- 1Molecular and Cellular Neurobiotechnology, Institute for Bioengineering of Catalonia, Barcelona Institute of Science and Technology, Barcelona, Spain
- 2Department of Cell Biology, Physiology and Immunology, Faculty of Biology, University of Barcelona, Barcelona, Spain
- 3Center for Networked Biomedical Research on Neurodegenerative Diseases (Ciberned), Barcelona, Spain
- 4Institute of Neuroscience, University of Barcelona, Barcelona, Spain
- 5Department of Pathology and Experimental Therapeutics, University of Barcelona, Barcelona, Spain
- 6Bellvitge University Hospital, Hospitalet de Llobregat, Barcelona, Spain
- 7Bellvitge Biomedical Research Institute (IDIBELL), Hospitalet de Llobregat, Barcelona, Spain
Human aging is accompanied by a relevant increase in age-associated chronic pathologies, including neurodegenerative and metabolic diseases. The appearance and evolution of numerous neurodegenerative diseases is paralleled by the appearance of intracellular and extracellular accumulation of misfolded proteins in affected brains. In addition, recent evidence suggests that most of these amyloid proteins can behave and propagate among neural cells similarly to infective prions. In order to improve understanding of the seeding and spreading processes of these “prion-like” amyloids, microfluidics and 3D lab-on-chip approaches have been developed as highly valuable tools. These techniques allow us to monitor changes in cellular and molecular processes responsible for amyloid seeding and cell spreading and their parallel effects in neural physiology. Their compatibility with new optical and biochemical techniques and their relative availability have increased interest in them and in their use in numerous laboratories. In addition, recent advances in stem cell research in combination with microfluidic platforms have opened new humanized in vitro models for myriad neurodegenerative diseases affecting different cellular targets of the vascular, muscular, and nervous systems, and glial cells. These new platforms help reduce the use of animal experimentation. They are more reproducible and represent a potential alternative to classical approaches to understanding neurodegeneration. In this review, we summarize recent progress in neurobiological research in “prion-like” protein using microfluidic and 3D lab-on-chip approaches. These approaches are driven by various fields, including chemistry, biochemistry, and cell biology, and they serve to facilitate the development of more precise human brain models for basic mechanistic studies of cell-to-cell interactions and drug discovery.
Introduction
The formation of β-sheet enriched misfolded protein aggregates (also termed amyloids) via self-assembly of proteins or polypeptides, with intrinsic or induced amyloidogenic properties, is the hallmark of numerous protein misfolding diseases (PMD) affecting humans (Greenfield et al., 2008). These include neural diseases such as Alzheimer’s disease (AD), Parkinson’s disease (PD), multiple system atrophy (MSA), amyotrophic lateral sclerosis (ALS), and prionopathies [i.e., Creutzfeldt–Jakob disease (CJD)], as well as non-neural diseases such as pancreatic amyloidosis, systemic amyloidosis, and type II diabetes, among others (see Skinner et al., 2005; Ramirez-Alvarado et al., 2010; Sigurdsson et al., 2012; Otzen, 2013 for references). For several years, considerable effort has been made to uncover the molecular basis of the aggregation process and the different strains of specific amyloids with aggregative and infective properties. As a recent example of this interest, a published study of R. Nonno’s lab reveals that a small autocatalytic, but non-fibrillar, 7 kDa fragment of the pathogenic prion currently observed in Gerstmann-Sträussler-Scheinker disease (GSS) patients is also infective (Vanni et al., 2020). In fact, all these studies share as their goal the development of appropriate and specific methods to inhibit their aggregation in real biosafety and clinical scenarios (Giles et al., 2017).
Focusing on neuronal PMDs, several assays for amyloid detection, aggregation, and amplification have been developed in recent years to address the demand for reliable and sensitive in vitro detection of the various amyloid species (from oligomers to fibrils) in human samples. These procedures are mainly based on detecting the presence or monitoring the self-aggregation process of pathogenic amyloids using in situ or ex situ approaches. In situ assays are straightforward but they require direct introduction of probe molecules into the aggregation assay and in some cases interference in the process can be observed. Interference of the aggregation process by probe molecules can be avoided in ex situ assays, where small samples of an aggregating protein solution are extracted and diluted at specified time points into a buffered solution containing an appropriate dye molecule. Another alternative is to promote the amplification of very small quantities of the active pathological species up to significantly detectable levels, as the polymerase chain reaction (PCR) does for nucleotides. In the first group, available techniques range from classic fluorometric assays [i.e., Thioflavin T (ThT) (Saeed and Fine, 1967), Congo red (Yakupova et al., 2019)], and benzofuranone (for α-synuclein (Lengyel-Zhand et al., 2020), to new biosensor-based methods.
In addition, alternative classical optical detection methods for ThT staining (the most used fluorometric method) include the use of colorimetry techniques using, among others, gold nanoparticles (i.e., Zhou et al., 2015) or FRET methods on CdTe quantum dots (Xia et al., 2016).
Of these, numerous electrochemical biosensing methods based on antibodies {i.e., (Prabhulkar et al., 2012; Li et al., 2016), aptamers (Ylera et al., 2002; Zhao et al., 2020), coupling peptides (Li et al., 2013), and peptides with affinity regions [e.g., for β-amyloid the PrP(95-110)] (Rushworth et al., 2014)} were developed as reliable alternatives for conventional amyloid detection (see also Kaushik et al., 2016; Zhang et al., 2020 for review). These offer advantages compared to conventional methods due to their portability, relatively easy handling, and greater sensitivity. Among the huge number of emerging strategies, of particular interest is the application of new materials such as graphene oxide as a reliable alternative to fluorometric assays (e.g., ThT) to detect β-amyloid in label-free systems (e.g., Huang H. et al., 2017). Their use allows researcher to achieve simultaneous detection of oligomeric and fibrillar structures in the same sample (a challenging problem in classical fluorometric assays) and in other higher sensitivity studies, or the recently developed electrochemical determination of β-amyloid oligomers using a graphene and reduced graphene oxide dual-layer biosensor (Sethi et al., 2020). These are just some examples of the tremendous progress that has been made in the development of sensors for detection of amyloids (mainly β-amyloid). Readers interested in the emerging biosensors and electrochemical detection of amyloids may obtain more details in recent reviews (e.g., Serafin et al., 2020; Zhang et al., 2020).
A second strategy is amplification of the pathogenic amyloid in the presence of its monomeric counterparts using biochemical reactions, such as the protein misfolding cyclic amplification (PMCA) method (Saborio et al., 2001; Soto et al., 2002), real-time quaking-induced conversion (RT-QuIC) assay (Wilham et al., 2010; Sano et al., 2018), and ASA assay (Colby et al., 2007). These methods have evolved and have been adapted for the reliable detection of the presence and aggregative stage of various amyloids (i.e., Orru et al., 2017; Saijo et al., 2019) in different biological samples (i.e., Fairfoul et al., 2016; Haley et al., 2016; Bongianni et al., 2017). In parallel to these biochemical methods, other engineering laboratories have developed ultrasensitive methods based on graphene oxide and entropy-driven strand displacement reaction (ESDR) with LOD of 20 pM (Zhou et al., 2018), or graphene field-effect transistor (G-FET) for β-amyloid formation (Kuo et al., 2018). Unfortunately, these methods are not currently used in patient-derived studies.
Neurodegenerative progression in PMDs runs in parallel with disease-specific characteristic intra- or extra-cellular accumulation of misfolded amyloids. In fact, cellular, molecular, biophysical, and biochemical studies have revealed that most PMDs are progressive disorders, and that amyloid-associated pathologies spread from diseased to healthy cells affecting different brain areas in a sequential basis (see Costanzo and Zurzolo, 2013; Goedert et al., 2017; Holmes and Diamond, 2017; Del Rio et al., 2018; Vilette et al., 2018; Meisl et al., 2020; Peng et al., 2020 for reviews). The spatiotemporal progression of these diseases seems to correlate, with some controversy (see below), with the brain propagation of the amyloid-associated neuropathology between anatomical pathways specific to each disorder, suggesting a cell-to-cell spreading of the disease (Saper et al., 1987; Bertrand et al., 2004; Braak and Del Tredici, 2009; Costanzo and Zurzolo, 2013; Goedert et al., 2017; Peng et al., 2020; Figure 1). However, this “connectome” view of the seeding and progression of the amyloid accumulation between connected areas is under debate for some PMDs [i.e., PD, please see Surmeier et al. (2017) for review]. In PD, when comparing the appearance and progression of the Lewy pathology in affected individuals with experimental mouse models of the disease, it seems to correlate better with cell- or region-autonomous mechanisms rather than connectivity (Surmeier et al., 2017). Nevertheless, several routes of inter-cellular amyloid spreading have been proposed such us membrane binding, receptor-mediated, and non-mediated endocytosis (i.e., Holmes et al., 2013; Mao et al., 2016; Aulic et al., 2017; Urrea et al., 2018; Rauch et al., 2020), multivesicular bodies (i.e., exosomes) (Sardar Sinha et al., 2018; Perez et al., 2019; Winston et al., 2019), or tunneling nanotubes (TNTs) (Costanzo and Zurzolo, 2013; Abounit et al., 2016; Zeinabad et al., 2016; Peng et al., 2020; Figure 1). Understanding the intercellular formation and transmission of these amyloids, also termed “pathogenic seeds” in some studies, has become a challenging issue in recent years (i.e., Vilette et al., 2018; Erana, 2019; Peng et al., 2020). Relevantly, the participation of neurons, astroglia, and oligodendrocytes also displaying insoluble amyloid inclusions in particular neurodegenerative diseases during seeding and spreading is still unknown and warrants further study (i.e., Kovacs et al., 2017; Ferrer, 2018).
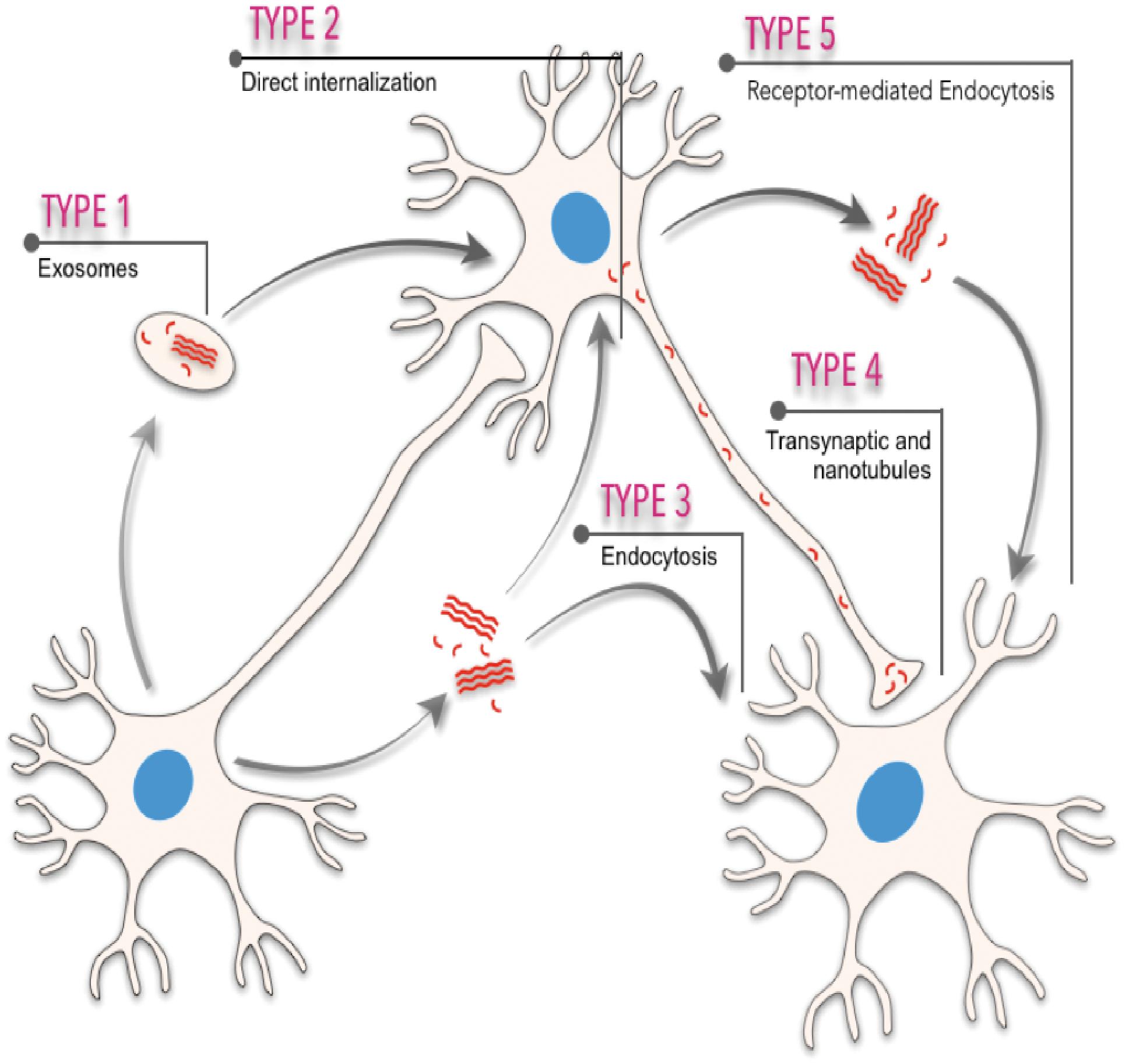
Figure 1. Scheme illustrating the different spreading pathways of a “hypothetical” pathogenic seed between different cells. Five different means of transmission are illustrated: (i) from exosomes or multivesicular bodies, (ii) direct internalization by interacting with plasma membranes, (iii) endocytosis or micropinocytosis processes, (iv) transsynaptic transmission and tunneling nanotubules, and (v) receptor-mediated uptake of the amyloid.
In addition, considering the evidence demonstrating the ability of these amyloids to disseminate protein misfolding as “pathologic seeds” from sick to healthy cells, a “prion-like” or “prionoid” hypothesis has been proposed (Ashe and Aguzzi, 2013; Aguzzi and Lakkaraju, 2016; Collinge, 2016; Woerman et al., 2018). Indeed, cell-spread of amyloid seeds can act as a self-propagating template disrupting cell viability and leading to both the death of recipient cells and the progression of the neurodegenerative disorder (Ashe and Aguzzi, 2013; Aguzzi and Lakkaraju, 2016; Collinge, 2016; Holmes and Diamond, 2017). However, some criticisms appeared with this terminology [i.e., for tau (Polanco and Gotz, 2015), β-amyloid (Watts and Prusiner, 2018), and MSA-derived α-synuclein (Woerman et al., 2020)]. We suggest to the reader interested in this topic examining the reports of Castilla’s and Requena’s laboratories (i.e., Castilla and Requena, 2015; Erana et al., 2017) and the recent review of Wells et al. (2019). In fact, prion diseases (e.g., CJD) are the only neurodegenerative disorders showing an infectious transmissible protein species, the pathogenic prion, capable of recapitulating a clinical disease (e.g., Prusiner, 1998a,b; Moore et al., 2009). Moreover, the prion strain also plays a crucial role in dictating the type (i.e., punctate vs. plaques) and anatomical distribution of the lesions of pathogenic PrP deposition in affected brains (see for example Fraser and Dickinson, 1973; Lasmezas et al., 1996; Scialo et al., 2019 for a recent review).
In this challenging scenario, microfluidics and lab-on-chip (LOC) technologies have emerged in the last 15–20 years as a plausible strategy to monitor some aspects of amyloid aggregation and amyloid-biological interactions, as well as a being a valuable, reproducible tool to analyze cell-to-cell seeding and spreading of pathogenic seeds from “prion-like” or “prionoid” proteins (Aguzzi and Rajendran, 2009; Scheckel and Aguzzi, 2018). In fact, the use of microfluidics and LOC technology has extended from technical fields (i.e., engineering, physics, and electronics) into a wide variety of biomedical scientific fields including pharmacology, oncology, immunology, and neurobiology.
