- Department of Preventive Medicine, Institute of Biomedical Informatics, Cell Signal Transduction Laboratory, Bioinformatics Center, Henan Provincial Engineering Center for Tumor Molecular Medicine, School of Software, School of Basic Medical Sciences, Henan University, Kaifeng, China
Gastric cancer (GC) is the second leading cause of cancer mortality and remains the fourth common cancer worldwide. The effective and feasible methods for predicting the possible outcomes for GC patients are still lacking. While genetic profiling might be suitable in some way, the application of gene expression signatures has been show to be a robust tool. Here, by performing a comprehensive search in PubMed, we provided an up-to-date summary of 39 prognostic gene signatures for GC patients, and described the processing procedure of the selection, calculation and construction of gene signature. We also reviewed current web tools including PROGgene and SurvExpress that can be used to analyze the prognostic value of multiple genes for GC. This review will aid in comprehensive understanding of the current prognostic gene signatures to accurately predict the outcome of GC patients, and may guide the future clinical management when the reliability of these signatures is validated in clinics.
Introduction
Gastric cancer (GC) is one of the leading causes of cancer-related death in many countries. In China, GC is identified as the second-leading risk for cancer-related lethality, ranking the second in frequent malignancy for male and the third for female (Chen et al., 2016). Due to poor diet habits, the Helicobacter pylori (Hp) infection and insufficient early endoscopic screening techniques, the GC incidence and mortality rates remain highin China (Li and Kaminishi, 2009). It is estimated that there are 221,478 GC patient deaths in China every year, roughly half of the world's gastric cancer deaths in 2012 (Strong et al., 2015). Nowadays outcomes of GC patients are still undesirable poor even with the advancement in surgery, chemotherapy, and radiotherapy (Peng et al., 2018), the overall 5-year GC survival rate is below 30% (Miller et al., 2016). However, the effective methods for predicting the outcome for the purpose of timely appropriate intervention for GC patients are still lacking (Yin et al., 2013). Thus, there is high clincial demand on valid biomarkers to assess in advance patient prognosis and tailor patient management (Yu et al., 2019).
Several single molecules have been reported to be related with GC patients' outcomes and peritoneal relapse (Taniguchi et al., 1998; Ishii et al., 2000). For example, the over-expressed HER2 gene was found to be associated with the lymph gland metastasis of GC (Hecht et al., 2016). P53 was proved to be an unfavorable biomarker of GC (Wang et al., 2016). However, the single biomarker applied in prognosis is less robust relative than the multiple biomarker-based models (He and Zuo, 2019). Many studies have demonstrated that signatures with an optimal combination of several candidate biomarkers could note worthily improve the predictive accuracy (Hu et al., 2019). Hence, gene signatures comprised of multiple genes are developed to strengthen the ability in predicting prognosis of cancer patients. Several gene signatures have been constructed to accurately predict the GC prognosis (Cho et al., 2011; Wang et al., 2016, 2017a; Hou et al., 2017; Lee et al., 2017). In 2005, Chen et al. developed a prognostic model using three genes based on gene expression profiles of primary GC tumor samples and adjacent mucosas (Chen et al., 2005). In 2007, Marchet et al. constructed a model with three genes to predict the lymph node involvement of cancer cells in a cohort of 32 primary gastric carcinoma patients (Marchet et al., 2007). Consequently, the goal of our current study was to perform a comprehensive systematic review for published GC prognostic signatures derived from the genome-wide studies. In this study, we carried out a systematic review of reported prognostic signatures for GC, and identified 39 gastric cancer prognostic signatures. We also summarized 3 universal strategies of signature selections, calculations and constructions. Furthermore, the web tools for the prognosis assessment of gene signatures in GC were introduced and discussed.
Materials and Methods
Selection Criteria of Studies
To identify the published gastric cancer prognosis signatures, we firstly searched in NCBI PubMed database using both MeSH terms and entry terms of “prognostic AND gene expression signature AND gastric cancer.” We also checked recent reports and reviews on this topic. In this study, we considered and selected signatures that were derived from mRNA expression profiling studies and were proven to be related to patients' survival outcomes by independent validation (Figure 1A).
