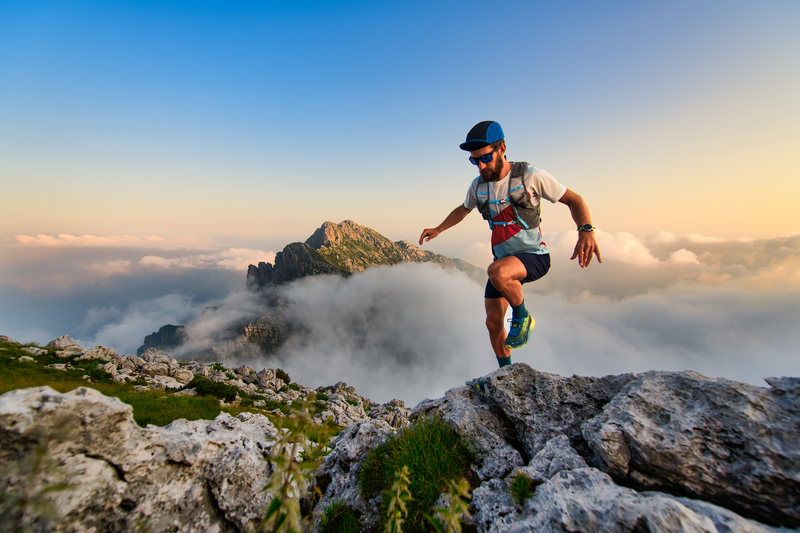
94% of researchers rate our articles as excellent or good
Learn more about the work of our research integrity team to safeguard the quality of each article we publish.
Find out more
ORIGINAL RESEARCH article
Front. Big Data
Sec. Data-driven Climate Sciences
Volume 8 - 2025 | doi: 10.3389/fdata.2025.1546223
This article is part of the Research Topic Air Quality and Biosphere-Atmosphere Interactions View all 4 articles
The final, formatted version of the article will be published soon.
You have multiple emails registered with Frontiers:
Please enter your email address:
If you already have an account, please login
You don't have a Frontiers account ? You can register here
The planet is experiencing global warming, with an increasing number of heat waves worldwide. Cities are particularly affected by the high temperatures because of the urban heat island (UHI) effect. This phenomenon is mostly explained by the land cover changes, reduced green spaces, and the concentration of infrastructure in urban settings. However, the reasons for the UHI are complex and involve multiple factors still understudied. Air pollution is one of them. This work investigates the link between particulate matter ≤2.5µm (PM 2.5 ) and air temperature by convergent cross-mapping (CCM), a statistical method to infer causation in dynamic nonlinear systems. A positive correlation between the concentration of fine particulate matter and urban temperature is observed. The causal relationship between PM 2.5 and temperature is confirmed in the most urbanized areas of the study site (Quito, Ecuador). The results show that (i) the UHI is present even in the most elevated capital city of the world, and (ii) air quality is an important contributor to the higher temperatures in urban than outlying areas. This study supports the hypothesis of a nonlinear threshold effect of pollution concentration on urban temperature.
Keywords: Air Quality, Urban temperature, causation, Statistical inferences, nonlinear effects
Received: 16 Dec 2024; Accepted: 27 Feb 2025.
Copyright: © 2025 Rybarczyk, Zalakeviciute, Ereminaite and Costa-Stolz. This is an open-access article distributed under the terms of the Creative Commons Attribution License (CC BY). The use, distribution or reproduction in other forums is permitted, provided the original author(s) or licensor are credited and that the original publication in this journal is cited, in accordance with accepted academic practice. No use, distribution or reproduction is permitted which does not comply with these terms.
* Correspondence:
Yves Philippe Rybarczyk, School of Information and Engineering, Dalarna University, Falun, 2829-516, Sweden
Disclaimer: All claims expressed in this article are solely those of the authors and do not necessarily represent those of their affiliated organizations, or those of the publisher, the editors and the reviewers. Any product that may be evaluated in this article or claim that may be made by its manufacturer is not guaranteed or endorsed by the publisher.
Research integrity at Frontiers
Learn more about the work of our research integrity team to safeguard the quality of each article we publish.