An Overview of Microfluidics and Lab-on-Chip (LOC) Platforms
Microfluidics platforms are devices containing microchannels with a height/width scale between 100 nm and 100 μm (Tabeling, 2005; Folch i Folch, 2013). Although for neuroscience, pioneer microfluidic studies were developed by Campenot (1977, 1982), (see also Taylor and Jeon, 2010; Neto et al., 2016 for reviews), from the electronics point of view, its origins begin in parallel to microelectronics by micro-fabrication techniques from the semiconductor industry in the 1970s and 1980s (please see Tabeling, 2005). After this, in the 1990s the concept of μTAS (miniaturized total chemical analysis systems) was developed to describe a microfluidic platform that could carry out all the functions required for analysis of an analyte: sample preparation including transport, and chemical reactions as well as selective analyte detection. From this, in terms of manufacture, terms like MEMs (microelectromechanical systems) and LOC devices emerged in the microelectronics and biomedical fields (Folch i Folch, 2013). As indicated in the introduction, LOC might include biosensors/electrochemical/optochemical sensors that have been developed in recent years. In fact, there is a parallel development of the complexity of the LOC devices (i.e., in terms of manufacture) directed to amyloid screening and monitoring with the development of new detection methods.
Today, although with some exceptions (Kamande et al., 2019), the vast majority of microfluidic LOC devices utilized in neurobiology are generated using the silicone elastomer polydimethyl-siloxane (PDMS) (McDonald et al., 2000; McDonald and Whitesides, 2002; Ng et al., 2002; Kuncova-Kallio and Kallio, 2006). PDMS is an economically cheap, biocompatible, soft, flexible, and easy-to-handle elastomer, with an index of refraction of 1.43 (similar to a glass coverslip ≈1.52), and good gas diffusion. Relevant electrical and thermal isolation are highly suitable for biological research (i.e., cell culture) and fluorescent microscopy (McDonald et al., 2000; McDonald and Whitesides, 2002; Ng et al., 2002; Kuncova-Kallio and Kallio, 2006). This new PDMS-based microfluidic application using the elastomer directing microfluidic, micropatterning, and microfabrication technologies to neurobiological experiments was developed 2003–2006 by Jeon’s lab (Taylor et al., 2003, 2005; Rhee et al., 2005; Park et al., 2006) with great development in recent years (see Neto et al., 2016 for review). These pioneer studies follow the experiments of Campenot (1977, 1982) in generating a simple, reproducible, and tunable culture platform for compartmentalized neural growth and differentiation. Most of the experiments designed to explore the cell dynamics of different amyloids are currently based on their pioneer microfluidic designs.
PDMS-based manufacture of LOC devices is mainly based on “soft-lithography” protocols (McDonald et al., 2000; Whitesides et al., 2001). Today, PDMS manufacture has evolved to multilayer LOCs as well as more complex platforms. In fact, due to their physical characteristics, different PDMS microfluidic chips can be bounded using plasma reactions (Tran H. T. et al., 2014) to generate multilayered LOCs (i.e., Saar et al., 2016). In fact, some of these multilayered LOCs are used today to analyze amyloid formation ex situ (i.e., Saar et al., 2016). Additional methods ranging from hot-embossing lithography (Jeon et al., 2011) to 3D printing methods (Au et al., 2016) have been developed to generate new LOC devices. Readers may obtain more information about LOC platform manufacturing strategies in reference microfabrication books (Minteer, 2006; Herold and Rasooly, 2009; Lee and Sundararajan, 2010; Folch i Folch, 2013). In this review we will focus on the PDMS-derived LOC devices with the greatest impact on the study of amyloids associated with neurodegeneration.
Why Use LOC Devices and Microfluidics in Amyloid-Related Studies?
Microfluidics and LOC devices hold a number of advantages for amyloid-related research at different levels: (1) most LOC devices reduce the use of reagents due to small reaction volumes; (2) some LOC devices can produce a large number of independent but repetitive compartments to allow protein-protein interactions (i.e., micro/nanodroplets, see below); (3) in most LOC platforms we have complete control over spatial and temporal parameters in the reaction conditions; (4) most LOC devices are compatible with several detection methods (i.e., optical or electrical sensors); and (5) some LOC devices allow for the differential culture of different cell types (i.e., neurons, astroglia, oligodendroglia, microglia, etc.) in the same platform in an interactive way – for example in: (i) microfluidically isolated chambers, (ii) cells growing on molecular or chemical gradients, (iii) cells growing under flows, and (iv) cells growing inside 3D structures [cellular aggregation (i.e., neurospheres, neurospheroids, and organoids) or biomaterial-derived scaffolds].
In the large bibliography focused on microfluidics with more than 3000 published reviews reported in PubmedTM, we will focus in this review on first describing the use of LOC platforms to monitor amyloid seeding and aggregation, and second whether LOCs help researchers understand cell-to-cell amyloid spreading and how LOC devices can help us analyze amyloid effects in neural network physiology. However, in order to understand their potential, first we will broadly summarize the most basic physical concepts of microfluidics that play crucial roles in the design of some of the approaches and devices that will then be further described. In addition, some specific references are included for those readers interested in particular aspects of microfluidics.
Some Basic Physical Concepts in Microfluidics
In biotechnology and bioengineering, microfluidics and LOC are present in various forms depending on the application and end use. The most usual form is single-phase microflows inside channels or capillary tubes. This is the typical case of a physiological buffer or a culture medium that contains a molecule or analyte that should be detected or analyzed using an LOC device. However, for different studies we need to use multiphase flows. This implies the use of two different non-miscible fluids (for example in micro/nanodroplet formation or cell encapsulation). However, in both single and multiphase flows, fluids are often moved by applying hydraulic pressure to the fluid inside the channel/s depending on the LOC design. In some cases, the applied force is a gravitational force but in other cases syringe pumps are used to move the fluid. In order to understand fluid dynamics in single-phase microflows inside small microchannels, first we need to focus our attention briefly on their behavior. In microfluidics one of the most frequently used key values is the Reynolds number (Re). This is a dimensionless number expressed as a function of the density of the fluid (ρ), the velocity of the fluid inside the channel (u), the length of the channel (L), and the dynamic viscosity of the fluid (η)
At the microscale (as happens in most LOC devices) the Re number is very low and the fluids behave like a laminar non-turbulent fluid. In fact, very few microfluidic devices used turbulent flows (with Re values > 2,000), but a differential range of fluid flows can be used in a laminar regime. Those microflows that show an Re value <0.5 follow the multiparametric Navier-Stokes formulation (see Tabeling, 2005; Bruus, 2008 for details). In these cases, aqueous fluids are considered Newtonian fluids which belong to the category of incompressible, uniform, and viscous fluids. However, for micro/nanodroplet or digital microdroplet approaches (see below) the use of two fluids (water and oil) with different behaviors is typical. In these cases, the LOC device can use non-Newtonian fluids that do not follow the formulation below. We refer the reader to classical reference books for specific formulation for non-Newtonian fluids (i.e., Böhme, 1987; Bruus, 2008; Berthier and Silberzan, 2010).
In addition to the Reynolds number, the interfacial tension also plays a role. It states the elastic tendency of a fluid in a surface to contract the surface-air interface in order to reduce its free energy (Sackmann et al., 2014). Interfacial tension is relevant in two immiscible fluids. In this situation and at this scale, interfacial tension dominates the gravitational force (Sackmann et al., 2014). Another dimensionless number is the capillary number. This number compares surface tension forces with viscous forces. Where σ is the interfacial tension, u is the velocity and η is the viscosity.
In addition to these values, the Bond number or the Weber number are also described. We refer the reader to Tabeling (2005), Bruus (2008), and Grimmer and Wille (2020) for more details. The factors are relevant for droplet formation. In this review we will consider the droplet formation in a T-junction system in detail (Figure 2; Teh et al., 2008). The reader can obtain current information on droplet LOC designs in Grimmer and Wille (2020). In the scheme, the fluid flows are determined as Qc and Qd and the dimension of the channels Wd and Wc. In this LOC, the Ca is one of the most important parameters in droplet generation. For example, when the Ca number is low, the incoming dispersed phase fluid tends to occupy the full section of the channel and droplet formation occurs at the downstream side of the T-junction corner. However, if Ca is higher the dispersed phase fluid occupies only a part of the channel and smaller droplets are formed. Practically, when a droplet is generated it continues to grow for a time tn for necking due to the continuous injection of the dispersed phase fluid. At the end, the final droplet volume V can be predicted (in an easiest vision) with this scaling law.
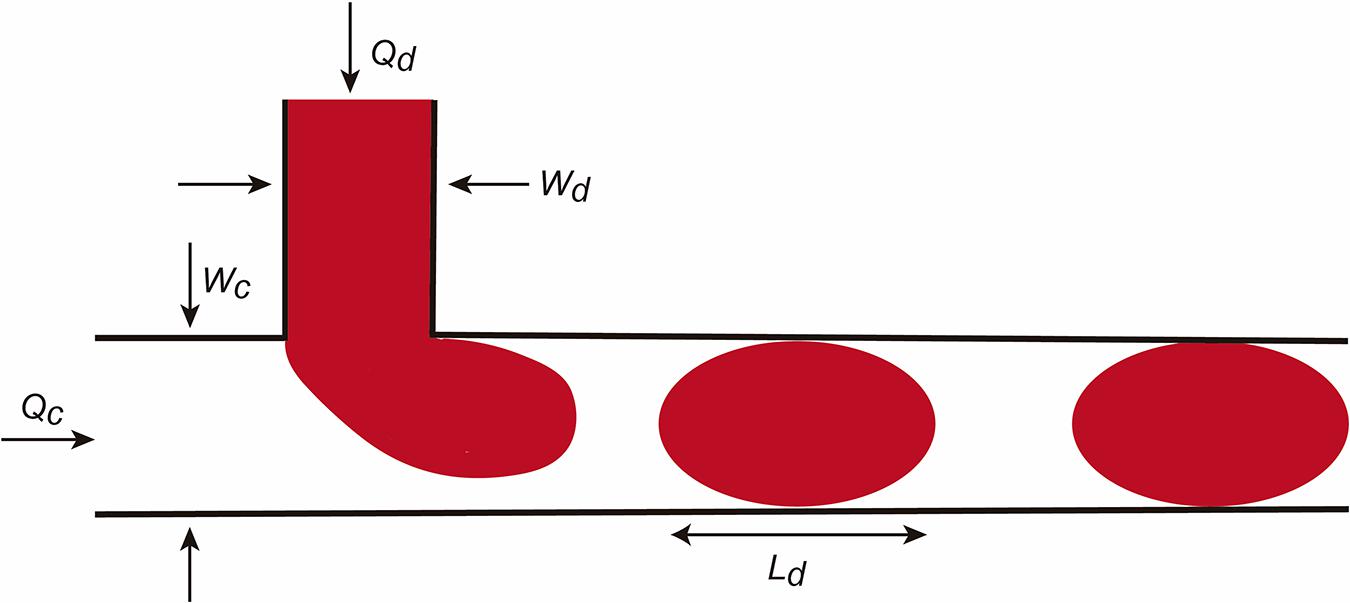
Figure 2. Scheme illustrating micro/nanodroplet formation in a classical T-junction LOC. Please see the main text for details concerning the Q and W values. In this configuration, the dispersed phase is pumped into the continuous phase orthogonally. As the dispersed phase enters the continuous, shear forces elongate the head of the dispersed phase until a segment eventually separates and relaxes into a sphere or plug shape as a result of interfacial tension.
Where Vc depends on Ca and the duration of necking tn and decreases as Ca increases. Another relevant factor in droplet formation is the flow rate radio Q (Q = Qd/Qc) (from dispersed and C for continuous phases). For example, for small Q the droplet is pinched off at the T-junction corner regardless of the Ca number. However, for large Q, increasing Ca will force the detachment point to move from the corner downstream. Due to the large surface-to-volume ratio, fluid/surface interactions largely affect droplet dynamics in the microchannels. In parallel, the presence of the droplet changes the hydrofluidic resistance of the microchannel. If fact, we can assume that the overall flow resistance of a channel can be estimated by:
Where R is the resistance of the channel, n is the number of droplets inside the channel, and Rd is the single droplet resistance. The value of Rd has been studied in several reports and is dependent of several factors such as the viscosity of the dispersed phase and the continuous phase, and the length of the droplet, as well as the geometry of the microchannel (see below). Considering these parameters today we can generate microdroplets for amyloid aggregation in micro/nanodroplets in a controlled space.
For non-bioengineering researchers, and considering Newtonian fluids, the third relevant aspect is that following microfluidic rules, we can correlate the behavior of a pressure-driven microfluidic laminar flow inside a channel with Ohm’s law of electricity (R = V/I). Thus, the ratio of the fluid pressure (P) ≈ V (voltage) and the volumetric fluid flow rate Q is the electrical current I (Figure 2). This occurs since under laminar flow, the pressure drop inside the microchannel is proportional to the flow rate: ΔP = RQ*Q. The hydraulic resistance RQ (also termed RH in some references) is dependent on microchannel geometry: the cross-section area (s) but also the length (L) of the microchannel. Thus, for an arbitrary section of the microchannel (with equal x and y dimensions) the value of RQ may be expressed as follows:
Where μ is the dynamic viscosity of the fluid and p is the perimeter of the microchannel. Thus, in this situation the Hagen-Poiseuille equation can be used in a channel with circular section to determine the ΔP as follows (reviewed in Shah and London, 1978):
In the case of a channel with square section [height (h) = width (w)] or rectangular section (when w/h > 1), the hydraulic resistance of the channel (RQ) can be calculated respectively as follows (see Grimmer and Wille, 2020 for details):
where a denotes (in rectangular section) a dimensionless parameter defined as
However, in a presence of a droplet (see above) the resistance of the microchannel dynamically changes. In fact, the values for the Rd of the droplet are described by this formulation
Where Ld is the length of the droplet, μd the viscosity of the dispersed phase, and μcont the viscosity of the continuous phase and the other parameters described above. Consider that the Hagen-Poiseuille law is similar to Ohm’s law (see above). Thus, these calculations are very useful to modulate and determine flow, pressures, and channel geometry as well as to simulate the behavior of our microfluidic flows (i.e., culture media, droplet formation) inside a particular LOC. As examples, we will describe two different microfluidic devices with wide use in neurobiology using correlative microfluidics as well as electric circuit modeling (please see Oh et al., 2012 and Robertson et al., 2014 for additional details).
