- 1West China Biomedical Big Data Center, West China Hospital, Sichuan University, Chengdu, China
- 2Vascular Surgery, West China Hospital, Sichuan University, Chengdu, China
- 3School of Information Management and Statistics, Hubei University of Economics, Wuhan, China
- 4School of Biomedical Informatics, The University of Texas Health Science Center at Houston, Houston, TX, United States
- 5Pancreatic Surgery, West China Hospital, Sichuan University, Chengdu, China
- 6Institute of Digest Surgery, West China Hospital, Sichuan University, Chengdu, China
Background: The timing of surgery for necrotizing pancreatitis remains a matter of controversial debate, which has not been resolved by randomized controlled trial (RCT). This study aims to classify surgical timing within or beyond 4 weeks for patients with infected necrotizing pancreatitis by using machine learning methods.
Methods: This study analyzed 223 patients who underwent surgery for infected pancreatic necrosis at West China Hospital of Sichuan University. We used logistic regression, support vector machine, and random forest with/without the simulation of generative adversarial networks to classify the surgical intervention within or beyond 4 weeks in the patients with infected necrotizing pancreatitis.
Results: Our analyses showed that interleukin 6, infected necrosis, the onset of fever and C-reactive protein were important factors in determining the timing of surgical intervention (< 4 or ≥ 4 weeks) for the patients with infected necrotizing pancreatitis. The main factors associated with postoperative mortality in patients who underwent early surgery (< 4 weeks) included modified Marshall score on admission and preoperational modified Marshall score. Preoperational modified Marshall score, time of surgery, duration of organ failure and onset of renal failure were important predictive factors for the postoperative mortality of patients who underwent delayed surgery (≥ 4 weeks).
Conclusions: Machine learning models can be used to predict timing of surgical intervention effectively and key factors associated with surgical timing and postoperative survival are identified for infected necrotizing pancreatitis.
Introduction
Necrotizing pancreatitis occurs in about 20% of patients suffering from acute pancreatitis (AP) (Banks, 1997). The current management guideline for necrotizing pancreatitis from IAP/APA (Working Group IAP/APA Acute Pancreatitis Guidelines, 2013) recommends delaying the timing of surgery until 4 or more weeks after initial necrotizing presentation to become walled-off shown in an addition file (Supplementary Figure 1). However, some patients with necrotizing pancreatitis will die before 4 weeks from the onset of AP. Therefore, how to identify those patients is an urgent problem to be solved. In addition to IAP/APA guideline, recommendations for surgical timing of necrotizing pancreatitis in the United States, United Kingdom, Italy, and Japan are also delayed as far as possible, without recommendations for individuals (Association et al., 2005; Tenner et al., 2013; Pezzilli et al., 2015; Yokoe et al., 2015).
Guo et al. concluded that the postoperative mortality of patients in 2 weeks with necrotizing pancreatitis was much higher than that after 2 weeks, and the prognosis of patients who did surgery before 4 weeks in necrotizing pancreatitis without persistent organ failure (POF) was same with that of patients who did surgery after 4 weeks in necrotizing pancreatitis without POF (Guo et al., 2014). A systematic review suggested that debridement should be done at least 12 days later for adult patients with necrotizing pancreatitis (Mowery et al., 2017). The first drainage time in step-up approach was 3.5–75.5 days from the onset of AP (Mowery et al., 2017). The timing of surgical intervention in necrotizing pancreatitis is controversial. A randomized controlled trial (RCT) which was established to optimize timings of surgery following PCD in patients with infected pancreatic necrosis was forced to stop early due to practical difficulties (Shenvi et al., 2016). The surgical timing problem has not been resolved by RCT.