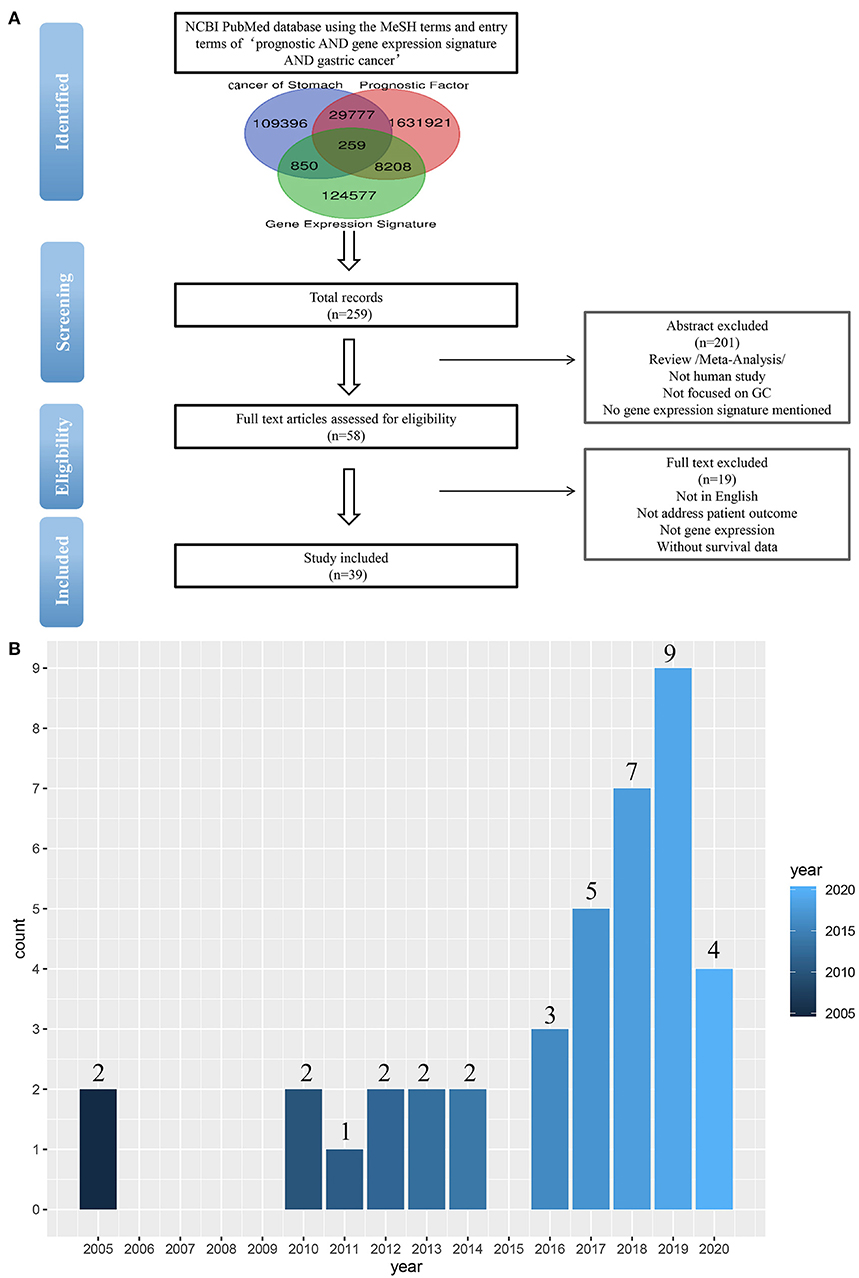
Figure 1. (A) Flow-chart diagram of literature collection (n = number of records). (B) The 39 studies published between 2005 and 2020.
As a result of our search, a total of 259 studies were obtained by removing the duplication. Then we excluded some studies rigorously according to our criteria. The criteria of exclusion were as follows: (i) Review/Meta-Analysis; (ii) No human study/Not focused on GC; (iii) No gene-expression signature mentioned /Not address patient outcome; (iv) Without survival data. Finally, 39 signatures were collected from 39 studies published between 2005 and 2020 (Figure 1B).
Statistical Collection
Two distinct survival association metrics were applied in evaluation of each prognostic model: (i) Hazard ratios (HRs) estimated by the Cox proportional-hazards regression model; (ii) The time-dependent receiver-operating characteristics (ROC) curves. Related information was listed in Table 1. We also provide the name and number of genes in each signature in Table 1 to facilitate the selection of the signatures for potential clinical application.
Results
Elaborate search results of prognostic signatures in GC were shown in Table 1 and Figure 2 (Chen et al., 2005; Motoori et al., 2005; Xu et al., 2009; Takeno et al., 2010; Cho et al., 2011; Bauer et al., 2012; Kim et al., 2012; Wang et al., 2013, 2017b, 2018; Lee et al., 2014; Pasini et al., 2014; Li et al., 2016; Zhao et al., 2016, 2019; Hou et al., 2017; Kuang et al., 2017; Lafrenie et al., 2017; Liu et al., 2018, 2019; Peng et al., 2018, 2020; Smyth et al., 2018; Wu et al., 2018; Yuzhalin et al., 2018; Chang and Lai, 2019; Chang et al., 2019; Dai et al., 2019; Jiang et al., 2019, 2020; Song et al., 2019; Bai et al., 2020; Guan et al., 2020). Briefly, we got 39 literatures in NCBI PubMed Database following the above procedure (Figure 1). In total, 39 published gastric cancer prognostic signatures were identified, which were reported to be associated with patients' overall survival (OS), disease free survival (DFS) or recurrence-free survival (RFS) with 3 favorable signatures and 36 unfavorable signatures from 259 related articles in NCBI Pubmed. These signatures were constructed from diverse technological process, and we divided their construction methods into three strategies (I, II, and III), including basing on differentially expression genes (DEGs), specific cellular pathway and molecular family. For example, Jin et al. established a 13-mRNA (DCLK1, FLRT2, MCC, PRICKLE1, RIMS1, SLC25A15, SLCO2A1, CDO1, GHR, CD109, SELP, UPK1B, and CD36) signature by which patients with higher risk score showed worse survival in both TCGA and GEO datasets (Peng et al., 2018). Wu et al. constructed a three gene signature (IHH, PTCH1, and SMO) based on the hedgehog signaling pathway as the gene model which was shown to be a unfavorable independent prognostic indicator (Wu et al., 2018). Bauer et al. constructed a novel signature (GSK3B, CTNNB1, and NOTCH2), a strong predictor for favorable outcomes with GSK3Bhigh, CTNNB1high and NOTCH2low (Bauer et al., 2012).
