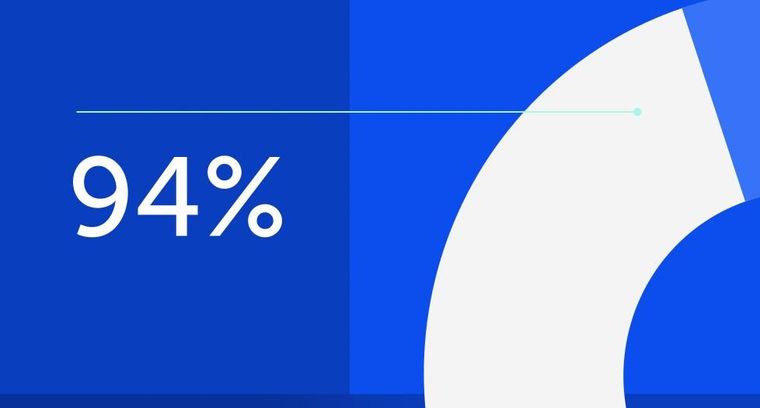
94% of researchers rate our articles as excellent or good
Learn more about the work of our research integrity team to safeguard the quality of each article we publish.
Find out more
ORIGINAL RESEARCH article
Front. Big Data
Sec. Cybersecurity and Privacy
Volume 8 - 2025 | doi: 10.3389/fdata.2025.1526480
The final, formatted version of the article will be published soon.
Select one of your emails
You have multiple emails registered with Frontiers:
Notify me on publication
Please enter your email address:
If you already have an account, please login
You don't have a Frontiers account ? You can register here
Intrusion detection has been of prime concern in the IoT environment due to the rapid increase in cyber threats. Most traditional Intrusion Detection Systems (IDSs) rely on centralized models, raising significant privacy concerns. Federated Learning (FL) offers a decentralized alternative; however, many existing FL-based IDS frameworks suffer from poor performance due to suboptimal model architectures and ineffective hyper-parameter selection. To address these challenges, this paper introduces a novel trust-centric FL framework for IDS based on the Tab Transformer model. We enhance the Tab model through an optimization process, utilizing a hyper-parameter tuning algorithm inspired by the nature-based Electric Eel Foraging Optimization (EEFO) algorithm. The goal of the developed framework is to improve the detection of IDS without using centralized data to preserve privacy. Whereas it enhances the processing and detection capability of the huge amounts of data generated from Internet of Things (IoT) devices. Our framework is tested on three IoT datasets: N-BaIoT, UNSW-NB15, and CICIoT2023 to ensure the performance of the model. Experimental results show that the proposed framework significantly exceeds traditional methods in terms of accuracy, precision, and recall. The results presented in this study confirm the effectiveness and superior performance of the proposed FL-based IDS framework.
Keywords: Federated learning, transformers, Intrusion detection system (IDS), cybersecurity, Internet of Things (IoT), optimization
Received: 11 Nov 2024; Accepted: 21 Apr 2025.
Copyright: © 2025 Fares, Abd Elaziz, Dahou and Shrahili. This is an open-access article distributed under the terms of the Creative Commons Attribution License (CC BY). The use, distribution or reproduction in other forums is permitted, provided the original author(s) or licensor are credited and that the original publication in this journal is cited, in accordance with accepted academic practice. No use, distribution or reproduction is permitted which does not comply with these terms.
* Correspondence: Ibrahim A. Fares, Faculty of Science, Zagazig University, Zagazig, Egypt
Disclaimer: All claims expressed in this article are solely those of the authors and do not necessarily represent those of their affiliated organizations, or those of the publisher, the editors and the reviewers. Any product that may be evaluated in this article or claim that may be made by its manufacturer is not guaranteed or endorsed by the publisher.
Supplementary Material
Research integrity at Frontiers
Learn more about the work of our research integrity team to safeguard the quality of each article we publish.