What's more, infection and organ failure have long been used as key factors in determining whether or not to undergo surgery and are considered as the determinants of mortality for the patients with necrotizing pancreatitis. Surgical indications for the patients with necrotic pancreatitis are determined empirically among clinicians (Gomatos et al., 2015; Van Grinsven et al., 2016). A prospective study observed that POF in the first week was more likely to determine mortality than infection in patients with necrotizing pancreatitis (Guo et al., 2013). While a prospective cohort study from the Netherlands showed that there were no associations between infection, onset of organ failure, duration of organ failure and mortality in the patients with necrotizing pancreatitis (Schepers et al., 2018). These findings are inconsistent. Additionally, current studies cannot explain the relationship between the suggested surgical indications of necrotizing pancreatitis, mortality and surgical timing (Van Grinsven et al., 2016).
Nowadays, artificial intelligence (AI) is increasingly used in medicine (Nature Medicine, 2019). Therefore, we applied the machine learning and deep learning methods in AI to extract the clinical features from the patients with infected necrotizing pancreatitis who received early surgery in West China Hospital of Sichuan University and analyzed the associations between early surgical treatment, organ failure, infection and clinical predictors. We also identified the key factors associated with patients' mortality following early (<4 weeks) or late (≥4 weeks) surgery.
Materials and Methods
Patients and Treatment Protocol
A total of 223 patients (median age: 43 years old, male: 60.99%) were analyzed in this study. Those patients were hospitalized and operated due to infected necrotizing pancreatitis in West China Hospital of Sichuan University from January 2009 to June 2012. The AP was diagnosed according to the classification system of 2012 revision of the Atlanta edition, and pancreatic necrosis or peripancreatic necrosis was determined by contrast-enhanced computed tomography (CECT). Its treatment protocol was reported previously (Guo et al., 2013, 2014). The patients with severe clinical signs of persistent degeneration were operated before 4 weeks and the remaining patients were operated after 4 weeks from the onset of AP. This study was approved by the ethics review board of West China Hospital of Sichuan University, and the need for informed consent was waived owing to the retrospective nature of the study.
Clinical Data Collection
The clinical data related above patients were collected, including infection, organ failure, operation time, postoperative mortality, postoperative complications, during hospitalization of those patients, etc. The collecting procedure and definitions of the indicators were described previously (Guo et al., 2013, 2014).
Statistical Analysis
To classify surgical timing, there was nothing worthwhile to learn about a failed surgery. For example, if a patient died after surgery, we regarded this kind of case as a failed one. So, the successful surgery needed to be learned. We assumed that the best surgical timing was the actual time of a successful surgery. Based on the time from the onset of AP to surgical intervention, the patients were divided into the early (<4 weeks) and delayed (≥4 weeks) surgery groups. The baseline conditions of these patients were analyzed, including organ failure, infection, etc. T-test, and Chi-square test were used to evaluate the difference between the two groups. We then analyzed the factors that affect surgical timing and the factors associated with postoperative mortality by feature selection. Finally, we used multiple classifiers to classify the patients and compared the classifiers' performance. Variables with a p < 0.05 were considered to be statistically significant.
Three classifiers were used in this study, including logistic regression (LR), support vector machine (SVM) and random forest (RF) (Le, 2019; Le et al., 2019b). The LR is a commonly used statistic model in the healthcare industry and SVM is a popular machine learning approach. RF is a classifier that uses multiple trees to train and predict and has both features of high accuracy and balancing errors when analyzing unbalanced classification data sets. In order to find predictors of postoperative mortality at different surgical timings, in addition to feature selection and classification of surgical timing in survived patients after surgery, we performed feature selection and classification of postoperative death in the early and delayed surgery. Finally, we divided the patients into three groups based on the surgical time and mortality for classification analyses.
The survived patients after surgery (n = 186) were divided into the early group (n = 73) and the delayed group (n = 113), to predict whether surgical treatment should be performed early;
The patients received early surgery (n = 106) were divided into the death group (n = 33) and survival group (n = 73), to predict the death rate of patients after receiving an early surgery.
The patients with delayed surgery (n = 117) were divided into death group (n = 4) and survival group (n = 113), to predict the death rate of patients after delayed surgery.