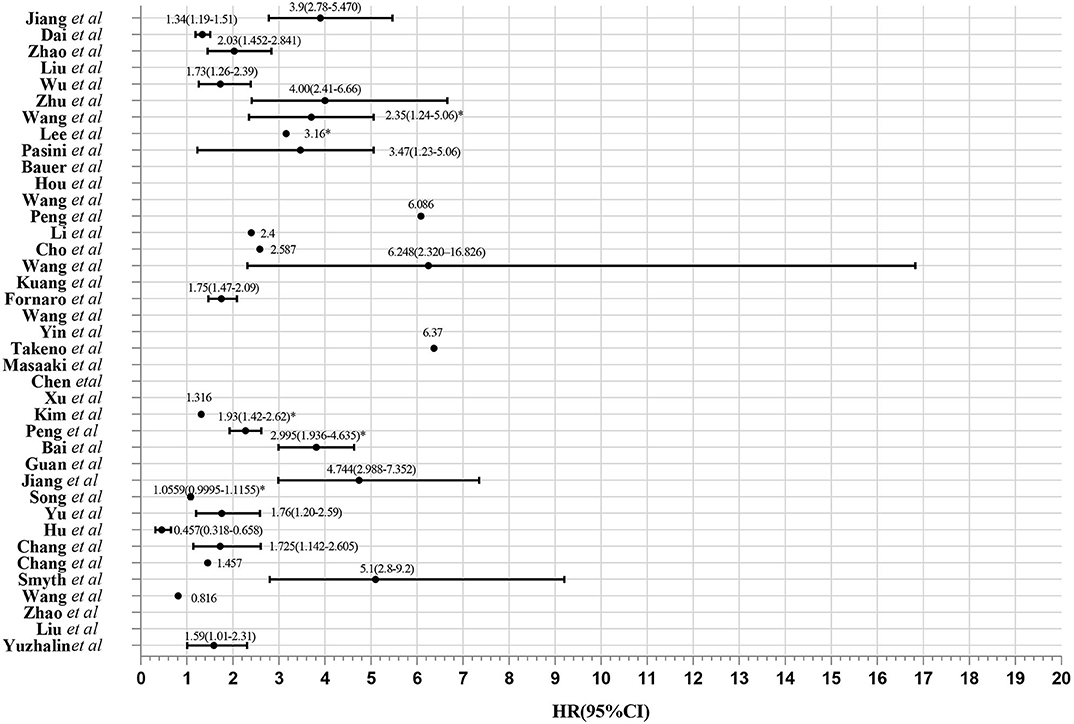
Figure 2. Evaluation of 39 gene expression signatures via Meta-analysis. HR from validation group is marked with *.
The Selection and Calculation of Multi-Gene Signature
Through analyzing these 39 articles, we summarized three common strategies to construct gene signatures (Figures 3–5). The major difference in developing signatures is the source of potential genes. Strategy I refers to finding differentially expressed genes (DEGs) and selecting candidate genes through univariate and multivariate Cox regression analysis. Strategy II focuses on one gene group of typical pathways, like Hedgehog signaling pathway-associated genes, then filtering genes. Strategy III means to obtain potential genes from molecular family and construct signatures based on their subtypes.
Gene Signature Based on DEGs With High Prognostic Values
In Strategy I, authors established gene signatures based on DEGs with high prognostic values. Based on DEGs derived from Cancer Genome Atlas (TCGA), Dai et al. constructed a 13-mRNA signature to predict the prognosis of GC patients (Dai et al., 2019). Liu et al. identified nine hub genes and constructed a 9-gene signature based on gene expression profiling datasets by integrated bioinformatics analysis (Liu et al., 2018). Masaaki et al. selected only genes specifically expressed in gastric tissues from clinical samples and established a 29-gene signature (Motoori et al., 2005). The main steps of the workflow of strategy I were as follows: (i) Identification of DEGs associated with GC survival; (ii) Selection of key candidate mRNAs for the validation; (iii) Risk score model construction; (iv) Validation of risk score model. The overall process is presented in Figure 3.
For example, Liu et al. identified nine hub genes and constructed a nine-gene signature based on gene expression profiling datasets by integrated bioinformatics analysis (Liu et al., 2018). They firstly screened differentially expressed genes (DEGs) using microarray and RNA sequencing data and conducted certain integrated analysis, including functional enrichment for identifying the potential key genes involving the pathogenesis and prognosis of GC. Then these key genes that significantly correlated with patients' survival were regarded as candidate prognostic genes by univariate and multivariate Cox analysis. Finally, a prognostic gene signature was developed according to a linear combination of gene expression values multiplied by a regression coefficient (β) accessed from the multivariate Cox proportional hazards regression model of each gene. The formula is as follows: Risk score = β1X1 + β2X2 + β3X3 +…βnXn (Liu et al., 2018). In order to validate the prognostic power of the signature, all the patients were divided into low- or high-risk groups according to the median prognostic score. Then a time-dependent ROC curve analysis was performed to calculate the predictive ability of the gene signature for clinical outcomes.
Signature Constructed With Prognosis Related Pathway
In this strategy, authors established gene signatures from a specific gene group from typical cellular pathway. Peng et al. identified an Immune-Based Prognostic Signature (Peng et al., 2020). Bai et al. constructed six-gene signature based on DNA methylation-driven genes (Bai et al., 2020). Hu et al. established a metastasis-related gene (DMGs) signature that predicting prognosis in patients with gastric cancer (Hu et al., 2019). Chang et al. identified a core Wnt signature of 16 genes associated with Wnt signaling (Chang and Lai, 2019). The main steps of the workflow of strategy II were as follows: (i) Identification of DEGs in GC; (ii) Identification of a typical pathway-related genes in GC; (iii) Genes selected in the previous step were integrated to constructed a gene signature; (iv) The Cox proportional hazards model was applied to test their association with overall survival; (v) Validation of the risk score model; The overall process is presented in Figure 4.