The Hydrodynamic Focusing/Mixing LOC Device
Hydrodynamic focusing is a method to control continuous flow for solution mixing of fluids or droplets. The basis is the formation by confining fluid streams or flows to small geometries or microchannels. The main advantage of this strategy is the achievement of very short complete mixing times while maintaining a highly controllable system which can be precisely modeled. In the basic system (Figure 3A), there are three inlet streams: one central stream to be focused and two streams that converge perpendicularly on the central stream (Figures 3A–C). In the example, we consider, in Figure 3B, the electric model in which the section of all channels is identical (α) and the value β is the distance between the reference point P0 and the end of the mixing channel. Following Kirchhoff’s two laws, we can approximately define the Wf size in the mixing channel by applying the following equation based in fluid dynamics:
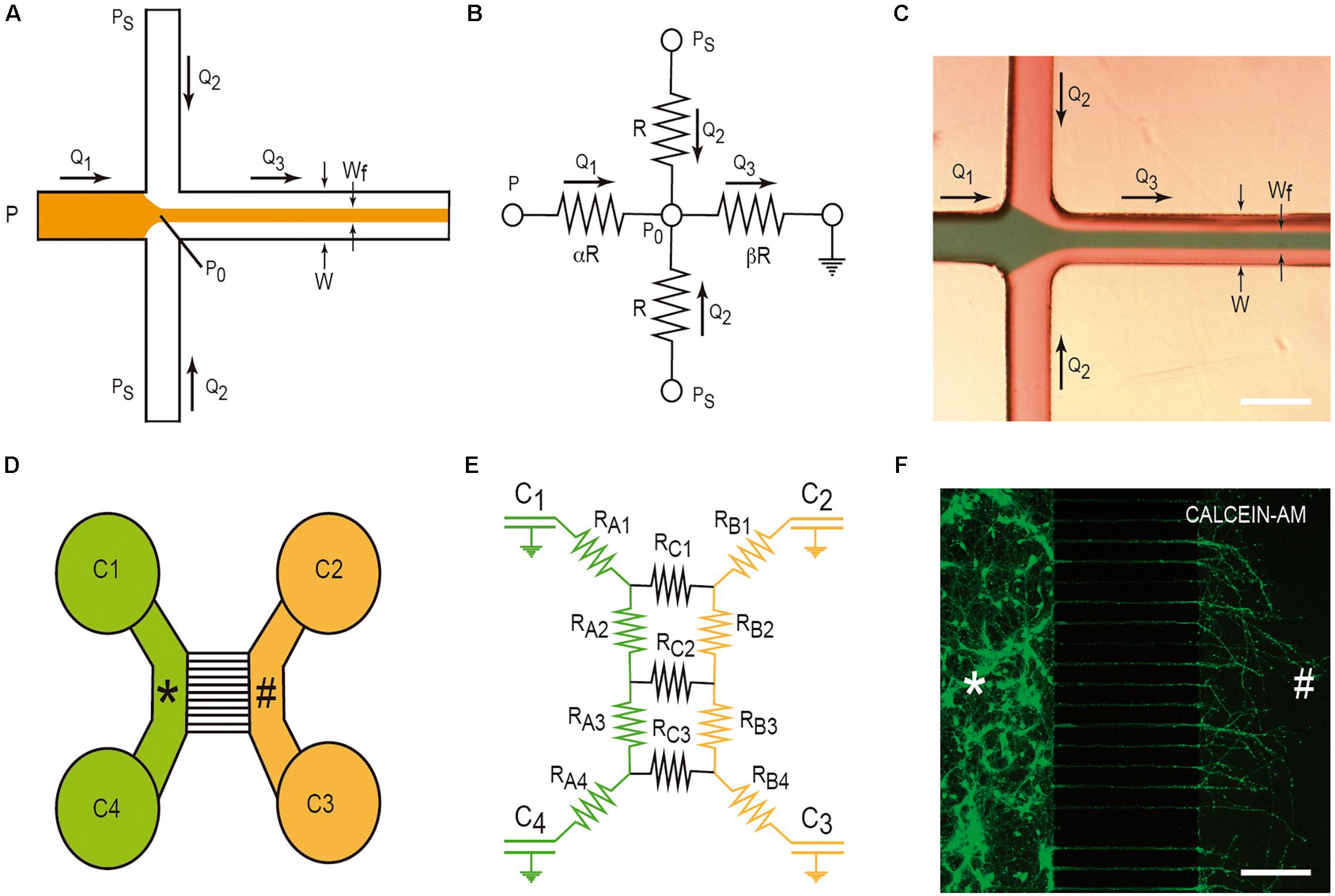
Figure 3. Examples of LOC devices and microfluidic platforms. Microfluidic hydrofluidic mixing device (A–C) and compartmentalized LOC device (D–F). For each device, the correlative fluidic and electric model is included. In (C,F), we provide two examples of the generation of hydrodynamic focusing (C) and the growth of axons labeled with CalceinTM. The two different compartments are labeled with * and # in (D–F). Please see manuscript section “Some Basic Physical Concepts in Microfluidics” for details of the correlation between fluidics laws and electric laws in LOC devices.
where g (λ) is a factor depending on the aspect ratio of the channel h/W, h being the height and W the weight of the microchannel, if h <<< W; λ −> 0, but more commonly h <1 and the formula is more complex (please see Berthier and Silberzan, 2006; Rodriguez-Villarreal et al., 2010 for details). Alternatively, and as first described by Knight et al. (1998), considering Ohm’s law and the electric model (Figure 3B), the summary of the calculation is the following:
Thus, using these parameters we can control the interaction between two different fluids by modulating Q1 and Q2. In fact, this was used in a large number of experiments (see Kirby, 2010; Badilescu and Packirisamy, 2011 for reference books). In a laminar flow regime mixing only occurs by diffusion. Thus, the mixing of the analytes depends only on their intrinsic diffusion coefficient (see Arter et al., 2020 for a recent review). After this, the diffusion parameters of the different elements (i.e., oligomers) can be controlled and analyzed during amyloid aggregation. In fact, these systems can also include several detection systems such as electrodes (stimulating or recording) as well as optical measurement. In this respect, the Peclét number (Pe) describes the relative rates of molecular convection relative to diffusion. Classically, the LOCs retain large values of Pe to prevent complete diffusional mixing over the timescale. Thus, LOCs are well suited to the study of protein-protein interactions. Typically, this is achieved through quantification of changes in the size or charge of proteins and complexes as they participate in the interaction. Due to the above-mentioned basis, the mixing rate of analytes under microfluidic flow can be measured by analyzing their diffusion coefficient and the hydrodynamic ratios of the biomolecules. With these approaches, the diffusion of α-synuclein (Zhang Y. et al., 2016) and β-amyloid (Scheidt et al., 2019) were analyzed. However, and as an alternative, these experiments have also been developed in droplets from fento to nanoliter volumes. These droplets can be generated at different frequencies (from 0.1 to 1 MHz). In contrast to bulk-phase studies in which aggregation reactions are dominated by secondary effects that rapidly amplify the rate of protein misfolding, masking primary nucleation events (i.e., Knowles et al., 2009), in LOCs droplets single nucleation events can be observed on a drop-by-drop basis, an approach that also enables observation of the spatial and temporal propagation of some fibrillar proteins (e.g., insulin; Knowles et al., 2011; Pfammatter et al., 2017).
Compartmentalized Cell Culture With Microchannels Growing Under Microfluidic Isolation in Two or More Chambers
This microfluidic device was developed to culture two or more different cell types (Figure 3D). In fact, their original goal was to compartmentalize cultured neurons in order to microfluidically isolate the somato-dendritic domain from the axonal domain for axonal RNA isolation for axotomy purposes (Figure 3D). This was achieved by using four open chambers with two channels that were interconnected by a variable number of microchannels (up to ∼100). These microchannels showed (in the original model) a small rectangular section with a high fluidic resistance due to its length. This largely reduced the fluidic flow between the two chambers. A more detailed description of the original microfluidic device can be found in Taylor et al. (2005). In order to isolate axons, although depending on the cultured neuron, the length of the microchannels should be more than 400–450 μm since dendrites or immature neurites are able to enter inside microchannels, but for cortical neurons, they only extend for a distance ≈150–250 μm in contrast to growing axons that might extend for distances longer than 1 mm inside the microchannels (Taylor et al., 2005; Figure 3F). Taking into account Ohm’s law, Robertson et al. (2014) developed an electric model of the device (Figures 3D,E). With this, we can model the volumetric transport of the media between the different chambers. In the modeled LOC device, the platform contains a network of resistances RA–C, linking four chambers C1-C4 (currently of 7–8 mm ∅). Considering the volume of each chamber (acting as an electronic capacitator), the hydrodynamic pressures (electrical voltage) and the volumetric flow rates (electric current) can be predicted over time as follows:
where the flow rate V(t) is the differential volume between wells over time and P(t) is the differential hydrostatic pressure between wells over time. Depending on the values of C and R and the initial conditions of volume of each chamber, the time constant t = RC/2 provides us data to estimate the rate of change of the hydraulic pressure, and consequently allows us to estimate the time needed to reach a volume equilibrium in the chambers of the LOC device. In addition, this procedure is a very useful tool during device design, empowering optimization of the relationship between the assay to be performed and design of the microchannel geometry (i.e., length, cross-sectional dimensions, etc.) (Robertson et al., 2014). On a practical basis, we can modulate the volume of each chamber to generate small but controlled media flows that will impair, in parallel with the fluidic resistance inside the small microchannels, the unwanted diffusion of a putative analyte or amyloid through the medium based on a differential concentration. This will be very useful to determine cell-to-cell prion/amyloid transmission; furthermore, we can determine whether an amyloid is taken up by specific domains of the healthy or unhealthy neuron or glial cell (see below). From the numerical data evaluation, the authors analyzed two putative configurations (flow paths): a pressure gradient between wells connecting a culture chamber (i.e., C2 and C3) and a pressure gradient across the microchannels. Using these numerical simulation, the estimated time constants for the pressure to equilibrate were 189 s across each chamber (tB) and 39,956 s across the microchannels (tC), respectively (Robertson et al., 2014). As tC is two orders of magnitude greater than tB, the two chambers can be considered fluidically isolated over short periods of time. However, the authors do not provide additional data concerning longer times. This is usually solved by increasing the volume of the culture medium in one part of the device with respect to the other. Another relevant aspect in these LOCs is the maintenance and survival of cultured cells. In our experience, we were unable to maintain cultured neurons for more than 20 days. This impairs a putative long-term experiment on infectivity and only specific processes can be analyzed. Furthermore, this limits the use of induced pluripotent stem cells (IPSc), with large differentiation protocols, in these devices. In order to avoid this, pre-differentiated neurospheroids are currently used in similar LOCs and some of these are commercially available (Osaki et al., 2020).
Amyloid Aggregation Studies in LOC Devices
Although most amyloid species were initially identified within the context of neurodegenerative diseases and their transmission (see Peng et al., 2020 for a recent review), several proteins have also been found to form amyloid-like fibrils [e.g., islet amyloid polypeptide (amylin) (Zheng et al., 2020) or insulin (Zheng et al., 2020)] outside the nervous system. Furthermore, increasing evidence has pointed toward amyloid formation being a generic self-aggregation property of proteins and polypeptide chains, with many polymeric species having been identified during the process (see Morris et al., 2009 for review). In fact, a key aspect in evaluating the aggregation kinetics of biomolecular species is the ability to monitor fibrillar growth as a function of time (see above). The monitoring of this process can be developed in LOC [e.g., in hydrodynamic focusing systems (i.e., Fitzpatrick et al., 2013; Arosio et al., 2016)], or using electrophoretic approaches in these systems (i.e., Saar et al., 2018).
However, it is well-known that the amyloid aggregation process is largely dependent on the interaction of the protein with several ions (i.e., Kim et al., 2018; Metrick et al., 2019), membranes (i.e., Terakawa et al., 2018; Alghrably et al., 2019), and other surfaces (e.g., water; Schladitz et al., 1999). Thus, researchers used different methods to avoid or control these in some cases unwanted interactions. One of the most widely used methods is the aggregation study inside micelles or micro/nanodroplets (water-oil) of different proteins (i.e., Shim et al., 2007; Teh et al., 2008; Casadevall i Solvas et al., 2012; Shembekar et al., 2016; Figure 4). Using microdroplets, many independent reactions can be monitored simultaneously in identical volumes which are several orders of magnitude smaller than what has been common in biochemical assays. On a practical level, the formation of the droplets, mainly by microfluidic focusing devices, is followed by their harvesting and maintenance, trapped or retained in reaction chambers to be further analyzed (i.e., Shim et al., 2007; Teh et al., 2008; Casadevall i Solvas et al., 2012; Shembekar et al., 2016; Figure 4B). In these cases (especially for sessile droplets exposed to air) (Casadevall i Solvas et al., 2012), the decrease in droplet volume over time due to the evaporation of water molecules increases the analyte/protein concentration inside the generated droplet, thereby increasing protein-protein interaction and, more relevantly, amplifying signal(s) associated with the aggregation that can be monitored with several methods, including Förster resonance energy transfer (FRET) (Figure 4). Other alternatives to enhance the process of reduction of the microdroplet volume using PDMS-derived microflows to enhance the reactions were also recently reported (i.e., DroMiCo; Kopp et al., 2020). This recently published method generates and traps the microdroplets, the aqueous flow rate is stopped in the LOC, and the oil flow rate is kept minimal at 2 μL/min to accelerate droplet shrinking and prevent entry of air into the device, in order to further analysis.
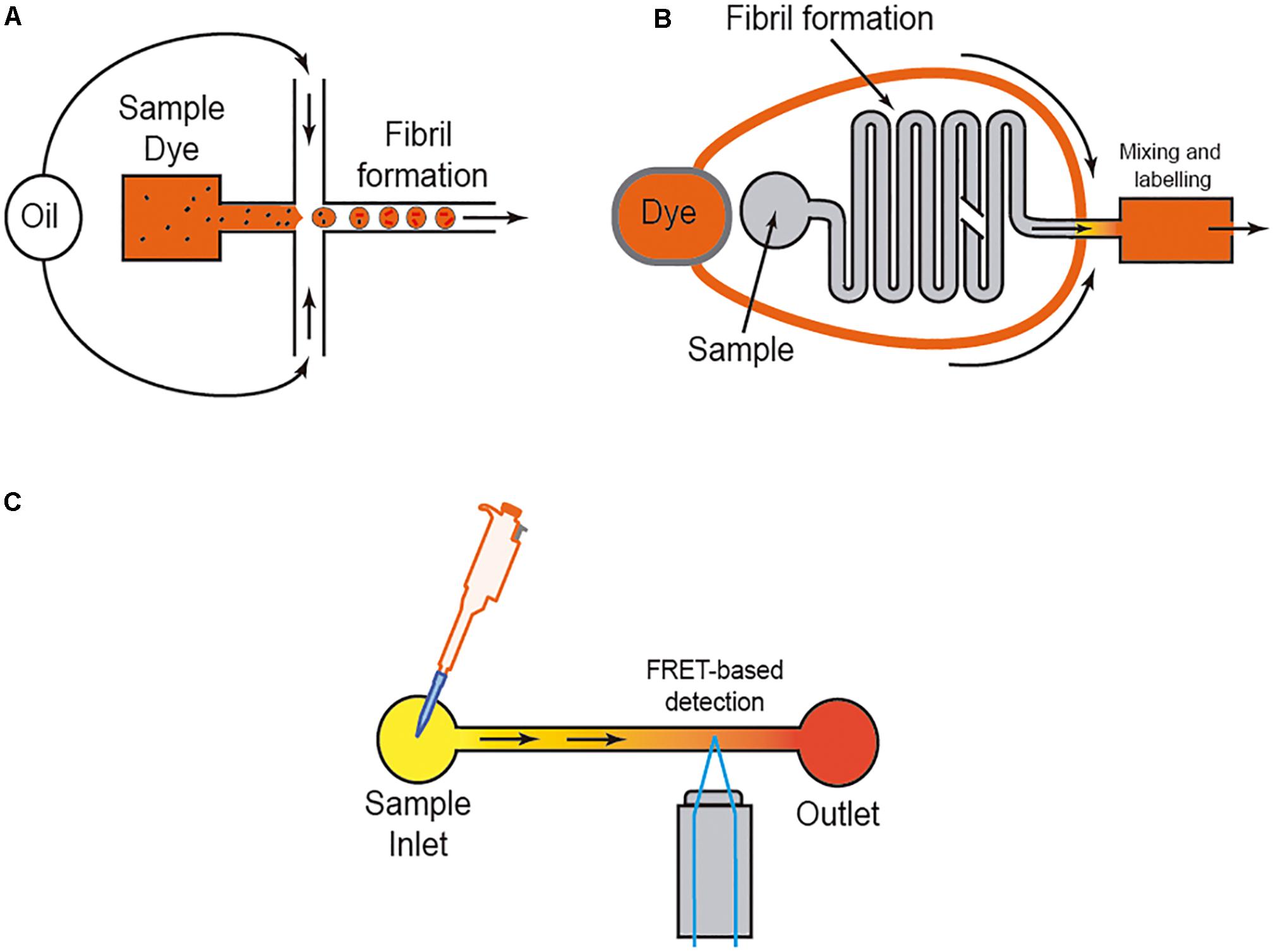
Figure 4. Scheme of three different approaches to analyzing amyloid formation under fluidic flow. In (A), the micro/nanodroplet formation (water-oil) is illustrated. In (B) a mixing LOC device is illustrated, and in (C) the use of FRET derived protein sensors to monitor protein-protein interaction is illustrated. In each example, the presence of the dye (usually ThT) is displayed.