To solve the problem of positive and negative sample imbalance and small sample size, which will severely affect the performance of classifiers, we used generative adversarial networks (GAN) to generate simulated samples, which had the same distributions as the real samples (Creswell et al., 2017). GAN, a recently developed deep learning approach (Goodfellow et al., 2014), shows promising simulation performances in many fields (Deshpande, 2013; Santana and Hotz, 2016; Li et al., 2017; Pascual et al., 2017), such as image synthesis, language processing, etc. Douzas and Bacao (2017) used a conditional version (referring to each category) of GAN to approximate the true data distribution and generated data for the minority class of various imbalanced datasets. To improve the effectiveness of a classifier, Fiore et al. (2017) trained a GAN model to mimic the original minority class examples and then merged the synthetic examples with training data into an augmented training set. More importantly, by using variant of GAN, Baowaly et al. (2019) have proved that GAN can adequately learn the data distribution of real electronic health records and efficiently generate realistic synthetic electronic health records. GAN is a powerful generation model (Goodfellow et al., 2014; Douzas and Bacao, 2017; Fiore et al., 2017; Wang et al., 2017). Therefore, we applied GAN to electronic medical records to investigate the timing of surgical intervention for the patients with infected necrotizing pancreatitis. In this study, the data was randomly divided into training dataset and testing dataset according to the ratio of 4:1. The real training dataset were used to train the simulated samples to optimize GAN parameters. The simulated samples generated by the GAN generator were filtered by the GAN discriminator. The simulated samples after filtration were tested by LR, SVM, and RF (Figure 1).
We used several classification indicators to determine the classification performance of our models, including accuracy, precision, recall, F1-measure and area under curve (AUC) (Le et al., 2017, 2019a). Accuracy provides a percentage of correct classification. Precision is a measurement of how many positive classifications are actual positive observations. Recall, a proportion of all real positive observations that are correct, is a measure of how many actual positive observations are classified correctly. F1-measure, the harmonic mean of precision and recall, is an “average” of both precision and recall. AUC is the area under the ROC curve. The greater the value of the indicators, the better the model performance. We combined multiple evaluation indicators to evaluate the performance of the models. The simulation for GAN was calculated in Python software and others were conducted using R software.
Results
Characteristics of Survived Patients After Early or Delayed Surgery
We compared the major organ failure in the early and delayed surgery groups as shown in an additional file (Supplementary Table 1). In general, there were no differences in POF and the number of organ failure systems between the two groups. The proportion of renal failure in the early group was higher than that in the delayed group. Onsets of renal failure and multiple organ failure in the delayed group were earlier, but the duration of organ failure was shorter. In terms of the preoperative POF, more than half of patients with POF were recovered before surgery, and the proportion of POF in the early group was higher than that in the delayed group. As shown in another additional file (Supplementary Table 2), the median time and interquartile range of surgery for the early group were 21 and 6 days and the delayed group was 37 and 21 days, respectively. More patients received continuous renal replacement therapy (CRRT) in the early group than those in the delayed group. The delayed group had a higher proportion of infected necrosis. The onset of fever in the early group was earlier than that in the delayed group. The proportion of abnormal interleukin 6 (IL-6) level in the delayed group was higher than that in the early group. There was no difference in the modified Marshall score between the two groups, but preoperational modified Marshall score was higher in the early group. The proportions of intra-abdominal bleeding and re-intervention were higher in early group. Age, length of hospital stay and gender composition ratio were similar between the two groups. There were no differences between the two groups in blood culture, sputum, white blood cell (WBC), C-reactive protein (CRP), procalcitonin (PCT), enterocutaneous fistula, and new-onset organ failure.
