entire range of values the flux can take up. This is a significant difference that sets co-FSEOF apart from other existing algorithms like OptKnock (Burgard et al., 2003) and OptReg (Pharkya and Maranas, 2006). Also, the algorithm validates and returns all the intervention strategies in a single run, contrary to the existing algorithms, most of which are sequential and require a separate run for each strategy obtained. The set of intervention strategies validated through FVA can be short-listed for experimental verification using the scores. The
Co-production can open new avenues for the sustainable production of chemicals. Designing bioprocesses for co-production using laboratory experiments alone is cumbersome and can result in sub-optimal strategies. co-FSEOF empowers us to explore and exploit microbial systems in a better fashion. It can be used to computationally study and optimize co-production by identifying intervention strategies for multiple metabolites and thereby improve the efficiency of bioprocesses. It should be noted that the co-optimization analysis was limited to pairs of metabolites to reduce the computational time. Nevertheless, co-FSEOF can be easily extended to co-optimize a more extensive set of metabolites. To conclude, this study can be used to identify various genetic manipulations that can co-optimize a set of products, which might be challenging to achieve through pure experimentation. It provides a novel and critical approach to study co-production computationally. We hope this study will aid the design and development of more sustainable bioprocesses.
Publicly available datasets were analyzed in this study. This data can be found here: http://bigg.ucsd.edu/. All models used in this work and the codes used for our analysis are available at https://github.com/RamanLab/co-FSEOF.
Conceptualization—LR, KR; Funding—KR; Writing—original draft—LR; Writing—review and editing—LR, KR. Signed by all authors as follows: Lavanya Raajaraam (LR), Karthik Raman (KR)* *Corresponding author.
The authors declare that the research was conducted in the absence of any commercial or financial relationships that could be construed as a potential conflict of interest.
All claims expressed in this article are solely those of the authors and do not necessarily represent those of their affiliated organizations, or those of the publisher, the editors and the reviewers. Any product that may be evaluated in this article, or claim that may be made by its manufacturer, is not guaranteed or endorsed by the publisher.
LR acknowledges the HTRA fellowship from the Ministry of Education, Government of India. KR acknowledges support from the Science and Engineering Board (SERB) MATRICS Grant MTR/2020/000490.
Akhtar, J., Idris, A., and Abd Aziz, R. (2014). Recent Advances in Production of Succinic Acid from Lignocellulosic Biomass. Appl. Microbiol. Biotechnol. 98 (3), 987–1000. doi:10.1007/s00253-013-5319-6
Badri, A., Raman, K., and Jayaraman, G. (2019). Uncovering Novel Pathways for Enhancing Hyaluronan Synthesis in Recombinant Lactococcus Lactis: Genome-Scale Metabolic Modeling and Experimental Validation. Processes 7 (6), 343. doi:10.3390/pr7060343
Bill, R. M. (2014). Playing Catch-Up with Escherichia coli: Using Yeast to Increase success Rates in Recombinant Protein Production Experiments. Front. Microbiol. 5, 85. doi:10.3389/fmicb.2014.00085