For example, Wu et al. identified and validated a Hedgehog (Hh) pathway-based 3-gene prognostic signature for gastric cancer (Wu et al., 2018). They first analyzed the prognostic values of 9 canonical Hh signaling pathway-associated genes for GC patients. Three members IHH, PTCH1, and SMO were identified to have significant prognostic value at cutoff values. Based on the established cutoff value, patients were divided into subgroups with high- or low-risk respectively, and univariate Cox proportional-hazards regression analysis was carried out to calculate the coefficient for each of the three Hh-associated biomarkers. Subsequently, the prognostic risk for each cancer case was scored by summing the coefficient-weighted expression of the IHH-PTCH1-SMO signature as follows: 3-gene signature score = (0.553*IHH value) + (0.457*PTCH1 value) + (0.411*SMO value) (Wu et al., 2018). To validate the prognostic Hh gene signature, another GEO dataset was used as validating data set. In particular, they also performed independent validation of the prognostic significance by immunohistochemistry (IHC).
Signature Construction With a Specific Gene Family
In the strategy III, authors assessed the prognostic value of a specific gene family with updated public resources and integrative bioinformatics analysis. Yu et al. investigated the biological and prognostic values of the NDRG family in GC (Yu et al., 2019). Chang et al. examined the prognostic significance of oxygen-sensing genes from the 2-oxoglutarate-dependent oxygenase family (Chang et al., 2019). The main steps of the strategy III were as follows: (i) Target on a specific gene family; (ii) Analyzing of prognostic values of the gene family with different clinic pathological features; (iii) Constructing a prognostic model; (iv) Validation of the prognostic model. The overall process is presented in Figure 5.
For example, the N-myc downstream-regulated gene (NDRG) family, NDRG1-4 has been involved in a wide spectrum of biological functions in multiple cancers. From this perspective, Yu et al. firstly investigated the mRNA of the NDRG family was investigated in The Cancer Genome Atlas (TCGA). For each individual in the GC data of TCGA, a prognostic risk score was computed based on the risk score equation based on the score value with an optimal cutoff. High/low-risk groups were determined by the algorithm of the prognostic risk score. Finally, the low-risk group displayed a significantly favorable survival outcome than the high-risk group (Yu et al., 2019).
Web Tools for the Prognosis Assessment of Gene Signature in Gastric Cancer
With advances in high-throughput techniques, a big volume of omics data were generated by next generation sequencing and gene microarray platforms, and these data have been deposited into public databases and can be leveraged to identify prognostic markers in different cancer types. Bioinformaticians have developed a number of online web tools for prognosis analysis such as OSkirc (Xie et al., 2019), OSlms (Wang et al., 2019), OSacc (Xie et al., 2019), OSblca (Zhang et al., 2019), OSuvm (Wang et al., 2019), PROGgene (Goswami and Nakshatri, 2013), SurvExpress (Aguirre-Gamboa et al., 2013), and KM plotter (Györffy et al., 2010). However, only two web servers including PROGgene and SurvExpress can be used to analyze the prognostic value of multiple genes as a signature.
In 2013, Goswami et al. implemented PROG gene, a survival analysis web tool based on gene expression for multiple cancer types. In 2014, they presented the second version of PROG gene, PROG geneV2, which has several enhancements over the previous version (Goswami and Nakshatri, 2014). In the PROGgeneV2, users can create the KM plots for published/curated gene signatures. PROGgeneV2 encompassed nearly ten thousand published/curated gene signatures from Molecular signature database to its repository. Users can directly search the keywords of gene signatures, and the application will retrieve genes included in the gene signature from the Molecular Signature Database. At last, a combined plot using mean of the expression of all genes in the signature will be presented for the entire signature (Goswami and Nakshatri, 2014).
In 2013, Raul et al. established SurvExpress, a web tool that performs the prognostic analysis of biomarkers and risk assessment for pan-cancers (Aguirre-Gamboa et al., 2013). SurvExpress can perform the assessment of single/multi-gene biomarkers in cancer. The prognostic index (PI, also called the risk score) is commonly utilized to stratify risk groups. Two methods are applied to generate risk groups in SurvExpress. The first method generates the risk groups by splitting the ordered PI (higher values for higher risk) with the median. The second method generates risk groups using an optimization algorithm from the ordered PI. A log-rank test was analyzed along all values of the arranged PI for two groups, and then the algorithm chooses the split point where the p-value is minimum.
Discussion
In this study, we provided a systematic review of prognostic gene signature in gastric cancer patients. The purpose of this study is to provide a gene list for further prospective clinical application, but not to restate the procedures and results of the original publications. Compared to previous studies, our study completed the following: (i) Performing a systematic literature search for GC prognostic signatures and yielding a comprehensive signature collection; (ii) Extracting three common strategies to construct novel gene signatures; (iii) Reviewing current web tools including PROGgene and SurvExpress that can be used to analyze the prognostic value of multiple genes for GC. It is also necessary to consider and integrate with other types of gene signatures for GC prognosis, such as miRNA and lncRNA signatures (Cheng, 2018; Chen et al., 2019). Although we have summarized three general strategies to construct gene signatures, other solutions should also be mentioned. For example, Lukas et al. used different gene expression patterns of GSK3B, CTNNB1, and NOTCH2 as a risk score to instead of using an equation to make risk score quantified. GSK3Bhigh, CTNNB1high, and NOTCH2low was linked with better outcomes (Bauer et al., 2012).