Predictors of Surgical Timing and Postoperative Mortality and Classification Performance
In order to classify the surgical timing (<4 or ≥4 weeks), the patients were divided into three groups as shown in the Methods based on the time of surgery and postoperative mortality, including the patients surviving after surgery (group 1), the patients received earlier surgery (group 2) and the patients underwent delayed surgery (group 3). LR, SVM, RF w/wo GAN were adopted to predict surgical timing from the three groups of patients, respectively, where LR was used as a statistical model and SVM and RF were used as machine learning models. The first group of patients was used to assess the predictors of surgical timing. The second group of patients was used for evaluating the predictors of mortality from early surgery and the third group of patients for the predictors of mortality from delayed. We used the stepwise selection procedures for the selection of independent variables (predictors) in LR. The Boruta function in R was applied to select important features in SVM, where the value of meanImp indicates the importance of a predictor. RF itself comes with a feature selection function, where the value of MeanDecreaseGini represents the importance of a feature. The larger the value, the more important it is.
The analysis results for group 1 indicated that IL-6, infected necrosis, the onset of fever and CRP were the important factors for surgical timing of patients with necrotizing pancreatitis (Table 1). The results of the following models are derived from the testing dataset. We also assessed the classification accuracy of the three models. The classified accuracy of RF (0.80) was higher than SVM (0.78) and LR (0.71). With the simulation by the GAN, the classification accuracies for all of the three models were improved. GAN-RF (accuracy: 0.89) had a better performance than GAN-SVM (0.84) and GAN-LR (0.83). The recall rates also reached 1 (Table 2).
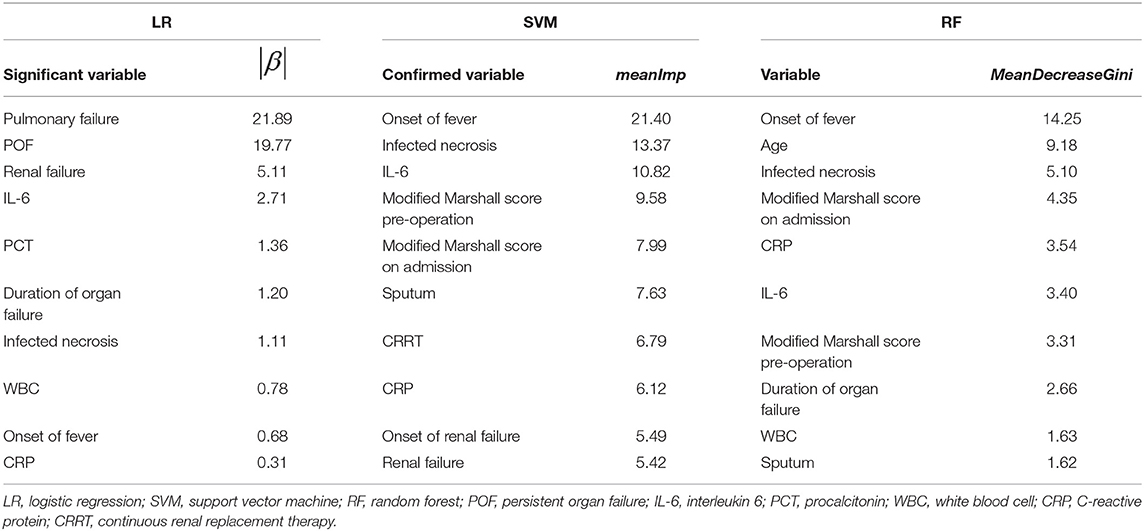
Table 1. Top important features for survived patients after early surgery (<4 weeks) compared with survived patients after delayed surgery (≥4 weeks).
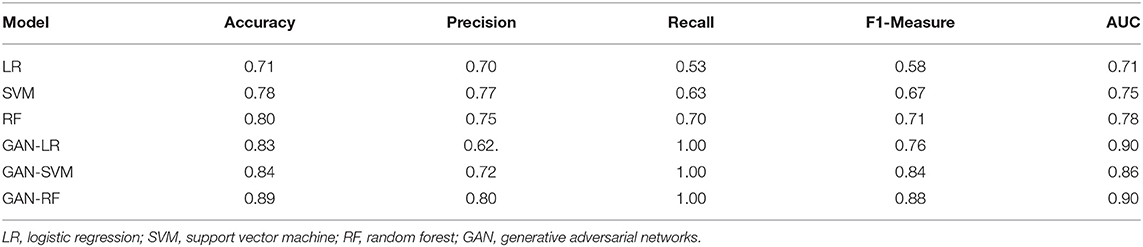
Table 2. Classification performance for survived patients after early (<4 weeks) or delayed surgery (≥4 weeks).