In fact, amyloid fibril formation typically displays sigmoidal growth kinetics (i.e., Ferrone et al., 1985; Knowles et al., 2009; Morris et al., 2009; Prusiner, 2017). Indeed, changes in the aggregation of fibrils make up a three-phase curve that can be monitored easily using ThT-fluorescence or, depending on the protein sequence, intrinsic amino-acidic residues (i.e., tryptophan) with particular fluorescence properties after aggregation (i.e., Knowles et al., 2009; Meisl et al., 2016; Toprakcioglu et al., 2019). In current aggregation kinetics, the first stage is a “lag-phase” also termed “nucleation-phase” displaying a very small increase in the amount of fluorescence over time (i.e., Arosio et al., 2015), followed by a fast-growing phase and the last phase or “steady state” equilibrium with higher fluorescence values. However, we should consider that this is a dynamic process that includes fibrillar fragmentation, nucleation, and other processes during all the phases. In fact, the duration of the “lag-phase” is highly dependent not only on the monomer concentration and seed formation, but also on physical parameters as well as the presence of molecular chaperones. For example, when sarkosyl-insoluble fractions from AD patients or other tauopathies are analyzed in bulk experiments, the lag-phase cannot be clearly distinguished due to the high concentration of preformed fibrils and “amyloid seeds” (i.e., Ferrer et al., 2020b) in the sample and the curve is almost logarithmic. In fact, the time evolution of the “lag-phase” is also of interest for the characterization of some amyloids (i.e., MSA-derived synuclein vs. PD-derived synuclein) with different seeding as well as propagative properties (i.e., Shahnawaz et al., 2020). Additionally, in most studies the molecular events that occur in this “lag-phase” of amyloid formation are not fully ascertained using classical analytical methods. However, LOC devices and microdroplet formation were very useful in describing, in greater detail, the sequential events during this fibril formation for different amyloids (i.e., Aβ; Arosio et al., 2014), silk fibroin, β-lactoglobulin, lysozyme (Toprakcioglu et al., 2019), human islet amyloid polypeptide (Marek et al., 2010), and sickle hemoglobin (Ferrone et al., 1985). In fact, the events that occur during this lag-phase are of special interest in the effort to understand amyloid dynamics during neurodegeneration (Orru et al., 2015; Masujin et al., 2016).
In neurodegeneration, although with some discrepancy, it is well-established that lower aggregative amyloid species are more relevant species (i.e., toxic) compared to large amyloid species in different diseases [i.e., for Aβ (Deshpande et al., 2006; Hepler et al., 2006) and α-synuclein (Conway et al., 2000; Winner et al., 2011; Chen and Cremades, 2018; Prots et al., 2018)]. More relevantly, researchers aim to determine the properties of the “propagative seeds” or “propagons” for particular amyloids. This is a challenging question, especially for “prion-like” proteins (Aguzzi and Lakkaraju, 2016). Over time, classical bulk methods have been modified to match those that include an amplification step. For example, using a new method, Arosio et al. (2014) detected Aβ propagons in a “lag-phase” of aggregation by sample filtration during the phase, followed by an amplification method with fresh Aβ monomer. This was followed by the quantification of the original propagon concentration using a calibration curve based on controlled seed concentration (Arosio et al., 2014). Using this method, the authors improved by two orders of magnitude the bulk technical approaches to allow the concentration of fibrillar Aβ (Arosio et al., 2014). However, the recent development of “digital microfluidics” which combines the use of microfluidics and high-throughput biological assays (Guo et al., 2012) has helped researchers to develop a digital amyloid quantitative assay (d-AQuA) aimed at allowing absolute quantification of single replicative units, the “propagons,” in the proof-of-concept manuscript of insulin (Pfammatter et al., 2017). In fact, the authors used a dilution method of nanodroplets with picolitres of volume containing (or not) “propagon” molecules that were further evaluated using ThT staining, following a Poisson distribution probabilistic model (Pfammatter et al., 2017). This method, although not developed for other amyloids, is faster than currently available methods (e.g., microplate assays) and will be of relevance for fast diagnosis of the presence of “pathological-seeds” for different PMDs. Parallel to these approaches, other groups have developed more automatic methods such as the microchannel-connected multiwell plate (μCHAMP) device (Park et al., 2016) that uses microdroplet formation and microfluidic transport to 96-well plates. The amount of Aβ (at a range of ≈ 10 pg/mL) is detected using a droplet-based magnetic bead immunoassay (Park et al., 2016). Thus, LOC devices are of relevance not only for the reduced sample volume required, but also for automatization and high-throughput assays of amyloid detection. In addition, recent reports illustrate the relevance of the use of aggregation-induced emission (AIE) dyes as a preferential strategy to identify protein fibrillogenesis, particularly the light up characteristic associated with binding events during the aggregation process. These dyes feature high emission efficiency in the aggregate state, strong photo-stability, and excellent biocompatibility (Wurthner, 2020). Examples of their use can be found in the analysis of insulin (Hong et al., 2012; Huang Q. et al., 2017) and of β-amyloid aggregation (Zhang J. D. et al., 2016; Fu et al., 2019; Yang et al., 2019). Readers may find a general survey of these techniques in Wurthner (2020).
LOC as Tool to Analyze Cell-To-Cell Transport and Behavior of Amyloids
As indicated above, studies of seeding and spreading of different amyloids in vitro must be complemented by complex cellular systems mimicking in vivo situation as well as animal experimentation. Indeed, several groups have developed in vitro cellular models to monitor seeding properties and their associated effects in cell survival. However, for some amyloids (e.g., tau) their overexpression in cells resists aggregation instead of hyperphosphorylation, and additional methods are needed to increase their intracellular content, avoiding phosphorylation. One of the alternatives is a commercially available BioPORTER QuikEase Protein Delivery Kit which can deliver active proteins intracellularly. This method was used by Guo and Lee (2011) to demonstrate that the seeding of normal tau induced by pathological tau led to the formation of NFL-like structures in treated cells (Guo and Lee, 2011). In other approaches, cell lines overexpressing mutated protein with aggregative properties fused with a flag (fluorescence) sequence (i.e., P301L-V5; Xu et al., 2016) have been used for high-throughput assays of tau aggregation. In the presence of an aggregating amyloid species (e.g., tau) the aggregation process can be followed by analyzing the emitted fluorescence. Some of these approaches have also included FRET methods (Shin et al., 2019). These cellular “biosensors,” mainly developed in HEK293 or H4 cells, have been adapted for fluorescence detection of other amyloids such as α-synuclein (Prusiner et al., 2015; Holmes and Diamond, 2017). In fact, these methods are already being supported by the use of ultra-resolution confocal microscopy (Kaminski and Kaminski Schierle, 2016) to monitor the amyloid seeding and aggregation processes in cell cultures at the nanoscale level. Today the use of these biosensors is mainly focused on drug screening. However, in a putative scenario the use of these cells in LOCs could be of relevance in determining key factors involved in the seeding process of amyloids (e.g., the relevance of a specific receptor in the process).
However, as indicated, researchers aimed to determine cell-to-cell mechanisms implicated in amyloid seeding and spreading (see introduction). In this respect, several studies reported using LOC devices, with two or more consecutive chambers: Aβ (i.e., Deleglise et al., 2014; Song et al., 2014), α-synuclein (i.e., Volpicelli-Daley et al., 2011; Freundt et al., 2012; Brahic et al., 2016; Urrea et al., 2018; Wang et al., 2018), tau (i.e., Wu et al., 2013; Dujardin et al., 2014; Calafate et al., 2015; Takeda et al., 2015; Usenovic et al., 2015; Congdon et al., 2016; Polanco et al., 2018), TDP-43 (i.e., Feiler et al., 2015), and dipeptide repeat proteins (DPRs) of the C9orf72 gene product associated with ALS and frontotemporal dementia (FTD). Westergard et al. (2016) were able to perform cell-to-cell transmission by navigating intracellularly along axons allowing seeding and propagation of the amyloid (reviewed in Urrea et al., 2018; Peng et al., 2020; Uemura et al., 2020). These studies reported the sequential transport of the “pathogenic seeds” as free seeds or in exosomes between different cell populations cultured in different chambers (Figure 5). In these experiments a differential volume between reservoirs is established to avoid diffusion transfer by the media between reservoirs. In fact, different LOCs have been designed for specific studies (e.g., co-culture of microglia, astroglia, and neurons (3D hNeuroGliAD) (Park et al., 2018). More relevantly, in several studies neurons derived from IPSc or neuronal progenitors are included to mimic specific neurodegenerative diseases (i.e., Choi et al., 2014; Ruiz et al., 2014; Park et al., 2018). These LOC approaches help researchers to ascertain the role of non-neuronal cells in the seeding and propagation process for particular amyloids (e.g., Aβ roles of microglia reactivity and migration (Cho et al., 2013; Park et al., 2018) and the role of astrocytes in α-synuclein seeding (Cavaliere et al., 2017). However, the emerging role of oligodendrocytes as non-neighboring cells during some amyloid transmission [i.e., α-synuclein (Tu et al., 1998; Uemura et al., 2019), tau (Ferrer et al., 2019, 2020a) see also (Ferrer, 2018) for recent review] has yet to be described in LOC devices. This is in contrast to the different LOC devices aimed at exploring myelination (Park et al., 2009; Yang et al., 2012; Lee et al., 2016). Further studies will help neuroscientists to determine their putative role in PMD.
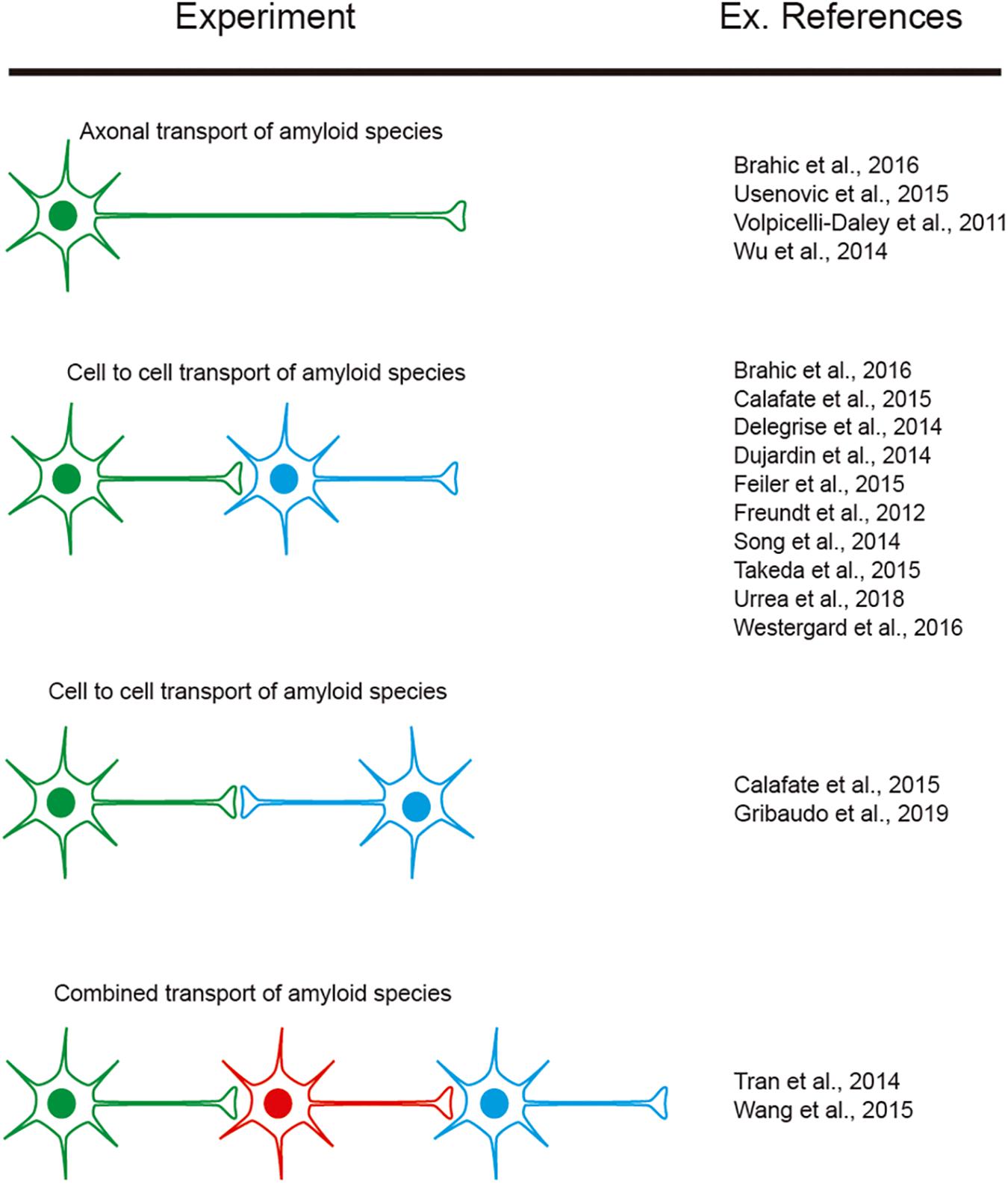
Figure 5. Examples of experiments designed to explore amyloid transport in axons as well as cell-to-cell transmission. The different colors of the illustrated cells represent the cells cultured in different reservoirs of compartmentalized LOC devices and their orientation. In addition, some references are included as examples of their exponential use in neurodegenerative neuroscience.
LOC as a Tool to Analyze the Neural Consequences of Amyloid Transmission
From the early development of the PDMS compartmentalized LOC, several groups combined electrical and optical measurements of neuronal activity with its compartmentalization (among others, Morales et al., 2008; Gladkov et al., 2017; Lassus et al., 2018; Lopes et al., 2018; Moutaux et al., 2018). And in some studies these LOCs devices were used as platforms to determine drug screening and cytotoxicity (i.e., Meissner et al., 2011; Du et al., 2016). As noted, the seeding and spreading of different amyloids is associated with an increase in progressive cell death. This was determined in classical cell cultures in single chambers but particularly in LOC with different chambers: (i.e., AβDeleglise et al., 2014; Ruiz et al., 2014; Li et al., 2017), α-synuclein (i.e., Tran H. T. et al., 2014; Prots et al., 2018; Gribaudo et al., 2019) and Tau (Wu et al., 2016) by direct exposure of cultured neurons to the pathogenic form of the amyloid. Cytotoxicity was measured with biochemical methods, multielectrode arrays (MEA), or changes in calcium transients [i.e., with Fluo4-AM or genetically encoded calcium indicators (GECIs)]. In this respect, several authors included microflows in 3D-derived cultures in LOC devices to determine the effects of exposure to amyloids. For example, Choi et al. (2013) developed a microfluidic platform capable of generating a gradient of Aβ oligomeric assemblies within microchannels to investigate their neurotoxicity. Two years later, Park et al. (2015) developed a pioneering microfluidic chip containing 3D-neurospheroids by providing an interstitial constant fluid flow. Using this 3D platform, the effect of the fluid flow on the neural proliferation, differentiation, and survival was investigated. The authors compared their results with similar Aβ treatments under static conditions, and the main conclusion was that treatment with Aβ under interstitial flow is more deleterious and largely reduces the viability of neural aggregates (Teller et al., 2015).