Chang et al. identified two signatures each consisting of a 5 genes, Signature 1 (KDM8, KDM6B, P4HTM, ALKBH4, ALKBH7) and signature 2 (KDM3A, P4HA1, ASPH, PLOD1, PLOD2), which can be used to predicting the OS in ten types of cancer patients (Chang et al., 2019). Yuzhalin et al. analyzed the extracellular matrix (ECM) genes significantly upregulated across a large cohort of patients with ovarian, lung, gastric and colon cancers and defined a nine-gene signature which was associated with poor prognosis in these cancer patients (Yuzhalin et al., 2018). Interestingly, these two literatures are both summarized in the strategy III. This might imply that the gene established by method III also has a good evaluation effect on the prognosis of other diverse types of cancer.
As well, we conducted a search in genes of 39 signatures to find the most overlapped gene. Three genes (COL1A1, COL1A2, EGFR) were used three times to construct signature in these articles, which indicated that they may be more powerful in GC prognosis and deserve to be noticed in GC prognostic gene signature construction in the future.
Notably, several drawbacks need to be discussed for these 39 gene signatures. For example, the limited number of clinical samples might affect there producibility of the prognostic signature, including more independent datasets from a certain or different race/ethnicity for cross-validation would improve the reliability of signature. Since GC incidences and survival outcomes differ significantly between Western and Asian countries (Macdonald, 2011). Zhu et al. found that their signature built based on Chinese patients is hard to be validated in the patients from other areas (Zhu et al., 2018). General signature research would also be limited by its retrospective study. The absent prospective study leads to low authenticity and acceptance of signatures in clinics. In a word, the combination of risk score and prospective randomized trials is of great necessities, in the hope that the true relevance of the risk score could be validated in the future study.
Data Availability Statement
All datasets generated for this study are included in the article/Supplementary Material.
Author Contributions
XG and LX: study concept and design. LX, LC, FW, LZ, and QW: acquisition of data. QW, LX, LC, and FW: analysis and interpretation of data. LX, LC, FW, and XG: draft of the manuscript and critical revision of the manuscript for intellectual content. All authors contributed to the article and approved the submitted version.
Funding
This study was supported by National Natural Science Foundation of China (No. 81801569), Program for Innovative Talents of Science and Technology in Henan Province (No. 18HASTIT048), China Postdoctoral Science Foundation (No. 2017M62237), Henan Postdoctoral Foundation (No. 001702052), Program for Science and Technology Development in Henan Province (Nos. 162102310391, 172102210187, and 202102310205), Program for Scientific and Technological Research of Henan Education Department (No. 14B520022), Kaifeng Science and Technology Major Project (No. 18ZD008), and Supporting grant of Bioinformatics Center of Henan University (No. 2018YLJC01).
Conflict of Interest
The authors declare that the research was conducted in the absence of any commercial or financial relationships that could be construed as a potential conflict of interest.
Supplementary Material
The Supplementary Material for this article can be found online at: https://www.frontiersin.org/articles/10.3389/fbioe.2020.00805/full#supplementary-material
Figure S1. Forest plot of the risk ratio for rates of survival on 39 gene signatures in GC.
References
Aguirre-Gamboa, R., Gomez-Rueda, H., Martínez-Ledesma, E., Martínez-Torteya, A., Chacolla-Huaringa, R., Rodriguez-Barrientos, A., et al. (2013). SurvExpress: an online biomarker validation tool and database for cancer gene expression data using survival analysis. PLoS ONE 8:e74250. doi: 10.1371/journal.pone.0074250
Bai, Y., Wei, C., Zhong, Y., Zhang, Y., Long, J., Huang, S., et al. (2020). Development and validation of a prognostic nomogram for gastric cancer based on DNA methylation-driven differentially expressed genes. Int. J. Biol. Sci. 16, 1153–1165. doi: 10.7150/ijbs.41587
Bauer, L., Langer, R., Becker, K., Hapfelmeier, A., Ott, K., Novotny, A., et al. (2012). Expression profiling of stem cell-related genes in neoadjuvant-treated gastric cancer: a NOTCH2, GSK3B and beta-catenin gene signature predicts survival. PLoS ONE 7:e44566. doi: 10.1371/journal.pone.0044566