We assessed the key factors affecting patient mortality in the group 2 patients using LR, SVM, and FR models, respectively. As shown in the Table 3, top-ranked factors associated with patient mortality include the modified Marshall score on admission and preoperational modified Marshall score. By combining with GAN, the classification accuracies of the three models for mortality in early surgery patients were largely improved. GAN-RF (0.99) and GAN-SVM (0.99) had a better performance in evaluating the key factors than GAN-LR (0.90) (Table 4).
As shown in the Table 5, the modified Marshall score pre-operation was predicted by all three models as an important factor for the mortality of patients who underwent delayed surgery. The time of surgery, duration of organ failure and onset of renal failure were top 5-ranked features predicted by SVM and RF models (Table 6). Due to the unbalanced positive and negative samples, we simulated this group of samples using GAN first and then did classification analysis for the postoperative mortality using three classifiers. The classification accuracies of GAN-LR, GAN-SVM, and GAN-RF were 0.97, 0.99, and 0.99, respectivel (Table 6).
Discussion
This study has two main highlights. (1) We compared the performance of machine learning models with a common statistic model (LR) and the performance of machine learning models were better. (2) We identified the key factors associated with surgical timing (<4 or ≥4 weeks) and postoperative survival for infected necrotizing pancreatitis and predicted the surgical timing by applying machine learning models.
An international survey shows that 55% of pancreatic specialists would wait for the effect of antibodies and postpone surgical management for the patients with infected pancreatic necrosis, whereas 45% of specialists would take an immediately action of surgical treatment after diagnosis (Abdelhafez et al., 2015). The time of operation varies greatly. Therefore, it is necessary to demonstrate if the patient with necrotizing pancreatitis needs early or delayed surgery individually. Previous studies using organ failure and infection as predictors of death obtained controversial results (Guo et al., 2013, 2014; Schepers et al., 2018). In our study, we assessed the impact of multiple clinical factors and comprehensive scores on surgical timing and postoperative mortality for the patients received the early or delayed surgery.
Early studies showed that the mortality of patients who received surgery within 2 weeks was much higher than that of surgery after 2 weeks (Besselink et al., 2007; Guo et al., 2013, 2014; Schepers et al., 2018), suggesting that early surgery should be conducted between 2 and 4 weeks. According to our analysis with multiple classifiers, IL-6, infected necrosis, onset of fever and CRP are important factors associated with the timing of surgery, which is consistent with the surgical indications used in clinic. The modified Marshall score is one of common factors used to assess patient mortality. Our analysis indicated that the mortality of the early surgery group was associated with the preoperative modified Marshall score and the modified Marshall score assessed at admission, suggesting that the modified Marshall score should be monitored in a real time for prediction. According to the Revised Atlanta Classification, organ failure is determined by modified Marshall score. The preoperative modified Marshall score was associated with the mortality after delayed surgery. Only two meaningful variables were obtained through stepwise regression of LR, including the preoperative modified Marshall score and circulatory failure. The preoperative modified Marshall score, the time of surgery, duration of organ failure and onset of renal failure were among of the top five important features selected by SVM and RF. A recent multicenter prospective cohort study reported that POF and multiple organ failure were the major determinants of AP severity, and the presence of infection was not associated with higher mortality (Sternby et al., 2017), consistent with our findings.