However, although effects of the pathogenic seeds have been evaluated in numerous manuscripts, little attention has been paid to elucidating the putative changes in neural networks during seeding and cell-to-cell progression between different areas. Due to the relevance of this issue, LOCs could be a relevant tool to ascertain this progressive neurodegeneration between different cell populations. This is of particular relevance for drug discovery, if our final goal is to block amyloid seeding, aggregation and progression. For most cases, a putative drug treatment (i.e., an inhibiting peptide) could be useful in silico, and probably non-cytotoxic in yeast, worm, fruit flies, or mammalian cells, but it could carry collateral effects in modifying the function of neuronal networks. To solve this, in our opinion, we need first to understand the changes induced by pathogenic amyloid during the seeding and aggregation process from both the cellular viewpoint and a system-wide perspective. One example can be seen in a pioneer study developed by Teller et al., in which primary cortical neurons were confined into groups using a homemade PDMS mask (Figure 6). In the study, the authors determined the synaptic activity and the development of neuronal networks by using calcium fluorescence probes (Fluo4-AM) and the analysis of the intracellular calcium changes to determine firing rate, coordination between different neurons, and the appearance and maturation of different connectivity “hubs” in the culture. After several days in culture to allow the maturation of connections between these neuronal groups, the authors incubated the culture with different aggregated forms of Aβ (with magnetite). Changes in neural network activity and the correlated neuronal interactions after the treatments were evaluated, and the effects of Aβ treatment in the destabilization of the generated network was determined in detail. In order to analyze this the authors used homemade calcium fluorescence analysis software NetCalTM (Orlandi et al., 2014; Teller et al., 2015; Figure 6). The data were also corroborated in our laboratory using mic2net software (Smedler et al., 2014). Thus, the group developed an in vivo-like platform for drug screening in the presence of progressive amyloid generation. Today the use of GECIs (i.e., GCaMP6) instead of classical fluorescence reporters (Fluo4-AM or Fluo8-AM) of neuronal activity and different analysis methods allow us to analyze the network activity of the same culture or neuronal network for longer time periods (Chen et al., 2013). These experiments will help researchers working in drug discovery to avoid the seeding and progression of the amyloids without perturbing neuronal activity.
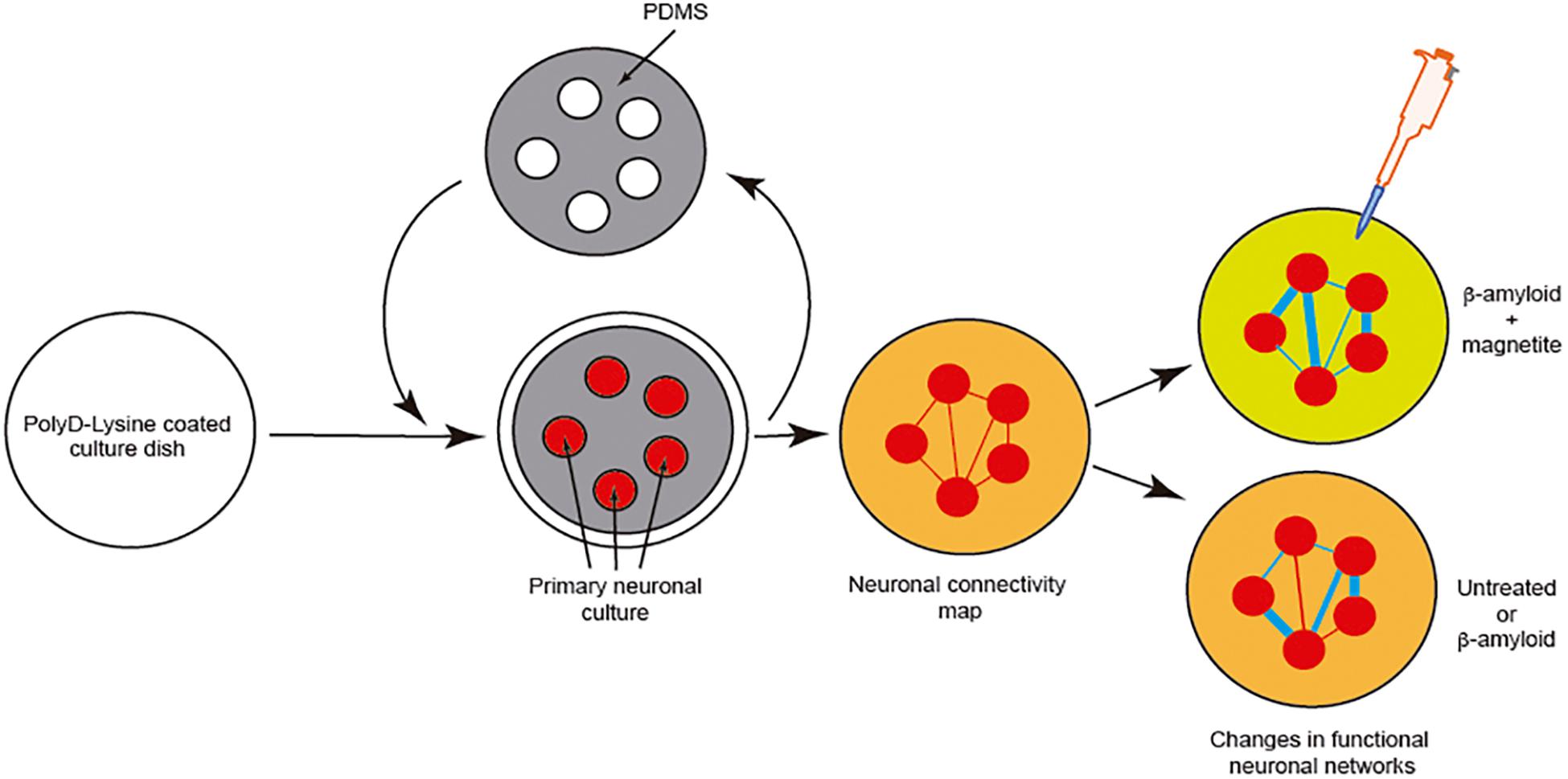
Figure 6. Example of the analysis of neuronal network changes mediated by amyloids (Aβ). We describe the experiment developed by Teller et al. (2015). In the experiment, the authors generated clusters of cells further interconnected in a network like the Atomium attraction in Brussels. After treatment with Aβ alone or Aβ-magnetite, the changes in the neural network were analyzed using NetCalTM software.
Concluding Remarks
In this review we have tried to summarize only a part of the state of the art concerning the use of some microfluidics and LOC devices in amyloid or “prion-like” seeding, aggregation, and cell-to-cell transmission research. Unfortunately, for prion infection other strategies would be more appropriate due to the time needed to develop a reliable prion infection. In fact, our lab studied the putative prion formation in IPSc cultured in 2D cultures for more than 150 days with negative results (Matamoros-Angles et al., 2018). Considering this, most probably a 3D culture is needed to develop prion infection, while LOCs will help us in other experimental situations. In this review, we have focused, on a practical basis, on those aspects that we consider relevant for biomedical researchers. In fact, most of the new techniques and proof-of-concept experiments involving aggregation, protein-protein interaction, and analyte detection are not fully described in this review, since, in some cases, they are not yet widely accepted by researchers. We refer the reader to some of the references of this review for additional information. In fact, here we have focused on two types of LOC that could be of interest and which are commonly used in laboratories. For example, micro/nanodroplets LOCs, compared to conventional microtiter plate assays, are an attractive platform for high-throughput studies. LOC devices derived from the compartmentalized culture of neurons or glial cells can help answer key questions in determining cell-specific differences in various disorders, revealing the participation of different cell types, uncovering the differing behaviors of the different “strains” of amyloid, etc. In addition, emerging technologies such as the use of graphene and other materials such as electrochemical and optochemical “biosensors” offer new opportunities to determine amyloid seeding and aggregation in more detail. As indicated, the bioengineering approaches have evolved in recent years, and today, some of them are tools for neurobiological laboratories, as an alternative to unnecessary animal experimentation following the 3Rs of biological research. Although we cannot assume that the use of LOC and microfluidics in neuroscience will follow Moore’s law of semiconductors, which suggests doubling of semiconductor numbers in a microprocessor every year, we believe that their use helps and will continue to help neuroscientists. Combined efforts from different disciplines—bioengineering, neurobiology, and clinical practice – in the face of new challenges will be mandatory for future biomedical research.
Author Contributions
JR and IF: drafting of manuscript, study conception and design, analysis and interpretation of data, critical revision, and final approval of the version to be published. Both authors contributed to the article and approved the submitted version.
Funding
This research was supported by the PRPSEM Project with ref. RTI2018-099773-B-I00 from the Spanish Ministry of Science, Innovation and Universities (MCIU/FEDER/AEI), the CERCA Programme, and by the Commission for Universities and Research of the Department of Innovation, Universities, and Enterprise of the Generalitat de Catalunya (SGR2017-648 to JR) and CIBERNED (CMED2018-2) to IF and JR. The project leading to these results received funding from “la Caixa” Foundation (ID 100010434) under the agreement LCF/PR/HR19/52160007 to JR and IF, and the María de Maeztu Unit of Excellence (Institute of Neurosciences, University of Barcelona) MDM-2017-0729 to JR. IF was funded by the Ministry of Economy and Competitiveness, Institute of Health Carlos III (ISCIII–Fondos FEDER, a way to build Europe: FIS PI17/00809).
Conflict of Interest
The authors declare that the research was conducted in the absence of any commercial or financial relationships that could be construed as a potential conflict of interest.
Acknowledgments
We thank Tom Yohannan for editorial advice and all members of the Del Rio and Ferrer labs for their comments.
References
Abounit, S., Wu, J. W., Duff, K., Victoria, G. S., and Zurzolo, C. (2016). Tunneling nanotubes: a possible highway in the spreading of tau and other prion-like proteins in neurodegenerative diseases. Prion 10, 344–351. doi: 10.1080/19336896.2016.1223003
Aguzzi, A., and Lakkaraju, A. K. K. (2016). Cell biology of prions and prionoids: a status report. Trends Cell Biol 26, 40–51. doi: 10.1016/j.tcb.2015.08.007
Aguzzi, A., and Rajendran, L. (2009). The transcellular spread of cytosolic amyloids, prions, and prionoids. Neuron 64, 783–790. doi: 10.1016/j.neuron.2009.12.016
Alghrably, M., Czaban, I., Jaremko, L., and Jaremko, M. (2019). Interaction of amylin species with transition metals and membranes. J. Inorg. Biochem. 191, 69–76. doi: 10.1016/j.jinorgbio.2018.11.004
Arosio, P., Cukalevski, R., Frohm, B., Knowles, T. P., and Linse, S. (2014). Quantification of the concentration of Abeta42 propagons during the lag phase by an amyloid chain reaction assay. J. Am. Chem. Soc. 136, 219–225. doi: 10.1021/ja408765u
Arosio, P., Knowles, T. P., and Linse, S. (2015). On the lag phase in amyloid fibril formation. Phys. Chem. Chem. Phys. 17, 7606–7618. doi: 10.1039/c4cp05563b
Arosio, P., Muller, T., Rajah, L., Yates, E. V., Aprile, F. A., Zhang, Y., et al. (2016). Microfluidic diffusion analysis of the sizes and interactions of proteins under native solution conditions. ACS Nano 10, 333–341. doi: 10.1021/acsnano.5b04713
Arter, W. E., Levin, A., Krainer, G., and Knowles, T. P. J. (2020). Microfluidic approaches for the analysis of protein-protein interactions in solution. Biophys. Rev. 12, 575–585. doi: 10.1007/s12551-020-00679-4
Ashe, K. H., and Aguzzi, A. (2013). Prions, prionoids and pathogenic proteins in Alzheimer disease. Prion 7, 55–59. doi: 10.4161/pri.23061
Au, A. K., Huynh, W., Horowitz, L. F., and Folch, A. (2016). 3D-Printed Microfluidics. Angew. Chem. Int. Ed. Engl. 55, 3862–3881.
Aulic, S., Masperone, L., Narkiewicz, J., Isopi, E., Bistaffa, E., Ambrosetti, E., et al. (2017). alpha-Synuclein Amyloids Hijack Prion Protein to Gain Cell Entry. Facilitate Cell-to-Cell Spreading and Block Prion Replication. Sci. Rep. 7:10050.
Badilescu, S., and Packirisamy, M. (2011). BioMEMS : Science and Engineering Perspectives. Boca Raton, FL: CRC Press.
Bertrand, E., Lechowicz, W., Szpak, G. M., Lewandowska, E., Dymecki, J., and Wierzba-Bobrowicz, T. (2004). Limbic neuropathology in idiopathic Parkinson’s disease with concomitant dementia. Folia Neuropathol. 42, 141–150.
Bongianni, M., Orru, C., Groveman, B. R., Sacchetto, L., Fiorini, M., Tonoli, G., et al. (2017). Diagnosis of human prion disease using real-time quaking-induced conversion testing of olfactory mucosa and cerebrospinal fluid samples. JAMA Neurol. 74, 155–162. doi: 10.1001/jamaneurol.2016.4614
Braak, H., and Del Tredici, K. (2009). Neuroanatomy and pathology of sporadic Parkinson’s disease. Adv. Anat. Embryol. Cell Biol. 201, 1–119. doi: 10.1007/978-3-540-79850-7_1
Brahic, M., Bousset, L., Bieri, G., Melki, R., and Gitler, A. D. (2016). Axonal transport and secretion of fibrillar forms of alpha-synuclein, Abeta42 peptide and HTTExon 1. Acta Neuropathol. 131, 539–548. doi: 10.1007/s00401-016-1538-0
Calafate, S., Buist, A., Miskiewicz, K., Vijayan, V., Daneels, G., De Strooper, B., et al. (2015). Synaptic Contacts enhance cell-to-cell Tau pathology propagation. Cell Rep. 11, 1176–1183. doi: 10.1016/j.celrep.2015.04.043
Campenot, R. B. (1977). Local control of neurite development by nerve growth factor. Proc. Natl. Acad. Sci. U.S.A. 74, 4516–4519. doi: 10.1073/pnas.74.10.4516
Campenot, R. B. (1982). Development of sympathetic neurons in compartmentalized cultures. Il Local control of neurite growth by nerve growth factor. Dev. Biol. 93, 1–12. doi: 10.1016/0012-1606(82)90232-9
Casadevall i Solvas, X., Turek, V., Prodromakis, T., and Edel, J. B. (2012). Microfluidic evaporator for on-chip sample concentration. Lab Chip 12, 4049–4054. doi: 10.1039/c2lc40746a
Castilla, J., and Requena, J. R. (2015). Prion-like diseases: looking for their niche in the realm of infectious diseases. Virus Res. 207, 1–4. doi: 10.1016/j.virusres.2015.06.001
Cavaliere, F., Cerf, L., Dehay, B., Ramos-Gonzalez, P., De Giorgi, F., Bourdenx, M., et al. (2017). In vitro alpha-synuclein neurotoxicity and spreading among neurons and astrocytes using Lewy body extracts from Parkinson disease brains. Neurobiol. Dis. 103, 101–112. doi: 10.1016/j.nbd.2017.04.011
Chen, S. W., and Cremades, N. (2018). Preparation of alpha-synuclein amyloid assemblies for toxicity experiments. Methods Mol. Biol. 1779, 45–60. doi: 10.1007/978-1-4939-7816-8_4
Chen, T. W., Wardill, T. J., Sun, Y., Pulver, S. R., Renninger, S. L., Baohan, A., et al. (2013). Ultrasensitive fluorescent proteins for imaging neuronal activity. Nature 499, 295–300. doi: 10.1038/nature12354
Cho, H., Hashimoto, T., Wong, E., Hori, Y., Wood, L. B., Zhao, L., et al. (2013). Microfluidic chemotaxis platform for differentiating the roles of soluble and bound amyloid-beta on microglial accumulation. Sci. Rep. 3:1823.
Choi, S. H., Kim, Y. H., Hebisch, M., Sliwinski, C., Lee, S., D’Avanzo, C., et al. (2014). A three-dimensional human neural cell culture model of Alzheimer’s disease. Nature 515, 274–278.
Choi, Y. J., Chae, S., Kim, J. H., Barald, K. F., Park, J. Y., and Lee, S. H. (2013). Neurotoxic amyloid beta oligomeric assemblies recreated in microfluidic platform with interstitial level of slow flow. Sci. Rep. 3:1921.
Colby, D. W., Zhang, Q., Wang, S., Groth, D., Legname, G., Riesner, D., et al. (2007). Prion detection by an amyloid seeding assay. Proc. Natl. Acad. Sci. U.S.A. 104, 20914–20919. doi: 10.1073/pnas.0710152105
Collinge, J. (2016). Mammalian prions and their wider relevance in neurodegenerative diseases. Nature 539, 217–226. doi: 10.1038/nature20415
Congdon, E. E., Lin, Y., Rajamohamedsait, H. B., Shamir, D. B., Krishnaswamy, S., Rajamohamedsait, W. J., et al. (2016). Affinity of Tau antibodies for solubilized pathological Tau species but not their immunogen or insoluble Tau aggregates predicts in vivo and ex vivo efficacy. Mol. Neurodegener. 11:62.