Boghigian, B. A., Armando, J., Salas, D., and Pfeifer, B. A. (2012). Computational Identification of Gene Over-expression Targets for Metabolic Engineering of Taxadiene Production. Appl. Microbiol. Biotechnol. 93 (5), 2063–2073. doi:10.1007/s00253-011-3725-1
Burgard, A. P., Pharkya, P., and Maranas, C. D. (2003). Optknock: A Bilevel Programming Framework for Identifying Gene Knockout Strategies for Microbial Strain Optimization. Biotechnol. Bioeng. 84 (6), 647–657. doi:10.1002/bit.10803
Cai, X., and Bennett, G. N. (2011). Improving the Clostridium Acetobutylicum Butanol Fermentation by Engineering the Strain for Co-production of Riboflavin. J. Ind. Microbiol. Biotechnol. 38 (8), 1013–1025. doi:10.1007/s10295-010-0875-6
Choi, H. S., Lee, S. Y., Kim, T. Y., and Woo, H. M. (2010). In Silico Identification of Gene Amplification Targets for Improvement of Lycopene Production. Appl. Environ. Microbiol. 76 (10), 3097–3105. doi:10.1128/aem.00115-10
Collas, F., Kuit, W., Clément, B., Marchal, R., López-Contreras, A. M., and Monot, F. (2012). Simultaneous Production of Isopropanol, Butanol, Ethanol and 2,3-butanediol by Clostridium Acetobutylicum ATCC 824 Engineered Strains. AMB Expr. 2 (1), 45. doi:10.1186/2191-0855-2-45
da Silva, T. L., Gouveia, L., and Reis, A. (2014). Integrated Microbial Processes for Biofuels and High Value-Added Products: the Way to Improve the Cost Effectiveness of Biofuel Production. Appl. Microbiol. Biotechnol. 98 (3), 1043–1053. doi:10.1007/s00253-013-5389-5
de Souza Queiroz, S., Jofre, F. M., dos Santos, H. A., Hernández-Pérez, A. F., and de Almeida Felipe, M. D. G. (2021). Xylitol and Ethanol Co-production from Sugarcane Bagasse and Straw Hemicellulosic Hydrolysate Supplemented with Molasses. Biomass Conv. Bioref. 1–10. doi:10.1007/s13399-021-01493-y
Dzurendova, S., Zimmermann, B., Kohler, A., Tafintseva, V., Slany, O., Certik, M., et al. (2020). Microcultivation and FTIR Spectroscopy-Based Screening Revealed a Nutrient-Induced Co-production of High-Value Metabolites in Oleaginous Mucoromycota Fungi. Plos One 15 (6), e0234870. doi:10.1371/journal.pone.0234870
Erickson, B., Nelson, , and Winters, P. (2012). Perspective on Opportunities in Industrial Biotechnology in Renewable Chemicals. Biotechnol. J. 7 (2), 176–185. doi:10.1002/biot.201100069
Fan, X., Wu, H., Jia, Z., Li, G., Li, Q., Chen, N., et al. (2018). Metabolic Engineering of Bacillus Subtilis for the Co-production of Uridine and Acetoin. Appl. Microbiol. Biotechnol. 102 (20), 8753–8762. doi:10.1007/s00253-018-9316-7
Gudmundsson, S., and Thiele, I. (2010). Computationally Efficient Flux Variability Analysis. BMC Bioinformatics 11 (1), 489. doi:10.1186/1471-2105-11-489
Heirendt, L., Arreckx, S., Pfau, T., Mendoza, S. N., Richelle, A., Heinken, A., et al. (2019). Creation and Analysis of Biochemical Constraint-Based Models Using the COBRA Toolbox v.3.0. Nat. Protoc. 14 (3), 639–702. doi:10.1038/s41596-018-0098-2
Julien-Laferrière, A., Bulteau, L., Parrot, D., Marchetti-Spaccamela, A., Stougie, L., Vinga, S., et al. (2016). A Combinatorial Algorithm for Microbial Consortia Synthetic Design. Sci. Rep. 6 (1), 29182. doi:10.1038/srep29182
Kang, Z., Gao, C., Wang, Q., Liu, H., and Qi, Q. (2010). A Novel Strategy for Succinate and Polyhydroxybutyrate Co-production in Escherichia coli. Bioresour. Technol. 101 (19), 7675–7678. doi:10.1016/j.biortech.2010.04.084
Kauffman, K. J., Prakash, P., and Edwards, J. S. (2003). Advances in Flux Balance Analysis. Curr. Opin. Biotechnol. 14 (5), 491–496. doi:10.1016/j.copbio.2003.08.001