According to our knowledge, this is the first time to apply SVM and RF to predict the timing of surgery and postoperative mortality of patients with infected necrotizing pancreatitis. The classification performance of RF and SVM was better than LR. Especially when GAN was applied in the simulation, the accuracies were obviously improved. It is most likely because GAN can generate simulation samples with the same distribution as the actual samples, enhancing the sample size. In term of model classification performance, the classification accuracies of three models were high. Therefore, based on the patient's routine laboratory test and organ failure status, we can apply the classifiers to predict whether the patient should undergo early or delayed surgery individually to reduce patient mortality. Our classification results provide good references for clinicians to make personized surgical plans for patients with infected necrotizing pancreatitis.
However, there are some limitations of this study. Since the categorical variables cannot be applied to the traditional GAN, we changed the categorical variables into continuous variables and then put them into the GAN model. Although we have reached a conclusion consistent with Baowaly et al. by using our proposed GAN, we need to further verify with more samples.
In summary, we (1) applied a better machine learning model compared with a statistic model to predict the surgical timing (<4 or ≥4 weeks) in patients with infected necrotizing pancreatitis; (2) identified the key factors associated with surgical timing and postoperative survival for infected necrotizing pancreatitis and predicted the surgical timing by applying machine learning models; and (3) provided good references for clinicians in developing personalized surgical plans for patients with infected necrotizing pancreatitis.
Data Availability Statement
The datasets generated during and/or analyzed during the current study are available from the corresponding author on reasonable request.
Ethics Statement
This study was approved by the ethics review board of West China Hospital of Sichuan University, and the need for informed consent was waived owing to the retrospective nature of the study.
Author Contributions
Data collection: QG. Data analysis: LL and ZZha. Data interpretation: XY and HL. Writing of the manuscript: LL. Research conception: XZ and ZZho. Critical revision of the manuscript: WZ.
Funding
This work was supported by Sichuan Science and Technology Program (No. 2019YFS0147), 1·3·5 project for disciplines of excellence, West China Hospital, Sichuan University (No. ZYJC18010), 1·3·5 project for disciplines of excellence-Clinical Research Incubation Project, West China Hospital, Sichuan University (No. 2019HXFH022), and Post-Doctor Research Project, West China Hospital, Sichuan University (No. 2019HXBH039).
Conflict of Interest
The authors declare that the research was conducted in the absence of any commercial or financial relationships that could be construed as a potential conflict of interest.
Supplementary Material
The Supplementary Material for this article can be found online at: https://www.frontiersin.org/articles/10.3389/fbioe.2020.00541/full#supplementary-material
References
Abdelhafez, M., Andersson, R., Andren-Sandberg, A., Ashley, S., Baal, M. V., Baron, T., et al. (2015). Diagnostic strategy and timing of intervention in infected necrotizing pancreatitis: an international expert survey and case vignette study. HPB 18, 49–56. doi: 10.1111/hpb.12491
Association, O. U. G. S., Working, P. O. T. B., Association, O. S. O. G., Pancreatic, S. O. G. B., and UK Working Party on Acute Pancreatitis (2005). UK guidelines for the management of acute pancreatitis. Gut 54(Suppl. 3), i1–i9. doi: 10.1136/gut.2004.057026
Baowaly, M. K., Lin, C., Liu, C., and Chen, K. (2019). Synthesizing electronic health records using improved generative adversarial networks. J. Am. Med. Inform. Assoc. 26, 228–241. doi: 10.1093/jamia/ocy142
Besselink, M. G. H., Verwer, T. J., Schoenmaeckers, E. J. P., Erik, B., Ridwan, B. U., Visser, M. R., et al. (2007). Timing of surgical intervention in necrotizing pancreatitis. Arch. Surg. 142, 1194–1201. doi: 10.1001/archsurg.142.12.1194
Creswell, A., White, T., Dumoulin, V., Kai, A., Sengupta, B., and Bharath, A. A. (2017). Generative adversarial networks: an overview. IEEE Signal. Proc. Mag. 35, 53–65. doi: 10.1109/MSP.2017.2765202
Deshpande, A. (2013). Deep Learning Research Review Week 1: Generative Adversarial Nets. Available online at: https://adeshpande3.github.io/Deep-Learning-Research-Review-Week-1-Generative-Adversarial-Nets (accessed July 17, 2018).