Chang, W. H., Forde, D., and Lai, A. G. (2019). Dual prognostic role of 2-oxoglutarate-dependent oxygenases in ten cancer types: implications for cell cycle regulation and cell adhesion maintenance. Cancer Commun. 39:23. doi: 10.1186/s40880-019-0369-5
Chang, W. H., and Lai, A. G. (2019). Pan-cancer genomic amplifications underlie a WNT hyperactivation phenotype associated with stem cell-like features leading to poor prognosis. Transl. Res. 208, 47–62. doi: 10.1016/j.trsl.2019.02.008
Chen, C. N., Lin, J., Chen, J. J., Lee, H., Yang, Y., Kuo, L., et al. (2005). Gene expression profile predicts patient survival of gastric cancer after surgical resection. J. Clin. Oncol. 23, 7286–7295. doi: 10.1200/JCO.2004.00.2253
Chen, J., Hu, B., Wang, W., Qian, X. J., Shan, B. J., and He, Y. F. (2019). A six-microRNA signature to predict outcomes of patients with gastric cancer. FEBS Open Bio 9, 538–547. doi: 10.1002/2211-5463.12593
Chen, W., Zheng, R., Baade, P. D., Zhang, S., Zeng, H., Bray, F., et al. (2016). Cancer statistics in China, 2015. CA 66, 115–132. doi: 10.3322/caac.21338
Cheng, P. (2018). A prognostic 3-long noncoding RNA signature for patients with gastric cancer. J. Cell. Biochem. 119, 9261–9269. doi: 10.1002/jcb.27195
Cho, J. Y., Lim, J. Y., Cheong, J. H., Y.-,Park, Y., S.-,Yoon, L., Kim, S. M., et al. (2011). Gene expression signature–based prognostic risk score in gastric cancer. Clin. Cancer Res. 17, 1850–1857. doi: 10.1158/1078-0432.CCR-10-2180
Dai, J., Li, Z. X., Zhang, Y., Ma, J. L., Zhou, T., You, W. C., et al. (2019). Whole genome messenger RNA profiling identifies a novel signature to predict gastric cancer survival. Clin. Transl. Gastroenterol. 10:e00004. doi: 10.14309/ctg.0000000000000004
Goswami, C. P., and Nakshatri, H. (2013). PROGgene: gene expression based survival analysis web application for multiple cancers. J. Clin. Bioinform. 3, 22–22. doi: 10.1186/2043-9113-3-22
Goswami, C. P., and Nakshatri, H. (2014). PROGgeneV2: enhancements on the existing database. BMC Cancer 14:970. doi: 10.1186/1471-2407-14-970
Guan, E., Tian, F., and Liu, Z. (2020). A novel risk score model for stomach adenocarcinoma based on the expression levels of 10 genes. Oncol. Lett. 19, 1351–1367.
Györffy, B., Lanczky, A., Eklund, A. C., Denkert, C., Budczies, J., Li, Q., et al. (2010). An online survival analysis tool to rapidly assess the effect of 22,277 genes on breast cancer prognosis using microarray data of 1,809 patients. Br. Cancer Res. Treat. 123, 725–731. doi: 10.1007/s10549-009-0674-9
He, R., and Zuo, S. (2019). A robust 8-gene prognostic signature for early-stage non-small cell lung cancer. Front. Oncol. 9:693. doi: 10.3389/fonc.2019.00693
Hecht, J. R., Bang, Y. J., Qin, S. K., Chung, H. C., Xu, J. M., Park, J. O., et al. (2016). Lapatinib in combination with capecitabine plus oxaliplatin in human epidermal growth factor receptor 2-positive advanced or metastatic gastric, esophageal, or gastroesophageal adenocarcinoma: TRIO-013/LOGiC-A randomized phase III trial. J. Clin. Oncol. 34, 443–451. doi: 10.1200/JCO.2015.62.6598
Hou, J. Y., Wang, G., Ma, J., Yang, Y., and Li, P. (2017). Identification of a prognostic 5-Gene expression signature for gastric cancer. J. Cancer Res. Clin. Oncol. 143, 619–629. doi: 10.1007/s00432-016-2324-z
Hu, B. L., Xie, M. Z., Li, K. Z., Li, J. L., Gui, Y. C., and Xu, J. W. (2019). Genome-wide analysis to identify a novel distant metastasis-related gene signature predicting survival in patients with gastric cancer. Biomed. Pharmacother. 117:109159. doi: 10.1016/j.biopha.2019.109159
Ishii, Y., Ochiai, A., Yamada, T., Akimoto, S., Yanagihara, K., Kitajima, M., et al. (2000). Integrin α6β4 as a suppressor and a predictive marker for peritoneal dissemination in human gastric cancer. Gastroenterology 118, 497–506. doi: 10.1016/S0016-5085(00)70255-1
Jiang, B., Sun, Q., Tong, Y., Wang, Y., Ma, H., Xia, X., et al. (2019). An immune-related gene signature predicts prognosis of gastric cancer. Medicine 98:e16273. doi: 10.1097/MD.0000000000016273
Jiang, H., Gu, J., Du, J., Qi, X., Qian, C., and Fei, B. (2020). A 21-gene support vector machine classifier and a 10-gene risk score system constructed for patients with gastric cancer. Mol. Med. Rep. 21, 347–359. doi: 10.3892/mmr.2019.10841
Kim, H. K., Choi, I. J., Kim, C. G., Kim, H. S., Oshima, A., Yamada, Y., et al. (2012). Three-gene predictor of clinical outcome for gastric cancer patients treated with chemotherapy. Pharmacogenom. J. 12, 119–127. doi: 10.1038/tpj.2010.87
Kuang, M., Cheng, J., Zhang, C., Feng, L., Xu, X., Zhang, Y., et al. (2017). A novel signature for stratifying the molecular heterogeneity of the tissue-infiltrating T-cell receptor repertoire reflects gastric cancer prognosis. Sci. Rep. 7:7762. doi: 10.1038/s41598-017-08289-z