Conway, K. A., Lee, S. J., Rochet, J. C., Ding, T. T., Williamson, R. E.,, and Lansbury, P. T.Jr. (2000). Acceleration of oligomerization, not fibrillization, is a shared property of both alpha-synuclein mutations linked to early-onset Parkinson’s disease: implications for pathogenesis and therapy. Proc. Natl. Acad. Sci. U.S.A. 97, 571–576. doi: 10.1073/pnas.97.2.571
Costanzo, M., and Zurzolo, C. (2013). The cell biology of prion-like spread of protein aggregates: mechanisms and implication in neurodegeneration. Biochem. J. 452, 1–17. doi: 10.1042/bj20121898
Del Rio, J. A., Ferrer, I., and Gavin, R. (2018). Role of cellular prion protein in interneuronal amyloid transmission. Prog. Neurobiol. 165-167, 87–102. doi: 10.1016/j.pneurobio.2018.03.001
Deleglise, B., Magnifico, S., Duplus, E., Vaur, P., Soubeyre, V., Belle, M., et al. (2014). beta-amyloid induces a dying-back process and remote trans-synaptic alterations in a microfluidic-based reconstructed neuronal network. Acta Neuropathol. Commun. 2:145. doi: 10.1186/preaccept-4848873741397798
Deshpande, A., Mina, E., Glabe, C., and Busciglio, J. (2006). Different conformations of amyloid beta induce neurotoxicity by distinct mechanisms in human cortical neurons. J. Neurosci. 26, 6011–6018. doi: 10.1523/jneurosci.1189-06.2006
Du, G., Fang, Q., and Den Toonder, J. M. (2016). Microfluidics for cell-based high throughput screening platforms - A review. Anal. Chim. Acta 903, 36–50. doi: 10.1016/j.aca.2015.11.023
Dujardin, S., Lecolle, K., Caillierez, R., Begard, S., Zommer, N., Lachaud, C., et al. (2014). Neuron-to-neuron wild-type Tau protein transfer through a trans-synaptic mechanism: relevance to sporadic tauopathies. Acta Neuropathol. Commun. 2:14.
Erana, H. (2019). The Prion 2018 round tables (II): Abeta, tau, alpha-synuclein.. are they prions, prion-like proteins, or what? Prion 13, 41–45. doi: 10.1080/19336896.2019.1569451
Erana, H., Venegas, V., Moreno, J., and Castilla, J. (2017). Prion-like disorders and Transmissible Spongiform Encephalopathies: an overview of the mechanistic features that are shared by the various disease-related misfolded proteins. Biochem. Biophys. Res. Commun. 483, 1125–1136. doi: 10.1016/j.bbrc.2016.08.166
Fairfoul, G., Mcguire, L. I., Pal, S., Ironside, J. W., Neumann, J., Christie, S., et al. (2016). Alpha-synuclein RT-QuIC in the CSF of patients with alpha-synucleinopathies. Ann. Clin. Transl. Neurol. 3, 812–818. doi: 10.1002/acn3.338
Feiler, M. S., Strobel, B., Freischmidt, A., Helferich, A. M., Kappel, J., Brewer, B. M., et al. (2015). TDP-43 is intercellularly transmitted across axon terminals. J. Cell Biol. 211, 897–911. doi: 10.1083/jcb.201504057
Ferrer, I. (2018). Oligodendrogliopathy in neurodegenerative diseases with abnormal protein aggregates: the forgotten partner. Prog. Neurobiol. 169, 24–54. doi: 10.1016/j.pneurobio.2018.07.004
Ferrer, I., Aguilo Garcia, M., Carmona, M., Andres-Benito, P., Torrejon-Escribano, B., Garcia-Esparcia, P., et al. (2019). Involvement of Oligodendrocytes in Tau Seeding and Spreading in Tauopathies. Front. Aging Neurosci. 11:112. doi: 10.3389/fnagi.2019.00112
Ferrer, I., Andres-Benito, P., Zelaya, M. V., Aguirre, M. E. E., Carmona, M., Ausin, K., et al. (2020a). Familial globular glial tauopathy linked to MAPT mutations: molecular neuropathology and seeding capacity of a prototypical mixed neuronal and glial tauopathy. Acta Neuropathol. 139, 735–771. doi: 10.1007/s00401-019-02122-9
Ferrer, I., Zelaya, M. V., Aguilo Garcia, M., Carmona, M., Lopez-Gonzalez, I., Andres-Benito, P., et al. (2020b). Relevance of host tau in tau seeding and spreading in tauopathies. Brain Pathol. 30, 298–318. doi: 10.1111/bpa.12778
Ferrone, F. A., Hofrichter, J., and Eaton, W. A. (1985). Kinetics of sickle hemoglobin polymerization. I. Studies using temperature-jump and laser photolysis techniques. J. Mol. Biol. 183, 591–610.
Fitzpatrick, A. W., Debelouchina, G. T., Bayro, M. J., Clare, D. K., Caporini, M. A., Bajaj, V. S., et al. (2013). Atomic structure and hierarchical assembly of a cross-beta amyloid fibril. Proc. Natl. Acad. Sci. U.S.A. 110, 5468–5473.
Fraser, H., and Dickinson, A. G. (1973). Scrapie in mice. Agent-strain differences in the distribution and intensity of grey matter vacuolation. J. Comp. Pathol. 83, 29–40.
Freundt, E. C., Maynard, N., Clancy, E. K., Roy, S., Bousset, L., Sourigues, Y., et al. (2012). Neuron-to-neuron transmission of alpha-synuclein fibrils through axonal transport. Ann. Neurol. 72, 517–524. doi: 10.1002/ana.23747
Fu, W., Yan, C., Guo, Z., Zhang, J., Zhang, H., Tian, H., et al. (2019). Rational design of near-infrared aggregation-induced-emission-active probes: in situ mapping of amyloid-beta plaques with ultrasensitivity and high-fidelity. J. Am. Chem. Soc. 141, 3171–3177. doi: 10.1021/jacs.8b12820
Giles, K., Woerman, A. L., Berry, D. B., and Prusiner, S. B. (2017). Bioassays and Inactivation of Prions. Cold Spring Harb. Perspect. Biol. 9:a023499. doi: 10.1101/cshperspect.a023499
Gladkov, A., Pigareva, Y., Kutyina, D., Kolpakov, V., Bukatin, A., Mukhina, I., et al. (2017). Design of cultured neuron networks in vitro with predefined connectivity using asymmetric microfluidic channels. Sci. Rep. 7:15625.
Goedert, M., Masuda-Suzukake, M., and Falcon, B. (2017). Like prions: the propagation of aggregated tau and alpha-synuclein in neurodegeneration. Brain 140, 266–278. doi: 10.1093/brain/aww230
Greenfield, J. G., Love, S., Louis, D. N., and Ellison, D. (2008). Greenfield’s Neuropathology. London: Hodder Arnold.
Gribaudo, S., Tixador, P., Bousset, L., Fenyi, A., Lino, P., Melki, R., et al. (2019). Propagation of alpha-synuclein strains within human reconstructed neuronal network. Stem Cell Rep. 12, 230–244. doi: 10.1016/j.stemcr.2018.12.007
Grimmer, A., and Wille, R. (2020). Designing Droplet Microfluidic Networks : A Toolbox for Designers. Berlin: Springer.
Guo, J. L., and Lee, V. M. (2011). Seeding of normal Tau by pathological Tau conformers drives pathogenesis of Alzheimer-like tangles. J. Biol. Chem. 286, 15317–15331. doi: 10.1074/jbc.m110.209296
Guo, M. T., Rotem, A., Heyman, J. A., and Weitz, D. A. (2012). Droplet microfluidics for high-throughput biological assays. Lab Chip 12, 2146–2155. doi: 10.1039/c2lc21147e
Haley, N. J., Siepker, C., Hoon-Hanks, L. L., Mitchell, G., Walter, W. D., Manca, M., et al. (2016). Seeded amplification of chronic wasting disease prions in nasal brushings and recto-anal mucosa-associated lymphoid tissues from elk by real-time quaking-induced conversion. J. Clin. Microbiol. 54, 1117–1126. doi: 10.1128/jcm.02700-15
Hepler, R. W., Grimm, K. M., Nahas, D. D., Breese, R., Dodson, E. C., Acton, P., et al. (2006). Solution state characterization of amyloid beta-derived diffusible ligands. Biochemistry 45, 15157–15167. doi: 10.1021/bi061850f
Holmes, B. B., Devos, S. L., Kfoury, N., Li, M., Jacks, R., Yanamandra, K., et al. (2013). Heparan sulfate proteoglycans mediate internalization and propagation of specific proteopathic seeds. Proc. Natl. Acad. Sci. U.S.A. 110, E3138–E3147.
Holmes, B. B., and Diamond, M. I. (2017). Cellular models for the study of prions. Cold Spring Harb. Perspect. Med. 7:a024026. doi: 10.1101/cshperspect.a024026
Hong, Y., Meng, L., Chen, S., Leung, C. W., Da, L. T., Faisal, M., et al. (2012). Monitoring and inhibition of insulin fibrillation by a small organic fluorogen with aggregation-induced emission characteristics. J. Am. Chem. Soc. 134, 1680–1689. doi: 10.1021/ja208720a
Huang, H., Li, P., Zhang, M., Yu, Y., Huang, Y., Gu, H., et al. (2017). Graphene quantum dots for detecting monomeric amyloid peptides. Nanoscale 9, 5044–5048. doi: 10.1039/c6nr10017a
Huang, Q., Xie, J., Liu, Y., Zhou, A., and Li, J. (2017). Detecting the Formation and Transformation of Oligomers during Insulin Fibrillation by a Dendrimer Conjugated with Aggregation-Induced Emission Molecule. Bioconjug. Chem. 28, 944–956. doi: 10.1021/acs.bioconjchem.6b00665
Jeon, J. S., Chung, S., Kamm, R. D., and Charest, J. L. (2011). Hot embossing for fabrication of a microfluidic 3D cell culture platform. Biomed. Microdevices 13, 325–333. doi: 10.1007/s10544-010-9496-0
Kamande, J. W., Nagendran, T., Harris, J., and Taylor, A. M. (2019). Multi-compartment microfluidic device geometry and covalently bound Poly-D-Lysine influence neuronal maturation. Front. Bioeng. Biotechnol. 7:84. doi: 10.3389/fbioe.2019.00084
Kaminski, C. F., and Kaminski Schierle, G. S. (2016). Probing amyloid protein aggregation with optical superresolution methods: from the test tube to models of disease. Neurophotonics 3:041807. doi: 10.1117/1.nph.3.4.041807
Kaushik, A., Jayant, R. D., Tiwari, S., Vashist, A., and Nair, M. (2016). Nano-biosensors to detect beta-amyloid for Alzheimer’s disease management. Biosens. Bioelectron. 80, 273–287. doi: 10.1016/j.bios.2016.01.065
Kim, A. C., Lim, S., and Kim, Y. K. (2018). Metal ion effects on abeta and Tau aggregation. Int. J. Mol. Sci. 19:128. doi: 10.3390/ijms19010128
Kirby, B. J. (2010). Micro- and Nanoscale Fluid Mechanics : Transport in Microfluidic Devices. New York, NY: Cambridge University Press.
Knight, J. B., Vishwanath, A., Brody, J. P., and Austin, R. H. (1998). Hydrodynamic focusing on a silicon chip: mixing nanoliters in microseconds. Phys. Rev. Lett. 80, 3863–3866. doi: 10.1103/physrevlett.80.3863
Knowles, T. P., Waudby, C. A., Devlin, G. L., Cohen, S. I., Aguzzi, A., Vendruscolo, M., et al. (2009). An analytical solution to the kinetics of breakable filament assembly. Science 326, 1533–1537. doi: 10.1126/science.1178250
Knowles, T. P., White, D. A., Abate, A. R., Agresti, J. J., Cohen, S. I., Sperling, R. A., et al. (2011). Observation of spatial propagation of amyloid assembly from single nuclei. Proc. Natl. Acad. Sci. U.S.A. 108, 14746–14751. doi: 10.1073/pnas.1105555108
Kopp, M. R. G., Linsenmeier, M., Hettich, B., Prantl, S., Stavrakis, S., Leroux, J. C., et al. (2020). Microfluidic shrinking droplet concentrator for analyte detection and phase separation of protein solutions. Anal. Chem. 92, 5803–5812. doi: 10.1021/acs.analchem.9b05329
Kovacs, G. G., Lee, V. M., and Trojanowski, J. Q. (2017). Protein astrogliopathies in human neurodegenerative diseases and aging. Brain Pathol. 27, 675–690. doi: 10.1111/bpa.12536
Kuncova-Kallio, J., and Kallio, P. J. (2006). PDMS and its suitability for analytical microfluidic devices. Conf. Proc. IEEE Eng. Med. Biol. Soc. 2006, 2486–2489.
Kuo, C. J., Chiang, H. C., Tseng, C. A., Chang, C. F., Ulaganathan, R. K., Ling, T. T., et al. (2018). Lipid-modified graphene-transistor biosensor for monitoring amyloid-beta aggregation. ACS Appl. Mater. Interfaces 10, 12311–12316. doi: 10.1021/acsami.8b01917
Lasmezas, C. I., Deslys, J. P., Demaimay, R., Adjou, K. T., Hauw, J. J., and Dormont, D. (1996). Strain specific and common pathogenic events in murine models of scrapie and bovine spongiform encephalopathy. J. Gen. Virol. 77(Pt 7), 1601–1609. doi: 10.1099/0022-1317-77-7-1601
Lassus, B., Naude, J., Faure, P., Guedin, D., Von Boxberg, Y., Mannoury La Cour, C., et al. (2018). Glutamatergic and dopaminergic modulation of cortico-striatal circuits probed by dynamic calcium imaging of networks reconstructed in microfluidic chips. Sci. Rep. 8:17461.
Lee, H. U., Nag, S., Blasiak, A., Jin, Y., Thakor, N., and Yang, I. H. (2016). Subcellular optogenetic stimulation for activity-dependent myelination of axons in a novel microfluidic compartmentalized platform. ACS Chem. Neurosci. 7, 1317–1324. doi: 10.1021/acschemneuro.6b00157
Lee, S.-J. J., and Sundararajan, N. (2010). Microfabrication for Microfluidics. Boston, MA: Artech House.
Lengyel-Zhand, Z., Ferrie, J. J., Janssen, B., Hsieh, C. J., Graham, T., Xu, K. Y., et al. (2020). Synthesis and characterization of high affinity fluorogenic alpha-synuclein probes. Chem. Commun. 56, 3567–3570. doi: 10.1039/c9cc09849f
Li, H., Xie, H., Cao, Y., Ding, X., Yin, Y., and Li, G. (2013). A general way to assay protein by coupling peptide with signal reporter via supermolecule formation. Anal. Chem. 85, 1047–1052. doi: 10.1021/ac302906c
Li, S. S., Lin, C. W., Wei, K. C., Huang, C. Y., Hsu, P. H., Liu, H. L., et al. (2016). Non-invasive screening for early Alzheimer’s disease diagnosis by a sensitively immunomagnetic biosensor. Sci. Rep. 6:25155.
Li, W., Xu, Z., Xu, B., Chan, C. Y., Lin, X., Wang, Y., et al. (2017). Investigation of the subcellular neurotoxicity of amyloid-beta using a device integrating microfluidic perfusion and chemotactic guidance. Adv. Healthc. Mater. 6:1600895. doi: 10.1002/adhm.201600895
Lopes, C. D. F., Mateus, J. C., and Aguiar, P. (2018). Interfacing microfluidics with microelectrode arrays for studying neuronal communication and axonal signal propagation. J. Vis. Exp. 142:e58878.