Douzas, G., and Bacao, F. (2017). Self-Organizing Map Oversampling (SOMO) for imbalanced data set learning. Expert Syst. Appl. 82, 40–52. doi: 10.1016/j.eswa.2017.03.073
Fiore, U., Santis, A. D., Perla, F., Zanetti, P., and Palmieri, F. (2017). Using generative adversarial networks for improving classification effectiveness in credit card fraud detection. Inform. Sci. 479, 448–455. doi: 10.1016/j.ins.2017.12.030
Gomatos, I. P., Halloran, C. M., Ghaneh, P., Raraty, M. G., Polydoros, F., Evans, J. C., et al. (2015). Outcomes from minimal access retroperitoneal and open pancreatic necrosectomy in 394 patients with necrotizing pancreatitis. Ann. Surg. 263, 992–1001. doi: 10.1097/SLA.0000000000001407
Goodfellow, I. J., Pouget-Abadie, J., Mirza, M., Xu, B., Warde-Farley, D., Ozair, S., et al. (2014). “Generative adversarial nets,”in International Conference on Neural Information Processing Systems (Montreal, QC), 2672–2680.
Guo, Q., Li, A., Xia, Q., Liu, X., Tian, B., Mai, G., et al. (2013). The role of organ failure and infection in necrotizing pancreatitis: a prospective study. Ann. Surg. 259, 1201–1207. doi: 10.1097/SLA.0000000000000264
Guo, Q., Li, A., Xia, Q., Lu, H., Ke, N., Du, X., et al. (2014). Timing of intervention in necrotizing pancreatitis. J. Gastrointest. Surg. 18, 1770–1776. doi: 10.1007/s11605-014-2606-1
Le, N. Q., Ho, Q. T., and Ou, Y. Y. (2017). Incorporating deep learning with convolutional neural networks and position specific scoring matrices for identifying electron transport proteins. J. Comput. Chem. 38, 2000–2006. doi: 10.1002/jcc.24842
Le, N. Q. K. (2019). iN6-methylat (5-step): identifying DNA N6-methyladenine sites in rice genome using continuous bag of nucleobases via Chou's 5-step rule. Mol. Genet. Genomics 294, 1173–1182. doi: 10.1007/s00438-019-01570-y
Le, N. Q. K., Huynh, T., Yapp, E. K. Y., and Yeh, H. (2019a). Identification of clathrin proteins by incorporating hyperparameter optimization in deep learning and PSSM profiles. Comput. Meth. Prog. Biol. 177, 81–88. doi: 10.1016/j.cmpb.2019.05.016
Le, N. Q. K., Yapp, E. K. Y., Ho, Q., Nagasundaram, N., Ou, Y., and Yeh, H. (2019b). iEnhancer-5Step: identifying enhancers using hidden information of DNA sequences via Chou's 5-step rule and word embedding. Anal. Biochem. 571, 53–61. doi: 10.1016/j.ab.2019.02.017
Li, J., Monroe, W., Shi, T., Jean, S., Ritter, A., and Jurafsky, D. (2017). Adversarial learning for neural dialogue generation. arXViv [preprint]. arXiv:1701.06547.
Mowery, N. T., Bruns, B. R., MacNew, H. G., Agarwal, S., Enniss, T. M., Khan, M., et al. (2017). Surgical management of pancreatic necrosis: a practice management guideline from the Eastern Association for the Surgery of Trauma. J. Trauma Acute Care Surg. 83, 316–327. doi: 10.1097/TA.0000000000001510
Nature Medicine (2019). Digital Medicine. Available online at: https://www.nature.com/collections/egjifhdcih (accessed March 1, 2019).
Pascual, S., Bonafonte, A., and Serrà, J. (2017). SEGAN: Speech Enhancement Generative Adversarial Network. arXViv [preprint]. arXiv:1703.09452.