Kondo, T., Tezuka, H., Ishii, J., Matsuda, F., Ogino, C., and Kondo, A. (2012). Genetic Engineering to Enhance the Ehrlich Pathway and Alter Carbon Flux for Increased Isobutanol Production from Glucose by Saccharomyces cerevisiae. J. Biotechnol. 159 (1), 32–37. doi:10.1016/j.jbiotec.2012.01.022
Kumar, P., and Kim, B. S. (2018). Valorization of Polyhydroxyalkanoates Production Process by Co-synthesis of Value-Added Products. Bioresour. Technol. 269, 544–556. doi:10.1016/j.biortech.2018.08.120
Kumelj, T., Sulheim, S., Wentzel, A., and Almaas, E. (2019). Predicting Strain Engineering Strategies Using iKS1317: A Genome-Scale Metabolic Model of Streptomyces Coelicolor. Biotechnol. J. 14 (4), e1800180. doi:10.1002/biot.201800180
Li, T., Elhadi, D., and Chen, G.-Q. (2017). Co-Production of Microbial Polyhydroxyalkanoates with Other Chemicals. Metab. Eng. 43, 29–36. doi:10.1016/j.ymben.2017.07.007
Liang, C. Y., Xu, J. L., Xu, H. J., Qi, W., Zhang, Y., Luo, W., et al. (2019). Gene Cloning and Characterization of an Organic Solvent-Stimulated β-glucosidase and its Application for the Co-production of Ethanol and Succinic Acid. Cellulose 26 (15), 8237–8248. doi:10.1007/s10570-019-02477-y
Minois, N. (2014). Molecular Basis of the 'Anti-Aging' Effect of Spermidine and Other Natural Polyamines - A Mini-Review. Gerontology 60 (4), 319–326. doi:10.1159/000356748
Mo, M. L., Palsson, B. Ø., and Herrgård, M. J. (2009). Connecting Extracellular Metabolomic Measurements to Intracellular Flux States in Yeast. BMC Syst. Biol. 3 (1), 37. doi:10.1186/1752-0509-3-37
Monk, J. M., Lloyd, C. J., Brunk, E., Mih, N., Sastry, A., King, Z., et al. (2017). iML1515, a Knowledgebase that Computes Escherichia coli Traits. Nat. Biotechnol. 35 (10), 904–908. doi:10.1038/nbt.3956
Moxley, W. C., and Eiteman, M. A. (2021). Pyruvate Production by Escherichia coli by Use of Pyruvate Dehydrogenase Variants. Appl. Environ. Microbiol. 87 (13), e00487–21. doi:10.1128/aem.00487-21
Nanda, S., Golemi-Kotra, D., McDermott, J. C., Dalai, A. K., Gökalp, I., and Kozinski, J. A. (2017). Fermentative Production of Butanol: Perspectives on Synthetic Biology. New Biotechnol. 37, 210–221. doi:10.1016/j.nbt.2017.02.006
Orth, J. D., Thiele, I., and Palsson, B. Ø. (2010). What Is Flux Balance Analysis? Nat. Biotechnol. 28 (3), 245–248. doi:10.1038/nbt.1614
Pharkya, P., and Maranas, C. D. (2006). An Optimization Framework for Identifying Reaction Activation/inhibition or Elimination Candidates for Overproduction in Microbial Systems. Metab. Eng. 8 (1), 1–13. doi:10.1016/j.ymben.2005.08.003
Pharkya, P., Burgard, A. P., and Maranas, C. D. (2003). Exploring the Overproduction of Amino Acids Using the Bilevel Optimization Framework OptKnock. Biotechnol. Bioeng. 84 (7), 887–899. doi:10.1002/bit.10857
Raj, K., and Krishnan, C. (2020). Improved Co-production of Ethanol and Xylitol from Low-Temperature Aqueous Ammonia Pretreated Sugarcane Bagasse Using Two-Stage High Solids Enzymatic Hydrolysis and Candida tropicalis. Renew. Energ. 153, 392–403. doi:10.1016/j.renene.2020.02.042
Ranganathan, S., Suthers, P. F., and Maranas, C. D. (2010). OptForce: An Optimization Procedure for Identifying All Genetic Manipulations Leading to Targeted Overproductions. Plos Comput. Biol. 6 (4), e1000744. doi:10.1371/journal.pcbi.1000744
Rocha, I., Maia, P., Rocha, M., and Ferreira, E. C. (2008). OptGene – A Framework for in Silico Metabolic Engineering in 10th International Conference on Chemical and Biological Engineering (Portugal: University of Minho), 218–219.