Lafrenie, R. M., Fornaro, L., Vivaldi, C., Lin, D., Xue, H., Falcone, A., et al. (2017). Prognostic relevance of a T-type calcium channels gene signature in solid tumours: A correlation ready for clinical validation. PLoS ONE 12:e0182818. doi: 10.1371/journal.pone.0182818
Lee, J., Cristescu, R., K.-,Kim, M., Kim, K., Kim, S. T., Park, S. H., et al. (2017). Development of mesenchymal subtype gene signature for clinical application in gastric cancer. Oncotarget 8:66305. doi: 10.18632/oncotarget.19985
Lee, J., Sohn, I., Do, I. G., Kim, K. M., Park, S. H., Park, J. O., et al. (2014). Nanostring-based multigene assay to predict recurrence for gastric cancer patients after surgery. PLoS ONE 9:e90133. doi: 10.1371/journal.pone.0090133
Li, X., Cai, H., Zheng, W., Tong, M., Li, H., Ao, L., et al. (2016). An individualized prognostic signature for gastric cancer patients treated with 5-Fluorouracil-based chemotherapy and distinct multi-omics characteristics of prognostic groups. Oncotarget 7:8743. doi: 10.18632/oncotarget.7087
Li, Z., and Kaminishi, M. (2009). A comparison of gastric cancer between Japan and China. Gastric Cancer 12, 52–53. doi: 10.1007/s10120-008-0495-2
Liu, D., Ma, X., Yang, F., Xiao, D., Jia, Y., and Wang, Y. (2019). Discovery and validation of methylated-differentially expressed genes in Helicobacter pylori-induced gastric cancer. Cancer Gene Ther. 27, 473–485. doi: 10.1038/s41417-019-0125-7
Liu, X., Wu, J., Zhang, D., Bing, Z., Tian, J., Ni, M., et al. (2018). Identification of potential key genes associated with the pathogenesis and prognosis of gastric cancer based on integrated bioinformatics analysis. Front. Genet. 9:265. doi: 10.3389/fgene.2018.00265
Macdonald, J. S. (2011). Gastric cancer: nagoya is not New York. Am. Soc. Clin. Oncol. 29, 4348–4350. doi: 10.1200/JCO.2011.37.5691
Marchet, A., Mocellin, S., Belluco, C., Ambrosi, A., De Marchi, F., Mammano, E., et al. (2007). Gene expression profile of primary gastric cancer: towards the prediction of lymph node status. Annals Surg. Oncol. 14, 1058–1064. doi: 10.1245/s10434-006-9090-0
Miller, K. D., Siegel, R. L., Lin, C. C., Mariotto, A. B., Kramer, J. L., Rowland, J. H., et al. (2016). Cancer treatment and survivorship statistics, 2016. CA 66, 271–289. doi: 10.3322/caac.21349
Motoori, M., Takemasa, I., Yano, M., Saito, S., Miyata, H., Takiguchi, S., et al. (2005). Prediction of recurrence in advanced gastric cancer patients after curative resection by gene expression profiling. Int. J. Cancer 114, 963–968. doi: 10.1002/ijc.20808
Pasini, F. S., Zilberstein, B., Snitcovsky, I., Roela, R. A., Mangone, F. R., Ribeiro, U. Jr., et al. (2014). A gene expression profile related to immune dampening in the tumor microenvironment is associated with poor prognosis in gastric adenocarcinoma. J. Gastroenterol. 49, 1453–1466. doi: 10.1007/s00535-013-0904-0
Peng, D., Gu, B., Ruan, L., Zhang, X., and Shu, P. (2020). Integrated analysis identifies an immune-based prognostic signature for the mesenchymal identity in gastric cancer. Biomed. Res. Int. 2020:9780981. doi: 10.1155/2020/9780981
Peng, P. L., Zhou, X. Y., Yi, G. D., Chen, P. F., Wang, F., and Dong, W. G. (2018). Identification of a novel gene pairs signature in the prognosis of gastric cancer. Cancer Med. 7, 344–350. doi: 10.1002/cam4.1303
Smyth, E. C., Nyamundanda, G., Cunningham, D., Fontana, E., Ragulan, C., Tan, I. B., et al. (2018). A seven-Gene Signature assay improves prognostic risk stratification of perioperative chemotherapy treated gastroesophageal cancer patients from the MAGIC trial. Ann. Oncol. 29, 2356–2362. doi: 10.1093/annonc/mdy407
Song, L. X., Wang, Y., and He, F. X. (2019). A 5-gene prognostic combination for predicting survival of patients with gastric cancer. Med. Sci. Monitor 25, 6313–6320. doi: 10.12659/MSM.914815
Strong, V. E., Wu, A., Selby, L. V., Gonen, M., Hsu, M., Song, K. Y., et al. (2015). Differences in gastric cancer survival between the U.S., and China. J. Surg. Oncol. 112, 31–37. doi: 10.1002/jso.23940
Takeno, A., Takemasa, I., Seno, S., Yamasaki, M., Motoori, M., Miyata, H., et al. (2010). Gene expression profile prospectively predicts peritoneal relapse after curative surgery of gastric cancer. Ann. Surg. Oncol. 17, 1033–1042. doi: 10.1245/s10434-009-0854-1
Taniguchi, K., Yonemura, Y., Nojima, N., Hirono, Y., Fushida, S., Fujimura, T., et al. (1998). The relation between the growth patterns of gastric carcinoma and the expression of hepatocyte growth factor receptor (c-met), autocrine motility factor receptor, and urokinase-type plasminogen activator receptor. Cancer 82, 2112–2122. doi: 10.1002/(SICI)1097-0142(19980601)82:11<2112::AID-CNCR5>3.0.CO;2-X