Mao, X., Ou, M. T., Karuppagounder, S. S., Kam, T. I., Yin, X., Xiong, Y., et al. (2016). Pathological alpha-synuclein transmission initiated by binding lymphocyte-activation gene 3. Science 353:aah3374. doi: 10.1126/science.aah3374
Marek, P., Mukherjee, S., Zanni, M. T., and Raleigh, D. P. (2010). Residue-specific, real-time characterization of lag-phase species and fibril growth during amyloid formation: a combined fluorescence and IR study of p-cyanophenylalanine analogs of islet amyloid polypeptide. J. Mol. Biol. 400, 878–888. doi: 10.1016/j.jmb.2010.05.041
Masujin, K., Orru, C. D., Miyazawa, K., Groveman, B. R., Raymond, L. D., Hughson, A. G., et al. (2016). Detection of atypical H-type bovine spongiform encephalopathy and discrimination of bovine prion strains by real-time quaking-induced conversion. J. Clin. Microbiol. 54, 676–686. doi: 10.1128/jcm.02731-15
Matamoros-Angles, A., Gayosso, L. M., Richaud-Patin, Y., Di Domenico, A., Vergara, C., Hervera, A., et al. (2018). iPS Cell Cultures from a Gerstmann-Straussler-Scheinker Patient with the Y218N PRNP Mutation Recapitulate tau Pathology. Mol. Neurobiol. 55, 3033–3048. doi: 10.1007/s12035-017-0506-6
McDonald, J. C., Duffy, D. C., Anderson, J. R., Chiu, D. T., Wu, H., Schueller, O. J., et al. (2000). Fabrication of microfluidic systems in poly(dimethylsiloxane). Electrophoresis 21, 27–40. doi: 10.1002/(sici)1522-2683(20000101)21:1<27::aid-elps27>3.0.co;2-c
McDonald, J. C., and Whitesides, G. M. (2002). Poly(dimethylsiloxane) as a material for fabricating microfluidic devices. Acc. Chem. Res. 35, 491–499. doi: 10.1021/ar010110q
Meisl, G., Kirkegaard, J. B., Arosio, P., Michaels, T. C., Vendruscolo, M., Dobson, C. M., et al. (2016). Molecular mechanisms of protein aggregation from global fitting of kinetic models. Nat. Protoc. 11, 252–272. doi: 10.1038/nprot.2016.010
Meisl, G., Knowles, T. P., and Klenerman, D. (2020). The molecular processes underpinning prion-like spreading and seed amplification in protein aggregation. Curr. Opin. Neurobiol. 61, 58–64. doi: 10.1016/j.conb.2020.01.010
Meissner, R., Eker, B., Kasi, H., Bertsch, A., and Renaud, P. (2011). Distinguishing drug-induced minor morphological changes from major cellular damage via label-free impedimetric toxicity screening. Lab Chip 11, 2352–2361. doi: 10.1039/c1lc20212j
Metrick, M. A. II, Do Carmo Ferreira, N., Saijo, E., Hughson, A. G., Kraus, A., Orru, C., et al. (2019). Million-fold sensitivity enhancement in proteopathic seed amplification assays for biospecimens by Hofmeister ion comparisons. Proc. Natl. Acad. Sci. U.S.A. 116, 23029–23039. doi: 10.1073/pnas.1909322116
Moore, R. A., Taubner, L. M., and Priola, S. A. (2009). Prion protein misfolding and disease. Curr. Opin. Struct. Biol. 19, 14–22.
Morales, R., Riss, M., Wang, L., Gavin, R., Del Rio, J. A., Alcubilla, R., et al. (2008). Integrating multi-unit electrophysiology and plastic culture dishes for network neuroscience. Lab Chip 8, 1896–1905. doi: 10.1039/b802165a
Morris, A. M., Watzky, M. A., and Finke, R. G. (2009). Protein aggregation kinetics, mechanism, and curve-fitting: a review of the literature. Biochim. Biophys. Acta 1794, 375–397. doi: 10.1016/j.bbapap.2008.10.016
Moutaux, E., Charlot, B., Genoux, A., Saudou, F., and Cazorla, M. (2018). An integrated microfluidic/microelectrode array for the study of activity-dependent intracellular dynamics in neuronal networks. Lab Chip 18, 3425–3435. doi: 10.1039/c8lc00694f
Neto, E., Leitao, L., Sousa, D. M., Alves, C. J., Alencastre, I. S., Aguiar, P., et al. (2016). Compartmentalized microfluidic platforms: the unrivaled breakthrough of in vitro tools for neurobiological research. J. Neurosci. 36, 11573–11584. doi: 10.1523/jneurosci.1748-16.2016
Ng, J. M., Gitlin, I., Stroock, A. D., and Whitesides, G. M. (2002). Components for integrated poly(dimethylsiloxane) microfluidic systems. Electrophoresis 23, 3461–3473. doi: 10.1002/1522-2683(200210)23:20<3461::aid-elps3461>3.0.co;2-8
Oh, K. W., Lee, K., Ahn, B., and Furlani, E. P. (2012). Design of pressure-driven microfluidic networks using electric circuit analogy. Lab Chip 12, 515–545. doi: 10.1039/c2lc20799k
Orlandi, J. G., Stetter, O., Soriano, J., Geisel, T., and Battaglia, D. (2014). Transfer entropy reconstruction and labeling of neuronal connections from simulated calcium imaging. PLoS One 9:e98842. doi: 10.1371/journal.pone.0098842
Orru, C. D., Favole, A., Corona, C., Mazza, M., Manca, M., Groveman, B. R., et al. (2015). Detection and discrimination of classical and atypical L-type bovine spongiform encephalopathy by real-time quaking-induced conversion. J. Clin. Microbiol. 53, 1115–1120. doi: 10.1128/jcm.02906-14
Orru, C. D., Groveman, B. R., Hughson, A. G., Manca, M., Raymond, L. D., Raymond, G. J., et al. (2017). RT-QuIC assays for prion disease detection and diagnostics. Methods Mol. Biol. 1658, 185–203. doi: 10.1007/978-1-4939-7244-9_14
Osaki, T., Uzel, S. G. M., and Kamm, R. D. (2020). On-chip 3D neuromuscular model for drug screening and precision medicine in neuromuscular disease. Nat. Protoc. 15, 421–449. doi: 10.1038/s41596-019-0248-1
Otzen, D. E. (2013). Amyloid Fibrils and Prefibrillar Aggregates : Molecular and Biological Properties. Weinheim: Wiley-VCH Verlag GmbH & Co.
Park, J., Koito, H., Li, J., and Han, A. (2009). Microfluidic compartmentalized co-culture platform for CNS axon myelination research. Biomed. Microdevices 11, 1145–1153. doi: 10.1007/s10544-009-9331-7
Park, J., Lee, B. K., Jeong, G. S., Hyun, J. K., Lee, C. J., and Lee, S. H. (2015). Three-dimensional brain-on-a-chip with an interstitial level of flow and its application as an in vitro model of Alzheimer’s disease. Lab Chip 15, 141–150. doi: 10.1039/c4lc00962b
Park, J., Wetzel, I., Marriott, I., Dreau, D., D’avanzo, C., Kim, D. Y., et al. (2018). A 3D human triculture system modeling neurodegeneration and neuroinflammation in Alzheimer’s disease. Nat. Neurosci. 21, 941–951. doi: 10.1038/s41593-018-0175-4
Park, J. W., Vahidi, B., Taylor, A. M., Rhee, S. W., and Jeon, N. L. (2006). Microfluidic culture platform for neuroscience research. Nat. Protoc. 1, 2128–2136. doi: 10.1038/nprot.2006.316
Park, M. C., Kim, M., Lim, G. T., Kang, S. M., An, S. S., Kim, T. S., et al. (2016). Droplet-based magnetic bead immunoassay using microchannel-connected multiwell plates (muCHAMPs) for the detection of amyloid beta oligomers. Lab Chip 16, 2245–2253. doi: 10.1039/c6lc00013d
Peng, C., Trojanowski, J. Q., and Lee, V. M. (2020). Protein transmission in neurodegenerative disease. Nat. Rev. Neurol. 16, 199–212.
Perez, M., Avila, J., and Hernandez, F. (2019). Propagation of Tau via extracellular vesicles. Front. Neurosci. 13:698. doi: 10.3389/fnins.2019.00698
Pfammatter, M., Andreasen, M., Meisl, G., Taylor, C. G., Adamcik, J., Bolisetty, S., et al. (2017). Absolute quantification of amyloid propagons by digital microfluidics. Anal. Chem. 89, 12306–12313. doi: 10.1021/acs.analchem.7b03279
Polanco, J. C., and Gotz, J. (2015). No full admission for tau to the exclusive prion club yet. EMBO J. 34, 2990–2992. doi: 10.15252/embj.201593311
Polanco, J. C., Li, C., Durisic, N., Sullivan, R., and Gotz, J. (2018). Exosomes taken up by neurons hijack the endosomal pathway to spread to interconnected neurons. Acta Neuropathol. Commun. 6:10.
Prabhulkar, S., Piatyszek, R., Cirrito, J. R., Wu, Z. Z., and Li, C. Z. (2012). Microbiosensor for Alzheimer’s disease diagnostics: detection of amyloid beta biomarkers. J. Neurochem. 122, 374–381. doi: 10.1038/s41576-018-0011-4
Prots, I., Grosch, J., Brazdis, R. M., Simmnacher, K., Veber, V., Havlicek, S., et al. (2018). alpha-Synuclein oligomers induce early axonal dysfunction in human iPSC-based models of synucleinopathies. Proc. Natl. Acad. Sci. U.S.A. 115, 7813–7818. doi: 10.1073/pnas.1713129115
Prusiner, S. B. (2017). Prion Biology : A Subject Collection from Cold Spring Harbor Perspectives in Biology. Cold Spring Harbor, NY: Cold Spring Harbor Laboratory Press.
Prusiner, S. B., Woerman, A. L., Mordes, D. A., Watts, J. C., Rampersaud, R., Berry, D. B., et al. (2015). Evidence for alpha-synuclein prions causing multiple system atrophy in humans with parkinsonism. Proc. Natl. Acad. Sci. U.S.A. 112, E5308–E5317.
Ramirez-Alvarado, M., Kelly, J. W., and Dobson, C. M. (2010). Protein Misfolding Diseases : Current and Emerging Principles and Therapies. Hoboken, NJ: Wiley.
Rauch, J. N., Luna, G., Guzman, E., Audouard, M., Challis, C., Sibih, Y. E., et al. (2020). LRP1 is a master regulator of tau uptake and spread. Nature 580, 381–385. doi: 10.1038/s41586-020-2156-5
Rhee, S. W., Taylor, A. M., Tu, C. H., Cribbs, D. H., Cotman, C. W., and Jeon, N. L. (2005). Patterned cell culture inside microfluidic devices. Lab Chip 5, 102–107. doi: 10.1039/b403091e
Robertson, G., Bushell, T. J., and Zagnoni, M. (2014). Chemically induced synaptic activity between mixed primary hippocampal co-cultures in a microfluidic system. Integr. Biol. 6, 636–644. doi: 10.1039/c3ib40221e
Rodriguez-Villarreal, A. I., Arundell, M., Carmona, M., and Samitier, J. (2010). High flow rate microfluidic device for blood plasma separation using a range of temperatures. Lab Chip 10, 211–219. doi: 10.1039/b904531g
Ruiz, A., Joshi, P., Mastrangelo, R., Francolini, M., Verderio, C., and Matteoli, M. (2014). Testing Abeta toxicity on primary CNS cultures using drug-screening microfluidic chips. Lab Chip 14, 2860–2866. doi: 10.1039/c4lc00174e
Rushworth, J. V., Ahmed, A., Griffiths, H. H., Pollock, N. M., Hooper, N. M., and Millner, P. A. (2014). A label-free electrical impedimetric biosensor for the specific detection of Alzheimer’s amyloid-beta oligomers. Biosens. Bioelectron. 56, 83–90. doi: 10.1016/j.bios.2013.12.036
Saar, K. L., Muller, T., Charmet, J., Challa, P. K., and Knowles, T. P. J. (2018). Enhancing the resolution of micro free flow electrophoresis through spatially controlled sample injection. Anal. Chem. 90, 8998–9005. doi: 10.1021/acs.analchem.8b01205
Saar, K. L., Yates, E. V., Muller, T., Saunier, S., Dobson, C. M., and Knowles, T. P. J. (2016). Automated ex situ assays of amyloid formation on a microfluidic platform. Biophys. J. 110, 555–560. doi: 10.1016/j.bpj.2015.11.3523
Saborio, G. P., Permanne, B., and Soto, C. (2001). Sensitive detection of pathological prion protein by cyclic amplification of protein misfolding. Nature 411, 810–813. doi: 10.1038/35081095
Sackmann, E. K., Fulton, A. L., and Beebe, D. J. (2014). The present and future role of microfluidics in biomedical research. Nature 507, 181–189. doi: 10.1038/nature13118
Saeed, S. M., and Fine, G. (1967). Thioflavin-T for amyloid detection. Am. J. Clin. Pathol. 47, 588–593.
Saijo, E., Groveman, B. R., Kraus, A., Metrick, M., Orru, C. D., Hughson, A. G., et al. (2019). Ultrasensitive RT-QuIC Seed Amplification Assays for Disease-Associated Tau, alpha-Synuclein, and Prion Aggregates. Methods Mol. Biol. 1873, 19–37. doi: 10.1007/978-1-4939-8820-4_2
Sano, K., Atarashi, R., Satoh, K., Ishibashi, D., Nakagaki, T., Iwasaki, Y., et al. (2018). Prion-like seeding of misfolded alpha-synuclein in the brains of dementia with lewy body patients in RT-QUIC. Mol. Neurobiol. 55, 3916–3930.
Saper, C. B., Wainer, B. H., and German, D. C. (1987). Axonal and transneuronal transport in the transmission of neurological disease: potential role in system degenerations, including Alzheimer’s disease. Neuroscience 23, 389–398. doi: 10.1016/0306-4522(87)90063-7
Sardar Sinha, M., Ansell-Schultz, A., Civitelli, L., Hildesjo, C., Larsson, M., Lannfelt, L., et al. (2018). Alzheimer’s disease pathology propagation by exosomes containing toxic amyloid-beta oligomers. Acta Neuropathol. 136, 41–56. doi: 10.1007/s00401-018-1868-1
Scheckel, C., and Aguzzi, A. (2018). Prions, prionoids and protein misfolding disorders. Nat. Rev. Genet. 19, 405–418. doi: 10.1038/s41576-018-0011-4
Scheidt, T., Lapinska, U., Kumita, J. R., Whiten, D. R., Klenerman, D., Wilson, M. R., et al. (2019). Secondary nucleation and elongation occur at different sites on Alzheimer’s amyloid-beta aggregates. Sci. Adv. 5:eaau3112. doi: 10.1126/sciadv.aau3112
Schladitz, C., Vieira, E. P., Hermel, H., and Mohwald, H. (1999). Amyloid-beta-sheet formation at the air-water interface. Biophys. J. 77, 3305–3310. doi: 10.1016/s0006-3495(99)77161-4
Scialo, C., De Cecco, E., Manganotti, P., and Legname, G. (2019). Prion and Prion-Like protein strains: deciphering the molecular basis of heterogeneity in neurodegeneration. Viruses 11:261. doi: 10.3390/v11030261
Serafin, V., Gamella, M., Pedrero, M., Montero-Calle, A., Razzino, C. A., Yanez-Sedeno, P., et al. (2020). Enlightening the advancements in electrochemical bioanalysis for the diagnosis of Alzheimer’s disease and other neurodegenerative disorders. J. Pharm. Biomed. Anal. 189, 113437. doi: 10.1016/j.jpba.2020.113437
Sethi, J., Van Bulck, M., Suhail, A., Safarzadeh, M., Perez-Castillo, A., and Pan, G. (2020). A label-free biosensor based on graphene and reduced graphene oxide dual-layer for electrochemical determination of beta-amyloid biomarkers. Mikrochim. Acta 187, 288.
Shah, R. K., and London, A. L. (1978). Laminar Flow Forced Convection in Ducts : A Source Book for Compact Heat Exchanger Analytical Data. New York, NY: Academic Press.