Pezzilli, R., Zerbi, A., Campra, D., Capurso, G., Golfieri, R., Arcidiacono, P. G., et al. (2015). Consensus guidelines on severe acute pancreatitis. Digest Liver Dis. 47, 532–543. doi: 10.1016/j.dld.2015.03.022
Santana, E., and Hotz, G. (2016). Learning a Driving Simulator. arXViv [preprint]. arXiv:1608.01230.
Schepers, N. J., Bakker, O. J., Besselink, M. G., Ahmed Ali, U., Bollen, T. L., Gooszen, H. G., et al. (2018). Impact of characteristics of organ failure and infected necrosis on mortality in necrotising pancreatitis. Gut 68:314657. doi: 10.1136/gutjnl-2017-314657
Shenvi, S., Gupta, R., Kang, M., Khullar, M., Rana, S. S., Singh, R., et al. (2016). Timing of surgical intervention in patients of infected necrotizing pancreatitis not responding to percutaneous catheter drainage. Pancreatology 16, 778–787. doi: 10.1016/j.pan.2016.08.006
Sternby, H., Bolado, F., Canaval-Zuleta, H. J., Marra-López, C., Hernando-Alonso, A. I., Del-Val-Antoñana, A., et al. (2017). Determinants of severity in acute pancreatitis: a nation-wide multicenter prospective cohort study. Pancreatology 17:S67. doi: 10.1016/j.pan.2017.05.212
Tenner, S., Baillie, J., DeWitt, J., and Vege, S. S. (2013). American College of Gastroenterology guideline: management of acute pancreatitis. Am. J. Gastroenterol. 108, 1400–1415. doi: 10.1038/ajg.2013.218
Van Grinsven, J., van Santvoort, H. C., Boermeester, M. A., Dejong, C. H., van Eijck, C. H., Fockens, P., et al. (2016). Timing of catheter drainage in infected necrotizing pancreatitis. Nat. Rev. Gastro Hepat, 13:306. doi: 10.1038/nrgastro.2016.23
Wang, K., Gou, C., Duan, Y., Lin, Y., Zheng, X., and Wang, F. Y. (2017). Generative adversarial networks: introduction and outlook. IEEE/CAA J. Automat. Sin. 4, 588–598. doi: 10.1109/JAS.2017.7510583
Working Group IAP/APA Acute Pancreatitis Guidelines, 2013~Working Group IAP/APA Acute Pancreatitis Guidelines. (2013). IAP/APA evidence-based guidelines for the management of acute pancreatitis. Pancreatology 13, e1–e15. doi: 10.1016/j.pan.2013.07.063
Keywords: classification, surgery, timing, machine learning, necrotizing pancreatitis
Citation: Lan L, Guo Q, Zhang Z, Zhao W, Yang X, Lu H, Zhou Z and Zhou X (2020) Classification of Infected Necrotizing Pancreatitis for Surgery Within or Beyond 4 Weeks Using Machine Learning. Front. Bioeng. Biotechnol. 8:541. doi: 10.3389/fbioe.2020.00541
Received: 02 March 2020; Accepted: 05 May 2020;
Published: 04 June 2020.
Edited by:
Shu Tao, UCLA Jonsson Comprehensive Cancer Center, United StatesReviewed by:
Khanh N. Q. Le, Taipei Medical University, TaiwanXimiao He, Huazhong University of Science and Technology, China
Copyright © 2020 Lan, Guo, Zhang, Zhao, Yang, Lu, Zhou and Zhou. This is an open-access article distributed under the terms of the Creative Commons Attribution License (CC BY). The use, distribution or reproduction in other forums is permitted, provided the original author(s) and the copyright owner(s) are credited and that the original publication in this journal is cited, in accordance with accepted academic practice. No use, distribution or reproduction is permitted which does not comply with these terms.
*Correspondence: Lan Lan, bGFubEBzY3UuZWR1LmNu