Wang, J., Gao, P., Song, Y., Sun, J., Chen, X., Yu, H., et al. (2018). Prognostic value of gastric cancer-associated gene signatures: evidence based on a meta-analysis using integrated bioinformatics methods. J. Cell. Mol. Med. 22, 5743–5747. doi: 10.1111/jcmm.13823
Wang, P., Wang, Y., Hang, B., Zou, X., and Mao, H. (2016). A novel gene expression-based prognostic scoring system to predict survival in gastric cancer. Oncotarget 7:55343. doi: 10.18632/oncotarget.10533
Wang, Q., Xie, L., Dang, Y., Sun, X., Xie, T., Guo, J., et al. (2019). OSlms: a web server to evaluate the prognostic value of genes in leiomyosarcoma. Front. Oncol. 9:190. doi: 10.3389/fonc.2019.00190
Wang, X., Liu, Y., Niu, Z., Fu, R., Jia, Y., Zhang, L., et al. (2017a). Prognostic value of a 25-gene assay in patients with gastric cancer after curative resection. Sci. Rep. 7:7515. doi: 10.1038/s41598-017-07604-y
Wang, Z., Chen, G., Wang, Q., Lu, W., and Xu, M. (2017b). Identification and validation of a prognostic 9-genes expression signature for gastric cancer. Oncotarget 8, 73826–73836. doi: 10.18632/oncotarget.17764
Wang, Z., Yan, Z., Zhang, B., Rao, Z., Zhang, Y., Liu, J., et al. (2013). Identification of a 5-gene signature for clinical and prognostic prediction in gastric cancer patients upon microarray data. Med. Oncol. 30:678. doi: 10.1007/s12032-013-0678-5
Wu, J., Wang, X., and Lu, W. (2018). Identification and validation of a Hedgehog pathway-based 3-gene prognostic signature for gastric cancers. Oncol. Lett. 16, 2263–2270. doi: 10.3892/ol.2018.8945
Xie, L., Wang, Q., Dang, Y., Ge, L., Sun, X., Li, N., et al. (2019). OSkirc: a web tool for identifying prognostic biomarkers in kidney renal clear cell carcinoma. Fut. Oncol. 15, 3103–3110. doi: 10.2217/fon-2019-0296
Xu, Z., Chen, J., and Shu, Y. (2009). Gene expression profile towards the prediction of patient survival of gastric cancer. Biomed. Pharmacother. 64, 133–139. doi: 10.1016/j.biopha.2009.06.021
Yin, Y., Zhuo, W., Zhao, Y., Chen, S., Li, J., Wang, L., et al. (2013). Converting a microarray signature into a diagnostic test: a trial of custom 74 gene array for clarification and prediction the prognosis of gastric cancer. PLoS ONE 8:e81561. doi: 10.1371/journal.pone.0081561
Yu, C., Hao, X., Zhang, S., Hu, W., Li, J., Sun, J., et al. (2019). Characterization of the prognostic values of the NDRG family in gastric cancer. Therap. Adv. Gastroenterol. 12:1756284819858507. doi: 10.1177/1756284819858507
Yuzhalin, A. E., Urbonas, T., Silva, M. A., Muschel, R. J., and Gordon-Weeks, A. N. (2018). A core matrisome gene signature predicts cancer outcome. Br. J. Cancer 118, 435–440. doi: 10.1038/bjc.2017.458
Zhang, G., Wang, Q., Yang, M., Yuan, Q., Dang, Y., Sun, X., et al. (2019). OSblca: a web server for investigating prognostic biomarkers of bladder cancer patients. Front. Oncol. 9:466. doi: 10.3389/fonc.2019.00466
Zhao, L., Jiang, L., He, L., Wei, Q., Bi, J., Wang, Y., et al. (2019). Identification of a novel cell cycle-related gene signature predicting survival in patients with gastric cancer. J. Cell Physiol. 234, 6350–6360. doi: 10.1002/jcp.27365
Zhao, X., Cai, H., Wang, X., and Ma, L. (2016). Discovery of signature genes in gastric cancer associated with prognosis. Neoplasma 63, 239–245. doi: 10.4149/209_150531N303
Keywords: gene signature, gastric cancer, overall survival, prognostic biomarker, TCGA, GEO
Citation: Xie L, Cai L, Wang F, Zhang L, Wang Q and Guo X (2020) Systematic Review of Prognostic Gene Signature in Gastric Cancer Patients. Front. Bioeng. Biotechnol. 8:805. doi: 10.3389/fbioe.2020.00805
Received: 21 March 2020; Accepted: 22 June 2020;
Published: 31 July 2020.
Edited by:
Madhuchhanda Bhattacharjee, University of Hyderabad, IndiaReviewed by:
Christoph Röcken, University of Kiel, GermanyAmit Kumar Yadav, Translational Health Science and Technology Institute (THSTI), India
Copyright © 2020 Xie, Cai, Wang, Zhang, Wang and Guo. This is an open-access article distributed under the terms of the Creative Commons Attribution License (CC BY). The use, distribution or reproduction in other forums is permitted, provided the original author(s) and the copyright owner(s) are credited and that the original publication in this journal is cited, in accordance with accepted academic practice. No use, distribution or reproduction is permitted which does not comply with these terms.
*Correspondence: Xiangqian Guo, eHFndW9AaGVudS5lZHUuY24=
†These authors have contributed equally to this work