Shahnawaz, M., Mukherjee, A., Pritzkow, S., Mendez, N., Rabadia, P., Liu, X., et al. (2020). Discriminating alpha-synuclein strains in Parkinson’s disease and multiple system atrophy. Nature 578, 273–277.
Shembekar, N., Chaipan, C., Utharala, R., and Merten, C. A. (2016). Droplet-based microfluidics in drug discovery, transcriptomics and high-throughput molecular genetics. Lab Chip 16, 1314–1331. doi: 10.1039/c6lc00249h
Shim, J. U., Cristobal, G., Link, D. R., Thorsen, T., Jia, Y., Piattelli, K., et al. (2007). Control and measurement of the phase behavior of aqueous solutions using microfluidics. J. Am. Chem. Soc. 129, 8825–8835.
Shin, W. S., Di, J., Murray, K. A., Sun, C., Li, B., Bitan, G., et al. (2019). Different amyloid-beta self-assemblies have distinct effects on intracellular Tau aggregation. Front. Mol. Neurosci. 12:268. doi: 10.3389/fnmol.2019.00268
Sigurdsson, E. M., Calero, M., and Gasset, M. (2012). Amyloid Proteins : Methods and Protocols. New York, NY: Humana Press.
Skinner, M., Grateau, G., and Kyle, R. A. (2005). Amyloid and amyloidosis. Boca Raton, Fl: CRC Press.
Smedler, E., Malmersjo, S., and Uhlen, P. (2014). Network analysis of time-lapse microscopy recordings. Front. Neural Circuits 8:111. doi: 10.3389/fncir.2014.00111
Song, H. L., Shim, S., Kim, D. H., Won, S. H., Joo, S., Kim, S., et al. (2014). beta-Amyloid is transmitted via neuronal connections along axonal membranes. Ann. Neurol. 75, 88–97. doi: 10.1002/ana.24029
Soto, C., Saborio, G. P., and Anderes, L. (2002). Cyclic amplification of protein misfolding: application to prion-related disorders and beyond. Trends Neurosci. 25, 390–394. doi: 10.1016/s0166-2236(02)02195-1
Surmeier, D. J., Obeso, J. A., and Halliday, G. M. (2017). Selective neuronal vulnerability in Parkinson disease. Nat. Rev. Neurosci. 18, 101–113. doi: 10.1038/nrn.2016.178
Takeda, S., Wegmann, S., Cho, H., Devos, S. L., Commins, C., Roe, A. D., et al. (2015). Neuronal uptake and propagation of a rare phosphorylated high-molecular-weight tau derived from Alzheimer’s disease brain. Nat. Commun. 6:8490.
Taylor, A. M., Blurton-Jones, M., Rhee, S. W., Cribbs, D. H., Cotman, C. W., and Jeon, N. L. (2005). A microfluidic culture platform for CNS axonal injury, regeneration and transport. Nat. Methods 2, 599–605. doi: 10.1038/nmeth777
Taylor, A. M., and Jeon, N. L. (2010). Micro-scale and microfluidic devices for neurobiology. Curr. Opin. Neurobiol. 20, 640–647. doi: 10.1016/j.conb.2010.07.011
Taylor, A. M., Rhee, S. W., Tu, C. H., Cribbs, D. H., Cotman, C. W., and Jeon, N. L. (2003). Microfluidic multicompartment device for neuroscience research. Langmuir 19, 1551–1556. doi: 10.1021/la026417v
Teh, S. Y., Lin, R., Hung, L. H., and Lee, A. P. (2008). Droplet microfluidics. Lab Chip 8, 198–220.
Teller, S., Tahirbegi, I. B., Mir, M., Samitier, J., and Soriano, J. (2015). Magnetite-Amyloid-beta deteriorates activity and functional organization in an in vitro model for Alzheimer’s disease. Sci. Rep. 5:17261.
Terakawa, M. S., Lin, Y., Kinoshita, M., Kanemura, S., Itoh, D., Sugiki, T., et al. (2018). Impact of membrane curvature on amyloid aggregation. Biochim. Biophys. Acta Biomembr. doi: 10.1016/j.bbamem.2018.04.012 [Epub ahead of print].
Toprakcioglu, Z., Challa, P., Xu, C., and Knowles, T. P. J. (2019). Label-free analysis of protein aggregation and phase behavior. ACS Nano 13, 13940–13948. doi: 10.1021/acsnano.9b05552
Tran, H. T., Chung, C. H., Iba, M., Zhang, B., Trojanowski, J. Q., Luk, K. C., et al. (2014). Alpha-synuclein immunotherapy blocks uptake and templated propagation of misfolded alpha-synuclein and neurodegeneration. Cell Rep. 7, 2054–2065. doi: 10.1016/j.celrep.2014.05.033
Tran, T. M., Cater, S., and Abate, A. R. (2014). Coaxial flow focusing in poly(dimethylsiloxane) microfluidic devices. Biomicrofluidics 8:016502. doi: 10.1063/1.4863576
Tu, P. H., Galvin, J. E., Baba, M., Giasson, B., Tomita, T., Leight, S., et al. (1998). Glial cytoplasmic inclusions in white matter oligodendrocytes of multiple system atrophy brains contain insoluble alpha-synuclein. Ann. Neurol. 44, 415–422. doi: 10.1002/ana.410440324
Uemura, N., Uemura, M. T., Lo, A., Bassil, F., Zhang, B., Luk, K. C., et al. (2019). Slow progressive accumulation of oligodendroglial alpha-synuclein (alpha-Syn) pathology in synthetic alpha-Syn fibril-induced mouse models of synucleinopathy. J. Neuropathol. Exp. Neurol. 78, 877–890. doi: 10.1093/jnen/nlz070
Uemura, N., Uemura, M. T., Luk, K. C., Lee, V. M., and Trojanowski, J. Q. (2020). Cell-to-cell transmission of tau and alpha-synuclein. Trends Mol. Med. doi: 10.1016/j.molmed.2020.03.012 [Epub ahead of print].
Urrea, L., Segura-Feliu, M., Masuda-Suzukake, M., Hervera, A., Pedraz, L., Garcia Aznar, J. M., et al. (2018). Involvement of cellular prion protein in alpha-synuclein transport in neurons. Mol. Neurobiol. 55, 1847–1860. doi: 10.1007/s12035-017-0451-4
Usenovic, M., Niroomand, S., Drolet, R. E., Yao, L., Gaspar, R. C., Hatcher, N. G., et al. (2015). Internalized Tau oligomers cause neurodegeneration by inducing accumulation of pathogenic Tau in human neurons derived from induced pluripotent stem cells. J. Neurosci. 35, 14234–14250. doi: 10.1523/jneurosci.1523-15.2015
Vanni, I., Pirisinu, L., Acevedo-Morantes, C., Kamali-Jamil, R., Rathod, V., Di Bari, M. A., et al. (2020). Isolation of infectious, non-fibrillar and oligomeric prions from a genetic prion disease. Brain 143, 1512–1524. doi: 10.1093/brain/awaa078
Vilette, D., Courte, J., Peyrin, J. M., Coudert, L., Schaeffer, L., Andreoletti, O., et al. (2018). Cellular mechanisms responsible for cell-to-cell spreading of prions. Cell. Mol. Life Sci. 75, 2557–2574. doi: 10.1007/s00018-018-2823-y
Volpicelli-Daley, L. A., Luk, K. C., Patel, T. P., Tanik, S. A., Riddle, D. M., Stieber, A., et al. (2011). Exogenous alpha-synuclein fibrils induce Lewy body pathology leading to synaptic dysfunction and neuron death. Neuron 72, 57–71. doi: 10.1016/j.neuron.2011.08.033
Wang, B., Underwood, R., Kamath, A., Britain, C., Mcferrin, M. B., Mclean, P. J., et al. (2018). 14-3-3 proteins reduce cell-to-cell transfer and propagation of pathogenic alpha-synuclein. J. Neurosci. 38, 8211–8232. doi: 10.1523/jneurosci.1134-18.2018
Watts, J. C., and Prusiner, S. B. (2018). beta-Amyloid Prions and the pathobiology of Alzheimer’s disease. Cold Spring Harb. Perspect. Med. 8:a023507.
Wells, C., Brennan, S. E., Keon, M., and Saksena, N. K. (2019). Prionoid proteins in the pathogenesis of neurodegenerative diseases. Front. Mol. Neurosci. 12:271. doi: 10.3389/fnmol.2019.00271
Westergard, T., Jensen, B. K., Wen, X., Cai, J., Kropf, E., Iacovitti, L., et al. (2016). Cell-to-Cell transmission of Dipeptide Repeat Proteins Linked to C9orf72-ALS/FTD. Cell Rep. 17, 645–652. doi: 10.1016/j.celrep.2016.09.032
Whitesides, G. M., Ostuni, E., Takayama, S., Jiang, X., and Ingber, D. E. (2001). Soft lithography in biology and biochemistry. Annu. Rev. Biomed. Eng. 3, 335–373. doi: 10.1146/annurev.bioeng.3.1.335
Wilham, J. M., Orru, C. D., Bessen, R. A., Atarashi, R., Sano, K., Race, B., et al. (2010). Rapid end-point quantitation of prion seeding activity with sensitivity comparable to bioassays. PLoS Pathog. 6:e1001217. doi: 10.1371/journal.ppat.1008222
Winner, B., Jappelli, R., Maji, S. K., Desplats, P. A., Boyer, L., Aigner, S., et al. (2011). In vivo demonstration that alpha-synuclein oligomers are toxic. Proc. Natl. Acad. Sci. U.S.A. 108, 4194–4199.
Winston, C. N., Aulston, B., Rockenstein, E. M., Adame, A., Prikhodko, O., Dave, K. N., et al. (2019). Neuronal exosome-derived human Tau is toxic to recipient mouse neurons in vivo. J. Alzheimers Dis. 67, 541–553. doi: 10.3233/jad-180776
Woerman, A. L., Patel, S., Kazmi, S. A., Oehler, A., Lee, J., Mordes, D. A., et al. (2020). Kinetics of alpha-synuclein prions preceding neuropathological inclusions in multiple system atrophy. PLoS Pathog. 16:e1008222. doi: 10.1371/journal.ppat.1008222
Woerman, A. L., Watts, J. C., Aoyagi, A., Giles, K., Middleton, L. T., and Prusiner, S. B. (2018). alpha-Synuclein: multiple system atrophy prions. Cold Spring Harb. Perspect. Med. 8:a024588. doi: 10.1101/cshperspect.a024588
Wu, J. W., Herman, M., Liu, L., Simoes, S., Acker, C. M., Figueroa, H., et al. (2013). Small misfolded Tau species are internalized via bulk endocytosis and anterogradely and retrogradely transported in neurons. J. Biol. Chem. 288, 1856–1870. doi: 10.1074/jbc.m112.394528
Wu, J. W., Hussaini, S. A., Bastille, I. M., Rodriguez, G. A., Mrejeru, A., Rilett, K., et al. (2016). Neuronal activity enhances tau propagation and tau pathology in vivo. Nat. Neurosci. 19, 1085–1092. doi: 10.1038/nn.4328
Wurthner, F. (2020). Aggregation-Induced Emission (AIE): a historical perspective. Angew. Chem. Int. Ed. Engl. 9, 14192–14196. doi: 10.1002/anie.202007525
Xia, N., Zhou, B., Huang, N., Jiang, M., Zhang, J., and Liu, L. (2016). Visual and fluorescent assays for selective detection of beta-amyloid oligomers based on the inner filter effect of gold nanoparticles on the fluorescence of CdTe quantum dots. Biosens. Bioelectron. 85, 625–632. doi: 10.1016/j.bios.2016.05.066
Xu, Y., Martini-Stoica, H., and Zheng, H. (2016). A seeding based cellular assay of tauopathy. Mol. Neurodegener. 11:32.
Yakupova, E. I., Bobyleva, L. G., Vikhlyantsev, I. M., and Bobylev, A. G. (2019). Congo Red and amyloids: history and relationship. Biosci. Rep. 39:BSR20181415.
Yang, I. H., Gary, D., Malone, M., Dria, S., Houdayer, T., Belegu, V., et al. (2012). Axon myelination and electrical stimulation in a microfluidic, compartmentalized cell culture platform. Neuromol. Med. 14, 112–118. doi: 10.1007/s12017-012-8170-5
Yang, Y., Li, S., Zhang, Q., Kuang, Y., Qin, A., Gao, M., et al. (2019). An AIE-active theranostic probe for light-up detection of Abeta aggregates and protection of neuronal cells. J. Mater. Chem. B 7, 2434–2441. doi: 10.1039/c9tb00121b
Ylera, F., Lurz, R., Erdmann, V. A., and Furste, J. P. (2002). Selection of RNA aptamers to the Alzheimer’s disease amyloid peptide. Biochem. Biophys. Res. Commun. 290, 1583–1588. doi: 10.1006/bbrc.2002.6354
Zeinabad, H. A., Zarrabian, A., Saboury, A. A., Alizadeh, A. M., and Falahati, M. (2016). Interaction of single and multi wall carbon nanotubes with the biological systems: tau protein and PC12 cells as targets. Sci. Rep. 6:26508.
Zhang, J. D., Mei, J., Hu, X. L., He, X. P., and Tian, H. (2016). Ratiometric Detection of beta-Amyloid and Discrimination from Lectins by a Supramolecular AIE Glyconanoparticle. Small 12, 6562–6567. doi: 10.1002/smll.201601470
Zhang, Y., Buell, A. K., Muller, T., De Genst, E., Benesch, J., Dobson, C. M., et al. (2016). Protein aggregate-ligand binding assays based on microfluidic diffusional separation. Chembiochem 17, 1920–1924. doi: 10.1002/cbic.201600384
Zhang, Y., Ren, B., Zhang, D., Liu, Y., Zhang, M., Zhao, C., et al. (2020). Design principles and fundamental understanding of biosensors for amyloid-beta detection. J. Mater. Chem. B. 8, 6179–6196. doi: 10.1039/D0TB00344A
Zhao, Y., Li, X., Yang, Y., Si, S., Deng, C., and Wu, H. (2020). A simple aptasensor for Abeta40 oligomers based on tunable mismatched base pairs of dsDNA and graphene oxide. Biosens. Bioelectron. 149:111840. doi: 10.1016/j.bios.2019.111840
Zheng, X., Liu, P., Yang, C., and Wu, X. (2020). Amyloid protein aggregation in diabetes mellitus accelerate intervertebral disc degeneration. Med. Hypotheses 141:109739. doi: 10.1016/j.mehy.2020.109739
Zhou, J., Meng, L., Ye, W., Wang, Q., Geng, S., and Sun, C. (2018). A sensitive detection assay based on signal amplification technology for Alzheimer’s disease’s early biomarker in exosome. Anal. Chim. Acta 1022, 124–130. doi: 10.1016/j.aca.2018.03.016
Keywords: lab-on-chip, amyloid propagation, microfluidics, fibril, seeding, spreading, prion-like, prionoid
Citation: del Rio JA and Ferrer I (2020) Potential of Microfluidics and Lab-on-Chip Platforms to Improve Understanding of “prion-like” Protein Assembly and Behavior. Front. Bioeng. Biotechnol. 8:570692. doi: 10.3389/fbioe.2020.570692
Received: 08 June 2020; Accepted: 18 August 2020;
Published: 08 September 2020.
Edited by:
Maria Lurdes Pinto, University of Trás-os-Montes and Alto Douro, PortugalReviewed by:
Byron Caughey, Rocky Mountain Laboratories (NIAID), United StatesVincent Beringue, Institut National de Recherche pour l’Agriculture, l’Alimentation et l’Environnement (INRAE), France
Copyright © 2020 del Rio and Ferrer. This is an open-access article distributed under the terms of the Creative Commons Attribution License (CC BY). The use, distribution or reproduction in other forums is permitted, provided the original author(s) and the copyright owner(s) are credited and that the original publication in this journal is cited, in accordance with accepted academic practice. No use, distribution or reproduction is permitted which does not comply with these terms.
*Correspondence: Jose A. del Rio, jadelrio@ibecbarcelona.eu; jadelrio@ub.edu; Isidre Ferrer, 8082ifa@gmail.com