Silva, F., Queiroz, J. A., and Domingues, F. C. (2012). Evaluating Metabolic Stress and Plasmid Stability in Plasmid DNA Production by Escherichia coli. Biotechnol. Adv. 30 (3), 691–708. doi:10.1016/j.biotechadv.2011.12.005
Srinivasan, A., S, V., Raman, K., and Srivastava, S. (2019). Rational Metabolic Engineering for Enhanced Alpha-Tocopherol Production in Helianthus Annuus Cell Culture. Biochem. Eng. J. 151, 107256. doi:10.1016/j.bej.2019.107256
Utrilla, J., Gosset, G., and Martinez, A. (2009). ATP Limitation in a Pyruvate Formate Lyase Mutant of Escherichia coli MG1655 Increases Glycolytic Flux to D-Lactate. J. Ind. Microbiol. Biotechnol. 36 (8), 1057–1062. doi:10.1007/s10295-009-0589-9
van Maris, A. J. A., Geertman, J.-M. A., Vermeulen, A., Groothuizen, M. K., Winkler, A. A., Piper, M. D. W., et al. (2004). Directed Evolution of Pyruvate Decarboxylase-Negative Saccharomyces cerevisiae, Yielding a C 2 -Independent, Glucose-Tolerant, and Pyruvate-Hyperproducing Yeast. Appl. Environ. Microbiol. 70 (1), 159–166. doi:10.1128/aem.70.1.159-166.2004
Varma, A., and Palsson, B. O. (1994). Metabolic Flux Balancing: Basic Concepts, Scientific and Practical Use. Nat. Biotechnol. 12 (10), 994–998. doi:10.1038/nbt1094-994
Xin, F., Dong, W., Jiang, Y., Ma, J., Zhang, W., Wu, H., et al. (2018). Recent Advances on Conversion and Co-production of Acetone-Butanol-Ethanol into High Value-Added Bioproducts. Crit. Rev. Biotechnol. 38 (4), 529–540. doi:10.1080/07388551.2017.1376309
Xu, C., Zhang, J., Zhang, Y., Guo, Y., Xu, H., and Xu, J. (2018). Long Chain Alcohol and Succinic Acid Co-production Process Based on Full Utilization of Lignocellulosic Materials. Curr. Opin. Green Sustain. Chem. 14, 1–9. doi:10.1016/j.cogsc.2018.04.012
Xu, J.-Z., Ruan, H.-Z., Liu, L.-M., Wang, L.-P., and Zhang, W.-G. (2019). Overexpression of Thermostable Meso-Diaminopimelate Dehydrogenase to Redirect Diaminopimelate Pathway for Increasing L-Lysine Production in Escherichia coli. Sci. Rep. 9 (1), 2423. doi:10.1038/s41598-018-37974-w
Yadav, A. N., Kour, D., Rana, K. L., Yadav, N., Singh, B., Chauhan, V. S., et al. (2019). “Metabolic Engineering to Synthetic Biology of Secondary Metabolites Production,” in New and Future Developments in Microbial Biotechnology and Bioengineering. Editors VK Gupta, and A Pandey, 279–320. doi:10.1016/b978-0-444-63504-4.00020-7
Yadav, B., Talan, A., Tyagi, R. D., and Drogui, P. (2021). Concomitant Production of Value-Added Products with Polyhydroxyalkanoate (PHA) Synthesis: A Review. Bioresour. Technol. 337, 125419. doi:10.1016/j.biortech.2021.125419
Yang, L., Cluett, W. R., and MahadevanEMILiO, R. (2011). EMILiO: A Fast Algorithm for Genome-Scale Strain Design. Metab. Eng. 13 (3), 272–281. doi:10.1016/j.ymben.2011.03.002
Zahoor, A., Küttner, F. T. F., Blank, L. M., and Ebert, B. E. (2019). Evaluation of Pyruvate decarboxylase‐negativeSaccharomyces Cerevisiaestrains for the Production of Succinic Acid. Eng. Life Sci. 19 (10), 711–720. doi:10.1002/elsc.201900080
Zhang, X., Jantama, K., Shanmugam, K. T., and Ingram, L. O. (2009). Reengineering Escherichia coli for Succinate Production in Mineral Salts Medium. Appl. Environ. Microbiol. 75 (24), 7807–7813. doi:10.1128/aem.01758-09
Keywords: metabolic modelling, genome-scale models, bioproduction, concomitant production, co-synthesis, constraint-based modelling
Citation: Raajaraam L and Raman K (2022) A Computational Framework to Identify Metabolic Engineering Strategies for the Co-Production of Metabolites. Front. Bioeng. Biotechnol. 9:779405. doi: 10.3389/fbioe.2021.779405
Received: 18 September 2021; Accepted: 02 December 2021;
Published: 07 January 2022.
Edited by:
Hua Ling, National University of Singapore, SingaporeReviewed by:
Meiyappan Lakshmanan, Bioprocessing Technology Institute (A∗STAR), SingaporeCopyright © 2022 Raajaraam and Raman. This is an open-access article distributed under the terms of the Creative Commons Attribution License (CC BY). The use, distribution or reproduction in other forums is permitted, provided the original author(s) and the copyright owner(s) are credited and that the original publication in this journal is cited, in accordance with accepted academic practice. No use, distribution or reproduction is permitted which does not comply with these terms.
*Correspondence: Karthik Raman, a3JhbWFuQGlpdG0uYWMuaW4=
Disclaimer: All claims expressed in this article are solely those of the authors and do not necessarily represent those of their affiliated organizations, or those of the publisher, the editors and the reviewers. Any product that may be evaluated in this article or claim that may be made by its manufacturer is not guaranteed or endorsed by the publisher.
Research integrity at Frontiers
Learn more about the work of our research integrity team to safeguard the quality of each article we publish.